光学学报, 2017, 37 (11): 1128001, 网络出版: 2018-09-07
基于深度残差网络的高光谱遥感数据霾监测
下载: 1254次
Hyperspectral Data Haze Monitoring Based on Deep Residual Network
图 & 表
图 1. 苏州地区高光谱数据和站点位置示意图。(a)站点位置示意图;(b) 2015年2月28日150通道; (c) 2015年3月18日179通道
Fig. 1. Diagrams of hyperspectral data and site location of Suzhou. (a) Diagram of station location; (b) February 28, 2015, passage 150; (c) March 18, 2015, passage 179
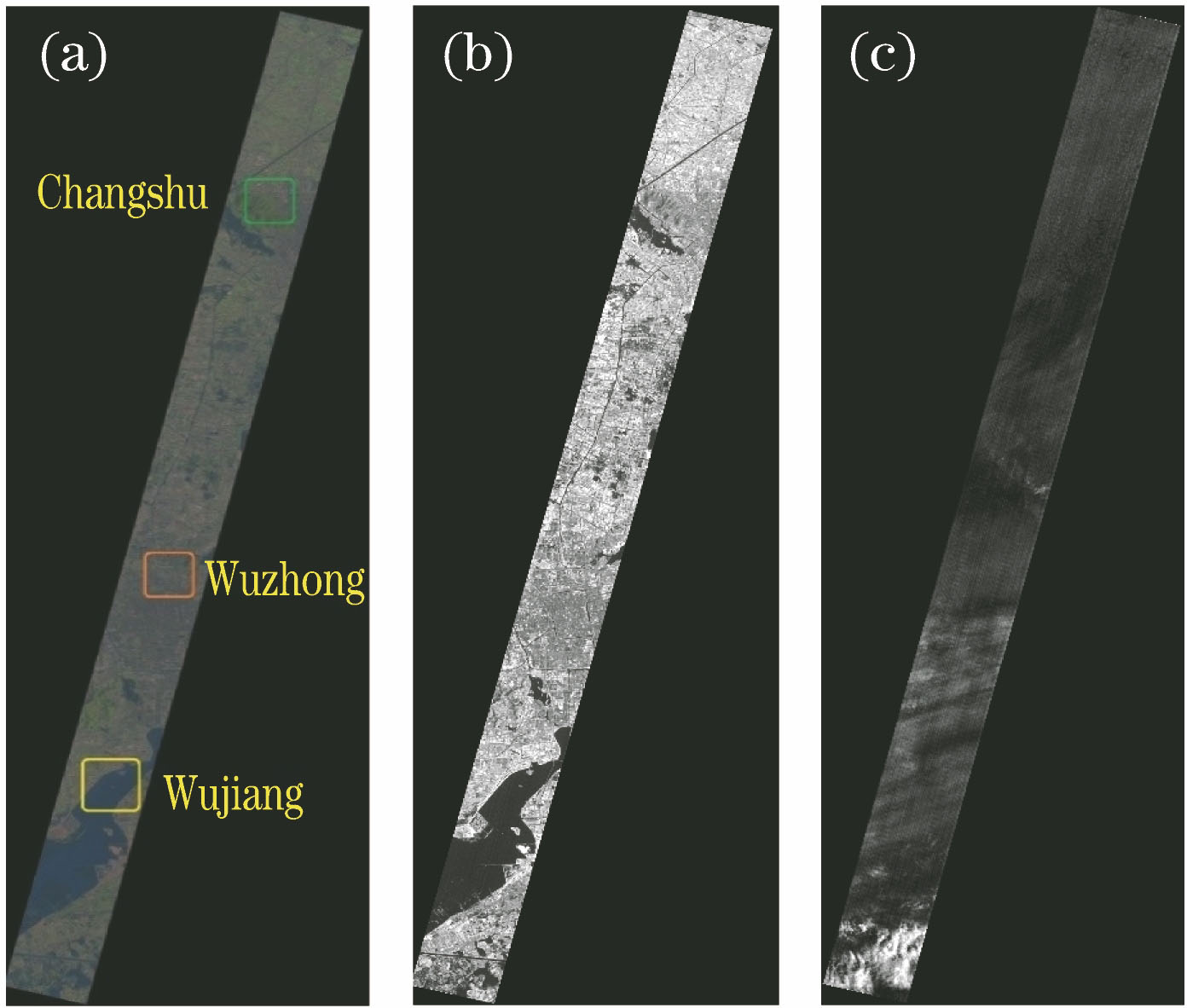
图 2. 苏州城区下垫面下不同霾状况平均光谱曲线
Fig. 2. Average spectral curves at underlying surface of Suzhou under different haze conditions
