激光反射层析成像技术的研究进展
下载: 1819次内封面文章特邀综述
Significance Laser has been widely used in the production and living of human. In remote sensing detection field, using laser to detect targets is probably the most precise method for perceiving the appearance of long-range targets at present. This is because laser is characterized by its high brightness, high collimation, and strong coherence. It can actively, real timely, and precisely acquire the three-dimensional (3D) information of the detected targets.
Laser imaging detection is a target detecting method applying laser beams as detection media, which are radiated to illuminate targets in the first place according to certain spatial distribution law. Then, the data including time delay, intensity, waveform, phase, and polarization of laser echoes reflected from the detected targets are collected. After being processed, these data are presented in images. Finally, feature extraction and object inversion, to obtain the information of targets, including distance, position, reflection attribute, structure size, and motion feature, are conducted based on the images. By doing so, targets are found, identified, and confirmed. This technology can provide the information, including the range images, gray images, and feature images of targets with high resolution that cannot be obtained using general imaging methods. In addition, the laser imaging detection, characterized by high-resolution, high-measurement accuracy, anti-interference ability, and strong anti-shadowing ability, is especially suitable for the detailed detection of targets.
Due to its many advantages such as high spatial resolution, strong anti-interference and so on, laser imaging has attracted more and more attentions in the field of space object surveillance and identification. One of the most promising laser imaging methods is the laser reflective tomography imaging, introduced by Parker, Knight, and Matson et al. According to the provided signature, it can be divided into range-resolved, Doppler-resolved and angle-angle-resolved. Range-resolved reflective tomography imaging technique can be used to obtain cross-sectional image of objects that is angularly unresolved while the data are range resolved, so it is especially applicable for an object that is not rotating fast enough. Meanwhile, it can be realized in both coherent and incoherent detection systems, considering that the Doppler-resolved and angle-angle resolved can only be used in coherent detection systems. When it is realized in incoherent detection systems, it has the characteristic that its spatial resolution is not related with the imaging distance but related with laser pulse-width, bandwidth of detectors and noise, and is also insensitive to turbulence.
According to the published documents, various research institutes at home and aboard, have taken studies on the laser reflective tomography imaging, mainly including Massachusetts Institute of technology Lincoln Laboratory, U.S. Air Force Phillips Laboratory, Swedish Defense Research Agency, Shanghai Institute of Optics and Fine Mechanics, National University of Defense Technology and Space Engineering University. Especially, Charles L. Matson from Air Force Phillips Laboratory, has conducted the first satellite feature reconstruction by use of range-resolved reflective tomography techniques from non-imaging laser radar data collected on an orbiting satellite. Many corresponding advances have been achieved. But there are still many problems need to be solved in terms of practicality and the improvement of reconstructed images quality. Hence, it is necessary to summarize the existing researches to guide the future development of this field more rationally.
