光学学报, 2020, 40 (17): 1710001, 网络出版: 2020-08-24
基于残差块和注意力机制的细胞图像分割方法
下载: 1065次
Cell Image Segmentation Method Based on Residual Block and Attention Mechanism
图 & 表
图 4. 数据集展示。(a)训练集图像;(b)对应真值图像
Fig. 4. Display of dataset. (a) Training set image; (b) corresponding ground truth
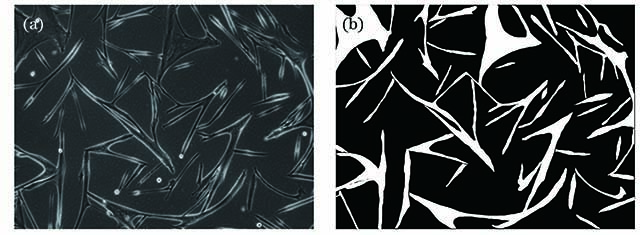
图 6. 第1组细胞分割结果。(a)原始图像;(b)真值图像;(c) Otsu模型;(d) FCN-8s模型;(e) U-Net模型;(f) SegNet模型;(g) R2U-Net模型;(h)所提模型
Fig. 6. Cell segmentation results of group 1. (a) Origin image; (b) ground truth; (c) Otsu model; (d) FCN-8s model; (e) U-Net model; (f) SegNet model; (g) R2U-Net model; (h) proposed model
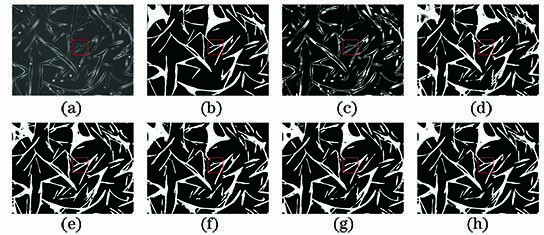
图 7. 第2组细胞分割结果。(a)原始图像;(b)真值图像;(c) Otsu模型;(d) FCN-8s模型;(e) U-Net模型;(f) SegNet模型;(g) R2U-Net模型;(h)所提模型
Fig. 7. Cell segmentation results of group 2. (a) Origin image; (b) ground truth; (c) Otsu model; (d) FCN-8s model; (e) U-Net model; (f) SegNet model; (g) R2U-Net model; (h) proposed model
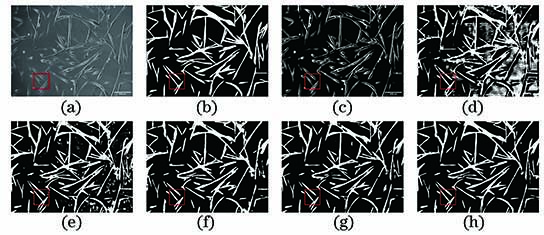
图 8. 第3组细胞分割结果。(a)原始图像;(b)真值图像;(c) Otsu模型;(d) FCN-8s模型;(e) U-Net模型;(f) SegNet模型;(g) R2U-Net模型;(h)所提模型
Fig. 8. Cell segmentation results of group 3. (a) Origin image; (b) ground truth; (c) Otsu model; (d) FCN-8s model; (e) U-Net model; (f) SegNet model; (g) R2U-Net model; (h) proposed model
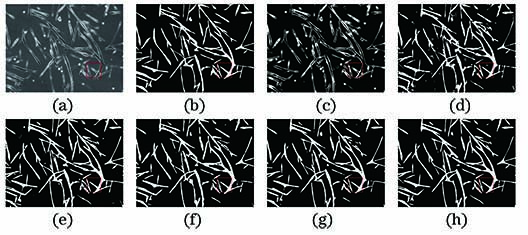
表 1不同分割模型的定量分析
Table1. Quantitative analysis of different segmentation models
|
表 2各模块对整体模型的影响
Table2. Influence of each module on whole model
|
张文秀, 朱振才, 张永合, 王新宇, 丁国鹏. 基于残差块和注意力机制的细胞图像分割方法[J]. 光学学报, 2020, 40(17): 1710001. Wenxiu Zhang, Zhencai Zhu, Yonghe Zhang, Xinyu Wang, Guopeng Ding. Cell Image Segmentation Method Based on Residual Block and Attention Mechanism[J]. Acta Optica Sinica, 2020, 40(17): 1710001.