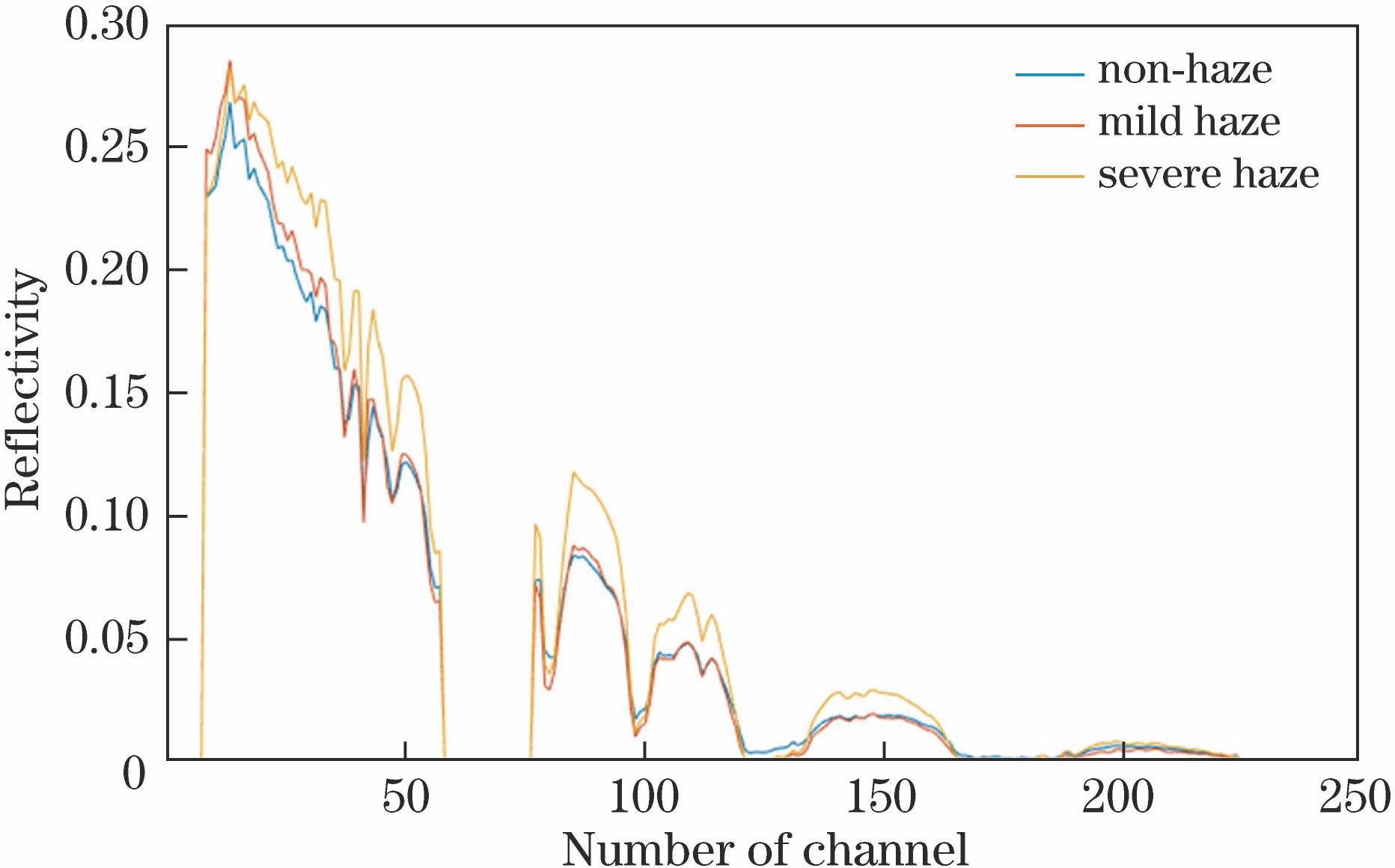
图 3. 霾(红色)与非霾(蓝色)的随机抽样光谱曲线
Fig. 3. Random sampling spectral curves of haze (red) and non-haze (blue)
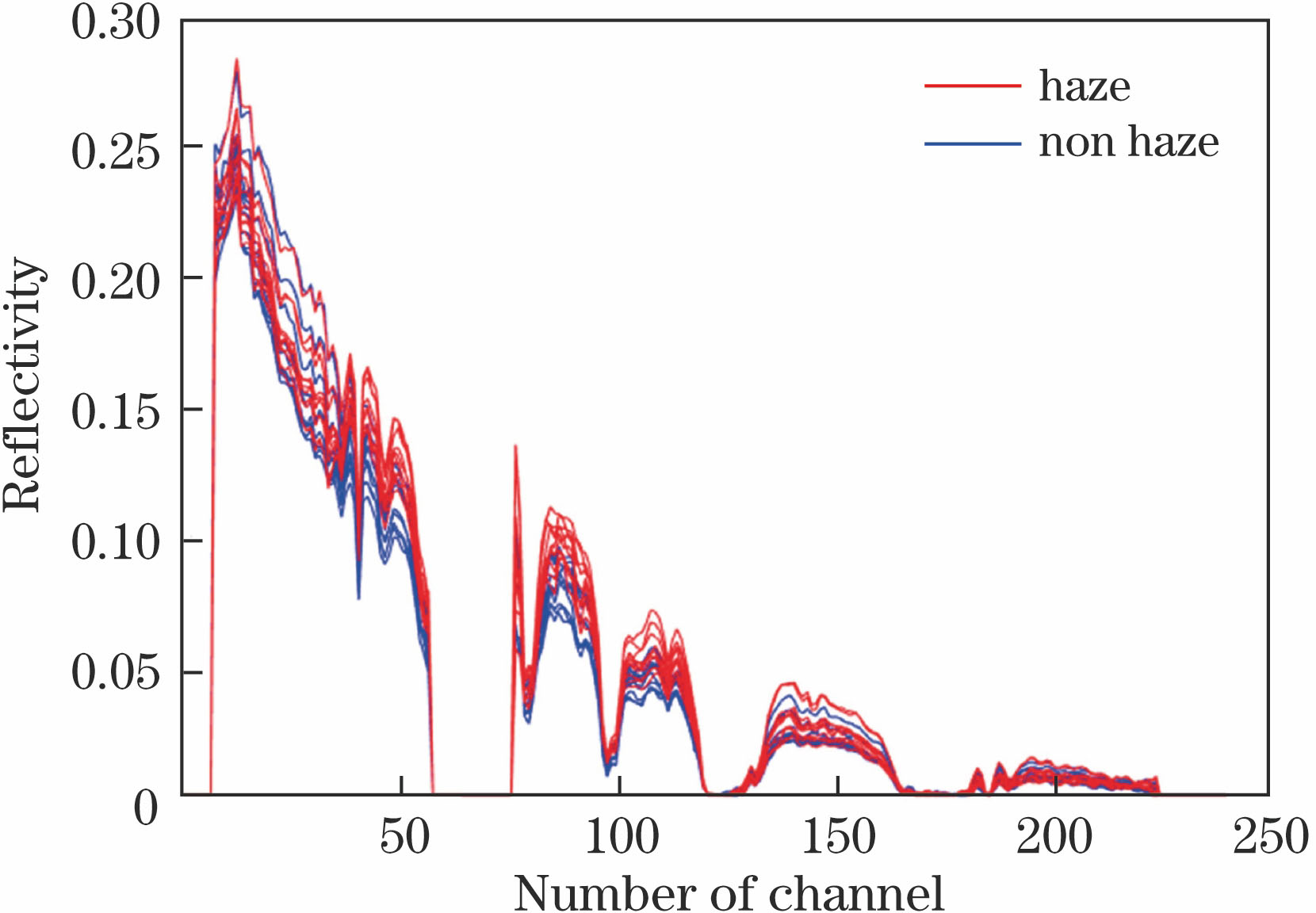
图 4. PCA特征下的霾(红色)与非霾(蓝色)散点图