Progress The progress of methods, and research development in the field of laser reflective tomography imaging techniques are summarized. First, the requirement of projection sampling, the resolution of reconstructed images, signal to noise ratio calculation, the projection type which can be used to reconstruct image, and the application mode of are introduced. Second, the processing methods of projections are summarized according to previously reported studies. Considering the effects on the reconstructed image, the processing of projection can be divided into projection registration and projection de-convolution. Xiaofeng Jin's research group from Shanghai Institute of Optics and Fine Mechanics, Chinese Academy of Sciences, has studied on and putted forward feature tracking method for projection registration (
Conclusion and Prospect Laser imaging is of great importance for long-range space target detection. In order to well applicated in space target detection, the technology of laser reflective tomography imaging still needs in-depth, engineering and practical explorations to promote the development of the long-range laser imaging detection technology in engineering aspects.
1 引言
计算机层析成像(CT)是通过测量不同方向上物理量的空间分布并从测量数据中计算出非叠加图像的一种数字成像手段[1]。Parker等[2]在激光雷达测量中运用层析成像技术以及基于光电倍增管和瞬时数字化仪的激光雷达实验系统,实现了对圆锥目标的距离分辨激光反射层析成像;Knight等[3-4]最先采用基于条纹相机的激光雷达实验系统,对圆锥目标、卫星模型等进行了距离分辨激光反射层析成像实验;Marino等[5]根据反射层析成像投影数据采集形式,将激光反射层析成像分为角度-距离分辨、角度-多普勒分辨、角度-角度分辨三种,指出典型的距离分辨测量既可以采用相干探测系统,也可以采用非相干探测系统,并开展了距离分辨和多普勒分辨的激光反射层析成像仿真验证和实验验证。Knight等[6-7]开展了基于角度-角度分辨的激光反射层析成像室内实验。激光反射层析成像的空间分辨率与成像距离无关,取决于激光的脉冲宽度、探测器的带宽和噪声,且不受传统成像系统中孔径大小的影响[8], Matson等[9-10]将其用于对卫星等空间目标的成像探测中。
基于反射层析成像在空间远距离高分辨率成像方面的技术优势[11-12],美国林肯实验室、空军实验室、Areté公司、瑞典防务研究局、上海光机所、上海技物所、国防科技大学以及航天工程大学等单位均对层析成像的基础理论问题、投影数据处理方法、图像重建算法、探测成像实验系统等方面开展了系统性研究,并进行了实验验证。本文首先回顾了激光反射层析成像技术的发展,然后阐述了已取得的阶段性研究成果和应用,最后探讨了反射层析成像面临的挑战和未来研究工作的重点。
2 激光反射层析成像的原理
激光反射层析成像[2]是在层析成像基础上发展而来的,层析成像是通过对物体进行不同角度的射线投影测量获取物体横截面信息的成像技术[13]。层析成像和激光反射层析成像的原理如
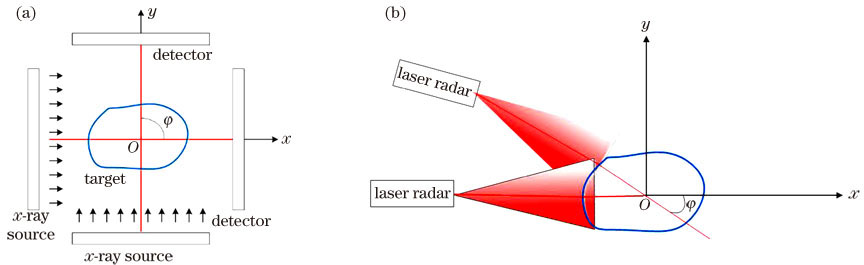
图 1. 计算机层析成像与激光反射层析成像原理图。(a)计算机层析成像;(b)激光反射层析成像
Fig. 1. Schematic diagrams of computerized tomography and laser reflection tomography. (a) Computerized tomography; (b) laser reflection tomography
定义p(r,φ)为横截面吸收系数g(x,y)在角度φ上的投影,r为空间距离,(x,y)为某点吸收系数处的空间位置坐标。层析成像的原理是傅里叶切片定理[14],即投影数据的一维傅里叶变换F1
由(1)式可知,当物体所有方向的投影数据已知时,可重建出物体的g
式中,φi为第i次的观测角度,Δφ为两次观测角度间的角度增量,
在激光反射层析成像中,g(x,y)为物体横截面反射系数分布函数,投影数据为单探测器获得的不同视角激光反射回波信号经离散采样后的离散数据,重建的图像是目标物体横截面二维轮廓像。在实际成像过程中,激光反射层析成像的结果为通过(2)式重建的图像,研究表明,由完全投影数据重建的图像是唯一的。获取完全投影数据,需要保证足够多的投影采样点,并保证经频域变换得到的目标图像完整、精准,因此,需要研究精确重建图像时的投影采样角度数和单投影的采样点数。其次,根据激光反射层析成像的特点,重建图像的分辨率仅取决于激光脉冲的宽度、探测器的带宽和噪声,信噪比的大小决定了重建图像的质量,因此,需要求解投影数据的信噪比甚至重建图像的信噪比。激光反射层析成像可在相干探测系统和非相干探测系统中实现,因此采用哪种投影数据重建图像以及如何提高重建图像的质量也是需要解决的问题。
3 激光反射层析成像的研究现状
3.1 成像基础理论问题
