基于数字全息的云中冰晶微物理参数观测方法
As an important component of the cloud droplet spectrum, ice crystals exert important effects on global radiation budget balance, global climate change, hydrological cycle, and weather modification. Due to the limitation of observation means, the understanding about the microphysical characteristics of ice crystal particles is not perfect till now. At present, it is difficult to identify the mixed phase of 2-100 μm ice crystals from droplets, and there is a bottleneck to provide microphysical parameters of ice crystals. The lack of sufficient ice crystal detection data can cause large differences in the mean value of ice water paths in different models, especially in mixed-phase clouds. Therefore, we study the microphysical parameters of ice crystals.
For these two problems, based on the digital holography theory, we propose to employ the global digital image fusion method, the local Tenengrad variance method, and the rotating caliper method for identifying mixed phase states of droplets and ice crystals in the cloud by combining the roundness concept of solid and liquid particles. Combined with optical image recognition technology, we obtain the area, perimeter, convex hull, and minimum enclosing rectangle data of ice crystals. Finally, the microphysical parameters of ice crystals are acquired by adopting the above data. The microphysical parameters of plate, dendritic and hexagonal ice crystals are obtained by observation experiments in low-temperature cloud chambers.
Some obvious conclusions can be obtained by adopting the proposed method. 1) By leveraging the rotating caliper method and the specific geometric parameter roundness F, the mixed phase identification of droplets and ice crystals in clouds is realized. Under the specific roundness threshold, the recognition rate of droplets and ice crystals is greater than 93% (Fig. 8). 2) Combined with optical image recognition technology, morphological data (the area, perimeter, convex hull, and minimum enclosing rectangle data) of ice crystal particles are obtained (Table 1). 3) The microphysical parameters of ice crystals are acquired by morphological data of ice crystals (Table 2). 4) When the digital hologram of ice crystal particles is obtained with the frequency of 30 frame/s, the three-dimensional kinetic velocity of ice crystal particles can also be acquired by this method (Fig. 11).
An ice crystal detection method based on a pulse-modulated laser, high-resolution optical system, and coaxial digital holography (DH) is presented. The local Tenengrad variance method, the rotating caliper method, and the specific geometric parameter roundness F are adopted for phase state identification of particles. To verify the validity of the detection method and identification algorithm, we observe the mixed particles of droplets and ice crystals in the cloud chamber. Additionally, the three-dimensional motion velocity and trajectory