激光与光电子学进展, 2020, 57 (2): 021012, 网络出版: 2020-01-03
面向无人机自主飞行的无监督单目视觉深度估计
下载: 1264次
Unsupervised Monocular Depth Estimation for Autonomous Flight of Drones
图 & 表
图 4. 训练过程中各部分损失函数。(a)重构图像与原图的结构相似性损失;(b)重构图像与原图之差绝对值损失;(c)总的图像重构损失;(d)视差图平滑性损失;(e)左右视差图一致性损失;(f)本模型的总损失
Fig. 4. Loss function of each part of training process. (a) Structural similarity loss of reconstructed image and original image; (b) absolute value loss of difference between reconstructed image and original image; (c) total image reconstruction loss; (d) loss of disparity smoothness; (e) loss of consistency in left and right disparity maps; (f) total loss of our model

图 5. 无人机实验平台。(a)无人机;(b) NVIDIA Jeston TX2与Pixhawk连接
Fig. 5. Platform of drone experiment. (a) Drone; (b) connection of NVIDIA Jeston TX2 and Pixhawk
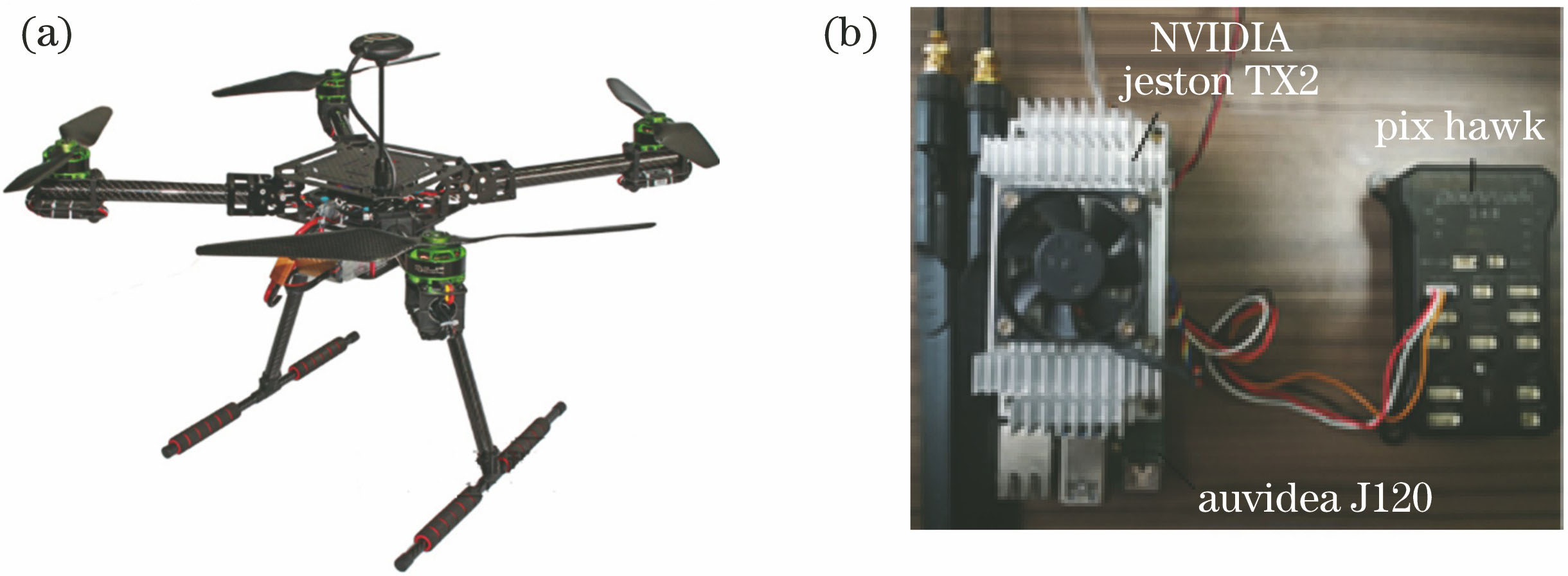