激光反射层析成像基础理论问题的研究主要集中在精确重建图像时的投影数据采样[15-16]、重建图像的分辨率[17-18]、信噪比的计算[15,19-21]、重建图像的投影数据类型[21-22]以及激光反射层析成像的应用方式[23-26]等。
Matson等[15-16]根据傅里叶切片定理,给出了精确重建图像对投影数据在角度和时间维的采样要求,并给出了角度采样间隔、投影角度数据量和单个角度下的采样数据长度等参数。反射层析成像的分辨率取决于脉冲宽度、探测器带宽以及噪声,在分析重建图像的分辨率时,需考虑投影数据和重建图像的信噪比。Matson等[15,19-21]给出了基于相干探测系统的回波信号电场表达式,并基于此建立了回波强度的计算公式,进而推导出考虑投影数据波形结构的投影数据信噪比以及基于投影数据重建图像的信噪比表达式。在反射层析成像中,投影数据既可以是电场数据如合成孔径雷达数据,也可以是强度数据如X射线。Matson[21-22]推导了将相干探测系统的激光回波信号转换为所需投影数据和图像数据的方程,并在此基础上建立了基于激光散斑噪声的重建图像信噪比表达式。理论结果表明,强度投影法比电场投影法得到的图像信噪比更高,模拟和室内实验的结果也表明,强度投影数据重建的图像质量明显优于电场信号重建出的图像。上述研究均通过直接观察判断图像的质量,Jin等[17]推导出滤波反投影重建算法下的点扩展函数,用距离分辨率取代基于瑞利准则的理论图像分辨率,模拟仿真单点目标在不同角度的成像结果,并验证了字母E在有限采样角度下的重建效果,如
![不同角度下重建的单点目标图像。(a) 15°;(b) 30°;(c) 45°;(d) 60°;(e) 90°;(f) 360°[17]](/richHtml/zgjg/2021/48/4/0401002/img_2.jpg)
图 2. 不同角度下重建的单点目标图像。(a) 15°;(b) 30°;(c) 45°;(d) 60°;(e) 90°;(f) 360°[17]
Fig. 2. Single-point target images reconstructed from different angles. (a) 15°; (b) 30°; (c) 45°; (d) 60°; (e) 90°; (f) 360°[17]
![基于滤波反投影算法重建的字母E。(a) 45°;(b) 90°;(c) 360°[17]](/richHtml/zgjg/2021/48/4/0401002/img_3.jpg)
图 3. 基于滤波反投影算法重建的字母E。(a) 45°;(b) 90°;(c) 360°[17]
Fig. 3. Letter E reconstructed based on the filtered back projection algorithm. (a) 45°; (b) 90°; (c) 360°[17]
![三个点目标在不同角度误差下重建的图像。(a)原始图像;(b)无误差图像;(c)高斯随机误差为(0,1);(d)高斯随机误差为(0,3);(e)高斯随机误差为(0,5);(f)高斯随机误差为(0,7)[18]](/richHtml/zgjg/2021/48/4/0401002/img_4.jpg)
图 4. 三个点目标在不同角度误差下重建的图像。(a)原始图像;(b)无误差图像;(c)高斯随机误差为(0,1);(d)高斯随机误差为(0,3);(e)高斯随机误差为(0,5);(f)高斯随机误差为(0,7)[18]
Fig. 4. Reconstructed images of three point targets under different angle errors. (a) Original image; (b) error-free image; (c) Gaussian random error is (0,1); (d) Gaussian random error is (0,3); (e) Gaussian random error is (0,5);(f) Gaussian random error is (0,7)[18]
![不同角度范围内三个点目标的仿真结果。(a)180°;(b)150°;(c)120°;(d)90°;(f)60°[18]](/richHtml/zgjg/2021/48/4/0401002/img_5.jpg)
图 5. 不同角度范围内三个点目标的仿真结果。(a)180°;(b)150°;(c)120°;(d)90°;(f)60°[18]
Fig. 5. Simulation results of three-point targets in different angle ranges. (a) 180°; (b) 150°; (c) 120°; (d) 90°; (f) 60°[18]
Qu等[23]探讨了基于正则化的地基对天基卫星目标图像重建方法,但地基对天基成像需要克服大气影响,且目标反射回波接收和投影数据处理难度大。因此,在仿真分析基于啁啾脉冲信号反射层析激光雷达成像处理[24]的基础上,又探讨了将其应用于星对星探测的可行性[26]。严毅等[25]将反射层析非相干处理方法与合成孔径激光雷达相结合,提出了聚束非相干合成孔径激光成像方法,采用侧视观察模式获取目标的角度-距离-强度信息,实现对二维平面目标的成像,结果如
![不同角度范围内的投影重建图像。(a)10°;(b)30°;(c)60°;(d)90°;(e)180°;(f)360°[25]](/richHtml/zgjg/2021/48/4/0401002/img_6.jpg)
图 6. 不同角度范围内的投影重建图像。(a)10°;(b)30°;(c)60°;(d)90°;(e)180°;(f)360°[25]
Fig. 6. Projection reconstructed images in different angle ranges. (a) 10°; (b) 30°; (c) 60°; (d) 90°; (e) 180°; (f) 360°[25]
综上所述,在相干探测系统中实现距离分辨激光反射层析成像,采集强度投影数据重建图像是最佳选择;同时,重建图像的质量与投影角度范围以及投影数据量密切相关,且星对星激光成像和聚束非相干合成孔径激光成像两种反射层析成像的应用中,均存在着投影角度范围过小导致的重建目标图像模糊和畸变等问题,因此需要研究有限角度和不完备投影数据情况下的图像高质量重建。
3.2 投影数据处理方法
3.2.1 投影数据配准方法
探测运动目标特别是空间目标时,平台随机抖动和目标在其他方向的随机运动,导致不同投影角度下旋转中心不再对准在同一直线下,基于未校准投影数据的成像结果会发生错位和畸变[27]。物体图像的重建需要投影数据的正确配准,常用相位恢复算法进行投影数据配准,经典的相位恢复算法是Gerchberg等[28]提出的G-S(Gerchberg-Saxton)算法,基本思想是根据衍射强度分布与目标振幅分布函数,在空域与频域间反复进行快速傅里叶变换及其逆变换,迭代恢复出目标相位信息。为提高相位恢复算法的收敛速度和精度,人们又相继提出了误差减小算法[29]、混合输入输出算法[30]、最速下降算法[31]、共轭梯度算法[31]、随机振幅调制算法[32]、顾-杨算法[33]等。Ford等[34]最早将相位恢复算法应用于距离分辨激光反射层析成像中,提出了基于混合输入输出算法的投影数据配准算法。但该算法需要增加傅里叶相位约束条件才能对实际数据有效,对迭代算法和迭代规则敏感,主要针对透射投影数据进行配准。Jin等[35]提出了基于误差减小算法和混合输入输出算法相结合的投影数据配准方法,并研究了不同矩阵作为相位恢复初始矩阵对相位恢复精度的影响,结果表明,初始矩阵为平移投影反重建图像、常数矩阵时相位恢复图像的质量更好。赵楠翔等[36]提出了基于模值加权相位恢复算法的投影数据配准方法,首先利用原始投影数据重建出目标原始待复原图像,再将目标原始待复原图像的频域模值与本次迭代产生的模值进行混合加权,得到约束后的替换矩阵为
