激光与光电子学进展, 2020, 57 (14): 141024, 网络出版: 2020-07-28
基于级联残差生成对抗网络的低照度图像增强
下载: 970次
Low-Light Image Enhancement Based on Cascaded Residual Generative Adversarial Network
图 & 表
图 4. 不同图像。(a)正常照度的River和Car;(b)照度为0.2的图像;(c)照度为0.35的图像;(d)照度为0.5的图像;(e)照度为0.2时HSV颜色空间图像;(f) H分量;(g) S分量;(h) V分量
Fig. 4. Different images. (a) River and car of normal-light images; (b) images with illuminance of 0.2; (c) images with illuminance of 0.35; (d) images with illuminance of 0.5; (e) HSV color space images with illuminance of 0.2; (f) H component; (g) S component; (h) V component

图 6. 不同算法对低照度图像Starfish的增强结果。(a)低照度图像;(b)正常照度图像;(c)文献[
6]算法;(d)文献[
7]算法;(e)文献[
8]算法;(f)文献[
9]算法;(g)文献[
10]算法;(h)文献[
11]算法;(i)文献[
12
Fig. 6. Enhanced results of low-light image Starfish by different algorithms. (a) Low-light image; (b) normal-light image; (c) Ref. [6] algorithm; (d) Ref. [7] algorithm; (e) Ref. [8] algorithm; (f) Ref. [9] algorithm; (g) Ref. [10] algorithm; (h) Ref. [11] algorithm; (i) Ref. [12] algorithm; (j) proposed algorithm

