激光与光电子学进展, 2020, 57 (6): 061007, 网络出版: 2020-03-06
融合扩张卷积网络与SLAM的无监督单目深度估计
下载: 1189次
Unsupervised Monocular Depth Estimation by Fusing Dilated Convolutional Network and SLAM
图 & 表
图 2. 标准卷积与扩张卷积滤波器对比图。(a)标准卷积滤波器;(b)扩张率为2的扩张卷积滤波器;(c)扩张率为3的扩张卷积滤波器
Fig. 2. Comparison of standard convolution and dilated convolution filters. (a) Standard convolution filter; (b) dilated convolution filter with dilation ratio of 2; (c) dilated convolution filter with dilation ratio of 3

图 3. 扩张卷积与标准卷积的可视化过程对比图。(a)标准卷积可视化过程;(b)扩张率为2的扩张卷积可视化过程;(c)扩张率为3的扩张卷积可视化过程
Fig. 3. Visualization process comparison of dilated convolution and standard convolution. (a) Visualization process of standard convolution; (b) visualization process of dilated convolution with dilation ratio of 2; (c) visualization process of dilated convolution with dilation ratio of 3
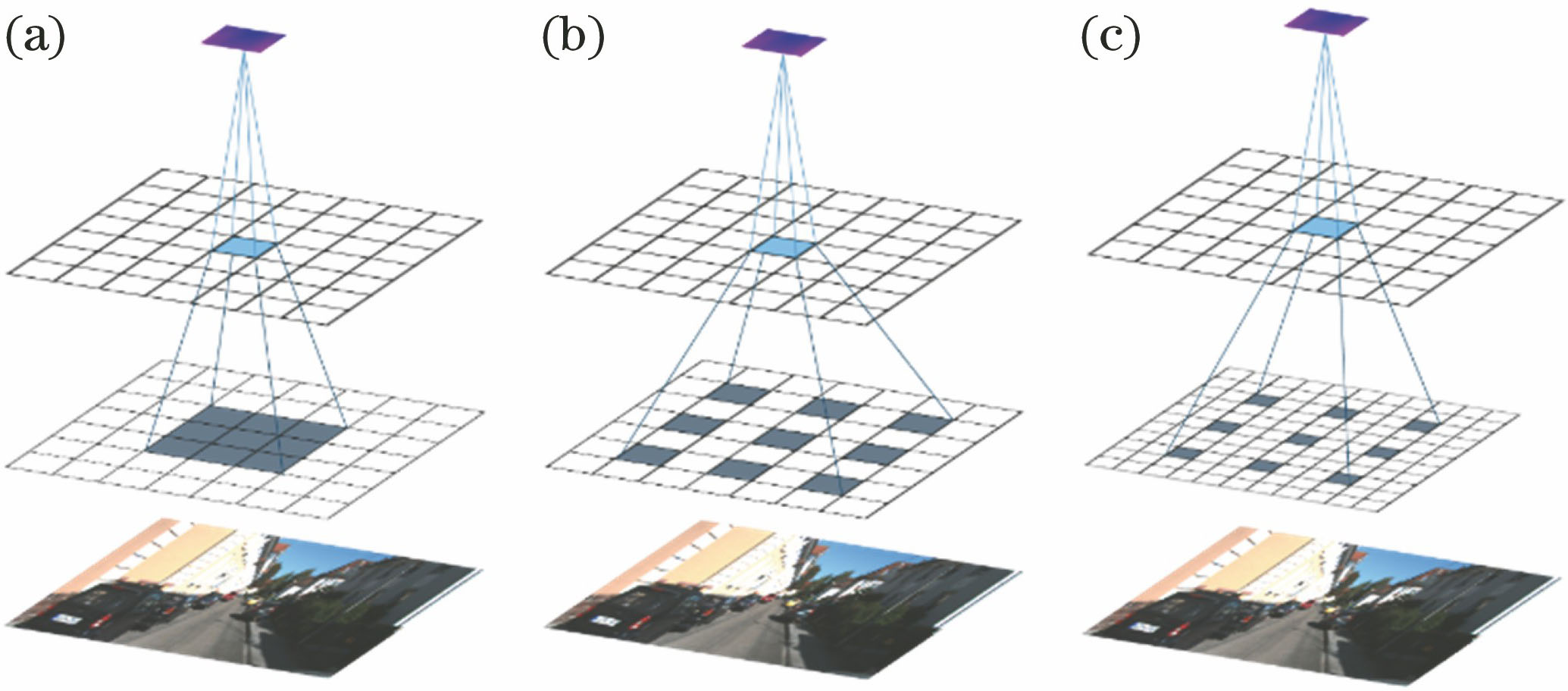
图 4. ORB-SLAM算法优化全局相机姿态总体流程
Fig. 4. Flow chart of optimizing global camera pose by ORB-SLAM algorithm
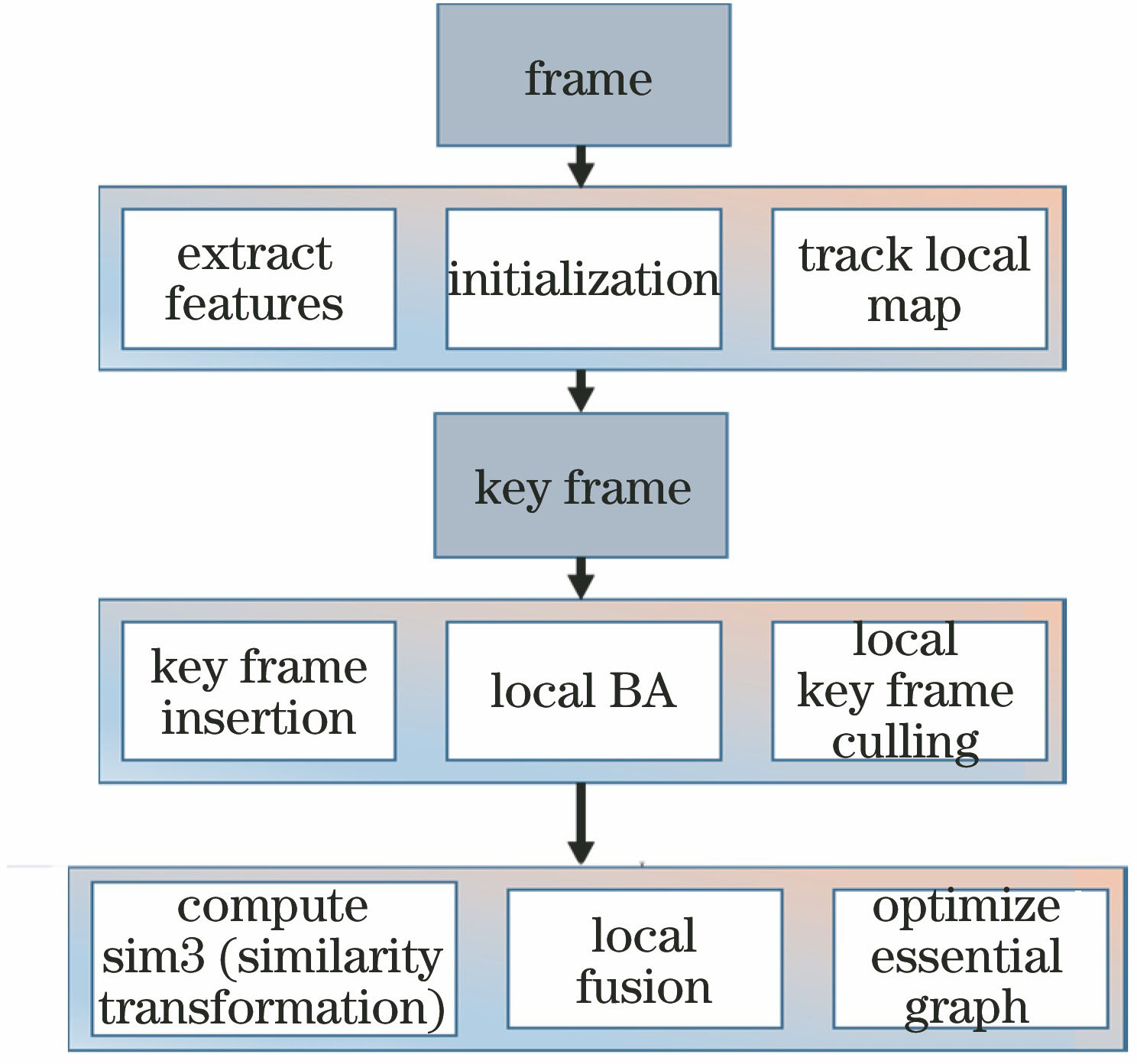