式中,Gp(u,v)为频域限制后的替换矩阵,G0(u,v)为初始图像的频谱矩阵,Gk(u,v)为k次迭代后的频谱矩阵,α为混合加权参数,可提高相位恢复效果与算法收敛的精度。按
虽然相位恢复算法在收敛速度和恢复精度方面有所改善,但其时域、频域上需要进行大量迭代运算和参数优化,相位恢复性能依赖选取的初始矩阵[37],从极坐标系转换到笛卡儿坐标系的插值处理会引入噪声等问题。因此,Jin等[38-39]提出了投影数据配准的特征点跟踪法,将投影数据中峰值或谷值看作特征点,通过跟踪特征点位置实现相邻投影数据的配准,配准前后的投影数据如
![配准前后的投影数据。(a)未配准的投影数据;(b)配准后的投影数据[38-39]](/richHtml/zgjg/2021/48/4/0401002/img_8.jpg)
图 8. 配准前后的投影数据。(a)未配准的投影数据;(b)配准后的投影数据[38-39]
Fig. 8. Projection data before and after registration. (a) Unregistered projection data; (b) registered projection data [38-39]
在投影数据配准处理方面,基于相位恢复算法和基于跟踪投影数据特征算法各有所长。相位恢复算法的限制条件较少,但计算量大且运行速度慢;跟踪投影数据特征算法计算量较小且运行速度快,但需要预先获取成像目标一定的先验信息。
3.2.2 投影数据解卷积方法
滤波反投影重建首先对投影数据进行离散傅里叶变换,然后与自定义的滤波器相乘,再对调整后的频率进行傅里叶逆变换,最后将调整后的投影数据进行反投影,即可重建出目标图像g
式中,
在滤波反投影重建中,对投影数据进行滤波处理可以改善重建图像的分辨率,但滤波器的主瓣高度和旁瓣衰减也会影响重建图像的空间分辨率[41];同时重建图像的分辨率也与脉冲宽度相关,脉冲越窄,重建图像的质量越好[42]。因此在激光脉冲宽度较大时,还需进行解卷积处理以提高重建图像分辨率[43]。Wang等[44]提出了基于贝叶斯的迭代最大似然估计解卷积方法,在贝叶斯理论基础上对待估计参数进行最大似然估计,采用斯特林渐进逼近解估计量的概率显式表达,再利用共轭梯度法求解最优估计量,可表示为
式中,Wj为第j个观测角度下采集到的激光回波波形,
谷雨等[45]提出了基于变分贝叶斯的非盲解卷积方法,假设反射分布投影J为服从高斯分布
式中,q(μ)为均值的先验概率分布,q(τ)为精度的先验概率分布,q*μ(μ)为均值的最优先验概率分布,μ0和λ0分别为高斯分布的超先验参数,a0和b0分别为Gamma分布的超先验参数,Eτ(τ)为均值的数学期望,N为采集的投影分布数据组数,q*τ(τ)为精度的最优先验概率分布。
上述两种方法均为非盲解卷积,需要一定先验信息即已知发射激光脉冲波形。林放等[46]提出多帧迭代的盲解卷积方法,通过观测回波数据,同时估计目标反射系数投影分布和入射脉冲波形信息,利用多角度测量的公约数性质,对估计结果进行迭代优化,得到相对精确的目标投影数据估计,算法流程如
上述三种方法均需要大量迭代优化计算,耗费时间较多。因此,Shi等[47]提出了一种基于基准回波脉冲的投影数据解卷积处理方法,基本思想是利用垂直照射某剖面的回波脉冲,经过一系列处理后作为基准脉冲,通过频域的傅里叶逆变换实现一维信号的时域解卷积。该方法无需大量迭代计算,但仅对具有对称形状的目标有效。
3.3 目标图像重建算法
拉冬-傅里叶变换是层析成像的基础成像算法,但投影数据测量范围为180°,而反射层析成像需要360°范围的投影数据。基于反射投影与投射投影的相似性[7],Jin等[48]提出了一种基于改进拉冬-傅里叶变换的反射层析成像算法,若相邻投影数据p(r,φ)和p(r,φ+180°)分别为目标不同表面的反射特征,将p(r,φ)和p(r,φ+180°)T叠加,可得到类似透射投影的投影数据,再进行拉冬-傅里叶变换即可重建出目标图像,可表示为
式中,T为轴对换,表示回波波形关于旋转中心的对称,Q为反射层析对应的透射投影频域变换,G为傅里叶切片定理给出的傅里叶域估计,u和v为变换或频率变量,K表示K轴,为投影数据p(r,φ)一维傅里叶变化后坐标系的横轴。拉冬-傅里叶变换成像法虽然简单易行,但精度较低、计算量较大,且重建图像有明显的伪影。因此滤波反投影算法[7, 49-50]成为激光反射层析成像最常用的标准算法,常用的滤波器函数有Shepp-Logan、Ram-Lak、Hanning以及Hamming等[43]。虽然滤波反投影算法重建出的图像分辨率较高,但反投影过程中需要平方、开方计算重建图像坐标点到光源点的距离,随着投影采样角度数的增加或重建图像像元间隔的减小,计算量会逐渐增大,从而降低图像重建的效率。金晓峰等[51]提出了一种计算重建图像坐标点到光源点距离的折线反投影算法,以重建图像坐标点到直线的距离替代图像坐标点到光源点的距离,避免了平方、开方计算,有效降低了计算复杂度。但傅里叶切片成像算法、滤波反投影算法以及折线反投影算法都存在抑制噪声能力差、重建图像边缘模糊等问题。
Yang等[52-53]研究了基于滤波反投影算法重构图像时投影角度间隔较大和角度不完备等问题,发现随投影角度间隔的增大和采样角度的减小,重建图像的质量逐渐下降,但采样角度对重建图像质量的影响更大。陈剑彪等[37]的研究发现,滤波反投影算法重建目标图像时,投影角度间隔特别大时会导致投影数据稀疏,重建出的目标图像边缘轮廓被弱化,很难辨识目标的原始形状。因此,在较大投影角度间隔和不完备角度下,滤波反投影重建算法并不是最佳方法。
杨彪等[54]将代数迭代重建(ART)算法应用于激光反射层析图像重建中,首先将待重建图像区域划分为n×n个相等的小正方形,令每个像素内部的灰度值f(x,y)为常数,
![三棱柱的重建结果。(a)直接反投影;(b)R-L滤波反投影;(c)S-L滤波反投影;(d)ART算法[54]](/richHtml/zgjg/2021/48/4/0401002/img_10.jpg)
图 10. 三棱柱的重建结果。(a)直接反投影;(b)R-L滤波反投影;(c)S-L滤波反投影;(d)ART算法[54]
Fig. 10. Reconstruction result of a triangular prism. (a) Direct back projection; (b) R-L filtered back projection; (c) S-L filtered back projection; (d) ART algorithm[54]
激光反射层析成像主要对非合作目标成像,通常难以获取360°全角度采样投影数据。此外,成像目标与成像系统之间相对旋转较快,还会导致激光对目标物体的扫描照射间隔角度较大,即使达到完备投影角度,也会出现投影间隔角度过大导致的投影角度欠采样,获取的投影数据为完备角度稀疏采样的投影数据。有限角度投影数据和完备角度稀疏采样投影数据可统称为不完全投影数据,因此不完全投影数据是激光反射层析成像投影数据的主要形式。目标图像重建算法的关键在于根据激光反射层析成像的应用场景,研究出面向不完全投影数据的图像重建算法。
3.4 探测成像实验系统
