近红外光谱传感物联网研究与应用进展
下载: 1163次特邀综述
Significance A variety of sensing applications in the Internet of Things (IoT) raise the diverse needs for sensors. The sensing ability of material composition is a weak part of the sensing layer of the IoT. Due to the technical limitations, traditional analysis methods, such as mass spectrometry and chromatography, cannot be directly applied in the field of the IoT. With the advantages of speediness, lossless and high-efficiency, the technology of near-infrared (NIR) spectroscopy can be applied to the applications of composition analysis. In recent years, the trend of commercialization of spectral analysis has promoted the development of miniaturization, networking and imaging of analytical equipment. The devices based on filters and array detectors are compact without moving parts, which makes them ideal for IoT applications. The miniaturized instruments have been successfully applied in many industrial and agricultural production fields such as petrochemical industry, grain screening, and pharmaceutical industry. With the successful application of machine learning and deep learning, NIR spectral analysis has an intelligent trend of component sensing.
InGaAs focal plane arrays (FPAs) have the advantages of working near room temperature, high detection rate, good uniformity and stability, which are beneficial to realize the miniaturization design of the NIR photoelectric system. As the core photoelectric sensor, InGaAs FPAs are widely used in NIR spectral analysis equipments. This paper introduces the key technologies of the NIR spectral sensing IoT with several kinds of compact InGaAs spectral sensors.
Progress NIR spectral sensing IoT (
Based on a 256×1 FPA, the 64-channel filter integrated spectral sensor (