图 7. 不同算法对低照度图像Man的增强结果。(a)低照度图像;(b)正常照度图像;(c)文献[
6]算法;(d)文献[
7]算法;(e)文献[
8]算法;(f)文献[
9]算法;(g)文献[
10]算法;(h)文献[
11]算法;(i)文献[
12Fig. 7. Enhanced results of low-light image Man by different algorithms. (a) Low-light image; (b) normal-light image; (c) Ref. [6] algorithm; (d) Ref. [7] algorithm; (e) Ref. [8] algorithm; (f) Ref. [9] algorithm; (g) Ref. [10] algorithm; (h) Ref. [11] algorithm; (i) Ref. [12] algorithm; (j) proposed algorithm
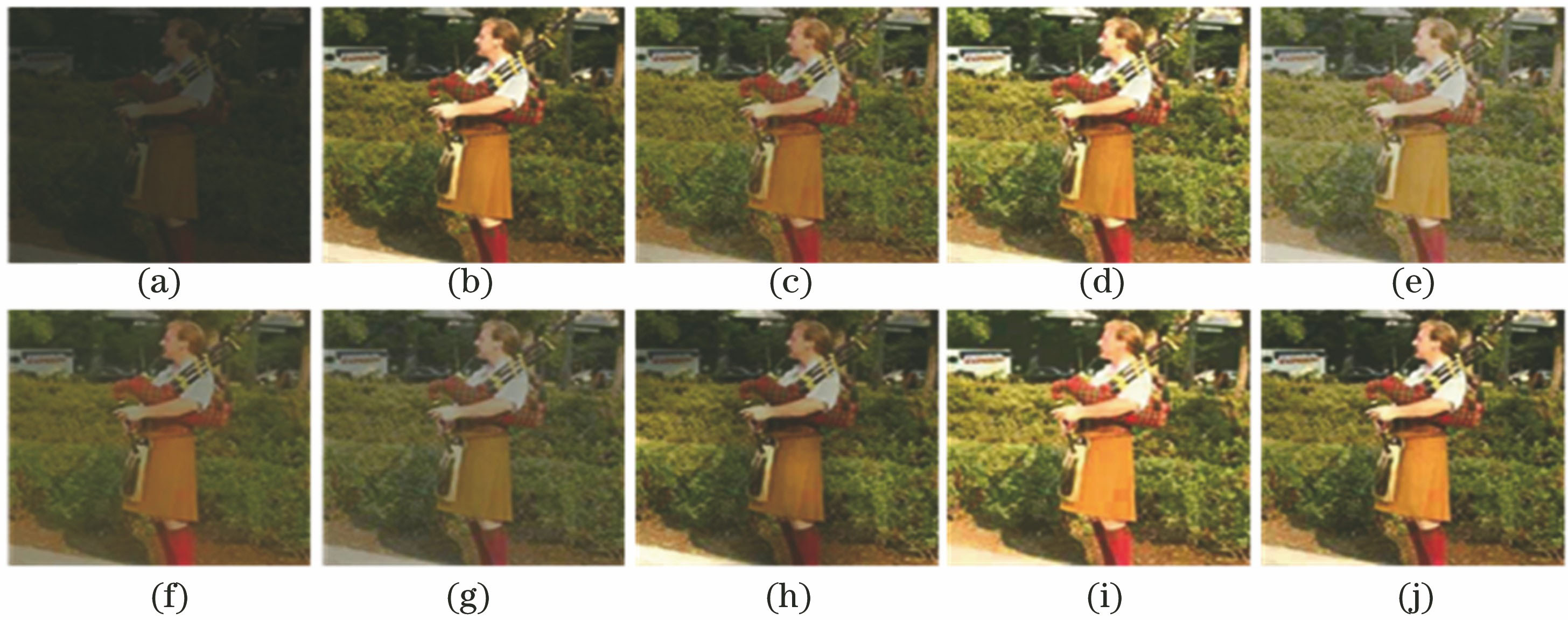
Fig. 7. Enhanced results of low-light image Man by different algorithms. (a) Low-light image; (b) normal-light image; (c) Ref. [6] algorithm; (d) Ref. [7] algorithm; (e) Ref. [8] algorithm; (f) Ref. [9] algorithm; (g) Ref. [10] algorithm; (h) Ref. [11] algorithm; (i) Ref. [12] algorithm; (j) proposed algorithm
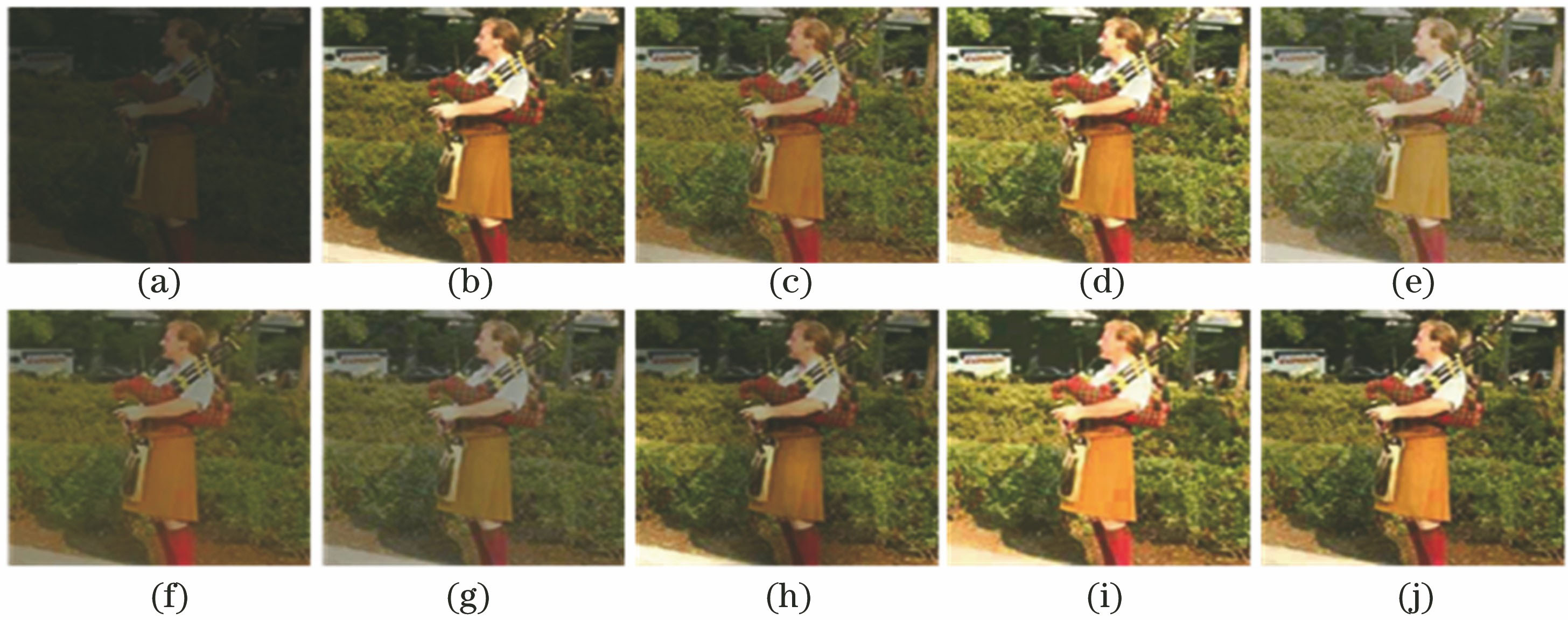
图 8. 不同算法对低照度图像Street的增强结果。(a)低照度图像;(b)正常照度图像;(c)文献[
6]算法;(d)文献[
7]算法;(e)文献[
8]算法;(f)文献[
9]算法;(g)文献[
10]算法;(h)文献[
11]算法;(i)文献[