激光反射层析成像的研究主要集中在距离分辨激光反射层析成像,对多普勒分辨激光反射层析成像,只能利用相干探测系统,如美国林肯实验室的Firepond相干激光雷达[56]和上海光机所的相干外差探测激光成像雷达[57]。对于距离分辨激光反射层析成像,既可利用相干探测系统,如Firepond相干激光雷达、美国空军实验室的HI-CLASS相干激光雷达[58]和相干成像激光测试平台(HILT)[59];也可利用非相干直接探测系统,如林肯实验室的激光反射层析成像系统[7]、瑞典防务研究局的TPSPC激光雷达[60]、上海光机所的直接探测激光反射层析成像雷达[27]和上海技物所的直接探测反射层析成像系统[61]。
表 1. 激光反射层析成像实验系统的主要参数
Table 1. Main parameters of experiment systems using in laser reflective tomography imaging
|
3.5 成像实验研究
国外的美国林肯实验室、空军实验室、Areté公司、瑞典**研究局以及国内的上海光机所、国防科技大学等单位相继开展了光反射层析成像外场短距离实验、真实卫星目标远距离实验以及实验室内验证实验。
3.5.1 距离分辨反射层析成像实验
针对镜面目标或漫反射目标的实验室内实验和外场实验,Parker等[2-4]利用基于光电倍增管和瞬时数字化仪的非相干激光雷达实验系统,对尺寸为170 cm×53 cm(170 cm为圆锥体的轴向长度,53 cm为圆锥体底部圆的直径)的圆锥体进行了成像实验。实验使用的激光脉冲宽度为100 ps,运用滤波反投影算法重建出如
![不同分辨率的圆锥目标重建图像。(a)低分辨率重建图像;(b)高分辨率重建图像[2-4]](/richHtml/zgjg/2021/48/4/0401002/img_11.jpg)
图 11. 不同分辨率的圆锥目标重建图像。(a)低分辨率重建图像;(b)高分辨率重建图像[2-4]
Fig. 11. Reconstructed images of aluminum cone in different resolution.(a) Low-resolution reconstructed image; (b) high-resolution reconstructed image[2-4]
空军实验室开展了基于相干激光探测系统的直接或相干探测距离分辨激光反射层析成像有效性实验验证。Matson等[9-10, 62]利用HI-CLASS相干探测激光雷达系统[58](高性能CO2激光雷达监视传感器),实现了对在轨卫星特征物体的重构成像,重构成像的特征物体是LACE卫星本体和伸缩臂上的两个锗角反射镜,成像时激光的脉冲宽度为1.5 ns。地基激光雷达观测天基目标时的观测角度范围有限,导致重建出的图像质量较差,伪影较多,目标形状轮廓不明显。
![镜面目标反射实验中LACE卫星锗角反射镜重建图像。(a)原始图像;(b)阈值化处理后图像[9-10, 62]](/richHtml/zgjg/2021/48/4/0401002/img_12.jpg)
图 12. 镜面目标反射实验中LACE卫星锗角反射镜重建图像。(a)原始图像;(b)阈值化处理后图像[9-10, 62]
Fig. 12. Reconstruction images of LACE satellite germanium retro-reflectors in specular target experiment. (a) Original image; (b) image after thresholding[9-10, 62]
Hanes等[8, 59, 63]利用HILT开展了针对漫反射目标的地面激光反射层析成像技术实验验证,对
![漫反射目标实验中卫星模型及重建图像。(a)卫星目标模型;(b)重建图像[8, 59, 63]](/richHtml/zgjg/2021/48/4/0401002/img_13.jpg)
图 13. 漫反射目标实验中卫星模型及重建图像。(a)卫星目标模型;(b)重建图像[8, 59, 63]
Fig. 13. Satellite model and its reconstructed image in diffused target experiment. (a) Satellite target model;(b) reconstructed image[8,59,63]
Murray等[64]利用对发射激光脉冲进行编码和回波解码的孔径编码非相干探测激光雷达,开展了基于脉冲压缩的距离分辨激光反射层析成像外场实验,探测距离为22.4 km,激光雷达采集放置在高为304.8 m、直径为1 m的多个匀速旋转角反射器的多组回波,重建的目标图像分辨率达到15 cm,实验场景及目标重建结果如
![远距离慢速旋转目标反射层析实验装置。(a)实验装置;(b)目标的二维拉冬变化图像;(c)重建图像[64]](/richHtml/zgjg/2021/48/4/0401002/img_14.jpg)
图 14. 远距离慢速旋转目标反射层析实验装置。(a)实验装置;(b)目标的二维拉冬变化图像;(c)重建图像[64]
Fig. 14. Long-distance and slow-rotating target reflection tomography experimental device. (a) Experimental device; (b) two-dimensional Ladon change image of the target; (c) reconstructed image[64]
在林肯实验室100 ps激光脉冲直接成像的基础上,Sun等[39, 65-66]开展了更窄脉冲宽度的直接探测激光反射层析成像实验室内的实验,实验中激光脉冲宽度为8 ps,分别采用反投影、滤波反投影和代数重建算法进行图像重建,结果如
![不同算法重建出的目标二维轮廓像。(a)反投影算法;(b)滤波反投影算法[39]](/richHtml/zgjg/2021/48/4/0401002/img_15.jpg)
图 15. 不同算法重建出的目标二维轮廓像。(a)反投影算法;(b)滤波反投影算法[39]
Fig. 15. Two-dimensional contour images of the target reconstructed by different algorithms. (a) Back projection algorithm; (b) filter back projection algorithm[39]
![代数重建算法重建的目标二维平面像。(a)5次迭代;(b)20次迭代[65]](/richHtml/zgjg/2021/48/4/0401002/img_16.jpg)
图 16. 代数重建算法重建的目标二维平面像。(a)5次迭代;(b)20次迭代[65]
Fig. 16. Two-dimensional plane image of the target reconstructed by the algebraic reconstruction algorithm. (a) 5 iterations; (b) 20 iterations[65]
![小船目标模型及重建图像。(a)小船模型;(b)去除伪影后的小船模型重建图像[60]](/richHtml/zgjg/2021/48/4/0401002/img_17.jpg)
图 17. 小船目标模型及重建图像。(a)小船模型;(b)去除伪影后的小船模型重建图像[60]
Fig. 17. Small boat target model and reconstructed image. (a) Small boat model; (b) reconstructed image of boat model after removing artifacts[60]
