Color ghost imaging via sparsity constraint and non-local self-similarity
Download: 807次
1. Introduction
Ghost imaging (GI) is a non-local imaging method, which reconstructs an unknown image by computing the intensity correlation function between the bucket signal from the object and the reference intensity distribution[1
Recently, color GI and multi-spectral GI have been receiving increasing interest[18
As we know, except for the prior knowledge of the target’s sparsity, most of natural images have some other characteristics such as orthogonality in three-dimensional spatial structure[24] and non-local self-similarity in both spatial and spectral structures[25,26]. In this paper, we propose a color GI approach to produce a color image, where the target is illuminated by a three-color non-orthogonal random pattern, and the photons from the object are directly collected by a single-pixel detector. Both the sparsity and non-local self-similarity of targets are utilized to improve the quality of the color GI in the image reconstruction process. The validity of this approach is verified by numerical simulation.
2. Method of Color Ghost Imaging and Image Reconstruction
The principle scheme and sampling procedure of the proposed color GI approach are shown in Fig. 1. The color pattern , which consists of three binary random coding patterns , , , with a duty cycle of 50%, is emitted from the digital light projector and illuminates the color scene . Then, the photons reflected from the scene are focused onto a single-pixel bucket detector by a collecting lens. We emphasize that different from Ref. [23], three encoded patterns are random and not orthogonal in both the spatial and temporal domains, and the red pattern only interacts with the object’s red channel . Therefore, the detection model can be expressed as where is the intensity recorded by the bucket detector, and denotes the detection noise. , and represent the red, green, and blue images of the color scene, respectively. In addition, denotes the th measurement, and is the total measurement number.
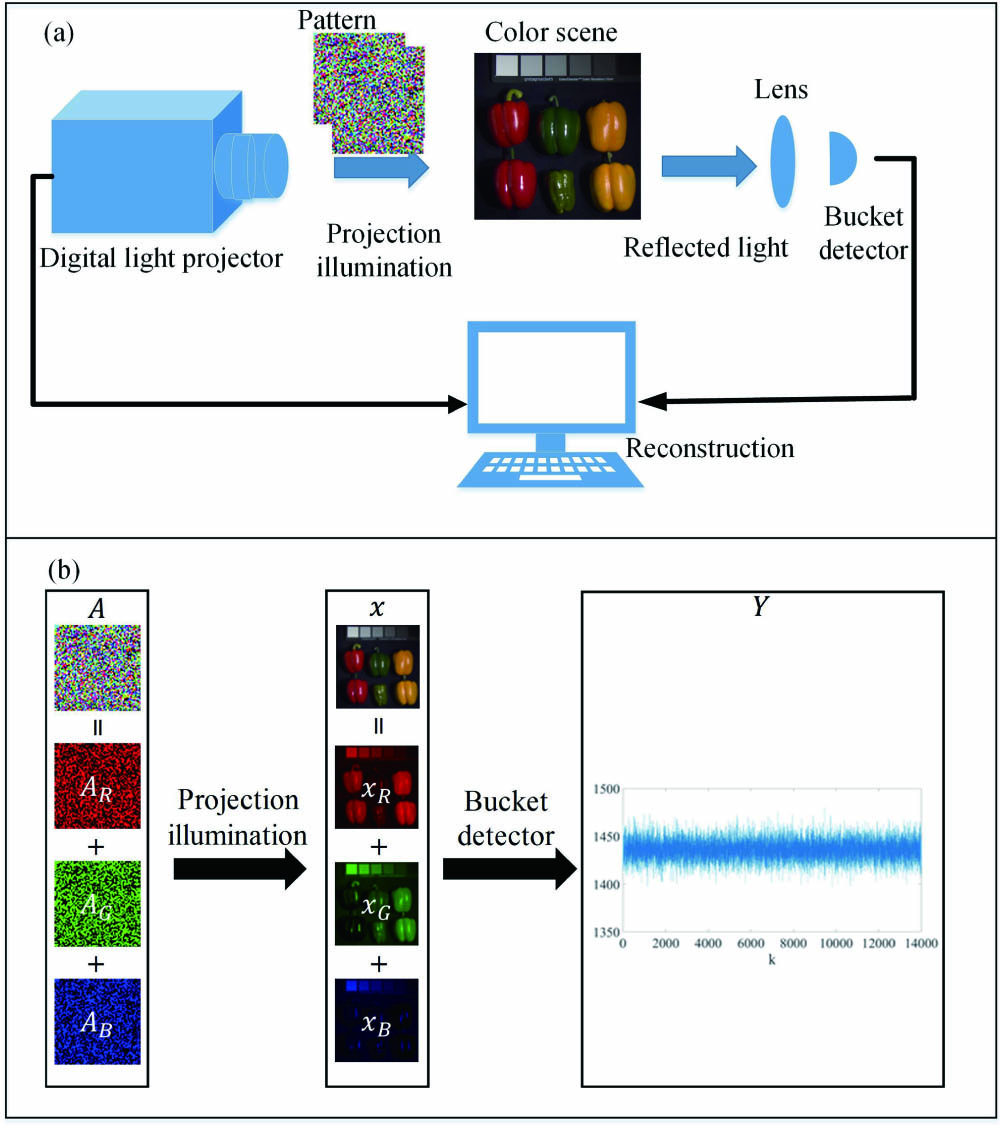
Fig. 1. (a) Principle scheme of proposed color ghost imaging and (b) its sampling model.
Obviously, as shown in Fig. 1, Eq. (1) can be described as a series of matrix operations, namely, where both and are column vectors, , , and are matrices, and is a column vector.