of ice crystals can be obtained from the sampling interval time and the three-dimensional coordinates and equivalent diameters of the center of mass at different time. This method solves the bottleneck problem that the existing observation technology cannot identify the phase states and obtain the microphysical parameters of ice crystals. Meanwhile, the method is of significance to improve the accuracy of numerical weather prediction and weather modification operation.
1 引言
冰晶是云滴谱中重要的组成部分之一,通常以独立固相存在于卷云、高积云、高层云及雨层云上部,也会与液滴形成混合相态存在于层云中。其具有形状种类繁多、覆盖地球范围广的特点。对全球辐射收支平衡、全球气候变化、水文循环、人工影响天气作业等具有重要的影响[1-3]。由于观测手段的限制,目前对冰晶粒子形状、大小、数浓度、时空演化等微物理特征的认识还不够完善,由此给研究人工增雨条件、人工催化技术、雷达定量测量降水和效果检验等带来诸多不便。缺少足够的冰晶探测数据也会造成不同模型中冰水路径平均值相差较大,特别是在混合相态的云中。近年来,国内外已发展了多种用于冰晶观测的遥感探测与定点观测技术[4-8]。在遥感探测中,利用偏振激光主动遥感冰晶粒子,利用退偏比和功率谱数据反演获得各项参数,然而反演过程需要对云滴谱和粒子特性进行假设,测量精度需要验证,也无法具体获得冰晶的真实粒子形状、大小、数浓度、时空演化等微物理特征;在定点观测中,糖盘法和玻片法是将落入糖盘或玻片的冰晶进行计数,再利用显微镜进行形貌观测,然而其观测面积太小,不适合检测低浓度冰晶,且冰晶会产生形变[9]。冰晶声学计数器法虽然能自动计数,但对大水滴也有响应,因而误差较大;散射法只能测出冰晶的浓度和尺度,但是无法获取冰晶形状。并且,现有观测手段没有考虑液滴和冰晶形成的混合相态。
采用电荷耦合器件(CCD)或互补金属氧化物半导体(CMOS)器件代替干板记录数字全息图,可实现数字全息技术(DH)的快速、实时、非破坏性的全场光学测量,该技术已经广泛应用于细胞、流场、运动颗粒等动态场的观测[10-15]。为了解决冰晶观测的瓶颈问题,本文在云微物理参数同步测量理论的基础上做了深入研究,进行算法创新及全息光路改进。提出了一种算法来实现混合相态识别并提取冰晶形貌,进而可以获取冰晶的微物理参数。此外,光路中引入脉冲激光器和显微系统的同时对CMOS相机重新选型进行系统改进。本文采用DH技术,利用全域数字图像融合方法、局部亮度梯度方差法和旋转卡壳法,结合固液相粒子圆度概念,实现了混合相态云中液滴和冰晶的相态识别;再结合光学图像识别技术,获得冰晶粒子的面积、周长、凸包和最小外接矩形数据;最终利用上述数据获得了冰晶微物理参数。通过在低温云室中的观测实验,获取了板状、枝状和六角冰晶的微物理参数,该方法解决了冰晶现有观测技术无法区分相态,以及获取微物理参数不全的瓶颈问题。同时,提升了云中液滴和冰晶的识别精度,对人工影响天气作业具有重大意义。