Fig. 4. Scatter plots of haze (red) and non-haze (blue) with PCA characteristics
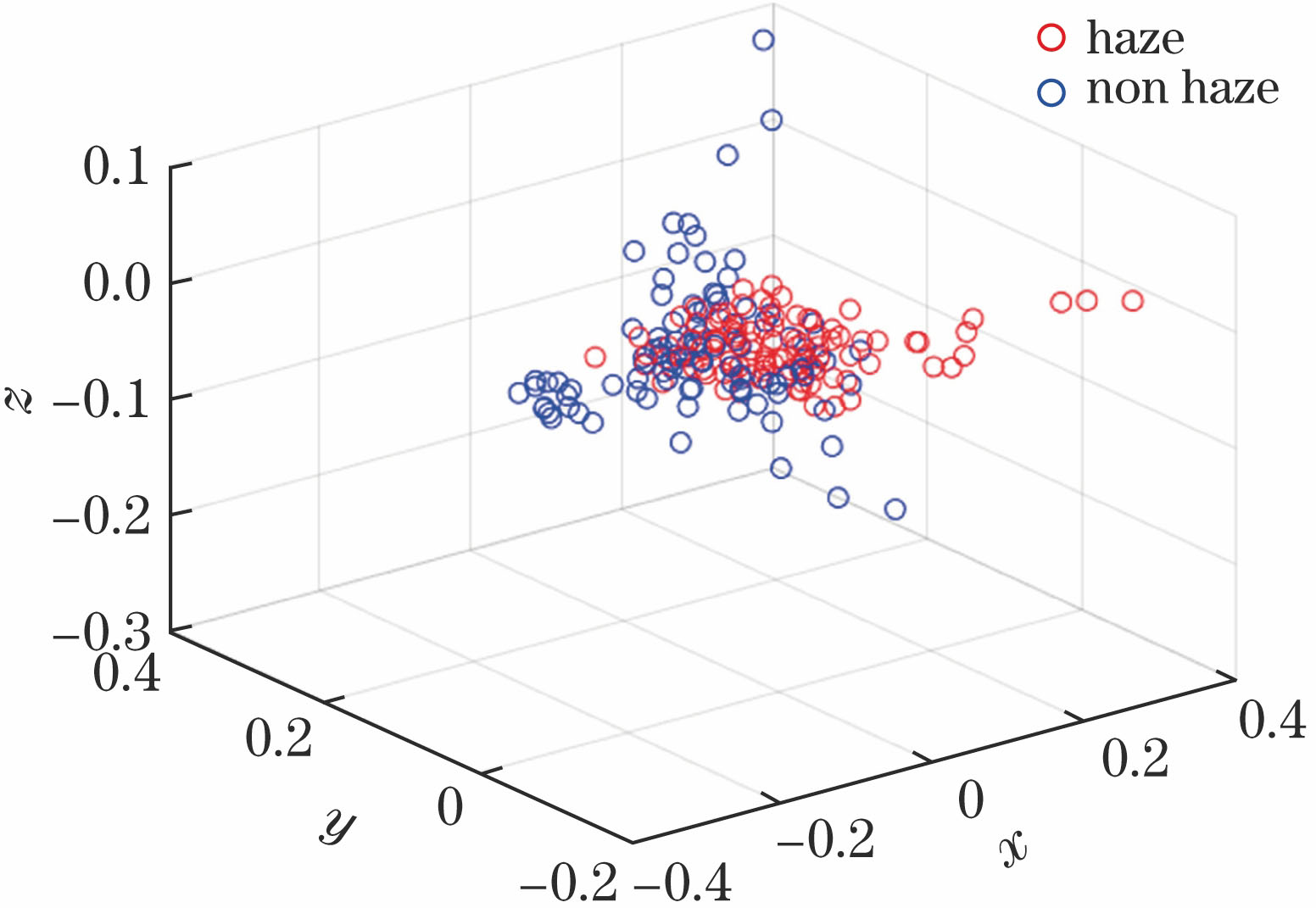
图 6. 高光谱霾监测深度残差网络整体框架
Fig. 6. Framework of deep residual network for hyperspectral haze monitoring
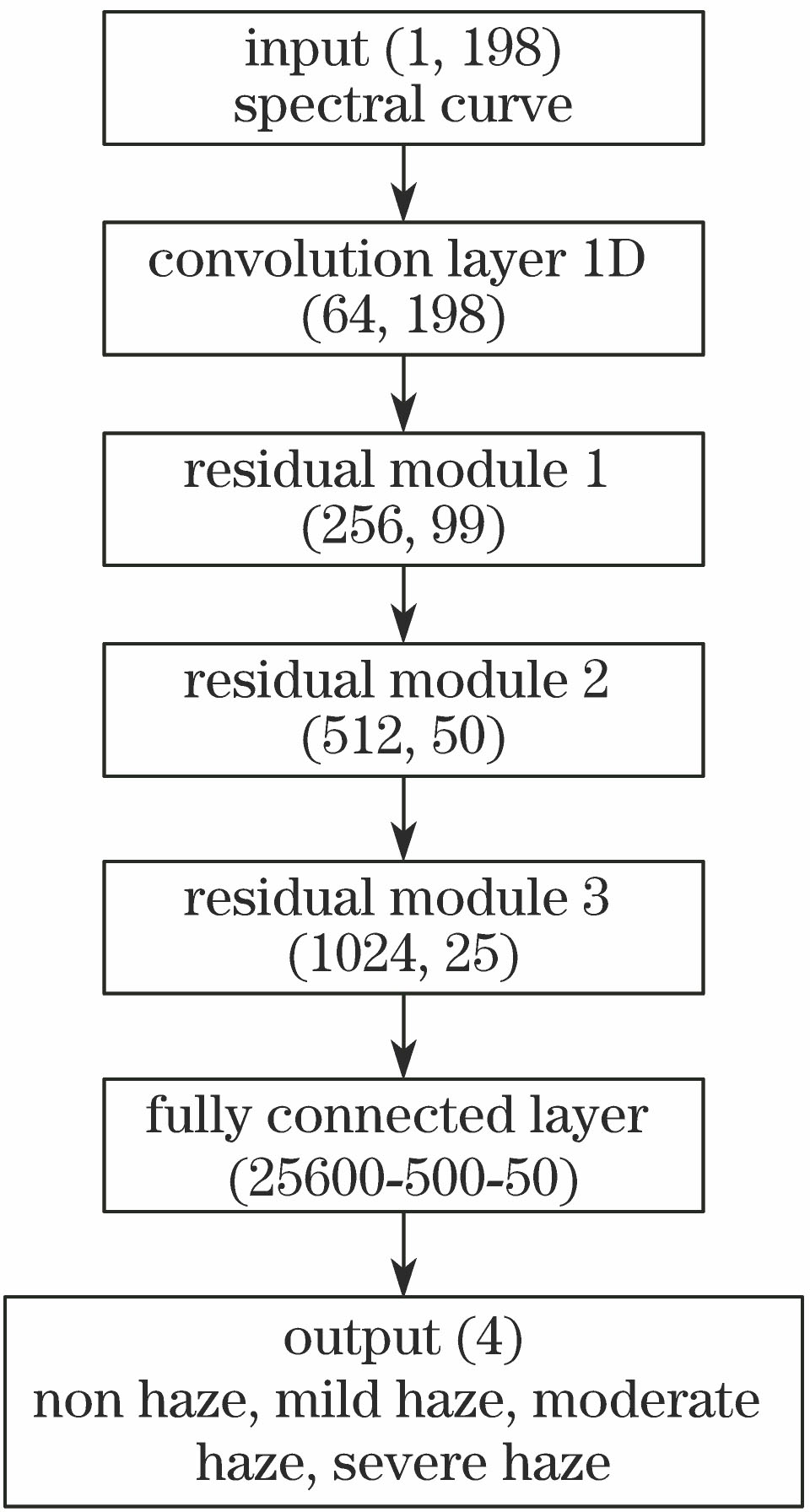
图 8. 不同网络深度下的CNN和ResNet性能对比图