For the spectral sensors with different structures, the corresponding spectral sensing nodes were further developed. The optical structure and wireless communication interface had been integrated in the spectral sensing node (
Conclusion and Prospect This paper introduces the system architecture and key technologies of the NIR spectral sensing IoT. In order to realize the miniaturization design of spectral sensing nodes, two kinds of micro spectral sensors are introduced, which are integrated multi-channel filter and integrated LVF. The performance of spectral sensors is compared and analyzed with the preparation process. The research and application of NIR sensing nodes, cloud server and mobile phone client are further introduced. Finally, the future development of NIR sensing IoT is proposed. In the long term, the future development direction of spectral sensing IoT is intellectualization. The integration of system on chip (SoC), wireless communication module and intelligent analysis algorithm will be further realized in the intelligent spectral sensors.
1 引言
进入21世纪以来,信息技术先后经历了互联网时代和移动互联网时代,正快速向万物互联的物联网(IoT)时代迈进[1]。从技术架构来看,IoT系统可以分为感知层、传输层和应用层。随着IoT云平台的日趋成熟,“IoT+行业应用”的模式开始在智慧城市、智慧农业、智能交通和智慧医疗等细分领域得到规模化的应用验证[2-6]。依托于传输层和应用层解决方案的多样化,IoT感知层节点设备迅速增加,在2017年达到84亿,首次超过全球人口数目[2]。面对IoT多样化的传感需求,感知层的感知以及认知能力亟需多样化和立体化的发展。对物质成分的传感能力是IoT感知层的薄弱环节,实验室中传统的质谱、色谱和化学分析方法受其技术特点所限,很难直接应用于注重微型化、低成本和时效性的IoT领域。
近红外(NIR)光谱分析技术具有快速、无损、高效的特点,是一种常用的物质成分分析手段[7]。近年来,光谱分析的商业化趋势推动了分析设备的微型化、网络化和成像化发展,并在石油化工、谷物筛选、制药等众多工农业生产领域取得成功应用[7-11]。随着机器学习和深度学习等技术在光谱分析领域的应用取得成功,NIR光谱分析进一步出现了智能化的发展趋势,为实现IoT领域的智能化成分感知奠定了技术基础[12-14]。