2 基本原理
同轴DH具有光路简单、信息密度高、远场条件下粒子双像干扰小等优点,广泛应用于三维动态场的记录和重建[16-20]。当平面波照射粒子时,粒子产生的衍射光(作为物体光)与未受干扰的光(作为参考光)发生干涉。干涉信息通过全息图记录下来。然后,利用菲涅耳-基尔霍夫衍射公式计算物体光波的重构。当测量路径中包含n个粒子时,根据标量衍射理论,全息图重建平面的强度分布UR(u,v)[21]可表示为
式中:λ为波长;R(x, y)为参考光;IH(x,y)为记录介质上干涉条纹的强度;k为波数[22];x和y分别表示粒子聚焦平面的水平和垂直坐标;u和v分别表示重建全息图所在平面的水平和垂直坐标;zR=zi(i=1,2,3,…)为重建距离。重建距离平面内的粒子聚焦且成像清晰,其他位置的粒子离焦且成像处于模糊状态。在第i平面上对每个全息图进行数值重建后,可以得到一系列不同时刻粒子的三维位置[23]。
为了确保粒子相态识别的准确性,粒子须在聚焦位置被识别。因此,本文使用再现距离与全域数字图像融合的方法,结合局部亮度梯度方差法(LTV),局部像素区域的宽和高分别为粒子x和y方向尺寸的两倍,高精度确定粒子的z轴位置[24]。LTV计算公式如下所示:
式中:GI为粒子局部区域内的梯度;
式中:*为卷积运算符号;I为局部区域内的亮度;Sobel算子的Sx和Sy因子分别为
在进行图像分析时,使用多边形近似来减少顶点数。在已知物体边界条件下,用最小外接矩形[25]的大小来描述基本轮廓是最简单的方法。由于检测到的颗粒尺寸较小,本文选择最小面积的外接矩形。本文用旋转卡壳法求出最小外接矩形,旋转卡壳法是对旋转法(在90°范围内以等间隔旋转图像对象)的改进。首先需要得到二维图像区域的凸包,然后沿凸边构建外接矩形,根据与下个凸边的夹角来连续旋转外接矩形而得到不同面积的外接矩形,从而比较得到最小的外接矩形。与计算量较大的旋转方法相比,该方法不仅可以节省时间,而且可以保证检测结果更加准确[26]。
在粒子聚焦位置被确定以及凸包和最小外接矩形被求出后,可以提取冰晶的质心三维坐标(x,y,z)、粒子面积Si、粒子周长Li、凸包面积So、凸包周长Lo、矩形长轴L、矩形短轴W、矩形面积Sr、矩形周长Lr和等效直径等信息。根据
按照给定的圆度阈值进行粒子相态识别,即可筛选出冰晶粒子。在此基础上,根据
3 实验系统
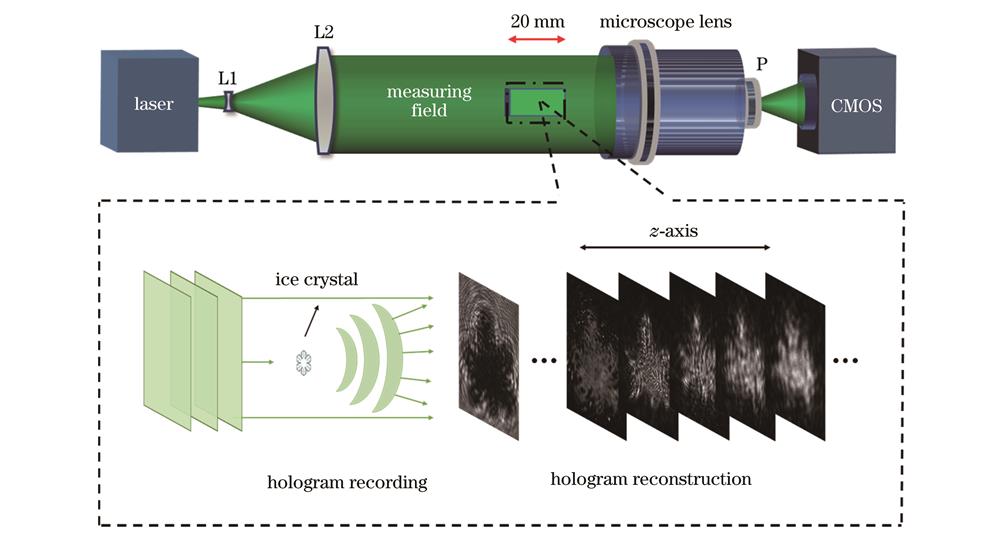
图 1. 冰晶观测与识别的同轴DH系统
Fig. 1. Coaxial DH system for ice crystal observation and identification
为了实现对液滴和冰晶混合相的观测。在如

图 3. 冰晶的生成与消散过程。(a)、(b)冰晶的增加过程;(c)冰晶的减少过程
Fig. 3. Formation and dissipation of ice crystals. (a), (b) Process of increasing ice crystals; (c) process of ice crystals reduction
4 算法与实验结果讨论
4.1 冰晶粒子微物理参数获取算法
冰晶粒子识别流程如
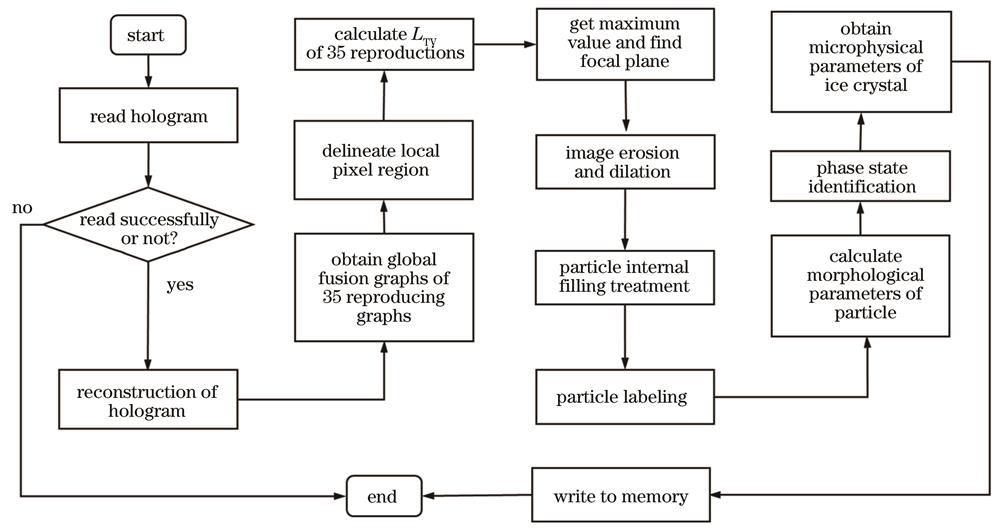
图 4. 冰晶粒子微物理参数获取算法流程图
