激光与光电子学进展, 2018, 55 (5): 051006, 网络出版: 2018-09-11
二维和三维卷积神经网络相结合的CT图像肺结节检测方法
下载: 2881次
Detection of Pulmonary Nodules CT Images Combined with Two-Dimensional and Three-Dimensional Convolution Neural Networks
图 & 表
图 2. CT切片序列原图与U-net预测图。(a)原图;(b)预测图
Fig. 2. CT slice original images and U-net prediction images. (a) Original images; (b) prediction images
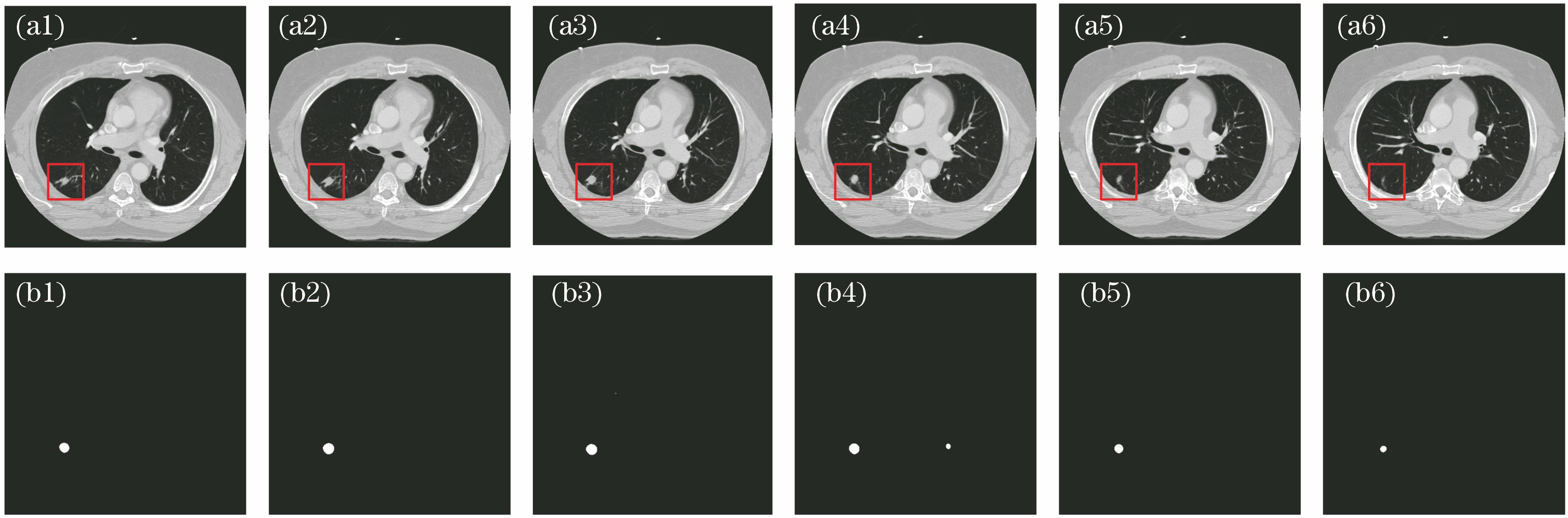
图 3. 3D卷积神经网络假阳性去除系统网络的结构
Fig. 3. Structure of 3D convolution neural network false positive removal system network

图 5. 数据库中不同尺寸结节的图像。(a)小结节;(b)中等结节;(c)大结节
Fig. 5. Images of nodules with different sizes in the database. (a) Small