按照波长范围和使用的传感器种类划分,NIR光谱传感主要可以分为两类,一类是使用电荷耦合元件(CCD)、互补金属氧化物半导体(CMOS)类基于已充分发展的Si材料传感器,受到材料本身特性限制,其响应波段位于NIR的短波端(700~1100nm),也可以进入可见光波段和紫外波段;另一类是使用InGaAs等化合物材料的传感器,其响应波段位于NIR的长波端(1100~2500nm),也可向NIR短波端及可见光波段拓展[7]。在遥感应用、红外探测器和红外材料等专业领域中,长波NIR波段常被称为短波红外。相比于短波NIR,长波NIR波段含有更丰富的特征信息并且吸收波带重叠程度更低,因而具有更广泛的应用前景和开发价值[7]。按照仪器的分光方式划分,可以将NIR光谱设备分为光栅型、滤光片型、傅里叶变换型和声光可调滤波器型等[7,15]。基于滤光片和阵列探测器的滤光片型光谱分析设备结构紧凑、不含移动部件,非常适合应用于对微型化和便携式设计要求较高的IoT领域[15-23]。得益于CMOS工艺的成熟,滤光片型短波NIR光谱仪较为常见[18-20]。国外已经报道了集成线性渐变滤光片(LVF)和128元InGaAs阵列的微型NIR光谱仪,其像元尺寸为30μm×500μm,中心距为50μm[1]。虽然当下此类光谱设备的分辨率不高(在900~1700nm范围内为10~20nm),但在吸收峰较宽、对光谱分辨率要求不高的NIR波段,仍然具有体积和成本方面的优势。
近10年来,中国科学院上海技术物理研究所在高灵敏度常规波长(0.9~1.7μm) InGaAs焦平面、延伸波长(1.0~2.5μm) InGaAs 焦平面以及新型多功能InGaAs探测器研究领域取得了良好进展[24-26],并进一步联合山东大学等相关单位在NIR光谱传感IoT中开展了开拓性的研究和应用探索。针对IoT感知层中物质成分传感的应用需求,采用滤光片作为分光部件与InGaAs线列焦平面耦合集成,可以研制微型化NIR光谱组件。基于光谱组件进一步设计光学测试附件、信号采集处理电路和无线通信模块,可以进一步形成NIR光谱感知节点,进行光谱传感IoT应用。文章首先介绍了NIR光谱传感IoT系统采用的网络架构和关键技术。接着,针对多种集成光谱组件的微型化结构设计、波长定标、性能测试和分析展开重点阐述,并结合传输层和应用层设计完成了基于光谱组件的NIR传感IoT应用验证。最后,对NIR光谱传感IoT的未来发展进行展望。
2 光谱传感物联网系统结构
相比于常规的IoT系统,NIR光谱传感IoT在感知层中加入定制化的NIR光谱感知节点,通过集成NIR光谱分析技术实现IoT感知层的成分传感功能[27-30]。一个典型的光谱传感IoT结构如
光谱感知节点可以看作是经过微型化、网络化和便携式设计改进的定制NIR光谱仪,在探测器、分光部件、信号采集电路和光学测试附件的基础上增加了无线通信模块,可以满足IoT的应用需求[28]。光谱感知节点是光谱传感IoT系统中的核心部件,光谱感知节点的性能和微型化程度与光谱组件的结构和设计方案息息相关。
3 近红外光谱组件
InGaAs 探测器不仅可在近室温情况下工作,还具有量子效率高、暗电流低和抗辐照特性强等优点,进而成为高灵敏度、低功耗、小型化、高可靠性NIR传感系统的理想选择[23-25]。将NIR InGaAs焦平面阵列与不同波段中心波长的滤光片耦合,可以获得内部集成分光部件的光谱组件。由于没有可移动部件,该结构的光谱组件结构紧凑易于集成,同时还具有高光能量利用率、高可靠性和低成本的优点。以广泛应用的全介质膜窄带通滤光片为例,采用光学镀膜工艺获得高低折射率膜层相互交替的多层反射膜结构,通过调控间隔层厚度,即可获得不同的透过率中心波长λ,其理论关系式为[20, 34]
式中,n为间隔层介质的折射率,d为间隔层厚度, θ为入射角,N为干涉级次。
3.1 集成式滤光片型光谱组件
采用多波段窄带通滤光片阵列和InGaAs线列探测器耦合是一种常用的NIR光谱组件研制方案。一种典型的64通道光谱组件结构图如
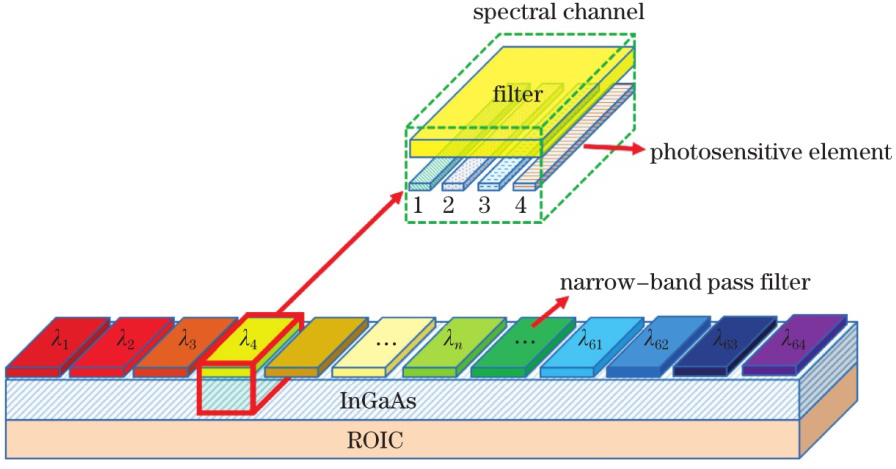
图 2. 集成64通道滤光片型256×1光谱组件结构图
Fig. 2. Architecture of 256×1 spectral sensor integrated 64 channels filter
