大气气溶胶粒径分布的多波长激光雷达反演
Atmospheric aerosols play a crucial role in climate change and atmospheric pollution. Multi-wavelength Raman lidars and lidars with high spectral resolution can accurately measure aerosol extinction and backscatter coefficients for retrieving aerosol particle size distribution, volume concentration, effective radius, and other microphysical properties, which is significant for studying regional and global ecological environments. However, retrieval errors exist in the extinction and backscattering coefficients detected by lidars. When the aerosol microphysical properties are retrieved, the number of unknown parameters required to be solved is often greater than that of optical measurement channels, which is a typical ill-posed inverse problem. As the retrieved results show significant uncertainty in some cases, additional constraints should be introduced to improve the retrieval stability. We propose an advanced regularization retrieval algorithm that introduces a priori mode radius range as a constraint to improve the retrieval accuracy of particle size distribution parameters of different aerosol types.
In our study, an advanced retrieval algorithm for aerosol microphysical properties based on the regularization method is developed. The entire algorithm process is shown in Fig. 1. Based on the Tikhonov regularization retrieval, the reliable retrieval of microphysical particle properties can be realized with a combined data set of particle backscattering coefficients at 355, 532, and 1064 nm and extinction coefficients at 355 nm and 532 nm. Generally, only those solutions for which the optical discrepancy term takes its minimum are selected in retrieval, but here all individual solutions that are within a certain range around this minimum solution are averaged. As a result, the retrieval stability can be improved. Additionally, referring to the aerosol models from the AERONET database, we obtain the volume mode radius ranges of coarse and fine mode aerosols. By employing this as a priori constraint, further selection is performed on the reconstructed particle size distribution to obtain the final retrieved results after averaging.
To test the effectiveness of a priori mode radius constraints on improving the retrieval accuracy of particle size distribution, we conduct the simulations of four typical tropospheric aerosol types: (i) urban aerosols, (ii) smoke aerosols, (iii) desert dust aerosols, and (iv) marine aerosols, with parameters derived from observation data from several AERONET stations. Fig. 2 compares the distribution changes of reconstructed particle sizes after introducing a priori constraints. Meanwhile, Table 2 quantitatively compares the results in Fig. 2 by adopting mean relative errors as the evaluation index. The comparison results indicate that introducing mode radius constraints significantly improves the retrieval results of coarse mode aerosols. Referring to the range of aerosol microphysical parameters (Table 3) given in historical data, we generate 1500 sets of bimodal log-normal distribution data to test the algorithm. Considering the effect of 20% random Gaussian noise, the relative errors of the retrieved effective radius, volume concentration, and surface area concentration are controlled within the range of ±33%,±45%,and ±50% respectively in the cases over 90%. This indicates that the algorithm has sound stability and can tolerate input error effects within a certain range.
We propose an advanced retrieval algorithm for aerosol microphysical properties based on the regularization method, which significantly improves the stability and accuracy of retrieval and solves the problem of large retrieved errors in some cases. The proposed algorithm improves the remote detection technology of aerosols by multi-wavelength lidars. These measurements can provide accurate information about aerosol microphysical properties. The vertical profile of aerosol parameters obtained from lidar detection can be a great improvement of aerosol modeling, which will help study the influence of aerosols on climate and environment.