nodules; (b) middle nodules; (c) big nodules
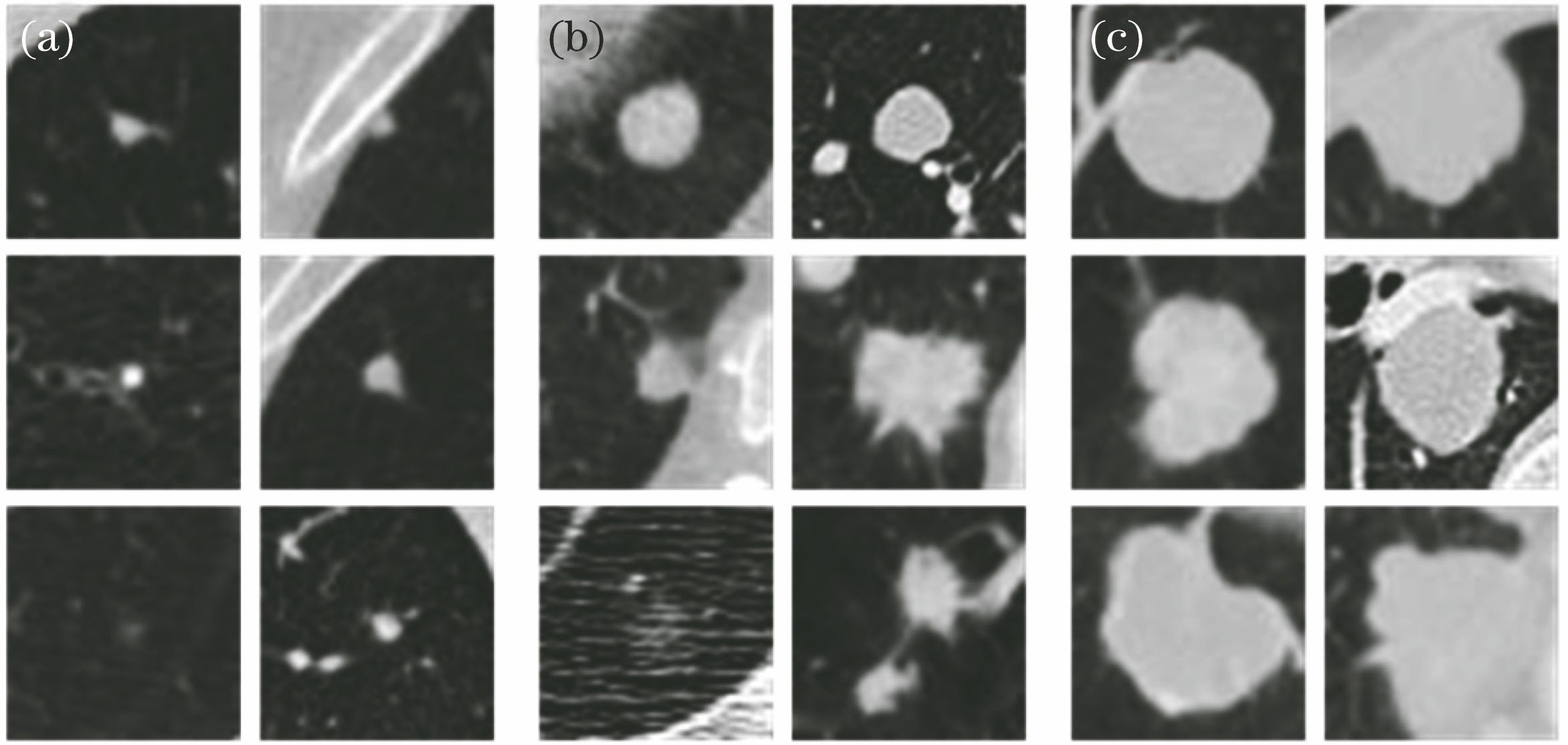
图 6. 3D卷积神经网络模型的训练误差和测试准确率曲线
Fig. 6. Training error and test accuracy curves of 3D convolution neural network model
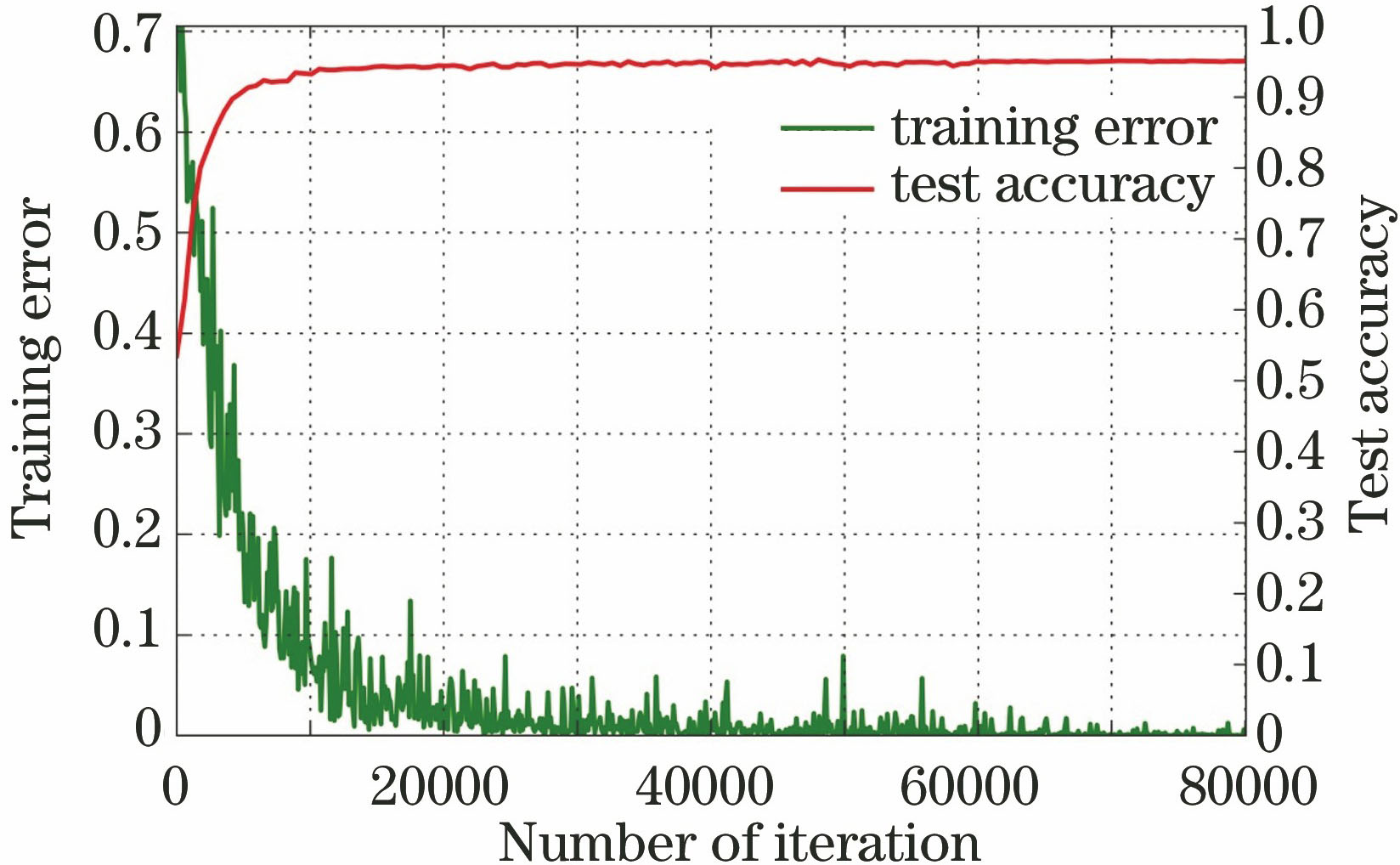
图 8. 输入图像块的尺度对实验结果的影响
