OAM-based diffractive all-optical classification
Object classification is an important aspect of machine intelligence. Current practices in object classification entail the digitization of object information followed by the application of digital algorithms such as deep neural networks. The execution of digital neural networks is power-consuming, and the throughput is limited. The existing von Neumann digital computing paradigm is also less suited for the implementation of highly parallel neural network architectures.1 Diffractive deep neural networks (), also known as diffractive optical networks or diffractive networks, form an all-optical visual processor with the potential to address some of these issues, especially when the information of interest is already represented in the analog domain.2 Comprising a set of spatially engineered thin surfaces that successively modulate the incident light, a diffractive network performs information processing in the analog optical domain, completing its task as the light is transmitted through a passive thin optical volume. This all-optical computing architecture can benefit from the numerous degrees of freedom of light, such as the spectrum, polarization, phase, and amplitude, achieving improved parallelism and performance.3
In their recently published paper, Zhang et al. bring the power of OAM mode multiplexing to all-optical object classification using diffractive optical networks.10 In their framework, information about the input object class is carried by the OAM distribution of the light. The beam that illuminates the object comprises several OAM mode components , one corresponding to each object class, as shown in Fig. 1. The diffractive network is optimized in a data-driven manner using deep learning to reinforce the OAM component corresponding to the class of the input object at the expense of the others. The authors report a blind testing accuracy of 85.49% on the MNIST handwritten digit test dataset. While higher inference accuracies have been achieved on the same dataset using diffractive optical classifiers, for a first attempt at all-optical classification using OAM, this represents a highly promising approach.
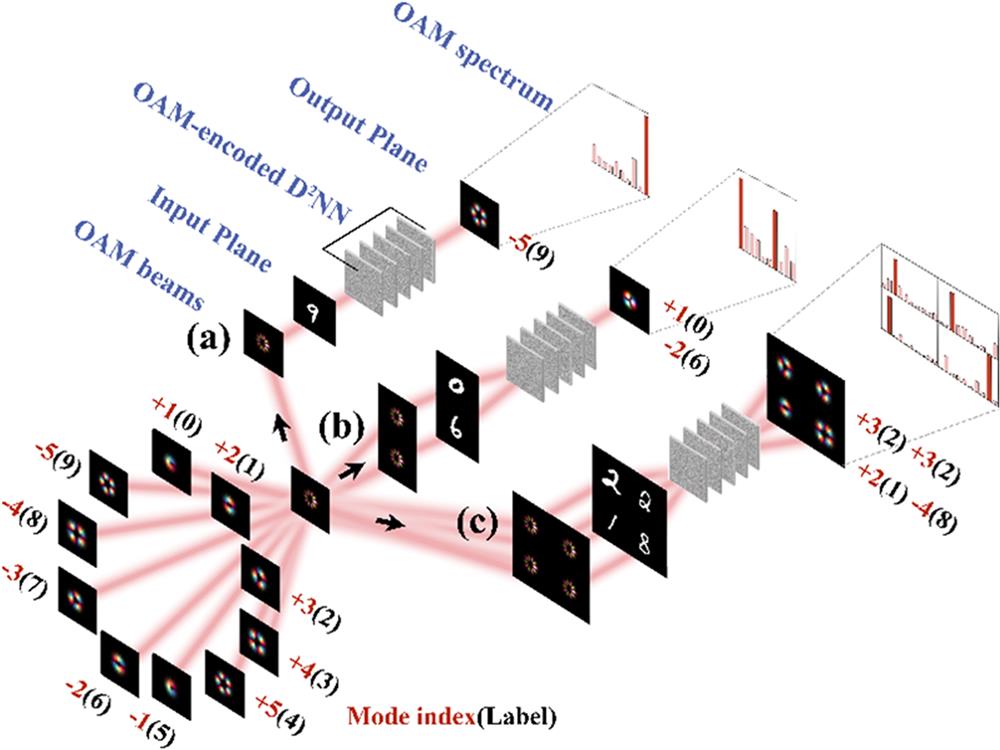
Fig. 1. OAM-based all-optical classification schemes with diffractive deep neural networks ( ). (a) A single classification task with a single detector OAM-encoded . (b) Multiple classification tasks with a single detector OAM-encoded . (c) Multiple classification tasks with a multidetector OAM-encoded . Adapted from Ref. 10.
The authors proceed to demonstrate the multitasking capability of this framework, which was demonstrated earlier using phase-encoding schemes.11 In one realization shown in Fig. 1(b), two different MNIST digits on the input plane are classified using a single detector, i.e., an OAM spectrum analyzer. The two strongest OAM components in the output light represent the classes of the input digits. This single-detector OAM-encoded for two-digit classification achieved a blind testing accuracy of 64.13%. However, this scheme fails for repeating classes, i.e., when both digits belong to the same class. This can be solved using phase-encoding, as demonstrated earlier, even where there is spatial overlap between the input objects.11 As an alternative approach, objects, possibly belonging to the same class, located at distinct positions on the input aperture can be classified by having detectors at the network output, as shown in Fig. 1(c). For example, the reported blind testing accuracy for handwritten digits on the input plane with detectors is 70.94% for and 40.13% for .10
Zhang et al. also showed that OAM-encoded are resilient to misalignment errors, which is a major advantage for practical settings. By multiplexing a multitude of OAM modes on the input beam, it is possible to utilize OAM-encoded for distinguishing among a large number of classes of the input object. For example, earlier work4 reported the use of spectral encoding (with wavelengths) to increase the number of classes that can be processed by a single-pixel diffractive optical network. Following a similar design strategy, it might also be possible to assign a separate task to each OAM mode for the same input scene. However, it will require advanced OAM mode multiplexing techniques, and the success will depend on the precision with which the different modes can be distinguished. The ability to generate OAM combs,12 together with the advancement of fabrication techniques for diffractive networks, could inspire novel OAM-encoded for diverse applications such as sensing, imaging, and communication, taking this exciting work of Zhang et al. into new frontiers.
Md Sadman Sakib Rahman, Aydogan Ozcan. OAM-based diffractive all-optical classification[J]. Advanced Photonics, 2024, 6(1): 010501.