一种全息体视图虚实场景融合显示的图像编码方法
下载: 679次
Holographic stereogram technology is attracting increasing attention in the field of three-dimensional (3D) display. According to the technical principle of holographic stereograms, the displayed 3D scene can be a real-world scene or a 3D model rendered by a computer, which creates conditions for the display of fused real and virtual scenes. Relevant research in the field of augmented reality (AR) shows that adding virtual 3D elements to a real scene can provide an observer with a more intuitive and intense visual experience. For example, in the exhibits of cultural relics, to protect the real artifact, a 3D image and specific information about a cultural relic are presented, and some virtual introductory signs can be superimposed on the image. However, how to correctly express the spatial relationship between real and virtual scenes has become a new problem. We propose an image coding method to realize scene fusion with a correct occlusion relationship by processing the sampled images of real and virtual scenes. We hope that the proposed method will provide a reference for further research into holographic stereogram AR display.
To address the problem of image coding in the fusion display of real and virtual scenes, this paper first analyzes the generation of occlusion between scenes. The analysis shows that the occlusion relationship is determined by the depth of different object points and provides the basic process of a method based on the depth value. However, the method based on depth value judgment relies too much on accurate depth calculation results, and with existing methods, ensuring accuracy is difficult. Therefore, a method based on instance segmentation and depth assignment is proposed. We use Mask R-CNN to segment the sampled images of the real scene and assign each instance a pseudo depth value according to the depth range of the virtual scene to ensure the occlusion relationship. Finally, the method based on the depth value is used for image coding.
The encoded image can integrate the virtual scene into the foreground and background of the real scene with the correct occlusion relationship; however, the fusion result is greatly affected by the effect of instance segmentation (Fig. 8). We use the EPISM method for holographic printing and obtain the reconstructed images from five perspectives. Consequently, the virtual scene is successfully integrated into the real scene (Fig. 11). We describe the reconstructed image in detail. Due to the influence of instance segmentation, the edge of the scene is inconsistent with the original scene, which is consistent with the previous analysis (Fig. 12). Finally, we compare the depth information of the real scene and the virtual scene in the reconstructed image. The comparison results demonstrate that the depth information of the reconstructed image is consistent with the sampling setting (Fig. 13).
To achieve the fusion display effect of real and virtual scenes in a holographic stereogram, an image coding method based on instance segmentation and depth value determination is proposed. A theoretical analysis and the experimental results show that the proposed image coding method can effectively add some virtual 3D elements in the real scene to enhance the visual experience. The combination of virtual and real scenes fully considers the occlusion relationship, which is not a simple scene superposition. The key to fully considering the occlusion relationship is to assign and determine the pseudo depth after scene layering. There is still a significant gap between the virtual reality fusion method discussed in this paper and the latest work in the AR field. However, the proposed method provides a basic idea for further research into holographic stereogram AR displays. For example, by continuously improving the efficiency of instance segmentation, the scene is more accurately layered. The accurate depth calculation method is studied, and the accurate depth value is used as the basic processing data, which can better show the effect of virtual real scene fusion display in a holographic stereogram. This paper only considers the simple and small scenes used in an experiment and tends to assume ideal conditions. In theory, the proposed method is suitable for scenes with a clear occlusion relationship between different instances in the sampled image; however, it cannot effectively deal with the occlusion between the same instances. Therefore, further analysis of complex scenes is required.