According to the measurement model displayed in Fig. 1 and Eq. (2), we will try to reconstruct the colored image by three different reconstruction algorithms based on compressive sensing. The first reconstruction method is to reconstruct the RGB images according to the same echo signal and the corresponding random patterns , , and , respectively. The reconstruction model (GISC_R) can be expressed as where , , and are, respectively, corresponding to the RGB images of the object, denotes the relaxation factor that determines the balance between the reconstruction error and the signal’s sparsity, and is the sum of the magnitudes of the discrete gradient in the horizontal and vertical directions, which can be represented as . In addition, the color image of objects can be obtained by synthesizing the images of , , and .
From Eqs. (2) and (3), it is obviously observed that Eq. (3) is a parallel reconstruction approach, and the computing scale of each single-wavelength image reconstruction is small, but the signal of the other two color images is considered noise in the reconstruction process of each single-wavelength image, which will lead to the degradation of the imaging quality.
Different from the first reconstruction method described in Eq. (3), the second reconstruction method is to directly restore the object’s RGB images at the same time, which has been widely used in the field of spectral imaging[27]. The reconstruction model (GISC) can be described as
Compared with Eq. (3), Eq. (4) has larger computing data, but only the detection noise will disturb the image reconstruction; thus, the reconstruction results of GISC will be better than that obtained by the method of GISC_R.
For natural images, except for prior knowledge of the target’s sparsity, non-local self-similarity is also a significant a priori characteristic, which was firstly utilized in the field of image denoising[25,26]. Non-local self-similarity is considered where there are lots of similar structures or textures in the spatial or spectral domain for an image. As shown in Fig. 2, the structure of the areas labeled by the red color square [Fig. 2(a)] is similar in the spatial domain for a single-wavelength image, and the labeled square areas of Figs. 2(b) and 2(c) are similar in the spectral domain. Generally speaking, as displayed in Fig. 2, compared with the spatial image, non-local self-similarity is much more obvious for color and spectral images. On the basis of Eq. (4), the reconstruction model of GISC and non-local self-similarity (GISCNL) can be expressed as where denotes the relaxation factor, and is the non-local self-similarity constraint. is the column vector containing all of the weights , and is the column vector containing all . In addition, and are the central pixel of similar patches and in the whole image . Compared with the second reconstruction method described in Eq. (4), the a priori knowledge of non-local self-similarity has been exploited in the reconstruction progress, and the reconstruction quality may be further enhanced by GISCNL.