1 引言
大气气溶胶是由大气介质和混在其中的固态或液态微粒组成的体系[1]。气溶胶可以通过直接效应影响地球-大气系统的辐射收支平衡,也可以通过改变云相态来间接影响区域天气和全球气候[2-3]。然而,准确量化这些气溶胶效应是十分困难的。2021年发布的联合国政府间气候变化专门委员会第六次气候变化评估报告显示,气溶胶对气候变化的影响强度还存在相当大的不确定性。为了准确评估气溶胶的辐射强度,可通过测量粒径分布(PSD)和复折射率等气溶胶微物理特性获得单次散射反照率(SSA)[4],进而研究气溶胶对气候的具体影响。因此,对气溶胶的微物理特性进行观测是一项十分有必要、有价值的研究。
尽管目前地面原位观测和被动遥感仪器已经能够获得气溶胶的光学特性和质量浓度等信息[1,5],但对于气溶胶垂直分布的认识仍然不足。研究气溶胶的垂直分布对于了解指定高度大气的污染水平、分析大气污染机制、厘清云与气溶胶相互作用的机理等方面有很大帮助[6-10]。激光雷达是一种能有效观测气溶胶的主动遥感仪器,它能够以高时空分辨率探测气溶胶的垂直分布信息,并实现全天时连续观测,通过联合观测多个不同波长通道还可进一步反演气溶胶的微物理特性[11-15]。
使用多波长激光雷达反演气溶胶微物理特性的研究自20世纪末以来已经取得长足的进步。德国莱布尼兹对流层研究所从多波长Raman-Mie激光雷达观测数据中成功反演了气溶胶粒径分布和复折射率,该激光雷达能提供6个波长的后向散射系数和2个波长的消光系数[11, 16]。俄罗斯普通物理所Veselovskii等[12, 17]使用2个消光系数和3个后向散射系数对反演解进行了平均处理,提高了正则化反演算法的精度和稳定性。西安理工大学Di等[18]采用正则化方法反演了不同垂直高度下的气溶胶微物理特性参数。除了被广泛应用的正则化方法[11-12],还有多种反演算法被应用于这一领域,如主成分分析法[19]、线性评估法[20]、排列搜索平均法[21]等。
由于激光雷达探测的消光和后向散射系数存在一定反演误差,且在反演气溶胶的微物理特性时,通常要求解的未知量个数大于光学特性的测量通道数,这属于典型的欠定问题,其反演结果在某些情况下表现出很大的不确定性,因此需要进一步的约束以提高其稳定性。本文提出一种改进的正则化反演方法,引入先验的模式半径分布范围作为约束,使得粒径分布的反演精度得到显著改善,能够准确地反演不同类型气溶胶的粒径分布参数。
2 反演方法
2.1 反演原理
355、532、1064 nm的多波长激光雷达常被用于探测气溶胶并反演其光学特性与微物理特性,该系统能够提供355、532、1064 nm波长处的后向散射系数(
气溶胶光学特性通过第一类Fredholm积分方程组与气溶胶粒子的粒径分布相关,其关系为
式中:
为了求解Fredholm积分方程组,使用应用广泛的正则化方法[11-12]。
式中:
式中:
若将权重系数
式中:
气溶胶的复折射率对光学特性影响显著,虽然正则化方法不能直接反演复折射率,但可以在不同复折射率和半径区间组成的查找表(LUT)内尝试多次反演粒径分布,然后在众多反演结果中选择最优解,从而确定复折射率。本研究通过比较输入光学特性参数
式中:
式中:
根据反演时采用的核函数类型,平均解
2.2 模式半径约束
使用多波长激光雷达系统探测不同波段的气溶胶光学特性进而反演其微物理特性的前提是,发射激光对粒径尺寸与波长相当的气溶胶粒子具有较强的响应且表现出一定的波长依赖性。在当前激光雷达系统广泛使用的“
尽管正则化方法无需对气溶胶的粒子谱分布形状进行假设即可反演气溶胶的微物理特性,但由于需要求解的未知量个数往往大于气溶胶光学特性的测量通道数,其求解过程仍存在欠定和对噪声敏感的问题。前文已经介绍了从所有备选解中选择部分合适的解进行平均以减少振荡的优化方法,但仅将重构光学特性参数和输入光学特性参数之间的相对误差作为筛选条件并不是总能获得令人满意的反演结果。
为了进一步提高双峰粒子谱分布的反演精度,可引入一些额外的先验信息作为约束。本文选择使用粗细模态的体积中值半径范围作为约束条件。根据AERONET(Aerosol robotic network)提供的全球各站点历史观测数据库[18, 27]的数据,细模态气溶胶体积中值半径范围为0.12~0.18 μm,而粗模态的体积中值半径则在1.9~3.7 μm范围内变化。基于这一先验知识,在经过光学特性相对误差筛选之后,可进一步对重构的粒径分布PSD进行筛选并取平均值,得到气溶胶的微物理特性反演结果,整个算法的流程如

图 1. 先验模式半径约束下的气溶胶微物理特性反演流程图
Fig. 1. Inversion flow chart of aerosol microphysical properties under a priori model radius constraints
3 结果与讨论
3.1 引入约束前后的反演结果对比
为检验先验模式半径约束对粒径分布反演精度的提升效果,仿真4种典型的对流层气溶胶:在人口稠密的城市与工业区因燃烧化石燃料产生的城市气溶胶;因森林和草原火灾产生的烟尘气溶胶;在沙漠和干旱地区因风蚀和扬沙产生的沙尘气溶胶;因海浪飞溅释放的海盐等物质组成的海洋气溶胶。上述气溶胶参数来自全球AERONET站点的观测数据[27],如
表 1. 双峰对数正态分布气溶胶的模拟参数
Table 1. Simulated parameters of bimodal log-normal aerosols
|
式中:
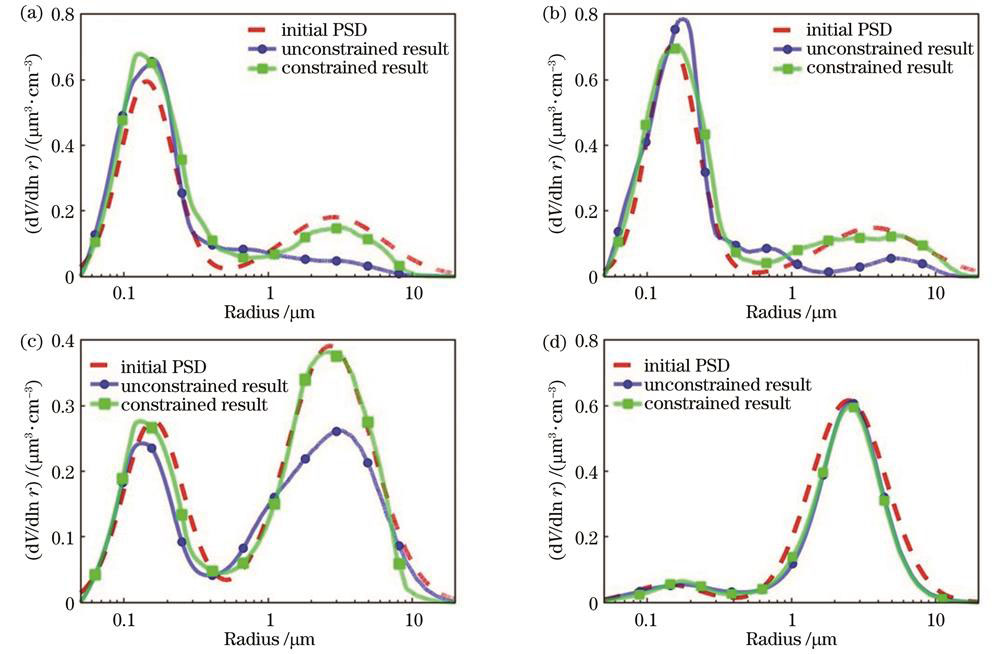
图 2. 4种气溶胶PSD的仿真反演结果。(a)城市气溶胶;(b)烟尘气溶胶;(c)海洋气溶胶;(d)沙尘气溶胶