1 引言
全息体视图打印技术[1]是光学全息术[2]与双目视差原理[3]的有机结合,它利用离散的二维图像近似重构原3D光场,为全息3D显示开辟了新路径,并且已经广泛应用于医疗、商业、**等领域[4-6]。1967年,Pole[7]利用复眼透镜阵列进行采样,将图像直接写入全息单元中,制作出第一幅全息体视图。随后,单步法[8]、两步法[9]相继出现,通过优化全息体视图的记录方式,提升了再现像的质量。20世纪末,随着计算机技术的迅速发展,针对全息体视图的研究从硬件设置转变为软件处理。无穷远相机法[10]、Lippmann法[11]、数字直写(DWDH)法[12]均能实现3D场景的快速写入与高质量无畸变显示。2017年,本课题组提出一种基于有效视角图像切片嵌合(EPISM)的单步全息体视图打印方法[13-16],该方法的处理速度更快、显示质量更高,适用于3D光场的快速编码写入及高分辨率再现。
全息体视图的数据源既可以是真实场景,又可以是计算机渲染的3D模型,这为虚实场景的融合显示提供了契机,而虚实场景的融合显示频繁出现在增强现实(AR)领域。相关研究表明,在真实场景中附加虚拟3D元素,可以带给观察者更直观、更震撼的视觉感受,因此结合全息理论研究虚实场景的融合显示具有一定的理论与现实意义。头戴式全息显示器是全息术与AR有机结合后的一种特殊应用。传统的计算机生成全息图(CGH)在全息显示器的工作中计算量大,数据冗余严重。Chen等[17]通过分析人眼瞳孔对有效波前传输的局限性,提出一种适用于头戴式全息显示器的CGH的低冗余、快速计算方法,将计算量降低至传统方法的1.5%,并且再现像质量良好。为了解决传统全息Maxwell显示器景深与图像质量之间固有的折中问题,Wang等[18]提出了一种基于球面波前与平面波前相结合的大自由度、高图像质量的成像方法。利用球面波和平面波两种全息Maxwell显示器实现了AR显示器自由度范围的互补。近年来,近眼全息与全息AR显示密切相关[19-20]。2019年,Yang等[21-22]提出一种用于近眼虚拟现实(VR)和AR 3D显示的具有多重投影图像的快速计算生成全息方法。通过将投影图像与相应的点扩展函数进行卷积,设计并开发了近眼AR全息3D显示系统。计算速度显著提高(是传统方法的38倍),可以生成无斑点噪声的高质量VR和AR 3D图像。Li等[23]提出一种实现3D光学透明AR的全息显示系统,他们利用体全息的角度复用性能,在光学全息元件上写入同时具有反射和透射功能的光栅。利用该元件,可以在全息显示的同时看到无干扰的真实场景。然而,在全息体视图领域,对3D场景虚实融合AR显示的研究相对较少。本课题组提出一种像素替换方法,将虚拟场景信息直接叠加到真实场景上[24],但该方法不能实现具有复杂遮挡关系的场景融合。
实际上,全息体视图的3D显示来自所有全息单元联合显示。因此,为了实现全息体视图的虚实融合显示,必须对图像进行有效编码。本文提出一种基于实例分割与深度值判定的图像编码方法。分析表明:场景存在遮挡关系的本质在于,同视线下多物点的深度值不同,深度小的物点会遮挡住深度大的物点。据此,提出一种基于深度值判定的编码方法。但是,考虑到现有方法求解的深度值不精确的问题,进一步提出一种利用实例分割算法对场景分层,为各层赋伪深度值,再进行深度值判定的方法,并介绍了图像编码方法的相关原理和实现细节。编码后的图像采用EPISM方法进行处理。光学实验结果表明,所提出的图像编码方法可以有效地实现全息体视图的虚实融合立体显示。