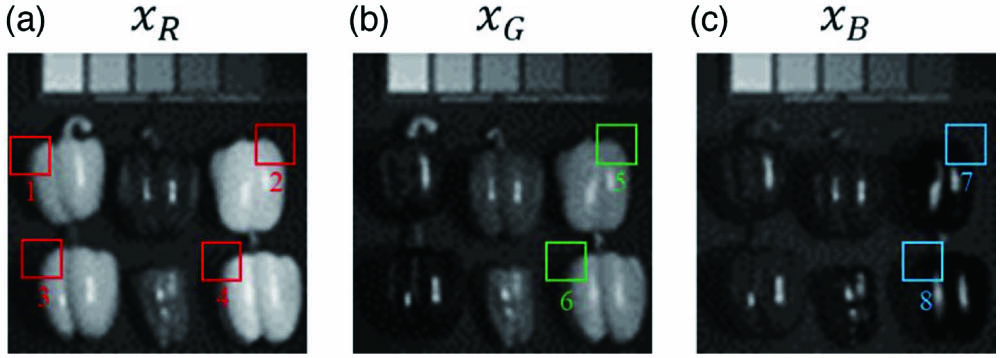
Fig. 2. Illustration of non-local self-similarity in the spatial domain for a single-wavelength image and in the spectral domain between two wavelength images.
In order to evaluate quantitatively the different reconstruction algorithms, the reconstruction quality is estimated by calculating the peak signal-to-noise ratio (PSNR)[28],
For a 0–255 gray-scale image, , and MSE is mean square error of the reconstruction image with respect to the original target, namely, where is the pixel number of the reconstructed image.
3. Simulation Results
To verify the feasibility of the proposed method, a pure trichromatic slits image and a generic RGB peppers image, as shown in the first row of Figs. 3(a1) and 3(b1), are used as the imaging targets. All simulations are based on MATLAB2014A with 8G memory, Intel Core i5-6200u laptop. Both random patterns illuminating the targets and the targets are pixels. Figures 3(a2)–3(a4) and Figs. 3(b2)–3(b4) illustrate the reconstructed RGB images of the slits and peppers, and their RGB images are shown in Figs. 3(a1) and 3(b1) based on the reconstruction model described in Eqs. (3)–(5). We have exploited the iterative reweighted least squares (IRLS) algorithm for the image reconstruction[29]. The PSNRs of corresponding reconstruction results are displayed in Fig. 3(c). The results shown in Fig. 3 suggest that the three reconstruction models above can recover the target’s color image and divided images of RGB. It is also clearly seen that the quality of GISC is better than GISC_R, and the reconstruction quality of GISCNL is the best in the same conditions when both the object’s sparsity and non-local self-similarity are exploited in the image reconstruction process. As shown in the last column of the table, for the same number of samples, GISC_R and GISC have almost the same processing time. Because of the higher computational complexity of GISCNL, GISCNL requires a longer processing time.