图 5. 三维空间点在图像平面上的投影过程
Fig. 5. Projection process of three-dimensional space points onto the image plane

图 6. 不同损失变化曲线。(a)重建损失;(b)平滑损失;(c)总体损失
Fig. 6. Curves for different losses. (a) Reconstruction loss; (b) smooth loss; (c) total loss
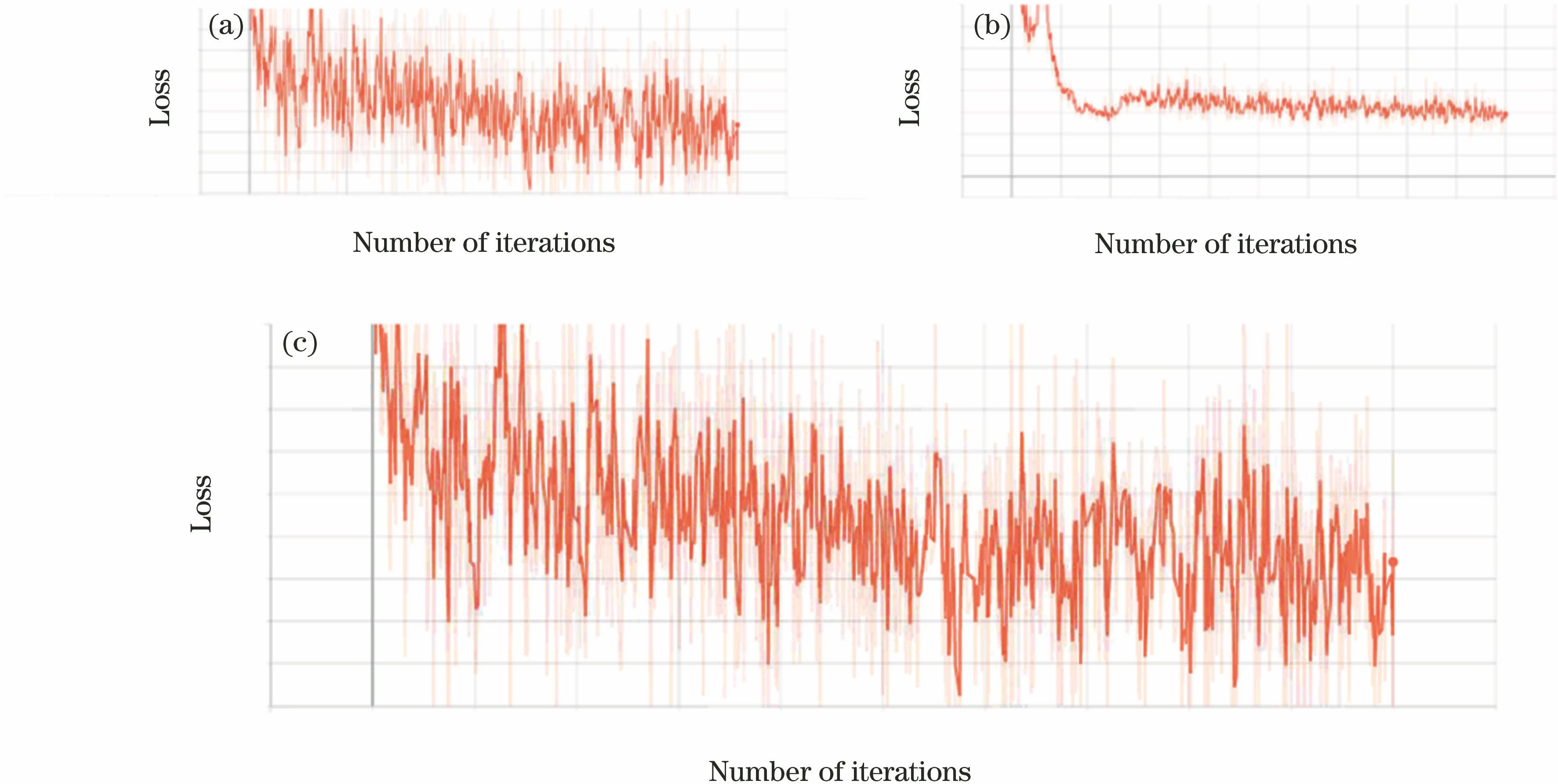
图 7. KITTI Odometry数据集中不同序列的相对相机姿态轨迹。(a) 00;(b) 01;(c) 09;(d) 02;(e) 03;(f) 10
Fig. 7. Camera pose trajectories for different sequences in the KITTI Odometry dataset. (a) 00; (b) 01; (c) 09; (d) 02; (e) 03; (f) 10