图 6. KITTI数据集上预测深度图实例。(a)输入的图片;(b)真实深度图;(c)文献[ 15]预测的深度图;(d)文献[ 20]预测的深度; (e) 本模型基于VGG-16预测的深度;(f)本模型基于ResNet-50预测的深度
Fig. 6. Examples of depth map predicted on KITTI dataset. (a) Input image; (b) ground truth depth map; (c) depth map predicted by Ref. [15] ; (d) depth map predicted in Ref. [20]; (e) depth map predicted by our model based on VGG-16; (f) depth map predicted by our model based on ResNet-50
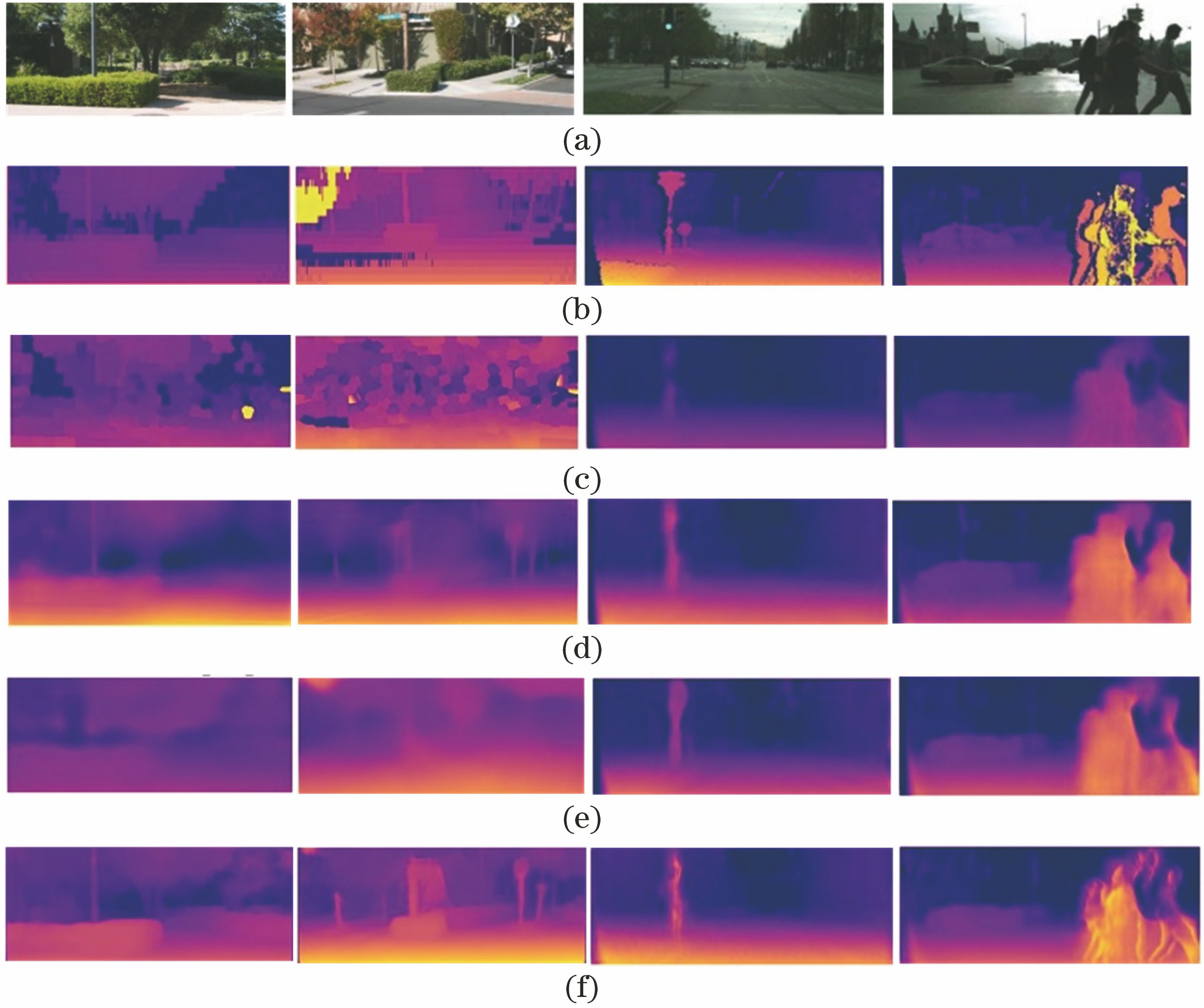
表 1KITTI数据集上实验结果对比
Table1. Comparison of experimental results on KITTI dataset
|
表 2Make3D数据集上实验结果对比
Table2. Comparisonof experimental results on Make3D dataset
|
赵栓峰, 黄涛, 许倩, 耿龙龙. 面向无人机自主飞行的无监督单目视觉深度估计[J]. 激光与光电子学进展, 2020, 57(2): 021012. Zhao Shuanfeng, Huang Tao, Xu Qian, Geng Longlong. Unsupervised Monocular Depth Estimation for Autonomous Flight of Drones[J]. Laser & Optoelectronics Progress, 2020, 57(2): 021012.