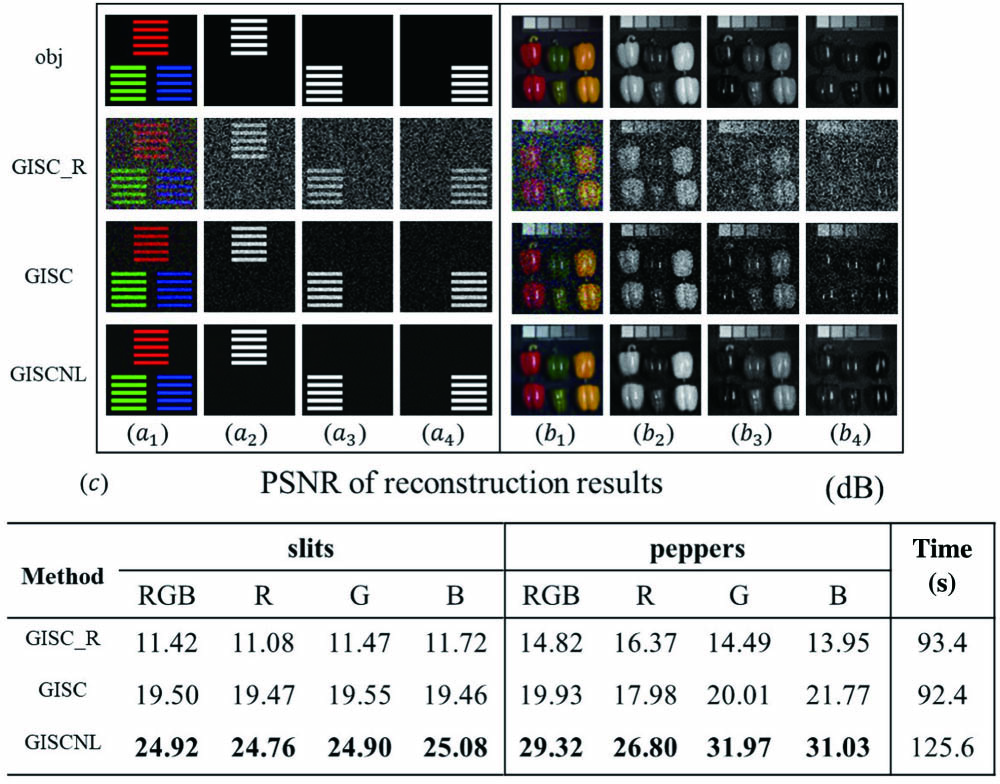
Fig. 3. Simulation results of three reconstruction algorithms in the case of detection and k . The first row is the object and their corresponding RGB images. The second row is the results of GISC_R. The third row is the results of GISC. The fourth row is the results of GISCNL. (c) The PSNR of reconstruction results in different reconstruction algorithms. The last column is the average processing time.
In Fig. 4, we have given the reconstructed results in different measurement number when the detection signal-to-noise ratio (SNR) is 30 dB. It is observed that the imaging quality is increased with the measurement number for the three reconstruction algorithms, and GISCNL is always the best (GISCNL is higher than 8 dB compared with GISC). What is more, both GISC and GISCNL have a significant enhancement as the measurement number increases. In addition, when the measurement number is greater than 10,000 (namely, the sampling compressive ratio ), the target can be perfectly reconstructed by GISCNL.
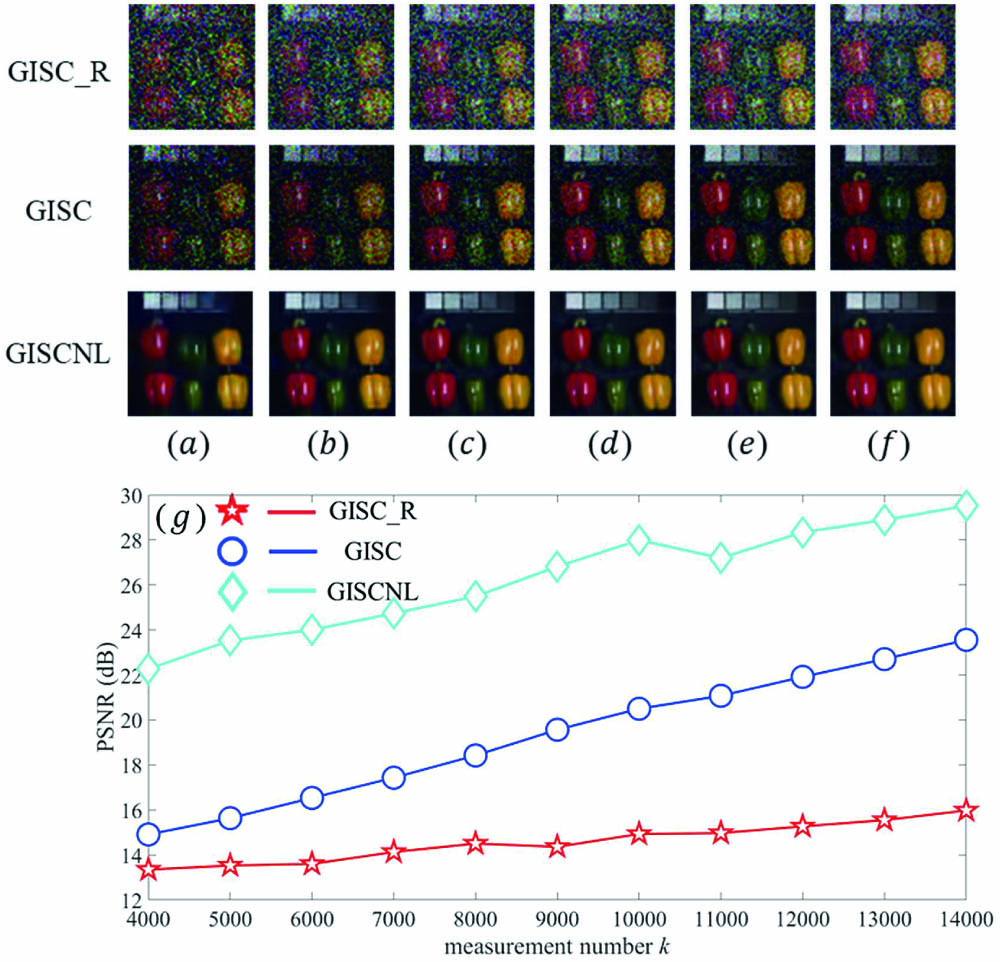
Fig. 4. Effect of measurement number k on the reconstruction quality when the detection . The first row is the results of GISC_R. The second row is the results of GISC. The third row is the results of GISCNL. (a)–(f) Corresponding reconstruction results when the measurement number k is 4000, 6000, 8000, 10,000, 12,000, and 14,000, respectively. ( ) The curve of PSNR versus k.