Fig. 4. Flow chart of microphysical parameter acquisition algorithm for ice crystal particles
4.2 实验数据与分析
在获得液滴和冰晶混合相态的数字全息图后,需要对每一个粒子进行判焦,获得其清晰再现像。沿z轴在整个40 mm的测量长度上,选取了35个再现平面。每个粒子在这些再现平面上都会成像,利用全域数字图像融合方法将35张再现全息图融合为一张,如
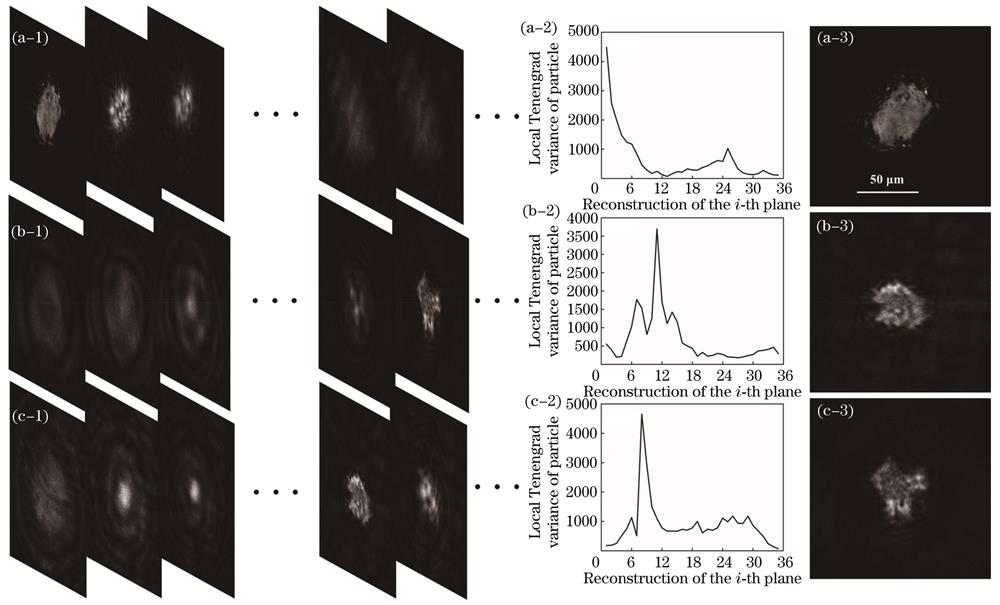
图 6. 局部亮度梯度方差与粒子判焦。(a-1)、(b-1)、(c-1)35个再现平面;(a-2)、(b-2)、(c-2)粒子的局部亮度梯度方差曲线;(a-3)、(b-3)、(c-3)清晰的成像位置
Fig. 6. Local Tenengrad variance and determine focus of particles. (a-1), (b-1), (c-1) 35 reproduction planes; (a-2), (b-2), (c-2) curves of local Tenengrad variance of particles; (a-3), (b-3), (c-3) clear imaging positions
获得每一个粒子清晰像后,对清晰像所处的那张再现全息图按照特定的阈值进行二值化。再对二值化图分别使用一次开运算和闭运算,可使粒子的边缘形成平滑的闭合曲线。如果粒子内部存在空洞,再使用填洞算法将空洞置1。对获得的完整粒子进行贴标签,获得每个粒子的包含像素的外轮廓,如

图 7. 冰晶与液滴的识别图。(a-1)、(a-2)、(a-3)不同冰晶的识别图;(b-1)、(b-2)、(b-3)不同液滴的识别图
Fig. 7. Identification of ice crystals and droplets. (a-1), (a-2), (a-3) Identification of different ice crystals; (b-1), (b-2), (b-3) identification of different droplets

图 8. 冰晶与液滴粒子的圆度曲线
Fig. 8. Roundness curves of ice crystal particles and droplet particles
识别出混合相态中的冰晶粒子后,可对冰晶粒子进行形态学分析。为了获得冰晶微物理参数,需要获得冰晶的质心三维坐标、粒子面积、粒子周长、凸包面积、凸包周长、矩形长短轴、矩形面积和矩形周长9个参数。
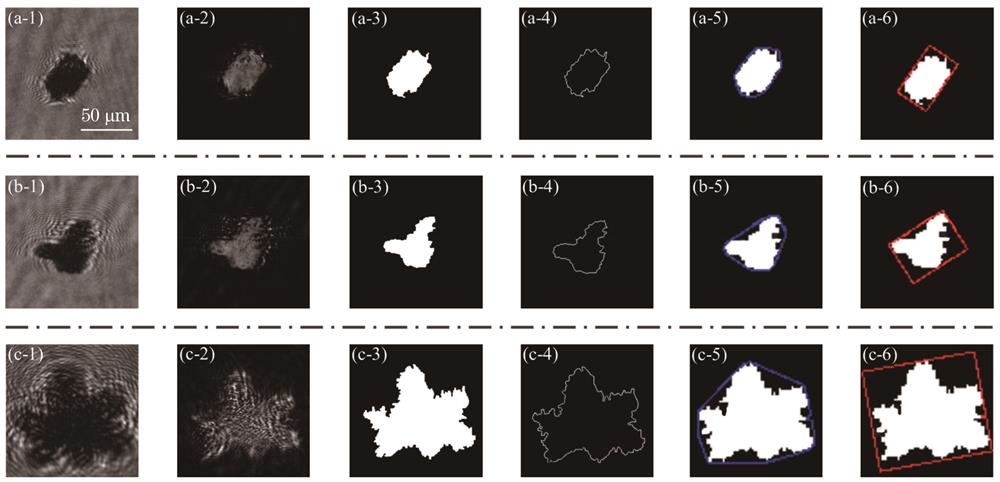
图 9. 冰晶粒子的形态学分析结果。(a-1)、(b-1)、(c-1)粒子的原始全息图;(a-2)、(b-2)、(c-2)粒子清晰的成像位置;(a-3)、(b-3)、(c-3)粒子的识别图;(a-4)、(b-4)、(c-4)粒子的轮廓提取;(a-5)、(b-5)、(c-5)粒子的凸包提取;(a-6)、(b-6)、(c-6)粒子的最小外接矩形提取