基于一款256×1焦平面研制此集成64通道滤光片型光谱组件。其中,光敏芯片设计为正入射的长条形结构,256个光敏元呈一字型排列,相邻光敏元中心距为50μm,单个光敏元尺寸为50μm×500μm。光敏芯片通过键合工艺与分布两侧的两个128×1读出集成电路(ROIC)完成交叉互联,通过过渡电基板完成信号引出。由于光敏元中心距为50μm,如采用每个像元作为一个光谱通道,滤光片阵列的每个通道区域较小,对微纳工艺的制备水平要求较高。考虑到滤光片通道制备以及与光敏元对准过程中的工艺误差会对光谱组件性能产生直接影响,因而采用4个相邻光敏元复用作为一个光谱通道,在256个光敏元上制备64个光谱通道,可以极大程度地减小光谱组件制备工程中的工艺误差。
LVF是一种楔形介质薄膜法布里-珀罗窄带通滤光片,通过特殊镀膜工艺将窄带滤光片的间隔层制作成楔形,不同位置的间隔层对应不同的等效光学厚度,从而对应线性变化的透过率中心波长[37-38]。采用一款900~1700 nm的LVF分别与256×1和512×2两种规格焦平面进行耦合研制LVF型光谱组件[39-40]。256×1焦平面设计参数与64通道滤光片型光谱组件所采用的焦平面一致,512×2焦平面采用25μm×25μm光敏元尺寸,两列光敏元平行放置,两列中间存在25μm间隔。LVF型光谱组件结构如
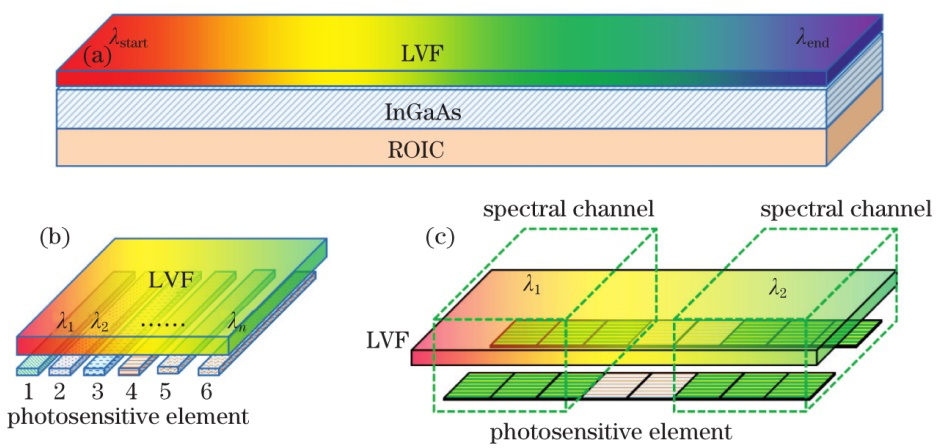
图 3. LVF型光谱组件。(a)组件结构图; (b)256×1型组件的光谱通道示意图; (c)512×2型组件的光谱通道示意图
Fig. 3. Spectral sensor integrated LVF. (a) Architecture of the spectral sensor; (b) spectral channels of 256×1 spectral sensor; (c) spectral channels of 512×2 spectral sensor
减小LVF和光敏芯片的间隔可以减小光谱串扰,提升光谱分辨率。LVF型光谱组件的装配方案如
3.2 光谱组件性能分析
采用单色仪和焦平面测试系统采集各光敏元对单色光的响应,按照设计结构完成对各个光谱通道的波长定标,并采用稀土氧化物玻璃等标准物质对标定结果进行验证。三种光谱组件的详细定标过程在早期论文中有详细介绍[35, 39-40],下面重点对不同光谱组件的性能进行对比和分析。
三种光谱组件的波长定标结果如
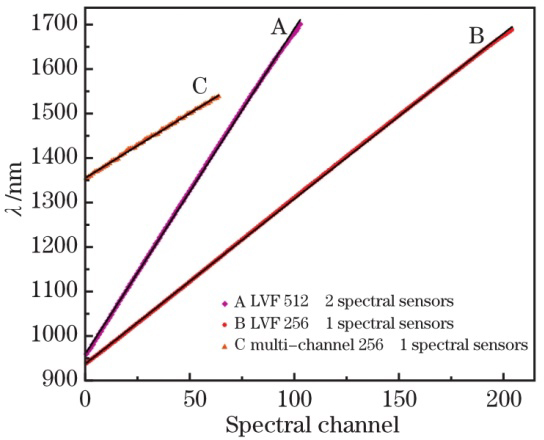
图 5. 三种光谱组件的光谱通道与中心波长关系
Fig. 5. Corresponding relations between wavelength and spectral channels of three spectral sensors
三种光谱组件光谱通道的响应曲线细节如
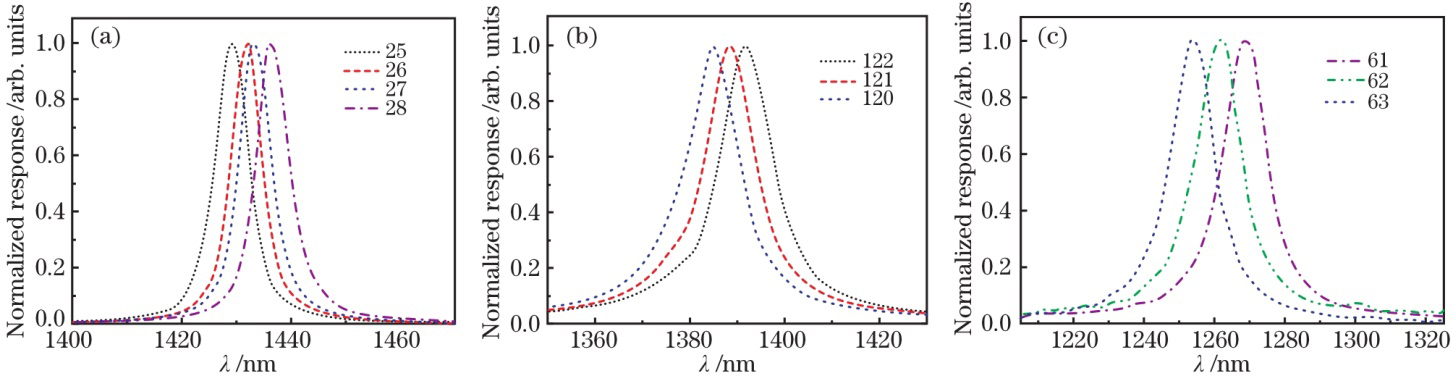
图 6. 三种光谱组件的归一化光谱响应。(a)64通道滤光片型光谱组件;(b)256×1 LVF型光谱组件; (c)512×2 LVF型光谱组件
Fig. 6. Normalized spectral response of three spectral sensors.(a) Spectral sensor integrated 64 channels filter; (b) 256×1 LVF spectral sensor;(c) 512×2 LVF spectral sensor
综上所述,现阶段滤光片型光谱组件的性能主要受滤光片性能所限。其中,多通道型滤光片主要存在与光敏芯片对准问题和性能一致性问题,LVF则是分辨率稍显不足,而普遍存在的通道间谱线交叠问题可以通过光谱通道结构设计予以显著改善。
4 近红外光谱传感物联网应用验证
光谱传感IoT应用验证采用

图 8. 光谱传感IoT实际应用效果图。(a)光谱感知节点测试的低压汞灯谱线; (b)手机客户端特定时间获取的测试谱线