Fig. 2. Retrieved aerosol PSD for four aerosols. (a) Urban aerosol; (b) smoke aerosol; (c) marine aerosol; (d) desert dust aerosol
为了量化对比引入先验模式半径约束前后重构谱分布与输入初始谱分布的相似程度,用平均相对误差来衡量两种方法得到的不同类型气溶胶的反演误差,其结果见
表 2. 两种方法求得的重构谱分布与初始谱分布之间的平均相对误差
Table 2. MRE between initial size distributions and reconstructed size distributions obtained by two methods
|
从上述PSD反演结果可以看出,在未经模式半径先验约束的情况下,
3.2 输入光学特性中的噪声对反演的影响
参考历史数据中给出的大气气溶胶微物理特性参数的分布范围[18, 27],生成约1500组不同类型的双峰对数正态分布数据对算法进行测试。测试过程中选取的微物理特性参数范围如
表 3. 仿真测试中选用的双峰对数正态分布参数范围
Table 3. Parameter range of bimodal log-normal aerosols chosen in simulation tests
|
在多波长激光雷达的实际观测过程中,系统误差和探测回波信号的随机噪声是不可避免的,通常认为输入光学特性参数的反演误差可控制在20%以内[12, 16]。为了更加准确和全面地评估算法的性能,在测试使用的光学特性输入数据集中引入高斯噪声作为随机误差(不确定度为10%或20%)[12, 18, 30]。假设高斯噪声的不确定度为
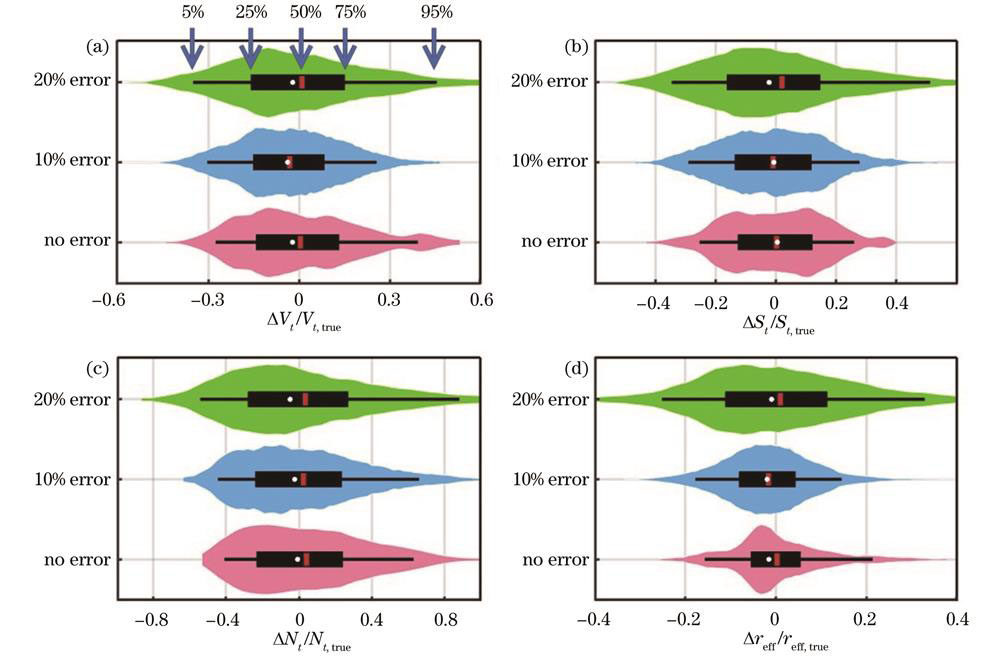
图 3. 气溶胶微物理特性的反演误差统计结果。 (a)体积浓度 ;(b)表面积浓度 ;(c)数浓度 ;(d)有效半径
Fig. 3. Statistical results of inversion errors of aerosol microphysical properties. (a) Volume concentration ; (b) surface area concentration ; (c) number concentration ; (d) effective radius
从
4 结论
基于三波长激光雷达所能提供的
值得注意的是,所提出的改进正则化算法主要针对气溶胶粒子谱分布反演进行优化,旨在实现对大粒径范围内双峰气溶胶粒径分布的精确反演,其在复折射率的反演上仍存在一定误差,亟待进一步改进。
[1] 毛节泰, 张军华, 王美华. 中国大气气溶胶研究综述[J]. 气象学报, 2002, 60(5): 625-634.