Fig. 8. Effect of the dimensions of the input image block on the experimental results
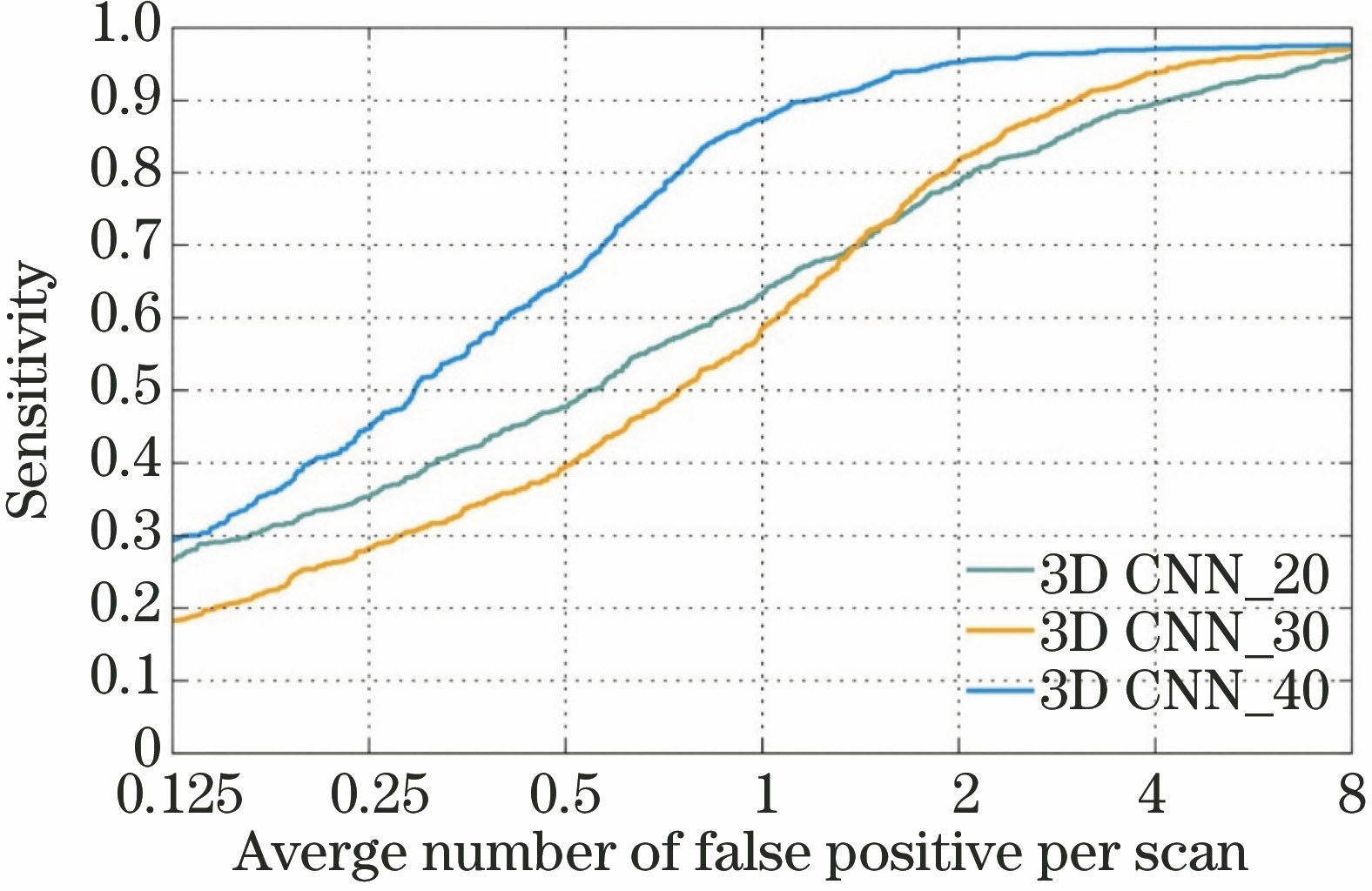
图 9. 网络模型的选择对实验结果的影响
Fig. 9. Effect of the selection of network model on experimental results
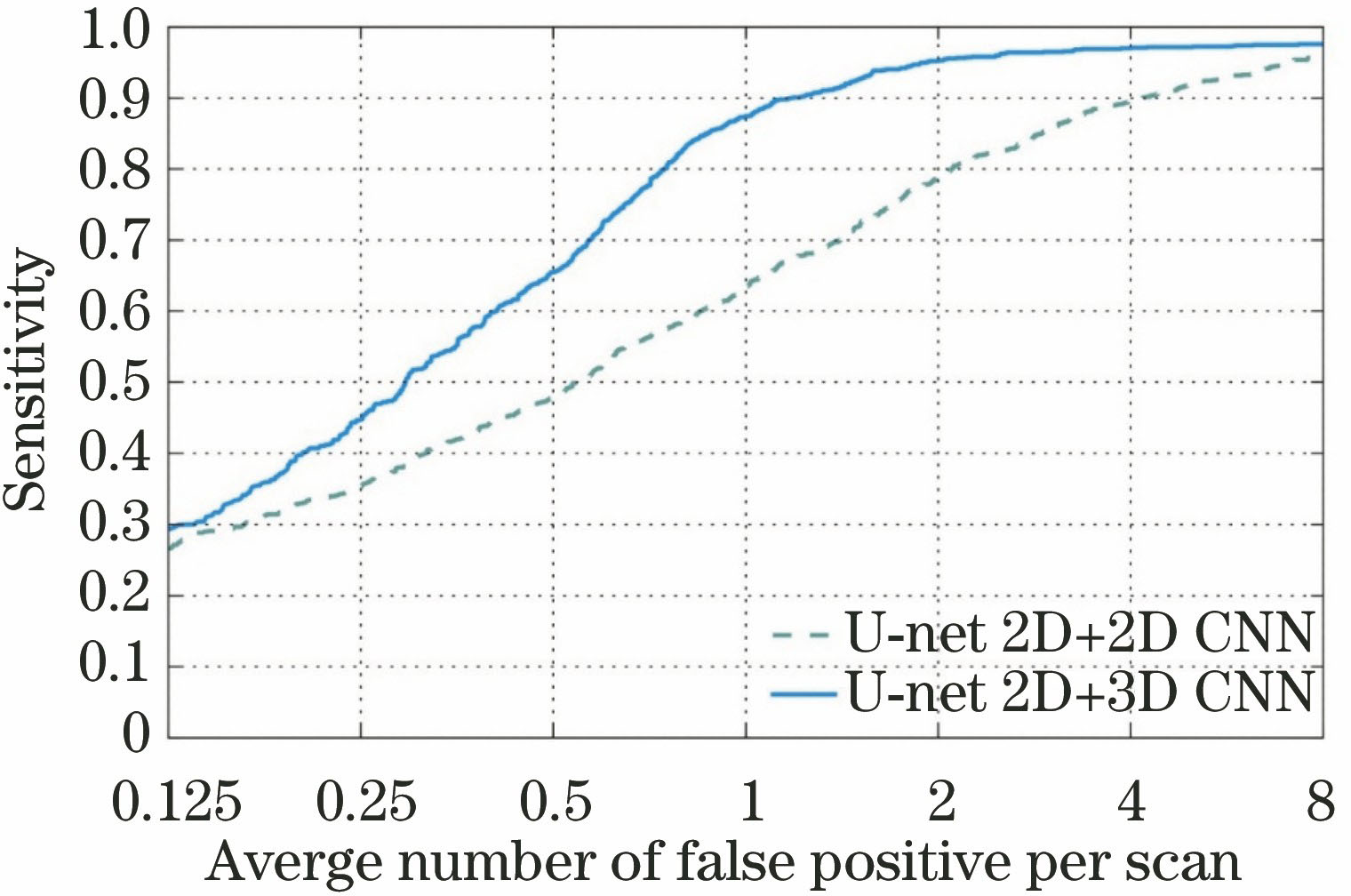
表 1LIDC-IDRI数据库中肺结节的检测算法比较
Table1. Comparison of detection algorithms of pulmonary nodules in LIDC-IDRI database
|
苗光, 李朝锋. 二维和三维卷积神经网络相结合的CT图像肺结节检测方法[J]. 激光与光电子学进展, 2018, 55(5): 051006. Guang Miao, Chaofeng Li. Detection of Pulmonary Nodules CT Images Combined with Two-Dimensional and Three-Dimensional Convolution Neural Networks[J]. Laser & Optoelectronics Progress, 2018, 55(5): 051006.