Fig. 9. Morphological analysis of ice crystal particles. (a-1), (b-1), (c-1) Original holograms of particles; (a-2), (b-2), (c-2) clear imaging positions of particles; (a-3), (b-3), (c-3) identification of particles; (a-4), (b-4), (c-4) contour extraction of particles; (a-5), (b-5), (c-5) convex hull extraction of particles; (a-6), (b-6), (c-6) extraction of smallest external rectangle of a particle
表 1. 图9中3个冰晶粒子的形态学数据
Table 1. Morphological data of three ice crystal particles in Fig. 9
|
表 2. 图9中3个冰晶粒子的微物理参数
Table 2. Microphysical parameters of three ice crystal particles in Fig. 9
|
在实验中,当利用30 frame/s的速度获取冰晶粒子数字全息图时,该方法还可获得冰晶粒子的动力学三维速度。图
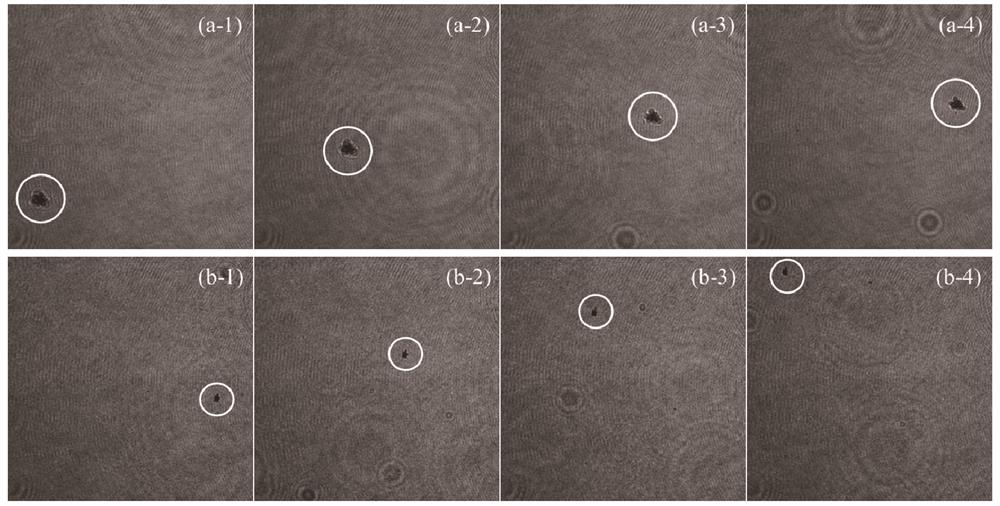
图 10. 冰晶粒子的运动全息图。(a-1)~(a-4)第一个冰晶粒子的运动全息图;(b-1)~(b-4)第二个冰晶粒子的运动全息图
Fig. 10. Motion holograms of ice crystal particles. (a-1)-(a-4) Motion hologram of first ice crystal particle; (b-1)-(b-4) motion hologram of second ice crystal particle
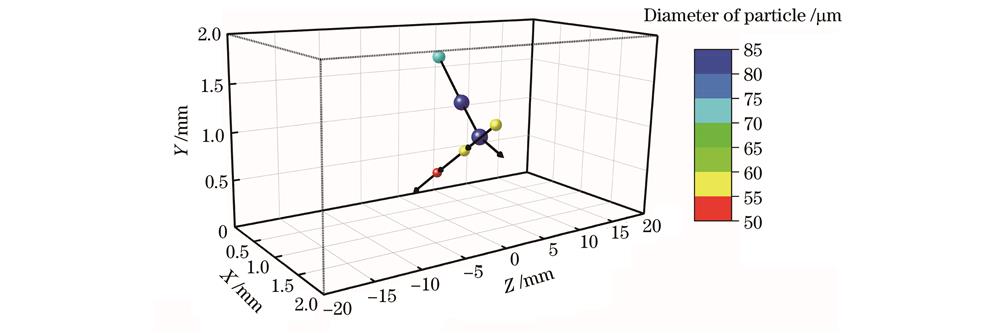
图 11. 冰晶粒子的三维运动轨迹
Fig. 11. Three-dimensional motion trajectories of ice crystal particles
5 结论
针对云中混合相态和单固相冰晶粒子的测量需求,本文提出了同轴DH的观测方法,结合粒子圆度,可实现冰晶和液滴混合相态中冰晶粒子的提取,以及冰晶形态学和动力学参数的测量。该方法提高了粒子相态识别率以及冰晶三维速度计算的准确性,在之后的实验中可以进一步开展以下工作:优化系统以减小系统误差;增加再现面来提高z轴的准确度以提高测量准确性,减小计算误差。然而,由于本文方法是一维观测,因此只能获得粒子投影截面。当计算固态水含量时,需要粒子厚度。因此利用该方法进行二维测量时,可进一步得到固态水含量。本文方法的空基应用可为研究卷云、高积云、高层云及雨层云提供有效的技术保障,也可为不同模型的数据验证提供可靠的数据支持。
[1] Yuan J L, Xia H Y, Wei T W, et al. Identifying cloud, precipitation, windshear, and turbulence by deep analysis of the power spectrum of coherent Doppler wind lidar[J]. Optics Express, 2020, 28(25): 37406-37418.