2 场景遮挡分析
全息体视图虚实场景的融合立体显示,不能只是真实3D场景与虚拟3D模型之间简单的叠加,二者应具有合理的,甚至复杂的遮挡关系,才能使观察者具有良好、真实的视觉体验。为便于分析,不妨提出以下3个假设:1)任一空间物点应具有唯一归属的性质,即空间中任何一个物点,要么属于真实3D场景,要么属于虚拟3D模型,这一假设符合人眼的观察规律;2)任一像素点对应的场景空间区域具有平面特性,即在采样时,任一像素点对应唯一的深度值;3)真实3D场景与虚拟3D模型都不具有透明属性(后续工作可以附加透明属性)。
基于上述假设,可以进一步分析虚、实场景之间的遮挡关系:两者在某一视角下呈现一定的遮挡关系,必然是两者物点的深度不一致造成的,深度小的物点将会遮挡住深度大的物点,并在图像对应像素点处留下强度信息。如
综上,虚、实场景有相互遮挡时,可以依据深度值进行图像编码,其原则是:预设虚、实场景的空间位姿后分别进行采样,计算图像的深度信息,并遍历所有像素点进行深度值比较,取舍相应场景图像的强度信息,直至完成所有采样图像的编码。
深度信息的精确计算成了新的问题。通常采用单目深度估计[25-27]、立体匹配[28-30]及基于深度学习的深度估计[31-33]等方法求解图像的深度值,但是这些方法很难得到精确的深度值:当虚、实场景距离较远、深度差值较大时,深度估计带来的误差尚可接受;当深度差值较小时,深度估计误差会造成错误取舍,使原本的遮挡关系完全相反。当然,也可用深度信息采集设备进行采样,如Kinect传感器[34-35]等,但这种硬件设备一般都存在极限距离。因此,若要实现虚实场景融合显示的图像编码,必须另辟蹊径。
事实上,上述编码方法的本质是通过深度值判定遮挡关系,而深度值的正确与否并不重要,只要满足要求即可。另外,当某一视角的编码图像同时保留虚、实场景的部分信息时,遮挡必定存在。因此,无需对采样图像进行精确的深度值计算,只要能判断场景的正确遮挡关系即可。可以采用实例分割方法对场景的前景与背景进行分层,依据遮挡关系为各层赋伪深度值,再利用深度值判定方法进行图像编码。在技术难度上,图像的实例分割要比精确的深度计算更加简单。参考
3 图像编码实现
基于第2节的讨论,可以将所提到的图像编码方法概括为:对真实场景采样,并对得到的采样图像进行实例分割;按照真实采样的参数设定进行虚拟场景采样,并渲染深度图;利用虚拟场景深度图计算真实场景分层后各层相应的深度值;利用深度值判定方法完成图像编码。下面根据此流程进行编码实验。
采用“士兵”模型作为真实场景的前景,山地图像作为背景。在3D Studio Max软件中渲染“BAM!”模型并进行采样。搭建了单相机时序采样系统:将单个MER2-502-79U3C型CMOS数字相机固定安装在Zolix KSA300型电控位移平台上,位移平台内的步进电机受到Zolix MC600运动控制器的驱动,可在水平和垂直两个自由度内以任意间隔移位采样,如