Mao J T, Zhang J H, Wang M H. Summary comment on research of atmospheric aerosl in China[J]. Acta Meteorologica Sinica, 2002, 60(5): 625-634.
[2] Giorgi F, Bi X Q, Qian Y. Direct radiative forcing and regional climatic effects of anthropogenic aerosols over East Asia: a regional coupled climate-chemistry/aerosol model study[J]. Journal of Geophysical Research: Atmospheres, 2002, 107(D20): AAC7-1.
[3] 尚倩, 李子华, 杨军, 等. 南京冬季大气气溶胶粒子谱分布及其对能见度的影响[J]. 环境科学, 2011, 32(9): 2750-2760.
Shang Q, Li Z H, Yang J, et al. Size distributions of aerosol particles and the impact on visibility in winter of Nanjing[J]. Environmental Science, 2011, 32(9): 2750-2760.
[4] 范学花, 陈洪滨, 夏祥鳌. 中国大气气溶胶辐射特性参数的观测与研究进展[J]. 大气科学, 2013, 37(2): 477-498.
Fan X H, Chen H B, Xia X A. Progress in observation studies of atmospheric aerosol radiative properties in China[J]. Chinese Journal of Atmospheric Sciences, 2013, 37(2): 477-498.
[5] Che H Z, Gui K, Xia X G, et al. Large contribution of meteorological factors to inter-decadal changes in regional aerosol optical depth[J]. Atmospheric Chemistry and Physics, 2019, 19(16): 10497-10523.
[6] Zhu J, Zhu B, Huang Y, et al. PM2.5 vertical variation during a fog episode in a rural area of the Yangtze River Delta, China[J]. Science of the Total Environment, 2019, 685: 555-563.
[7] Liu C, Huang J P, Wang Y W, et al. Vertical distribution of PM2.5 and interactions with the atmospheric boundary layer during the development stage of a heavy haze pollution event[J]. Science of the Total Environment, 2020, 704: 135329.
[10] Huang Z T, Chang C Y, Chen K P, et al. Tunable lasing direction in one-dimensional suspended high-contrast grating using bound states in the continuum[J]. Advanced Photonics, 2022, 4(6): 066004.
[11] Müller D, Wandinger U, Ansmann A. Microphysical particle parameters from extinction and backscatter lidar data by inversion with regularization: theory[J]. Applied Optics, 1999, 38(12): 2346-2357.
[12] Veselovskii I, Kolgotin A, Griaznov V, et al. Inversion with regularization for the retrieval of tropospheric aerosol parameters from multiwavelength lidar sounding[J]. Applied Optics, 2002, 41(18): 3685-3699.
[13] 王玉峰, 高飞, 朱承炫, 等. 对流层高度大气温度、湿度和气溶胶的拉曼激光雷达系统[J]. 光学学报, 2015, 35(3): 0328004.
[16] Müller D, Wandinger U, Ansmann A. Microphysical particle parameters from extinction and backscatter lidar data by inversion with regularization: simulation[J]. Applied Optics, 1999, 38(12): 2358-2368.
[17] Veselovskii I, Kolgotin A, Griaznov V, et al. Inversion of multiwavelength Raman lidar data for retrieval of bimodal aerosol size distribution[J]. Applied Optics, 2004, 43(5): 1180-1195.
[18] Di H G, Wang Q Y, Hua H B, et al. Aerosol microphysical particle parameter inversion and error analysis based on remote sensing data[J]. Remote Sensing, 2018, 10(11): 1753.
