激光与光电子学进展, 2020, 57 (22): 221006, 网络出版: 2020-10-24
基于信息熵和梯度因子的改进Criminisi图像修复方法
下载: 935次
An Improved Criminisi Image Inpainting Method Based on Information Entropy and Gradient Factor
图 & 表
图 1. 全局搜索与动态范围搜索的修复效果。(a)原始图像;(b)全局搜索;(c)动态范围搜索
Fig. 1. Inpainting effect of global search and dynamic range search. (a) Original images; (b) global search; (c) dynamic range search
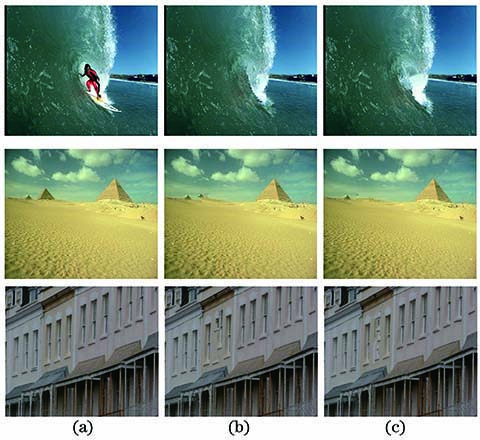
图 3. 不同模块尺寸的修复效果。(a)原始图像;(b) 5×5尺寸;(c) 7×7尺寸;(d) 9×9尺寸;(e) 11×11尺寸
Fig. 3. Inpainting effects of different sizes. (a) Original images; (b) size 5×5; (c) size 7×7; (d) size 9×9; (e) size 11×11

图 4. 提出算法与6种算法修复效果对比图。(a)原始图像;(b) MD算法修复结果;(c) PDE算法修复结果;(d) NN算法修复结果;(e)文献[ 20]算法修复结果;(f) Criminisi算法修复结果;(g)文献[ 3]算法修复结果;(h)本文算法修复结果
Fig. 4. Inpainting effects comparison between six different algorithms and the proposed algorithm. (a) Original images; (b) inpainting results by MD algorithm; (c) inpainting results by PDE algorithm; (d) inpainting results by NN algorithm; (e) inpainting results by Ref.[20] method; (f) inpainting results by Criminisi algorithm; (g) inpainting results by Ref.[3] method; (h) inpainting results by proposed algorithm

表 1不同算法修复效果的评价参数对比
Table1. Inpainting effects comparison of evaluation index for different algorithms
|
表 2不同算法的运行时间对比
Table2. Comparison of running time of different algorithms unit: s
|
王凤随, 刘正男, 付林军. 基于信息熵和梯度因子的改进Criminisi图像修复方法[J]. 激光与光电子学进展, 2020, 57(22): 221006. Fengsui Wang, Zhengnan Liu, Linjun Fu. An Improved Criminisi Image Inpainting Method Based on Information Entropy and Gradient Factor[J]. Laser & Optoelectronics Progress, 2020, 57(22): 221006.