图 4. 真实场景与虚拟场景的采样。(a) 单相机时序采样系统;(b)真实场景“士兵”模型图像;(c)虚拟场景“BAM!”模型图像;(d)渲染得到的深度图
Fig. 4. Sampling of real and virtual scenes. (a) Single camera timing sampling system;(b) real scene“soldier”model image; (c) virtual scene“BAM!”model image and (d) its depth map
虚拟场景采样与真实场景采样设置保持一致,同时渲染其“z深度”通道,得到“BAM!”模型图像的深度图。其中zmin=10 cm、zmax=30 cm,“BAM!”模型距离相机14.3 cm。
综合比较了多种实例分割方法[36-38],决定采用效果较好的Mask R-CNN实例分割网络架构[39-40]进行分层处理。Mask R-CNN由何凯明提出,可以实现目标检测、语义分割、实例分割,其在Faster R-CNN[41-42]的基础上,增加了一个完全卷积网络(FCN),在目标检测的基础上实现了语义分割,进而实现了实例分割,
利用Windows操作系统及计算能力较强的NVIDIA TITAN Xp型号显卡,在Tensorflow深度学习开源框架上基于Keras模型库搭建了Mask R-CNN网络架构。利用labelme标注工具制作了带有掩码标签的400幅“士兵”模型图像数据集(实际需要编码的图像为19321幅),其中350幅用于训练,50幅用于验证,如
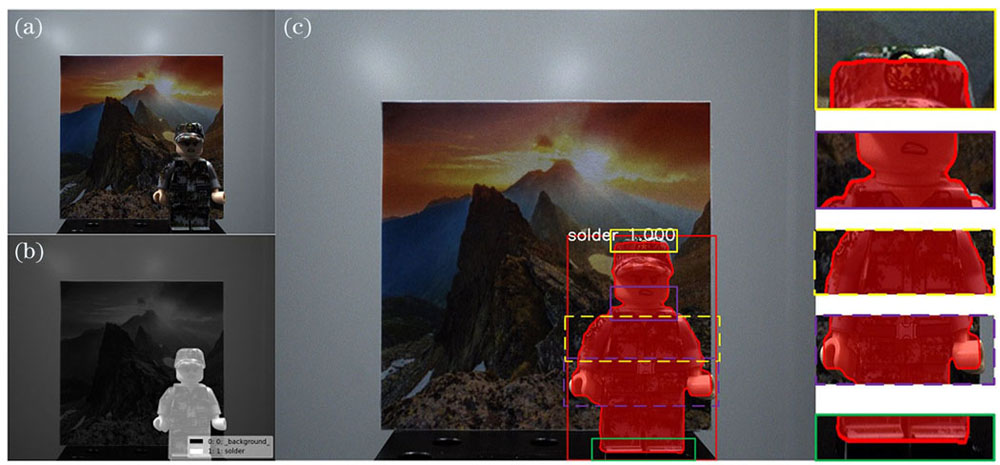
图 6. 自制数据集的测试效果。(a)“士兵”模型图像;(b)带有掩码的标签图像;(c)实例分割结果
Fig. 6. Test effects of self-made data sets. (a) Image of soldier model; (b) corresponding label image with mask; (c) instance segmentation result
利用训练好的模型对所有要编码的士兵模型图像进行预测,得到相应的带有前景掩码的图像。由于采用的场景仅有一个实例,且虚拟场景深度图的灰度范围为0~255,因此利用数字图像处理方法对这些图像进行黑白二值化处理,得到具有伪深度值的深度图(前景为255,背景为0),以保证下一步进行深度值判定时结果的正确性,如
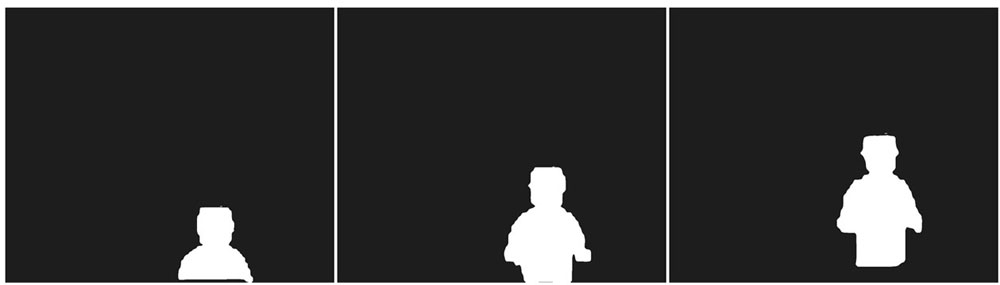
图 7. 3张黑白二值化处理后的伪深度图像
Fig. 7. Three pseudo depth images after black-and-white binary processing
利用基于深度值判定的方法完成图像编码。