In order to verify the robust capacity of the reconstruction algorithms, the corresponding reconstruction results are shown in Fig. 5 when the measurement number is fixed, and the detection SNR is 15 dB, 20 dB, 25 dB, 30 dB, 35 dB, and 40 dB, respectively. It is obviously observed that the reconstruction quality is stable for GISCNL when the detection , whereas GISC is still worse than GISCNL even if the detection SNR reaches 40 dB, which suggests that GISCNL has a good robustness even if the sampling number is far below the Nyquist limit.
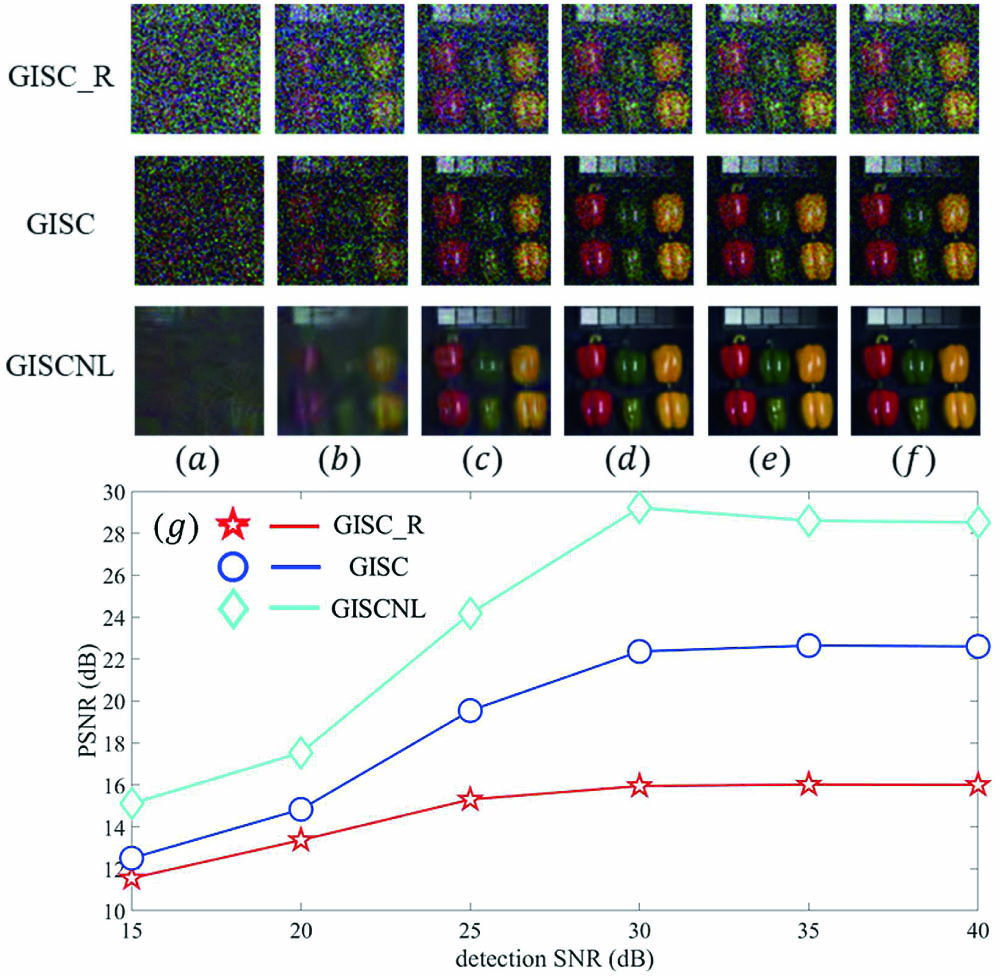
Fig. 5. Influence of detection SNR on the reconstruction quality when k . The first row is the results of GISC_R. The second row is the results of GISC. The third row is the results of GISCNL. (a)–(f) Corresponding reconstruction results when the detection SNR is 15 dB, 20 dB, 25 dB, 30 dB, 35 dB, and 40 dB, respectively. (g) The curve of PSNR versus detection SNR.
4. Conclusions