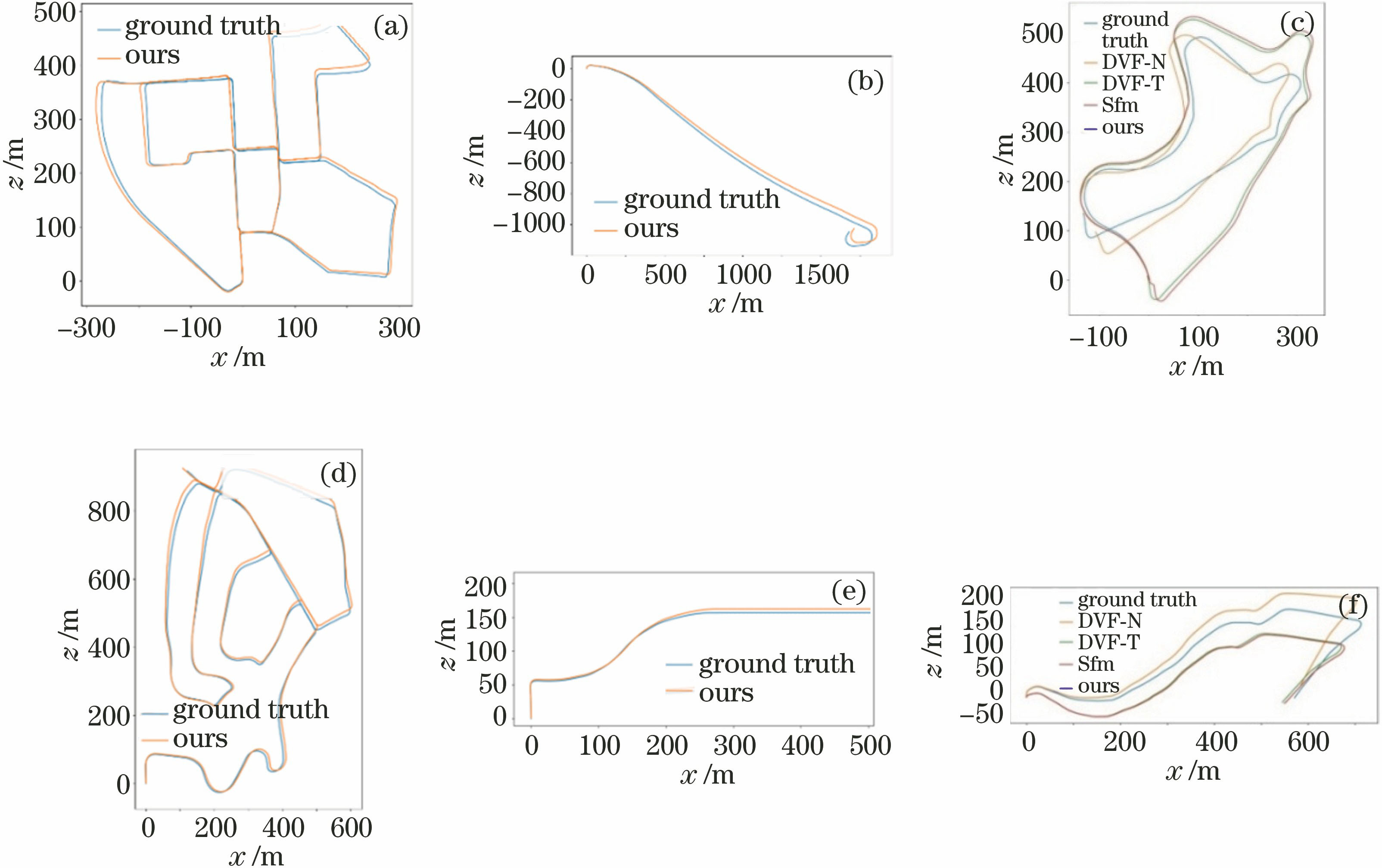
表 1KITTI Odometry数据集09和10序列的RMSE比较
Table1. RMSE comparison of 09 and 10 sequences in the KITTI Odometry dataset
|
表 2深度估计模型的TUM评估结果比较
Table2. Comparison of TUM evaluation results for depth estimation model
|
戴仁月, 方志军, 高永彬. 融合扩张卷积网络与SLAM的无监督单目深度估计[J]. 激光与光电子学进展, 2020, 57(6): 061007. Renyue Dai, Zhijun Fang, Yongbin Gao. Unsupervised Monocular Depth Estimation by Fusing Dilated Convolutional Network and SLAM[J]. Laser & Optoelectronics Progress, 2020, 57(6): 061007.