编码后的视角图像不可直接用于全息打印,需进行进一步处理,本实验采用本课题组提出的基于有效视角图像分割与重组(EPISM)的方法进行图像处理。该方法参考传统两步法[9],根据光线追迹原理与光路的可逆性,模拟人眼的锥形放射状观察效果,对原始视角图像进行分割重组处理,得到最终需要曝光的图像。EPISM方法处理速度快,利用其得到的再现像具有分辨率高、凸出显示和记录介质的优点。EPISM方法的详细内容参考文献[12]。
4 实验与结果
采用EPISM全息体视图打印方法进行图像预处理,得到用于全息打印的曝光图像。实验光路设置如
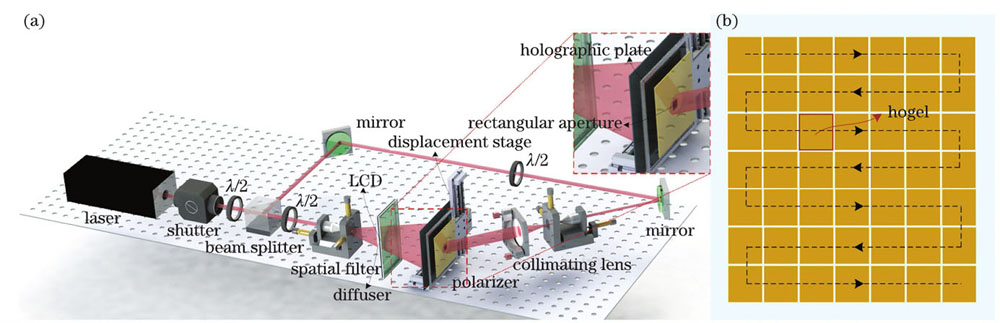
图 9. 全息打印示意图。(a)打印光路示意图;(b)全息单元打印顺序
Fig. 9. Schematic of holographic printing. (a) Schematic of printing optical path; (b) printing sequence of hogels
打印结束后,全息干板经显影、漂白等处理,可在红光条件下再现3D像。如
不同视角下拍摄的图像如
比较虚拟场景和真实场景的深度信息,结果如
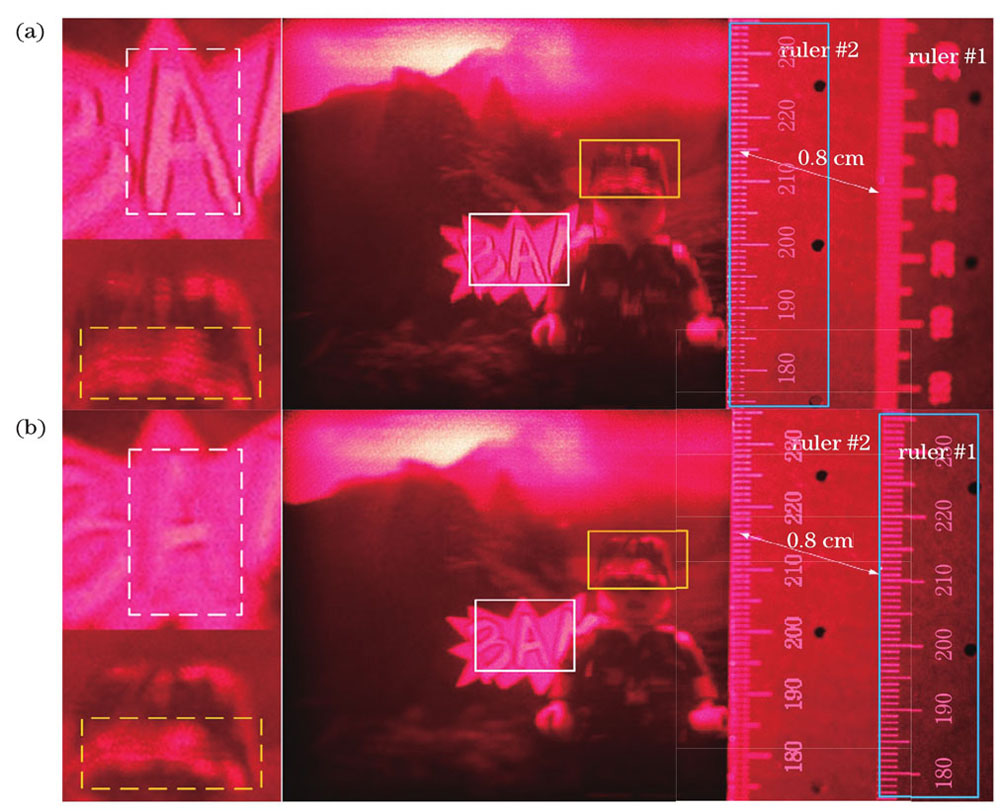
图 13. 相机聚焦在不同位置时虚拟场景与真实场景的深度比较。(a)标尺#2;(b)标尺#1
Fig. 13. Depth comparison between virtual scene and real scene when the camera focus on different positions. (a) Ruler #2; (b) ruler #1
5 结论
为了实现全息体视图虚实场景的融合显示效果,提出一种基于实例分割与深度值判定的图像编码方法。理论分析与实验结果表明:在全息体视图领域,所提的图像编码方法可以有效地在真实场景内部附加一些虚拟3D元素以增强视觉感受。虚实场景之间的结合不是简单的场景叠加,而是充分考虑了遮挡关系,实现这一点的关键在于场景分层后的伪深度赋值与判定。所提出的虚实融合方法与AR领域的最新工作需求仍有很大差距,但提出的方法为全息体视图AR显示这一研究方向提供了新的思路,并可进一步深入挖掘,比如:通过不断提升实例分割效能对场景进行更为精确的分层;研究精确的深度计算方法,用精确的深度值作为基本处理数据,更能展现全息体视图虚实场景融合显示的效果。需要指出的是,本文仅讨论了简单、小型的场景,偏向于理想条件。理论上,所提方法适用于采样图像中具有明确的不同实例之间存在遮挡关系的场景,但不能处理同一实例之间的遮挡,因此复杂场景的相关工作仍需深入研究。
[1] 樊帆, 闫兴鹏, 李沛, 等. 针对一般情形的全息单元有效视角图像切片嵌合法全息体视图打印[J]. 中国激光, 2019, 46(12): 1209001.
[2] Gabor D. A new microscopic principle[J]. Nature, 1948, 161(4098): 777.
[3] Qian N. Binocular disparity and the perception of depth[J]. Neuron, 1997, 18(3): 359-368.