Fig. 8. Comparison of performance of CNN and ResNet with different network depths
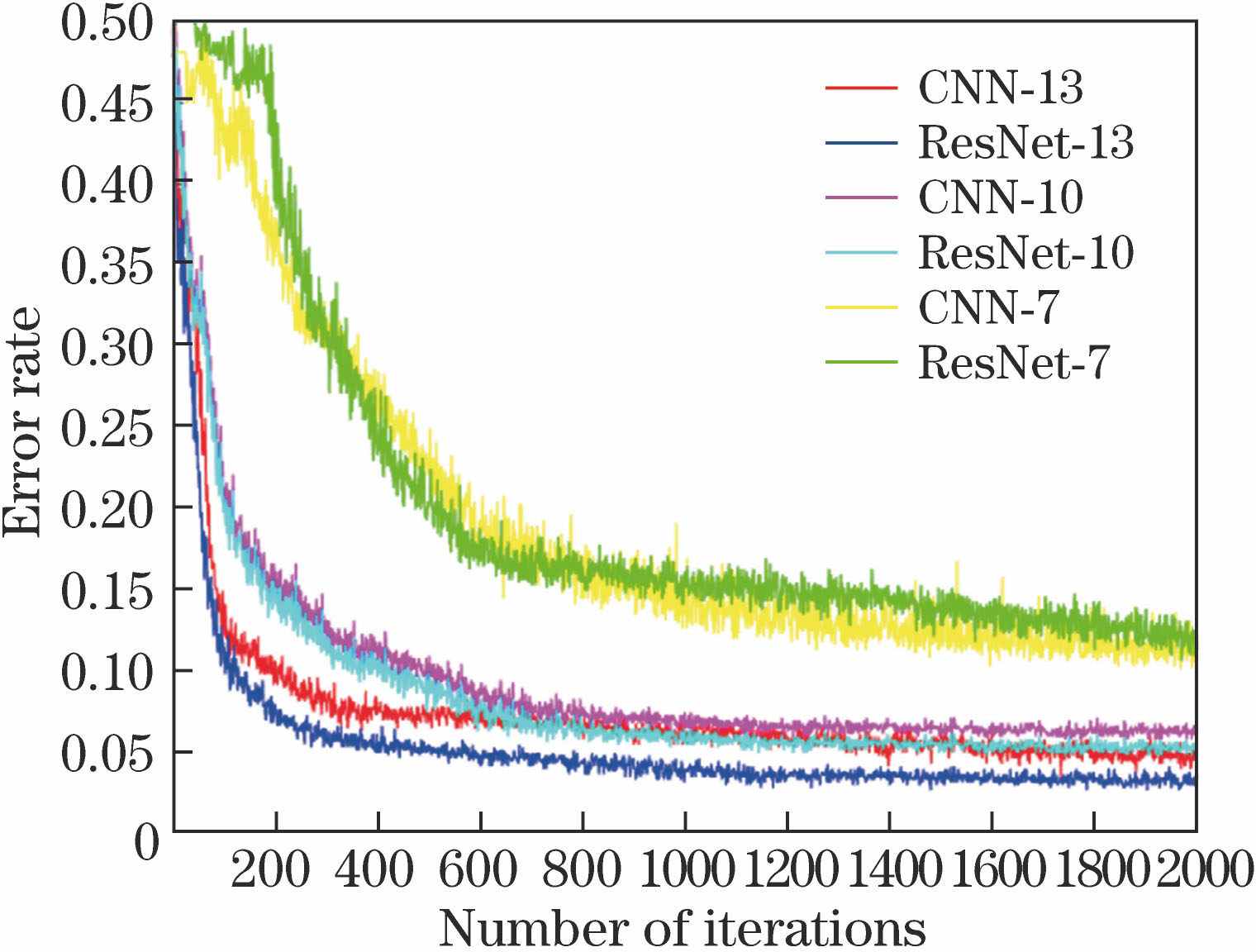
图 10. 大训练样本下网络性能对比结果。(a)训练误差;(b)测试误差
Fig. 10. Comparison of network performance with large training sampling. (a) Training error; (b) test error