考虑到激光反射层析成像的工程应用实际情况,Wang等[44-45]进行了基于6.5 ns激光脉冲的距离分辨激光反射层析成像实验,在实验距离为40 m时对
![卫星模型及其重建图像。(a)卫星模型;(b)处理前的重建图像;(c)处理后的重建图像[44-45]](/richHtml/zgjg/2021/48/4/0401002/img_18.jpg)
图 18. 卫星模型及其重建图像。(a)卫星模型;(b)处理前的重建图像;(c)处理后的重建图像[44-45]
Fig. 18. Satellite model and reconstructed image. (a) Satellite model; (b) reconstructed image before processing;(c) reconstructed image after processing[44-45]
3.5.2 多普勒分辨反射层析成像实验
Murray等[64]验证了对慢速旋转目标进行成像的可行性,但对高速旋转目标进行成像时,需采用多普勒分辨激光反射层析成像[27]。Marino等[5, 7]利用Firepond二氧化碳相干激光雷达,开展了多普勒分辨激光反射层析成像实验,利用匹配滤波、拉冬-傅里叶变换以及反投影等成像算法,实现了5.4 km处的圆锥目标和火箭模型二维截面局部细节(尖角或不连续点)的图像重建,火箭模型成像结果如
![火箭成像模型及其重建图像。(a)火箭模型;(b)火箭模型的多普勒分辨数据;(c)火箭模型的重建图像[5, 7]](/richHtml/zgjg/2021/48/4/0401002/img_19.jpg)
图 19. 火箭成像模型及其重建图像。(a)火箭模型;(b)火箭模型的多普勒分辨数据;(c)火箭模型的重建图像[5, 7]
Fig. 19. Rocket imaging model and its reconstructed image. (a) Rocket model; (b) Doppler resolution data of the rocket model; (c) reconstructed image of the rocket model[5, 7]
金晓峰等[57]开展了多普勒分辨激光反射层析成像的实验室内实验,对距离8.9 m处旋转角速度为6/5π的三棱柱进行成像,并用滤波反投影成像算法对
![调整后角度-多普勒投影图及重建的目标图像。(a)调整后的角度-多普勒投影图;(b)目标重建图像[57]](/richHtml/zgjg/2021/48/4/0401002/img_20.jpg)
图 20. 调整后角度-多普勒投影图及重建的目标图像。(a)调整后的角度-多普勒投影图;(b)目标重建图像[57]
Fig. 20. Modified angle-Doppler projections and its reconstructed image. (a) Modified angle-Doppler projections; (b) reconstructed image of the target[57]
综上所述,利用激光反射层析成像实验系统已开展了实验室内和外场的短距离水平照射激光反射层析成像实验;利用现有地基激光相干探测系统,也成功进行了空间卫星目标的数百公里级激光反射层析成像实验,充分验证了距离分辨激光反射层析成像在远距离成像中潜在的应用价值。但成像中多采用滤波反投影成像算法,当观测角度范围和投影角度受限时,重建图像存在着模糊和畸变等问题,降低了反射层析成像重建图像的质量。多普勒分辨激光反射层析成像虽然与距离分辨激光反射层析成像方法相同,但在获取远距离探测的投影数据时,需突破解多普勒模糊、远距离微弱回波高灵敏度接收以及投影数据变换处理等难题,技术难度相当复杂,目前还难以开展数百公里距离的外场实验。
4 结论
激光反射层析成像技术具有空间分辨率与距离无关,图像分辨率仅取决于激光脉冲宽度、探测器带宽和噪声,且不受传统成像系统中的孔径大小影响等特点,在远距离空间目标激光探测、天基对天基激光成像探测方面具有潜在应用价值。但面向未来应用场景,还需要在以下方面进行研究。
1) 开展激光反射层析与其他激光成像探测方法融合研究,扩展激光反射层析重建图像的应用范围。激光反射层析成像获取的是目标二维轮廓像,无法反映目标结构、层次等特征,因此需要将激光反射层析成像与其他激光探测方法融合,获取更丰富的目标图像。文献[ 25]将激光反射层析处理算法与合成孔径激光雷达技术相融合,得到聚束非相干合成孔径激光成像方法,获得目标的二维平面像,应用价值更高。
2) 发展效率和精度兼顾、简单易行的投影数据处理方法,解决影响其面向实际应用的关键问题。在投影数据配准方面,重点研究从投影数据本身提取确定旋转中心,简单易行,兼顾配准速度和精度的投影数据配准算法;在投影数据解卷积方面,重点由非盲解卷积向盲解卷积方向发展。压缩盲解卷积[67-69]方法是投影数据盲解卷积的潜在方向。
3) 运用新的图像重建算法和图像后处理方法提升重建图像质量,解决影响重建图像质量的核心问题。压缩感知(CS)理论[70-72]可为不完全投影数据重建图像提供新思路。根据CS理论,在图像迭代重建增加关于稀疏变换后图像的稀疏性约束,可解决视角少不完备投影数据的精确重建问题[73]。CS理论已在医学成像中得到深入研究[74-85],激光反射层析成像图像重建中也可应用CS理论。面向不完备投影数据重建,结合CS和图像盲解卷积技术[86-87],可提高重建图像的质量,实现高分辨率的图像重建。此外,文献[ 88-90]提出了基于深度学习的有限角度投影数据图像重建算法,先补齐投影数据再进行图像重建,有效提高了不完备投影数据重建图像的质量,为激光反射层析成像中有限角度投影数据的重建图像问题提供了参考。
4) 升级改善系统硬件能有效提高激光反射层析成像性能。激光反射层析成像是为实现远距离目标高精度探测而发展的,需要大功率稳定的激光光源、窄脉宽的激光器、高灵敏度高速大带宽的光电探测器和高速度高深度的激光回波信号采集存储设备等。系统硬件的改善升级能有效提高原始投影数据质量,进而提升重建图像的空间分辨率和质量。
5) 构建设计实用化激光反射层析成像系统,推动激光反射层析成像技术走向实际应用。距离分辨激光反射层析成像虽已开展数百公里外场实验,但均基于现有成熟的地基相干探测系统。围绕激光反射层析成像在星对星远距离成像的应用,在解决投影数据处理方法和图像重建算法基础上,构建设计适用于空间应用的实用化工程系统,促进激光反射层析成像技术的应用,进而反哺并推动激光反射层析成像技术的发展。
6) 突破远距离多普勒分辨激光反射层析成像关键技术,拓展激光反射层析成像的应用范围。对高速旋转目标进行成像探测,多普勒分辨激光反射层析成像是目前潜在的可用方法。因此,需要在近距离多普勒分辨激光反射层析成像的研究基础上,围绕着制约远距离成像的关键因素,重点突破解多普勒模糊、远距离微弱回波高灵敏度接收以及投影数据变换处理等核心技术。
[1] Kalender WA. 计算机层析成像基本原理、系统技术、图像质量及应用[M]. 许州, 陈浩, 王远, 译. 3版. 北京: 清华大学出版社, 2016: 298.
Kalender WA. Computed tomography: fundamentals system technology image quality applications[M]. Xu Z, Chen H, Wang Y., Transl. 3rd ed. Beijing: Tsinghua University Press, 2016: 298.