Fig. 8. Practical application demonstration pictures of spectral sensing IoT. (a) Spectrum of low pressure mercury lamp tested by spectral sensing node; (b) test spectrum obtained by mobile phone client at a specific time
对于三种不同结构的光谱组件,均进一步开展相应的光谱感知节点研制。针对64通道滤光片型光谱组件,开展节点专用的光学结构设计,探讨远距离探测及入射光角度带来的问题和影响,取得了有益效果[41-42]。使用256×1 LVF型光谱感知节点测试了SRM 2035a稀土氧化物玻璃、低压汞灯、多种激光器的光谱曲线,验证了节点的基本性能参数[28]。基于512×2 LVF型光谱感知节点搭建测试平台,对4种不同标称乙醇浓度的酒类样本进行测试,获得了实际应用验证[30]。在光谱感知节点的无线通信接口上,分别开展了基于433 MHz无线模块、Zigbee协议组网、GPRS、3G和NB-IoT蜂窝网络的接口开发,实现了NIR光谱数据的近距离和广域传输,为后续光谱传感网络的野外应用奠定了技术基础[28,31]。分别使用“阿里云”和“华为云”搭建ECS平台,实现了光谱数据的多平台转存和发布。在光谱分析模型开发上,针对土壤样本开展有机质和总氮含量数据预处理和建模研究[43],实现了崂山绿茶和日照绿茶的产地溯源和生产日期鉴别验证[44-45]。在NIR光谱分析软件开发上,自主开发绿茶成分分析软件ARCO-NIR,实现了光谱信息预处理、特征波长提取、定性识别和定量回归四大功能模块的集成[36]。在手机客户端分析软件开发上,实现了光谱曲线的绘制和图像的保存功能,集成了光谱数据的归一化、一阶微分、二阶微分、峰值寻找等数据处理功能和温湿度、二氧化碳浓度、光照强度等环境数据显示功能[32-33]。在下一代光谱组件的研发上,通过在ROIC内部片上集成逐次逼近寄存器(SAR)结构模数转换器(ADC),实现了光谱组件传感器内的数字化,并取得了应用验证[46-48]。片内数字化不仅有效改善了组件的噪声性能,更为下一步片上的智能化信号处理奠定了技术基础。
在光谱传感IoT的后续发展中,需要考虑光谱分析模型的嵌入方式。从整个网络结构来看,光谱感知节点集成、ECS集成和手机客户端集成是三个可行方案。光谱感知节点集成的优势在于数据在系统最前端处理,可以显著减小数据传输和服务器存储压力,缺点是对节点的数据处理能力要求较高,且分析模型的配置灵活性较差,在当前的技术水平下有较高的后续维护成本。相比于节点,智能手机拥有更高的算力,但位置位于系统最末端,需要对大量的原始数据进行多次传输。ECS位于IoT的平台层,拥有最强的算力,将多种应用的模型布置在云平台,可以直接完成光谱数据的处理和分析,并将结果反馈至用户手机客户端,具备较高的技术可行性。长远来看,光谱传感IoT未来的发展方向是网络节点的智能化,在分光结构和ADC集成的基础上,进一步实现片上系统(SoC)、无线通信模块以及智能分析算法的集成,最终由NIR光谱感知节点发展为专用的NIR智能光谱传感器。
5 结束语
介绍了NIR光谱传感IoT系统的网络架构和关键技术。为了实现光谱感知节点的微型化设计,提出集成多通道滤光片和集成LVF这两种微型光谱组件结构,在波长定标的基础上,结合焦平面、滤光片的特性及耦合工艺对两种光谱组件开展性能对比和分析。标定结果表明,多通道型滤光片主要存在与光敏芯片对准问题和性能一致性问题,LVF则是分辨率稍显不足,通道间谱线交叠问题可以通过光谱通道结构设计予以显著改善。基于集成式光谱组件,进一步介绍了NIR光谱感知节点、ECS和手机客户端的研究应用现状,并对NIR光谱传感IoT的未来发展进行了展望。
[1] 方家熊. 中国电子信息工程科技发展研究[M]. 北京: 科学出版社, 2018: 1- 23.
Fang JX. Research on the development of electronic information engineering technology in China[M]. Beijing: Science Press, 2018: 1- 23.