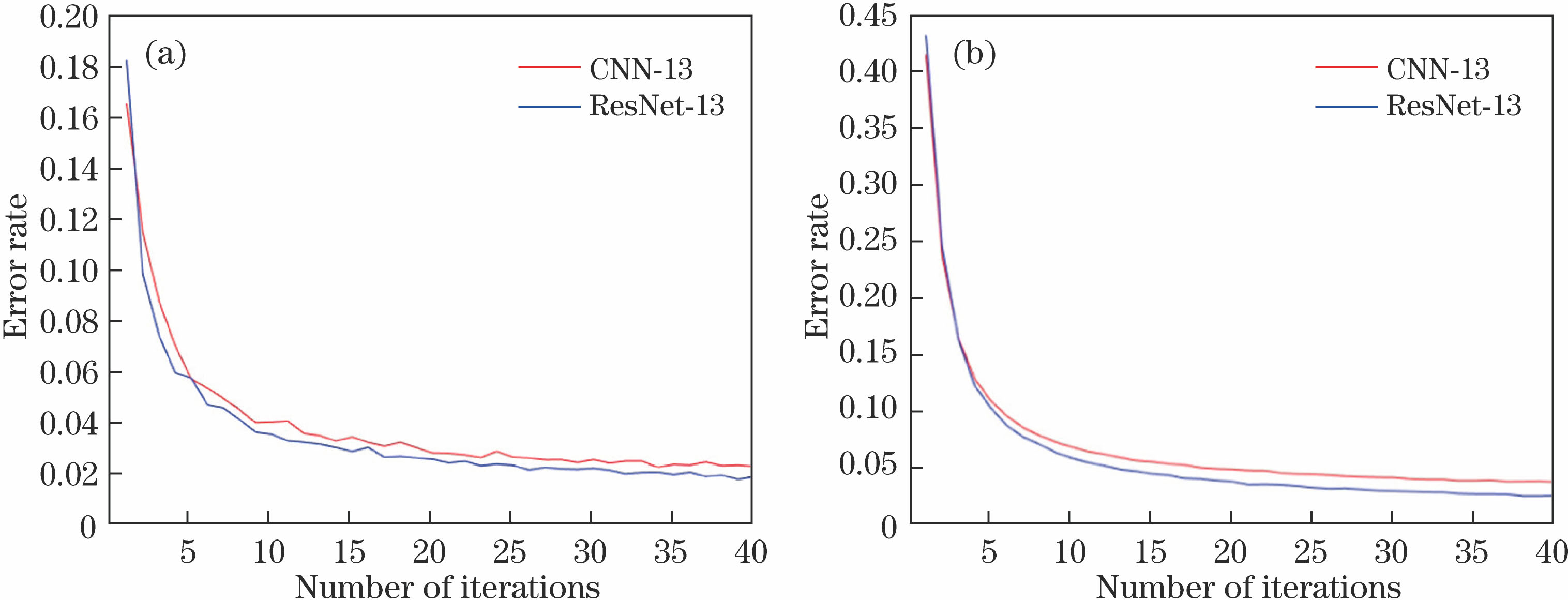
图 11. BP与CNN-13、Resnet-13的大训练样本对比实验结果
Fig. 11. Experiment results of large training samples of BP, CNN-13 and ResNet-13
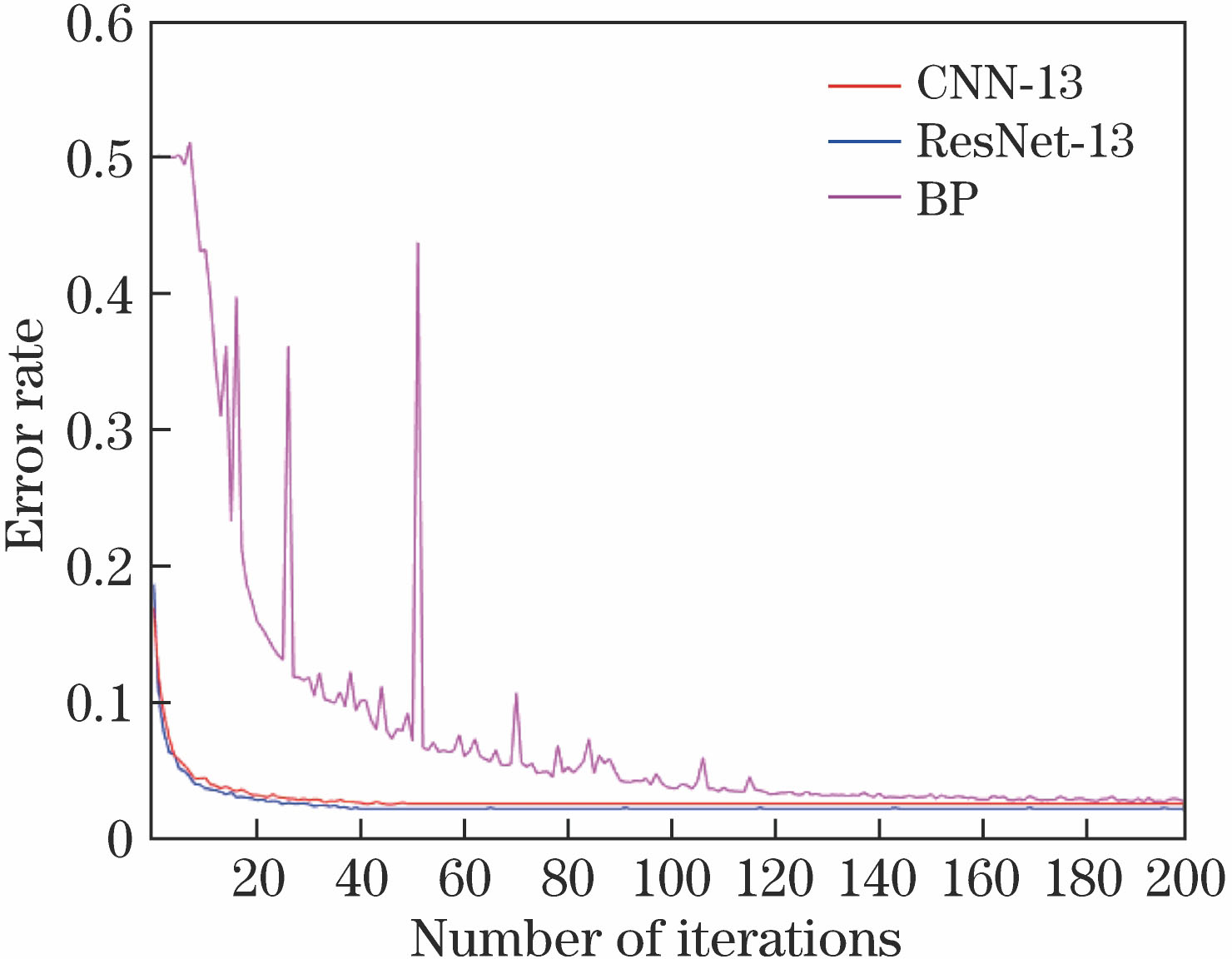
图 12. 苏州地区2015年1月26日霾监测结果。(a) 站点位置示意图;(b) SVM结果;(c) DBN结果;(d) Resnet结果
Fig. 12. Haze monitoring results of Suzhou on January 26, 2015. (a) Diagram of site location; (b) result of SVM; (c) result of DBN; (d) result of Resnet

表 1不同霾识别方法的对比实验结果
Table1. Experimental results of haze recognition with different methods
|
表 2霾分类混淆矩阵
Table2. Confusion matrix of haze classification
|
陆永帅, 李元祥, 刘波, 刘辉, 崔林丽. 基于深度残差网络的高光谱遥感数据霾监测[J]. 光学学报, 2017, 37(11): 1128001. Yongshuai Lu, Yuanxiang Li, Bo Liu, Hui Liu, Linli Cui. Hyperspectral Data Haze Monitoring Based on Deep Residual Network[J]. Acta Optica Sinica, 2017, 37(11): 1128001.