[4] Liu P, Sun X D, Zhao Y, et al. Ultrafast volume holographic recording with exposure reciprocity matching for TI/PMMAs application[J]. Optics Express, 2019, 27(14): 19583-19595.
[6] Bjelkhagen H I, Brotherton-Ratcliffe D. Ultrarealistic imaging: the future of display holography[J]. Optical Engineering, 2014, 53(11): 112310.
[7] Pole R V. 3-D imagery and holograms of objects illuminated in white light[J]. Applied Physics Letters, 1967, 10(1): 20-22.
[8] Debitetto D J. Holographic panoramic stereograms synthesized from white light recordings[J]. Applied Optics, 1969, 8(8): 1740-1741.
[9] King M C, Noll A M, Berry D H. A new approach to computer-generated holography[J]. Applied Optics, 1970, 9(2): 471-475.
[10] Halle M W, Benton S A, Klug M A, et al. Ultragram: a generalized holographic stereogram[J]. Proceedings of SPIE, 1991, 1461: 142-155.
[11] Yamaguchi M, Ohyama N, Honda T. Holographic three-dimensional printer: new method[J]. Applied Optics, 1992, 31(2): 217-222.
[12] Myridis N E. Ultra-realistic imaging: advanced techniques in analogue and digital colour holography, by Hans Bjelkhagen and David Brotherton-Ratcliffe[J]. Contemporary Physics, 2014, 55(3): 247-248.
[13] Su J, Yuan Q, Huang Y, et al. Method of single-step full parallax synthetic holographic stereogram printing based on effective perspective images' segmentation and mosaicking[J]. Optics Express, 2017, 25(19): 23523-23544.
[14] Fan F, Jiang X Y, Yan X P, et al. Holographic element-based effective perspective image segmentation and mosaicking holographic stereogram printing[J]. Applied Sciences, 2019, 9(5): 920.
[15] Su J, Yan X, Jiang X, et al. Characteristic and optimization of the effective perspective images' segmentation and mosaicking (EPISM) based holographic stereogram: an optical transfer function approach[J]. Scientific Reports, 2018, 8(1): 4488.
[16] Yan X, Zhang T, Wang C, et al. Analysis on the reconstruction error of EPISM based full-parallax holographic stereogram and its improvement with multiple reference plane[J]. Optics Express, 2019, 27(22): 32508-32522.
[18] Wang Z, Zhang X, Lv G, et al. Hybrid holographic Maxwellian near-eye display based on spherical wave and plane wave reconstruction for augmented reality display[J]. Optics Express, 2021, 29(4): 4927-4935.
[19] Chang C L, Bang K, Wetzstein G, et al. Toward the next-generation VR/AR optics: a review of holographic near-eye displays from a human-centric perspective[J]. Optica, 2020, 7(11): 1563-1578.
[20] He Z H, Sui X M, Jin G F, et al. Progress in virtual reality and augmented reality based on holographic display[J]. Applied Optics, 2018, 58(5): A74-A81.
[21] Yang X, Zhang H B, Wang Q H. A fast computer-generated holographic method for VR and AR near-eye 3D display[J]. Applied Sciences, 2019, 9(19): 4164.
[22] Yang X, Lin S F, Wang D, et al. Holographic AR display based on free-form lens combiner and LED illumination[J]. Proceedings of SPIE, 2019, 1120: 210-215.
[23] Li G, Lee D, Jeong Y, et al. Holographic display for see-through augmented reality using mirror-lens holographic optical element[J]. Optics Letters, 2016, 41(11): 2486-2489.
[24] 张腾, 闫兴鹏, 王晨卿, 等. 多参考平面的EPISM全息体视图[J]. 中国激光, 2020, 47(9): 0909001.
[25] RoyA, TodorovicS. Monocular depth estimation using neural regression forest[C]//2016 IEEE Conference on Computer Vision and Pattern Recognition (CVPR), June 27-30, 2016, Las Vegas, NV, USA. New York: IEEE Press, 2016: 5506-5514.
[27] Yan H, Zhang S L, Zhang Y, et al. Monocular depth estimation with guidance of surface normal map[J]. Neurocomputing, 2018, 280: 86-100.
[28] Isard M, Blake A. CONDENSATION: conditional density propagation for visual tracking[J]. International Journal of Computer Vision, 1998, 29(1): 5-28.