[19] Donovan D P, Carswell A I. Principal component analysis applied to multiwavelength lidar aerosol backscatter and extinction measurements[J]. Applied Optics, 1997, 36(36): 9406-9424.
[20] Veselovskii I, Dubovik O, Kolgotin A, et al. Linear estimation of particle bulk parameters from multi-wavelength lidar measurements[J]. Atmospheric Measurement Techniques, 2012, 5(5): 1135-1145.
[21] Chemyakin E, Müller D, Burton S, et al. Arrange and average algorithm for the retrieval of aerosol parameters from multiwavelength high-spectral-resolution lidar/Raman lidar data[J]. Applied Optics, 2014, 53(31): 7252-7266.
[22] Ansmann A, Riebesell M, Wandinger U, et al. Combined Raman elastic-backscatter LIDAR for vertical profiling of moisture, aerosol extinction, backscatter, and LIDAR ratio[J]. Applied Physics B, 1992, 55(1): 18-28.
[23] Liu D, Yang Y Y, Cheng Z T, et al. Retrieval and analysis of a polarized high-spectral-resolution lidar for profiling aerosol optical properties[J]. Optics Express, 2013, 21(11): 13084-13093.
[24] Xiao D, Wang N C, Shen X E, et al. Development of ZJU high-spectral-resolution lidar for aerosol and cloud: extinction retrieval[J]. Remote Sensing, 2020, 12(18): 3047.
[25] BohrenC F, HuffmanD R. Absorption and scattering by a sphere[M]//Absorption and scattering of light by small particles. Singapore: John Wiley & Sons Inc, 1998: 82-129.
[26] Mishchenko M I, Travis L D, Kahn R A, et al. Modeling phase functions for dustlike tropospheric aerosols using a shape mixture of randomly oriented polydisperse spheroids[J]. Journal of Geophysical Research: Atmospheres, 1997, 102(D14): 16831-16847.
[27] Dubovik O, Holben B, Eck T F, et al. Variability of absorption and optical properties of key aerosol types observed in worldwide locations[J]. Journal of the Atmospheric Sciences, 2002, 59(3): 590-608.
[28] 卫晓东, 张华. 非球形沙尘气溶胶光学特性的分析[J]. 光学学报, 2011, 31(5): 0501002.
[29] 周妹, 常建华, 陈思成, 等. 一种基于朴素贝叶斯分类器的气溶胶类型识别模型[J]. 光学学报, 2022, 42(18): 1801006.
[30] Wang N C, Xiao D, Veselovskii I, et al. This is FAST: multivariate Full-permutAtion based Stochastic foresT method: improving the retrieval of fine-mode aerosol microphysical properties with multi-wavelength lidar[J]. Remote Sensing of Environment, 2022, 280: 113226.
[31] Böckmann C. Hybrid regularization method for the ill-posed inversion of multiwavelength lidar data in the retrieval of aerosol size distributions[J]. Applied Optics, 2001, 40(9): 1329-1342.
[32] Müller D, Wagner F, Althausen D, et al. Physical properties of the Indian aerosol plume derived from six-wavelength lidar Observations on 25 March 1999 of the Indian Ocean Experiment[J]. Geophysical Research Letters, 2000, 27(9): 1403-1406.
李晓涛, 刘东, 肖达, 张凯, 胡先哲, 李蔚泽, 毕磊, 孙文波, 吴兰, 刘崇, 邓洁松. 大气气溶胶粒径分布的多波长激光雷达反演[J]. 光学学报, 2024, 44(6): 0601013. Xiaotao Li, Dong Liu, Da Xiao, Kai Zhang, Xianzhe Hu, Weize Li, Lei Bi, Wenbo Sun, Lan Wu, Chong Liu, Jiesong Deng. Retrieval of Aerosol Particle Size Distribution from Multi-Wavelength Lidar[J]. Acta Optica Sinica, 2024, 44(6): 0601013.