[2] 于文平. 《2017—2018中国物联网发展年度报告》发布[J]. 物联网技术, 2018, 8(10): 5-6.
[3] 陈海明, 崔莉. 面向服务的物联网软件体系结构设计与模型检测[J]. 计算机学报, 2016, 39(5): 853-871.
[4] 熊本海, 杨振刚, 杨亮, 等. 中国畜牧业物联网技术应用研究进展[J]. 农业工程学报, 2015, 31(S1): 237-246.
[5] 王静远, 李超, 熊璋, 等. 以数据为中心的智慧城市研究综述[J]. 计算机研究与发展, 2014, 51(2): 239-259.
[6] 李瑾, 郭美荣, 高亮亮. 农业物联网技术应用及创新发展策略[J]. 农业工程学报, 2015, 31(S2): 200-209.
[7] 褚小立. 近红外光谱分析技术实用手册[M]. 北京: 机械工业出版社, 2016.
Chu XL. Handbook of near infrared spectroscopy[M]. Beijing: China Machine Press, 2016.
[8] 褚小立, 史云颖, 陈瀑, 等. 近五年我国近红外光谱分析技术研究与应用进展[J]. 分析测试学报, 2019, 38(5): 603-611.
[9] 褚小立, 陆婉珍. 近五年我国近红外光谱分析技术研究与应用进展[J]. 光谱学与光谱分析, 2014, 34(10): 2595-2605.
[10] 王多加, 周向阳, 金同铭, 等. 近红外光谱检测技术在农业和食品分析上的应用[J]. 光谱学与光谱分析, 2004, 24(4): 447-450.
[11] 刘文清, 陈臻懿, 刘建国, 等. 环境监测领域中光谱学技术进展[J]. 光学学报, 2020, 40(5): 0500001.
[12] 刘立新, 何迪, 李梦珠, 等. 基于高光谱技术与机器学习的新疆红枣品种鉴别[J]. 中国激光, 2020, 47(11): 1111002.
[13] 倪超, 李振业, 张雄, 等. 基于短波近红外高光谱和深度学习的籽棉地膜分选算法[J]. 农业机械学报, 2019, 50(12): 170-179.
Ni C, Li Z Y, Zhang X, et al. Filmsorting algorithm in seed cotton based on near-infrared hyperspectral image and deep learning[J]. Transactions of the Chinese Society for Agricultural Machinery, 2019, 50(12): 170-179.
[14] 谈爱玲, 王晓斯, 楚振原, 等. 基于近红外光谱融合与深度学习的玉米成分定量建模方法[J]. 食品与发酵工业, 2020, 46(23): 213-219.
Tan A L, Wang X S, Chu Z Y, et al. Research on quantitative modeling method of maize composition based on near infrared spectrum fusion and deep learning[J]. Food and Fermentation Industries, 2020, 46(23): 213-219.
[18] Schmidt O, Kiesel P, Bassler M. Performance of chip-size wavelength detectors[J]. Optics Express, 2007, 15(15): 9701-9706.
[20] 于新洋. 线性渐变滤光片型近红外水果品质分析仪及应用研究[D]. 北京: 中国科学院大学, 2016: 2- 38.
Yu XY. Development and application of a handheld near-infrared spectrometer based on a linear variable filter for measuring the internal quality of fruit[D]. Beijing: University of Chinese Academy of Sciences, 2016: 2- 38.
[24] 李雪, 邵秀梅, 李淘, 等. 短波红外InGaAs焦平面探测器研究进展[J]. 红外与激光工程, 2020, 49(1): 0103006.
[25] Li X, Gong H M, Fang J X, et al. The development of InGaAs short wavelength infrared focal plane arrays with high performance[J]. Infrared Physics & Technology, 2017, 80: 112-119.
[26] 邵秀梅, 龚海梅, 李雪, 等. 高性能短波红外InGaAs焦平面探测器研究进展[J]. 红外技术, 2016, 38(8): 629-635.
[27] 王绪泉. 新型光谱组件的网络节点设计及实验[D]. 济南: 山东大学, 2016: 2- 38.
Wang XQ. The design and experiments of network node based on a new monolithically integrated multichannel spectral sensor[D]. Jinan: Shandong University, 2016: 2- 38.