[2] Parker J K, Craig E B, Klick D I, et al. Reflective tomography: images from range-resolved laser radar measurements[J]. Applied Optics, 1988, 27(13): 2642-2643.
[3] Knight F K, Klick D. Ryan-Howard D P, et al. Laser radar reflective tomography utilizing a streak camera for precise range resolution[J]. Applied Optics, 1989, 28(12): 2196-2198.
[4] Knight F K, Klick D I. Ryan-Howard D P, et al. Two-dimensional tomographs using range measurements[J]. Proceedings of SPIE, 1989, 0999: 269-280.
[6] Knight F K, Klick D I. Ryan-Howard D P, et al. Visible laser radar: range tomography and angle-angle-range detection[J]. Optical Engineering, 1991, 30(1): 55-65.
[7] Knight F, Kulkarni S, Marino R, et al. Tomographic techniques applied to laser radar reflective measurements[J]. The Lincoln Laboratory Journal, 1989, 2(2): 143-158.
[11] 金晓峰, 张鹏, 刘春华, 等. 远距离高分辨激光成像雷达技术[J]. 激光与光电子学进展, 2013, 50(5): 050002.
[12] 孙建锋, 闫爱民, 刘德安, 等. 远距离激光成像雷达进展[J]. 激光与光电子学进展, 2009, 46(8): 49-54.
[13] 庄天戈. CT原理与算法[M]. 上海: 上海交通大学出版社, 1992: 77- 97.
Zhuang TG. Principle and algorithm of CT[M]. Shanghai: Shanghai Jiao Tong University Press, 1992: 77- 97.
[14] Kak A C, Slaney M, Wang G. Principles of computerized tomographic imaging[J]. Medical Physics, 2002, 29(1): 106-108.
[18] Yan Y, Jin X F, Zhou S P, et al. Imaging resolution analysis using Fourier-slice theorem in reflective tomography laser radar[J]. Proceedings of SPIE, 2013, 8868: 88680V.
[24] 瞿福琪, 胡以华, 冷蛟峰. 基于啁啾脉冲的反射层析激光雷达成像[J]. 光电工程, 2012, 39(4): 55-59.
[25] 严毅, 金晓峰, 孙建锋, 等. 聚束非相干合成孔径激光成像雷达研究[J]. 光学学报, 2012, 32(2): 0211003.
[26] 瞿福琪, 胡以华, 焦均均, 等. 星对星激光雷达反射层析成像技术探讨[J]. 光子学报, 2013, 42(1): 48-53.
[27] 金晓峰. 基于CT原理的激光成像雷达关键技术研究[D]. 上海: 中国科学院上海光学精密机械研究所, 2012: 52- 53.
Jin XF. Research on key technologies of imaging ladar based on CT principles[D]. Shanghai: Shanghai Institute of Optics and Fine Mechanics Chinese Academy of Science, 2012: 52- 53.
[31] Fienup J R. Phase retrieval algorithms: a comparison[J]. Applied Optics, 1982, 21(15): 2758-2769.
[33] Yang G Z, Dong B Z, Gu B Y, et al. Gerchberg-Saxton and Yang-Gu algorithms for phase retrieval in a nonunitary transform system: a comparison[J]. Applied Optics, 1994, 33(2): 209-218.
[36] 赵楠翔, 胡以华. 激光反射层析成像相位恢复算法研究[J]. 红外与激光工程, 2019, 48(10): 1005005.
[37] 陈剑彪, 孙华燕, 赵延仲, 等. 基于相位恢复的失配反射层析图像重建算法[J]. 光学学报, 2018, 38(4): 0411002.
[40] 周德力, 黄庚华, 石亮, 等. 非合目标的激光反射层析投影配准方法[J]. 科学技术与工程, 2014, 14(21): 104-107, 112.
[41] 谷雨, 胡以华, 郝士琦, 等. 激光反射层析成像中滤波反投影算法特性研究[J]. 激光与红外, 2015, 45(12): 1500-1504.
[44] Wang J C, Zhou S W, Shi L, et al. Image quality analysis and improvement of ladar reflective tomography for space object recognition[J]. Optics Communications, 2016, 359: 177-183.
[45] 谷雨, 胡以华, 郝士琦, 等. 变分贝叶斯解卷积法在激光反射层析成像中的应用[J]. 光学学报, 2016, 36(6): 0611003.
[46] 林放, 王金诚, 张华, 等. 多帧迭代盲解卷积在激光反射断层成像的应用[J]. 光学学报, 2017, 37(9): 0911001.
[47] Shi L, Wang L, Xu S L, et al. Projections reconstruction in laser reflective tomography imaging through the use of basic wave pulse[J]. Proceedings of SPIE, 2020, 1142: 114270E.
[51] 金晓峰, 孙建锋, 严毅, 等. 反射层析激光雷达小系统成像模拟[J]. 光学学报, 2010, 30(3): 747-752.
[53] 杨彪, 胡以华, 李敏乐, 等. 激光反射断层成像目标的重构建模仿真[J]. 光学学报, 2018, 38(11): 1128002.
[54] 杨彪, 胡以华. 代数迭代法在激光反射断层成像目标重构中的应用[J]. 红外与激光工程, 2019, 48(7): 0726002.
[55] 杨富强, 张定华, 黄魁东, 等. CT不完全投影数据重建算法综述[J]. 物理学报, 2014, 63(5): 9-20.
[57] 金晓峰, 严毅, 孙建锋, 等. 基于角度-多普勒分辨的反射层析激光成像雷达研究[J]. 光学学报, 2012, 32(8): 0828001.
[61] 周德力. 星载激光反射层析成像关键技术研究[D]. 上海: 中国科学院研究生院, 2014: 60- 82.
Zhou DL. Study on key technologies of spaceborne laser reflection tomography imaging[D]. Shanghai: University of Chinese Academy of Sciences, 2014: 60- 82.
[62] Matson C L, Mosley D E. Reflective tomography reconstruction of satellite features: field results[J]. Applied Optics, 2001, 40(14): 2290-2296.