[2] 宫纯文, 魏合理, 李学彬, 等. 取向比对圆柱状冰晶粒子光散射特性的影响[J]. 光学学报, 2009, 29(5): 1155-1159.
[3] Wang D X, Stachlewska I S, Delanoë J, et al. Spatio-temporal discrimination of molecular, aerosol and cloud scattering and polarization using a combination of a Raman lidar, Doppler cloud radar and microwave radiometer[J]. Optics Express, 2020, 28(14): 20117-20134.
[4] 姚娜, 张苗苗, 卜令兵, 等. 基于机载高光谱分辨率激光雷达的气溶胶分类研究[J]. 光学学报, 2023, 43(24): 2428005.
[5] Borovoi A, Galileiskii V, Morozov A, et al. Detection of ice crystal particles preferably oriented in the atmosphere by use of the specular component of scattered light[J]. Optics Express, 2008, 16(11): 7625-7633.
[6] 方颖, 孙晓兵, 提汝芳, 等. 基于高分五号02星偏振载荷的冰雪上空云检测算法研究[J]. 光学学报, 2023, 43(24): 2428004.
[7] Ding J C, Yang P, Holz R E, et al. Ice cloud backscatter study and comparison with CALIPSO and MODIS satellite data[J]. Optics Express, 2016, 24(1): 620-636.
[8] 黄亦鹏, 李万彪, 赵玉春, 等. 基于雷达与卫星的对流触发观测研究和临近预报技术进展[J]. 地球科学进展, 2019, 34(12): 1273-1287.
Huang Y P, Li W B, Zhao Y C, et al. A review of radar-and satellite-based observational studies and nowcasting techniques on convection initiation[J]. Advances in Earth Science, 2019, 34(12): 1273-1287.
[9] 张铮, 曹静. 光固印模法测量人造冰晶和雾滴[J]. 气象学报, 1985, 43(2): 172-179.
Zhang Z, Cao J. Measuring artificial droplets and ice crystals by photosolidified replication[J]. Acta Meteorologica Sinica, 1985, 43(2): 172-179.
[10] Zhang Y Y, Zhao J L, Di J L, et al. Real-time monitoring of the solution concentration variation during the crystallization process of protein-lysozyme by using digital holographic interferometry[J]. Optics Express, 2012, 20(16): 18415-18421.
[11] 戴思清, 豆嘉真, 张继巍, 等. 基于数字全息术的近场成像与应用[J]. 光学学报, 2020, 40(1): 0111008.
[12] Das B, Yelleswarapu C S. Dual plane in-line digital holographic microscopy[J]. Optics Letters, 2010, 35(20): 3426-3428.
[13] Sang X Z, Yu C X, Yu M, et al. Applications of digital holography to measurements and optical characterization[J]. Optical Engineering, 2011, 50(9): 091311.
[14] 刘雅坤, 肖文, 车蕾平, 等. 基于数字全息显微层析的癌细胞空泡化成像研究[J]. 中国激光, 2022, 49(20): 2007209.
[15] Bellanger C, Brignon A, Colineau J, et al. Coherent fiber combining by digital holography[J]. Optics Letters, 2008, 33(24): 2937-2939.
[16] 张熙, 王绶玙, 孔艳, 等. 透射式K空间变换数字全息三维成像技术研究[J]. 中国激光, 2021, 48(21): 2109001.
[17] Solís S M, Santoyo F M, del Socorro Hernández-Montes M. 3D displacement measurements of the tympanic membrane with digital holographic interferometry[J]. Optics Express, 2012, 20(5): 5613-5621.