[28] 王绪泉, 黄松垒, 于月华, 等. 微型长波近红外物联网节点及实验研究[J]. 红外与毫米波学报, 2018, 37(1): 42-46.
[29] 王果山. 新型光谱感知物联网节点系统设计及实验[D]. 济南: 山东大学, 2018: 20- 58.
Wang GS. Design and experiment of a new spectral-sensing IoT node system[D]. Jinan: Shandong University, 2018: 20- 58.
[31] 王凯. 基于光谱数据的多参数实时监测系统设计与实现[D]. 济南: 山东大学, 2016: 10- 58.
WangK. Design and implementation of real-time monitoring system based on spectral data[D]. Jinan: Shandong University, 2016: 10- 58.
[32] 魏永畅, 王绪泉, 魏杨, 等. 基于Android系统的光谱传感物联网移动终端设计[J]. 计算机测量与控制, 2019, 27(3): 131-136.
[33] 魏永畅. 光谱传感物联网专用移动终端设计与实验[D]. 北京: 中国科学院大学, 2019: 21- 58.
Wei YC. Design and experiment of dedicated mobile terminal in spectral sensing Internet of Things[D]. Beijing: University of Chinese Academy of Sciences, 2019: 21- 58.
[34] 赵永强, 刘芯羽, 汤超龙. 光谱滤光片阵列进展[J]. 激光与光电子学进展, 2020, 57(19): 190004.
[36] 庄新港. 近红外光谱分析应用研究及新型光谱感知节点入射光学系统设计[D]. 济南: 山东大学, 2017: 15- 108.
Zhuang XG. Applied study of near-infrared spectroscopy and incidence optical system design of new spectral sensing node[D]. Jinan: Shandong University, 2017: 15- 108.
[37] 王世丰, 袁艳, 苏丽娟, 等. 线性渐变滤光片光谱特征参数测试方法[J]. 光子学报, 2017, 46(11): 1112002.
[39] 王绪泉, 黄松垒, 于月华, 等. 集成线性渐变滤光片和InGaAs焦平面的微型光谱模组及其波长定标[J]. 光子学报, 2018, 47(5): 0530001.
[40] 王绪泉, 黄松垒, 柯鹏瑜, 等. 集成滤光片型近红外光谱组件的时空域性能改善研究[J]. 光子学报, 2021, 50(4): 0430001.
Wang X Q, Huang S L, Ke P Y, et al. Improvement of LVF-based NIR spectral sensor on both spatial and time domains[J]. Acta Photonica Sinica, 2021, 50(4): 0430001.
[42] 庄新港, 史学舜, 王恒飞, 等. 角度调谐短波红外光谱组件光谱分辨率特性研究[J]. 红外与毫米波学报, 2018, 37(3): 332-337.
[43] 焦德晓. 应用不同预处理方法建模测定土壤有机质和总氮含量[D]. 济南: 山东大学, 2019: 15- 55.
Jiao DX. Determination of soil organic matter and total nitrogen by modeling with different spectral pretreatment methods[D]. Jinan: Shandong University, 2019: 15- 55.
[45] 庄新港, 王丽丽, 吴雪原, 等. 基于近红外的移动窗口BP神经网络实现山东绿茶产地溯源[J]. 红外与毫米波学报, 2016, 35(2): 200-205.
[46] 魏杨, 王绪泉, 魏永畅, 等. 微型近红外物联网节点的传感器输出数字化应用研究[J]. 红外与激光工程, 2019, 48(9): 0904002.
[47] 魏杨, 王绪泉, 黄张成, 等. 短波红外InGaAs线性焦平面数字化输出研究[J]. 红外与毫米波学报, 2018, 37(3): 257-261.
[48] 魏杨. 数字输出的微型短波红外铟镓砷光谱组件研究[D]. 北京: 中国科学院大学, 2019: 21- 108.
WeiY. Study on compact short-wave infrared InGaAs spectral sensor with digital output[D]. Beijing: University of Chinese Academy of Sciences, 2019: 21- 108.
王绪泉, 王丽丽, 方家熊. 近红外光谱传感物联网研究与应用进展[J]. 中国激光, 2021, 48(12): 1210001. Xuquan Wang, Lili Wang, Jiaxiong Fang. Research and Application Progresses of Near-Infrared Spectral Sensing Internet of Things[J]. Chinese Journal of Lasers, 2021, 48(12): 1210001.