[64] MurrayJ, TriscariJ, FetzerG, et al. Tomographic lidar[C]∥Applications of Lasers for Sensing and Free Space Communications 2010, January 31-February 3, 2010,San Diego, California. Washington, D.C.: OSA, 2010: LSWA1.
[67] Li Y Q, Zhang G S. A seismic blind deconvolution algorithm based on Bayesian compressive sensing[J]. Mathematical Problems in Engineering, 2015, 2015(3): 1-11.
[68] ValenteD, IlowJ, CadaM. Blind deconvolution using compressed sensing in time dispersive MIMO OFDM systems[C]∥2015 IEEE 11th International Conference on Wireless and Mobile Computing, Networking and Communications (WiMob), October 19-21, 2015, Abu Dhabi, United Arab Emirates.New York: IEEE Press, 2015: 296- 301.
[69] StogerD, MathematikZ, JungP, et al.Blind deconvolution and compressed sensing[C]∥2016 4th International Workshop on Compressed Sensing Theory and its Applications to Radar, Sonar and Remote Sensing (CoSeRa), September 19-22, 2016. Aachen, Germany.New York: IEEE Press, 2016: 24- 27.
[71] Donoho D L. Compressed sensing[J]. IEEE Transactions on Information Theory, 2006, 52(4): 1289-1306.
[72] Romberg J. Imaging via compressive sampling[J]. IEEE Signal Processing Magazine, 2008, 25(2): 14-20.
[73] Sidky EY, ChartrandR, Pan XC. Image reconstruction from few views by non-convex optimization[C]∥2007 IEEE Nuclear Science Symposium Conference Record, October 26-November 3, 2007, Honolulu, HI, USA.New York: IEEE Press, 2007: 3526- 3530.
[74] 刘晓晖. 基于压缩感知的磁共振图像稀疏重建算法研究[D]. 广州: 南方医科大学, 2018: 31- 81.
Liu XH. Research on sparse reconstruction algorithm for MR images based on compressed sensing[D]. Guangzhou: Southern Medical University, 2018: 31- 81.
[75] 张红雨. 基于压缩感知理论的核磁共振图像重建算法研究[D]. 天津: 天津大学, 2017: 13- 30.
Zhang HY. Research on magnetic resonance image reconstruction algorithms using compressed sensing theory[D]. Tianjin: Tianjin University, 2017: 13- 30.
[76] 郑孙易. 基于压缩感知的计算机断层图像重建算法研究[D]. 沈阳: 东北大学, 2017: 33- 61.
Zheng SY. Research on computer tomography image reconstruction algorithm based on compressed sensing[D]. Shenyang: Northeastern University, 2017: 33- 61.
[77] 陈浩. 基于压缩感知理论的MRI图像重建算法研究[D]. 合肥: 中国科学技术大学, 2016: 33- 60.
ChenH. MRI reconstruction algorithms based on compressed sensing[D]. Hefei: University of Science and Technology of China, 2016: 33- 60.
[78] 陈庆贵, 卢洪义, 于光辉, 等. 基于先验图像-压缩感知的CT局部重建算法[J]. 计算机辅助设计与图形学学报, 2016, 28(4): 632-636.
[79] 吴珏蓉. 大呼吸运动下高时空分辨率腹部动态磁共振灌注图像的压缩感知重建[D]. 杭州: 浙江大学, 2016: 19- 56.
Wu JR. Compressed sensing reconstruction of high spatio-temporal resolution abdominal dynamic magnetic resonance perfusion images with large respiratian motion[D]. Hangzhou: Zhejiang University, 2016: 19- 56.
[81] Rose SD, Andersen MS, Sidky EY, et al.TV-constrained incremental algorithms for low-intensity CT image reconstruction[C]∥2015 IEEE Nuclear Science Symposium and Medical Imaging Conference (NSS/MIC), October 31 - November 7, 2015, San Diego, CA, USA.New York: IEEE Press, 2015: 1- 3.
[82] Rose SD, Sidky EY, Pan XC. TV constrained CT image reconstruction with discretized natural pixels[C]∥2016 IEEE Nuclear Science Symposium, Medical Imaging Conference and Room-Temperature Semiconductor Detector Workshop (NSS/MIC/RTSD), October 29-November 6, 2016, Strasbourg, France.New York: IEEE Press, 2016: 1- 3.
[84] ZhangZ, Ye JH, RoseS, et al.Preliminary study of TV-constrained-likelihood-maximization image reconstruction from list-mode TOF-PET data[C]∥2016 IEEE Nuclear Science Symposium, Medical Imaging Conference and Room-Temperature Semiconductor Detector Workshop (NSS/MIC/RTSD), October 29-November 6, 2016, Strasbourg, France.New York: IEEE Press, 2016: 1- 3.
[86] Chen ZY, BasarabA, KouaméD. Enhanced ultrasound image reconstruction using a compressive blind deconvolution approach[C]∥2017 IEEE International Conference on Acoustics, Speech and Signal Processing (ICASSP), March 5-9, 2017, New Orleans, LA, USA.New York: IEEE Press, 2017: 6245- 6249.
[88] 王蕾. 基于深度生成网络的不完全投影CT图像重建方法[D]. 南京: 南京邮电大学, 2019: 15- 59.
WangL. CT reconstruction from incomplete projections based on deep generative networks[D]. Nanjing: Nanjing University of Posts and Telecommunications, 2019: 15- 59.
[89] 白家男. 基于上下文编码生成对抗性网络的不完全投影数据2D/3D CT图像重建算法研究[D]. 南京: 南京邮电大学, 2019: 18- 52.
Bai JN. 2D/3D CT reconstruction from incomplete projections by using a contextual autoencoder network[D]. Nanjing: Nanjing University of Posts and Telecommunications, 2019: 18- 52.
Article Outline
胡以华, 张鑫源, 徐世龙, 赵楠翔, 石亮. 激光反射层析成像技术的研究进展[J]. 中国激光, 2021, 48(4): 0401002. Yihua Hu, Xinyuan Zhang, Shilong Xu, Nanxiang Zhao, Liang Shi. Research Progress of Laser Reflective Tomography Techniques[J]. Chinese Journal of Lasers, 2021, 48(4): 0401002.