[29] Sekine Y. Effects of country-of-origin information on product evaluation: an information processing perspective[J]. Neuroscience & Biobehavioral Reviews, 2017, 72(6): 232.
[30] ChangJ R, ChenY S. Pyramid stereo matching network[C]//2018 IEEE/CVF Conference on Computer Vision and Pattern Recognition, June 18-23, 2018, Salt Lake City, UT, USA. New York: IEEE Press, 2018: 5410-5418.
[31] Liu F Y, Shen C H, Lin G S, et al. Learning depth from single monocular images using deep convolutional neural fields[J]. IEEE Transactions on Pattern Analysis and Machine Intelligence, 2016, 38(10): 2024-2039.
[32] SchwarzM, SchulzH, BehnkeS. RGB-D object recognition and pose estimation based on pre-trained convolutional neural network features[C]//2015 IEEE International Conference on Robotics and Automation (ICRA), May 26-30, 2015, Seattle, WA, USA. New York: IEEE Press, 2015: 1329-1335.
[33] ZhanH Y, GargR, WeerasekeraC S, et al. Unsupervised learning of monocular depth estimation and visual odometry with deep feature reconstruction[C]//2018 IEEE/CVF Conference on Computer Vision and Pattern Recognition, June 18-23, 2018, Salt Lake City, UT, USA. New York: IEEE Press, 2018: 340-349.
[34] Khoshelham K. Accuracy analysis of Kinect depth data[J]. The International Archives of the Photogrammetry, Remote Sensing and Spatial Information Sciences, 2012, XXXVIII-5/W12: 133-138.
[35] SmisekJ, JancosekM, PajdlaT. 3D with Kinect[M]. New York: IEEE Press, 2011: 1154-1160.
[36] Hyyppa J, Kelle O, Lehikoinen M, et al. A segmentation-based method to retrieve stem volume estimates from 3-D tree height models produced by laser scanners[J]. IEEE Transactions on Geoscience and Remote Sensing, 2001, 39(5): 969-975.
[37] DaiJ F, HeK M, SunJ. Instance-aware semantic segmentation via multi-task network cascades[C]//2016 IEEE Conference on Computer Vision and Pattern Recognition (CVPR), June 27-30, 2016, Las Vegas, NV, USA. New York: IEEE Press, 2016: 3150-3158.
[38] GuptaS, GirshickR, ArbeláezP, et al. Learning rich features from RGB-D images for object detection and segmentation[M]//Fleet D, Pajdla T, Schiele B, et al. Computer vision-ECCV 2014. Lecture notes in computer science. Cham: Springer, 2014, 8695: 345-360.
[39] HeK M, GkioxariG, DollarP, et al. Mask R-CNN[C]//2017 IEEE International Conference on Computer Vision (ICCV), October 22-29, 2017. Venice. New York: IEEE Press, 2017: 2980-2988.
[40] Xu Y Y, Li D W, Xie Q, et al. Automatic defect detection and segmentation of tunnel surface using modified Mask R-CNN[J]. Measurement, 2021, 178: 109316.
[41] GirshickR. Fast R-CNN[C]//2015 IEEE International Conference on Computer Vision (ICCV), December 7-13, 2015. Santiago, Chile. New York: IEEE Press, 2015: 1440-1448.
[42] Ren S Q, He K M, Girshick R, et al. Faster R-CNN: towards real-time object detection with region proposal networks[J]. IEEE Transactions on Pattern Analysis and Machine Intelligence, 2017, 39(6): 1137-1149.
[43] LinT Y, MaireM, BelongieS, et al. Microsoft COCO: common objects in context[M]//Fleet D, Pajdla T, Schiele B, et al. Computer vision-ECCV 2014. Lecture notes in computer science. Cham: Springer, 2014, 8693: 740-755.
刘云鹏, 汪熙, 刘新蕾, 荆涛, 蒋晓瑜, 闫兴鹏. 一种全息体视图虚实场景融合显示的图像编码方法[J]. 中国激光, 2022, 49(4): 0409002. Yunpeng Liu, Xi Wang, Xinlei Liu, Tao Jing, Xiaoyu Jiang, Xingpeng Yan. An Image Encoding Method for Fusion Display of Virtual and Real Scenes in Holographic Stereogram[J]. Chinese Journal of Lasers, 2022, 49(4): 0409002.