12
Fig. 8. Enhanced results of low-light image Street by different algorithms. (a) Low-light image; (b) normal-light image; (c) Ref. [6] algorithm; (d) Ref. [7] algorithm; (e) Ref. [8] algorithm; (f) Ref. [9] algorithm; (g) Ref. [10] algorithm; (h) Ref. [11] algorithm; (i) Ref. [12] algorithm; (j) proposed algorithm
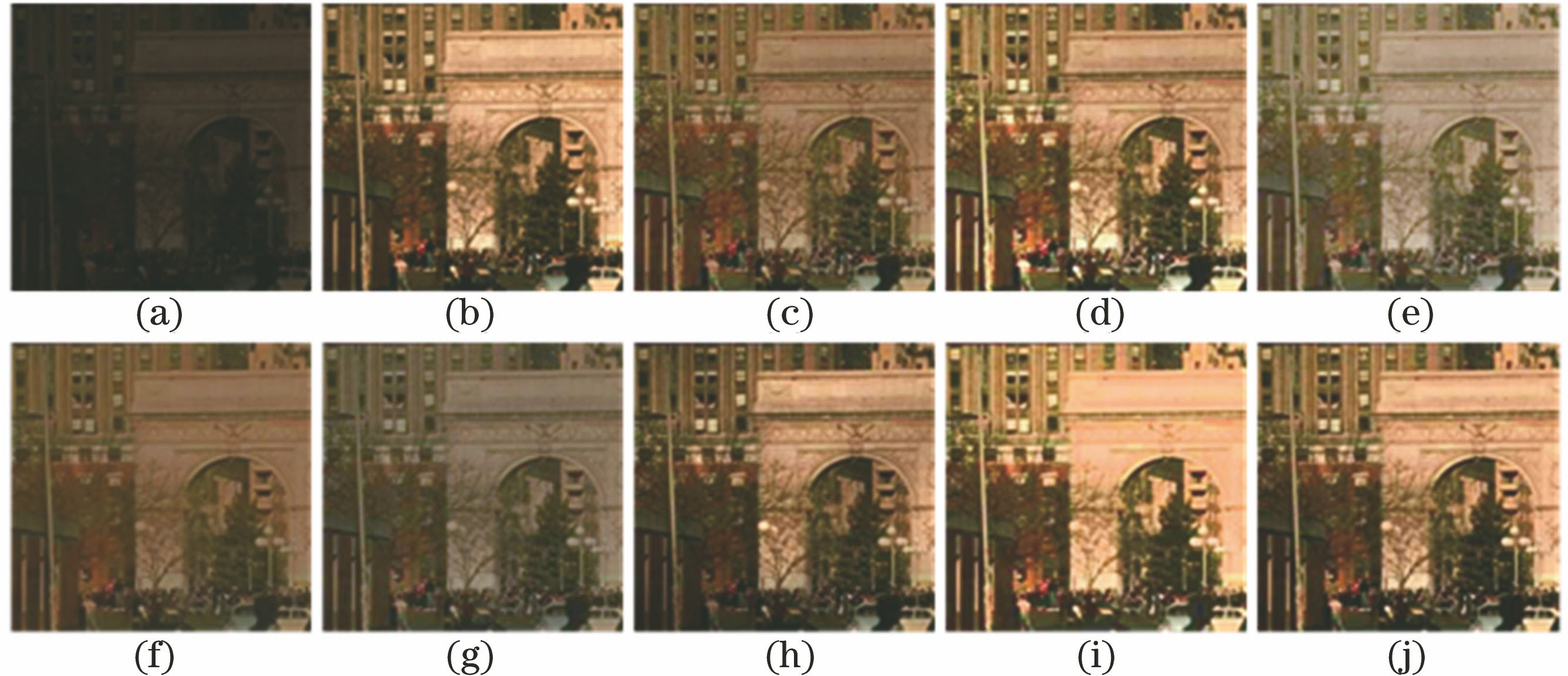
图 9. 不同算法对真实的低照度图像Pocky的增强结果。(a)真实的低照度图像;(b)文献[ 6]算法;(c)文献[ 7]算法;(d)文献[ 8]算法;(e)文献[ 9]算法;(f)文献[ 10]算法;(g)文献[ 11]算法;(h)文献[ 12
Fig. 9. Enhancement results of real low-light image Pocky by different algorithms. (a) Real low-light image; (b) Ref. [6] algorithm; (c) Ref. [7] algorithm; (d) Ref. [8] algorithm; (e) Ref. [9] algorithm; (f) Ref. [10] algorithm; (g) Ref. [11] algorithm; (h) Ref. [12] algorithm; (i) proposed algorithm

图 10. 不同算法对真实低照度图像Palace的增强效果。(a)真实的低照度图像;(b)文献[ 6]算法;(c)文献[ 7]算法;(d)文献[ 8]算法;(e)文献[ 9]算法;(f)文献[ 10]算法;(g)文献[ 11]算法;(h)文献[ 12
