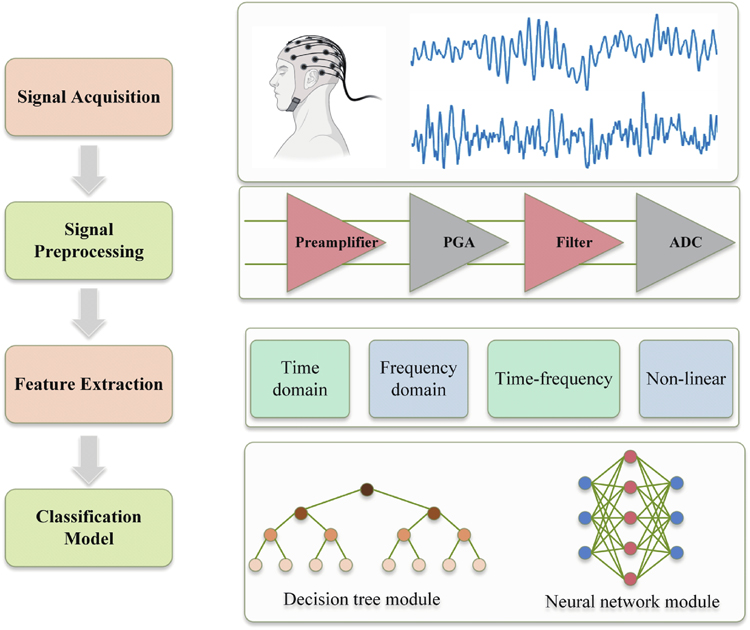
Author Affiliations
Abstract
1 Laboratory of Microelectronic Devices & Integrated Technology, Institute of Microelectronics of the Chinese Academy of Sciences, Beijing 100029, China
2 School of Integrated Circuits, University of Chinese Academy of Sciences, Beijing 101408, China
3 School of Information and Electronics, Beijing Institute of Technology, Beijing 100081, China
Epilepsy is a common neurological disorder that occurs at all ages. Epilepsy not only brings physical pain to patients, but also brings a huge burden to the lives of patients and their families. At present, epilepsy detection is still achieved through the observation of electroencephalography (EEG) by medical staff. However, this process takes a long time and consumes energy, which will create a huge workload to medical staff. Therefore, it is particularly important to realize the automatic detection of epilepsy. This paper introduces, in detail, the overall framework of EEG-based automatic epilepsy identification and the typical methods involved in each step. Aiming at the core modules, that is, signal acquisition analog front end (AFE), feature extraction and classifier selection, method summary and theoretical explanation are carried out. Finally, the future research directions in the field of automatic detection of epilepsy are prospected.
epilepsy electroencephalography automatic detection analog front end feature extraction classifier Journal of Semiconductors
2023, 44(12): 121401
西安工程大学电子信息学院, 陕西 西安 710048
为了提高脑电信号的分类准确率,提出一种基于人工蜂群算法和BP神经网络的分类方法。针对反向传播(BP)神经网络存在全局搜索能力差、对初始权重敏感和人工蜂群算法的搜索公式精于探索但疏于开发等问题,采用全局搜索因子来增强人工蜂群算法的开发能力,再加入交叉运算来解决人工蜂群算法的全局搜索。采用改进的算法来优化BP神经网络对初始权重敏感的问题,进而实现对脑电信号的分类。实验结果表明,所提算法对脑电信号的分类准确率更高,分类准确率达到91.5%,而且可以加快收敛速度。
光计算 神经网络 脑电信号 人工蜂群 交叉运算 激光与光电子学进展
2020, 57(21): 212001