[18] 何炳恩, 张利萍, 龚湘君, 等. 基于数字全息显微技术的微纳粒子动态行为表征[J]. 激光与光电子学进展, 2023, 60(8): 0811006.
[19] Yao L C, Chen J, Sojka P E, et al. Three-dimensional dynamic measurement of irregular stringy objects via digital holography[J]. Optics Letters, 2018, 43(6): 1283-1286.
[20] Molaei M, Sheng J. Imaging bacterial 3D motion using digital in-line holographic microscopy and correlation-based de-noising algorithm[J]. Optics Express, 2014, 22(26): 32119-32137.
[21] 高攀, 王骏, 赵成成, 等. 基于数字全息干涉术的云微物理参数同步测量方法[J]. 物理学报, 2021, 70(9): 099201.
Gao P, Wang J, Zhao C C, et al. Simultaneous measurement of cloud microphysical parameters based on digital holographic interferometry[J]. Acta Physica Sinica, 2021, 70(9): 099201.
[22] Gao P, Wang J, Tang J B, et al. Investigation of cloud droplets velocity extraction based on depth expansion and self-fusion of reconstructed hologram[J]. Optics Express, 2022, 30(11): 18713-18729.
[23] Singh D K, Panigrahi P K. Improved digital holographic reconstruction algorithm for depth error reduction and elimination of out-of-focus particles[J]. Optics Express, 2010, 18(3): 2426-2448.
[24] Yao L C, Wu C Y, Wu Y C, et al. Investigating particle and volatile evolution during pulverized coal combustion using high-speed digital in-line holography[J]. Proceedings of the Combustion Institute, 2019, 37(3): 2911-2918.
[25] 董鹏, 周烽, 赵悰悰, 等. 基于双目视觉的水下海参尺寸自动测量方法[J]. 计算机工程与应用, 2021, 57(8): 271-278.
Dong P, Zhou F, Zhao C C, et al. Automatic measurement of underwater sea cucumber size based on binocular vision[J]. Computer Engineering and Applications, 2021, 57(8): 271-278.
[26] 王书宇, 田建艳, 蔡文站, 等. 基于孔特征的弱纹理堆叠工件识别[J]. 计算机工程与应用, 2022, 58(3): 282-288.
Wang S Y, Tian J Y, Cai W Z, et al. Recognition of stacked texture-less workpieces based on hole features[J]. Computer Engineering and Applications, 2022, 58(3): 282-288.
[27] 黄敏松, 雷恒池. 改进的Holroyd云粒子形状识别方法及其应用[J]. 气象学报, 2020, 78(2): 289-300.
Huang M S, Lei H C. An improved Holroyd cloud particle habit identification method and its application[J]. Acta Meteorologica Sinica, 2020, 78(2): 289-300.
[28] 张荣, 李宏宇, 周旭, 等. DMT机载云粒子图像形状识别及其应用[J]. 应用气象学报, 2021, 32(6): 735-747.
Zhang R, Li H Y, Zhou X, et al. Shape recognition of DMT airborne cloud particle images and its application[J]. Journal of Applied Meteorological Science, 2021, 32(6): 735-747.
[29] 宋世坤, 王书海. 基于BP神经网络的雪花形状分类研究[J]. 无线互联科技, 2018, 15(18): 109-110.
Song S K, Wang S H. Study on the shape classification of snowflakes based on BP neural network[J]. Wireless Internet Technology, 2018, 15(18): 109-110.
Article Outline
杨晨遇, 王骏, 张川, 周浩, 杨军胜, 岳治国, 梁谷, 刘晶晶, 华灯鑫. 基于数字全息的云中冰晶微物理参数观测方法[J]. 光学学报, 2024, 44(6): 0601017. Chenyu Yang, Jun Wang, Chuan Zhang, Hao Zhou, Junsheng Yang, Zhiguo Yue, Gu Liang, Jingjing Liu, Dengxin Hua. Observation Method of Microphysical Parameters of Ice Crystals in Cloud Based on Digital Holography[J]. Acta Optica Sinica, 2024, 44(6): 0601017.