Fig. 10. Enhancement results of real low-light image Palace by different algorithms. (a) Real low-light image; (b) Ref. [6] algorithm; (c) Ref. [7] algorithm; (d) Ref. [8] algorithm; (e) Ref. [9] algorithm; (f) Ref. [10] algorithm; (g) Ref. [11] algorithm; (h) Ref. [12] algorithm; (i) proposed algorithm

图 11. 不同算法对比结果。(a)均值;(b)平均梯度
Fig. 11. Comparison results of different algorithms. (a) Average; (b) average gradient

图 12. 生成器网络与生成对抗网络模型对低照度图像增强结果的主观对比。(a)低照度图像;(b)正常照度图像;(c)生成器网络;(d)生成对抗网络
Fig. 12. Subjective comparison of low-light image enhancement results by generator network and generative adversarial network. (a) Low-light image; (b) normal-light image; (c) results of generator network; (d) results of generative adversarial network

表 1不同算法的PSNR和SSIM
Table1. PSNR and SSIM of different algorithms
|
陈清江, 屈梅. 基于级联残差生成对抗网络的低照度图像增强[J]. 激光与光电子学进展, 2020, 57(14): 141024. Qingjiang Chen, Mei Qu. Low-Light Image Enhancement Based on Cascaded Residual Generative Adversarial Network[J]. Laser & Optoelectronics Progress, 2020, 57(14): 141024.