In summary, we proposed a novel color GI approach to recover a colored scene, and the non-local self-similarity of targets is also utilized in the image reconstruction process. The simulation results demonstrate that the reconstruction quality can be obviously enhanced, and GISCNL is always better than GISC in the same conditions. Although the validity of GISCNL is verified by color GI, the method can be extended to multi-spectral imaging, and the imaging resolution in both the spatial and spectral domains may be also improved because much more a priori knowledge has been used. This technique will also be helpful in promoting the application of GI.
[4] M. Angelo, Y. Shih. Quantum imaging. Laser Phys. Lett., 2005, 2: 567.
[5] A. Gatti, M. Bache, D. Magatti, E. Brambilla, F. Ferri, L. Lugiato. Coherent imaging with pseudo-thermal incoherent light. J. Mod. Opt., 2006, 53: 739.
[17] D. Pelliccia, A. Rack, M. Scheel, V. Cantelli, D. Paganin. Experimental X-ray ghost imaging. Phys. Rev. Lett., 2016, 117: 219902.
[18] D. Duan, S. Du, Y. Xia. Multiwavelength ghost imaging. Phys. Rev. A, 2013, 88: 053842.
[28] W. Gong. High-resolution pseudo-inverse ghost imaging. Photon. Res., 2015, 3: 234.
Article Outline
Pengwei Wang, Chenglong Wang, Cuiping Yu, Shuai Yue, Wenlin Gong, Shensheng Han. Color ghost imaging via sparsity constraint and non-local self-similarity[J]. Chinese Optics Letters, 2021, 19(2): 021102.