北京邮电大学信息光子学与光通信国家重点实验室,北京 100876
针对电子计算中摩尔定律不断减慢、电子晶体管的规模接近物理极限等造成的计算速度难以进一步提高的问题,提出了一种基于VGG16的衍射光子神经网络(VGG16-DONN)结构。该结构利用光衍射层作为VGG16的光学前端,替换了VGG16中计算耗时占比最大的第一层电卷积层,分别对CelebA数据集和猫狗数据集进行分类(分类精度分别达到86.34%和88.53%),实现了与电子神经网络相当的分类精度。此外,基于此结构,提出了一种面向情境依赖处理(CDP)的VGG16-DONN方法,对CelebA数据集进行分类(平均精度为83.10%),同样达到了与电子神经网络相当的分类精度。不难看出,VGG16-DONN以及其与CDP模块相结合的方式,除了能够借助光计算速度快的优势克服电子神经网络计算慢的问题外,还能够达到与电子神经网络相当的精度,这对于图像处理、医疗、通信等领域都具有重要意义。
光计算 衍射光子神经网络 情境依赖处理 正交权重修改算法 光学学报
2022, 42(19): 1920001
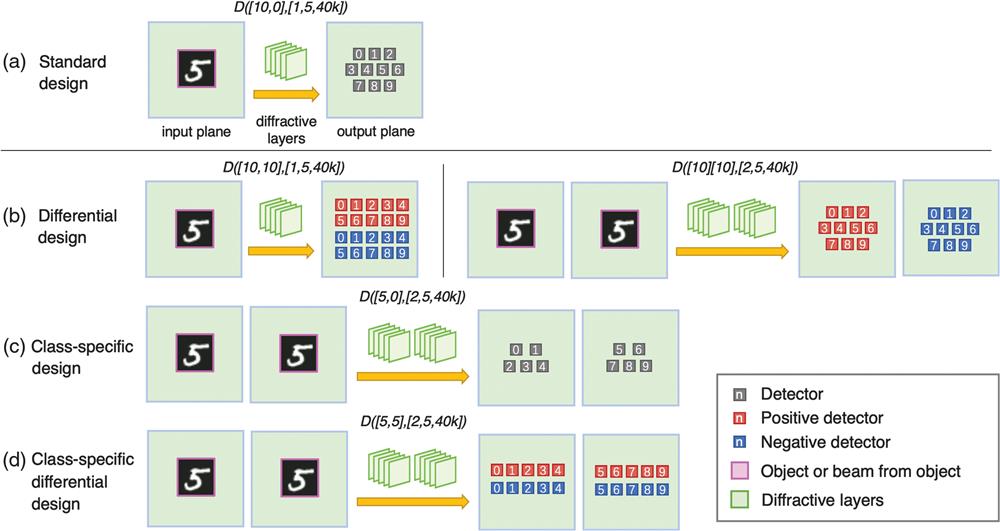
Jingxi Li 1,2,3†Deniz Mengu 1,2,3Yi Luo 1,2,3Yair Rivenson 1,2,3Aydogan Ozcan 1,2,3,*
Author Affiliations
Abstract
1 University of California at Los Angeles, Department of Electrical and Computer Engineering, Los Angeles, California, United States
2 University of California at Los Angeles, Department of Bioengineering, Los Angeles, California, United States
3 University of California at Los Angeles, California NanoSystems Institute, Los Angeles, California, United States
Optical computing provides unique opportunities in terms of parallelization, scalability, power efficiency, and computational speed and has attracted major interest for machine learning. Diffractive deep neural networks have been introduced earlier as an optical machine learning framework that uses task-specific diffractive surfaces designed by deep learning to all-optically perform inference, achieving promising performance for object classification and imaging. We demonstrate systematic improvements in diffractive optical neural networks, based on a differential measurement technique that mitigates the strict nonnegativity constraint of light intensity. In this differential detection scheme, each class is assigned to a separate pair of detectors, behind a diffractive optical network, and the class inference is made by maximizing the normalized signal difference between the photodetector pairs. Using this differential detection scheme, involving 10 photodetector pairs behind 5 diffractive layers with a total of 0.2 million neurons, we numerically achieved blind testing accuracies of 98.54%, 90.54%, and 48.51% for MNIST, Fashion-MNIST, and grayscale CIFAR-10 datasets, respectively. Moreover, by utilizing the inherent parallelization capability of optical systems, we reduced the cross-talk and optical signal coupling between the positive and negative detectors of each class by dividing the optical path into two jointly trained diffractive neural networks that work in parallel. We further made use of this parallelization approach and divided individual classes in a target dataset among multiple jointly trained diffractive neural networks. Using this class-specific differential detection in jointly optimized diffractive neural networks that operate in parallel, our simulations achieved blind testing accuracies of 98.52%, 91.48%, and 50.82% for MNIST, Fashion-MNIST, and grayscale CIFAR-10 datasets, respectively, coming close to the performance of some of the earlier generations of all-electronic deep neural networks, e.g., LeNet, which achieves classification accuracies of 98.77%, 90.27%, and 55.21% corresponding to the same datasets, respectively. In addition to these jointly optimized diffractive neural networks, we also independently optimized multiple diffractive networks and utilized them in a way that is similar to ensemble methods practiced in machine learning; using 3 independently optimized differential diffractive neural networks that optically project their light onto a common output/detector plane, we numerically achieved blind testing accuracies of 98.59%, 91.06%, and 51.44% for MNIST, Fashion-MNIST, and grayscale CIFAR-10 datasets, respectively. Through these systematic advances in designing diffractive neural networks, the reported classification accuracies set the state of the art for all-optical neural network design. The presented framework might be useful to bring optical neural network-based low power solutions for various machine learning applications and help us design new computational cameras that are task-specific.
optical computation optical neural networks deep learning optical machine learning diffractive deep neural networks Advanced Photonics
2019, 1(4): 046001
为了方便快速地计算像差,采用MATLAB中GUI工具,从球面光路计算的向量公式出发,得到了一计算像差的程序界面,在程序界面输入各个相关参量,点击界面上各种像差菜单就能得到不同视场角在光瞳上不同入射点的各种像差,并可以计算系统的焦距和理想像面的像距以及给出像面的模糊程度(同一物点发出的不同方向光线的聚焦程度)的具体数值。结果表明,该软件程序语言简单,程序语句大大减少,占用机时少,运行结果正确。程序通过编译后可以脱离MATLAB运行环境独立运行。
几何光学 光路计算 像差 程序界面 geometrical optics optical computation geometrical aberration program interface
本文介绍全并行矩阵乘法的实验研究过程和结果,运算充分利用光的并行性,做一次三个矩阵乘法或二维变换只要一个系统时钟周期。文中讨论了误差的来源和误差的消除方法,给出了两个和三个(正实数)矩阵乘法的实验结果(模拟运算),两个矩阵相乘的精度为1.2%,三个矩阵相乘的精度为1.23%。
光计算技术 信息处理 图像变换
本文给出了执行光学并行阵列逻辑的一种新的空间振幅编码图形法的原理和特性。为了设计高速并行复合运算,本文还给出了系统的算法,并澄清了AND和OR阵列门的物理意义。也给出了在8f相干光学处理系统中执行的16种复合逻辑运算的结果。
数字光计算 光学阵列逻辑 空间编码图形法
根据图像反差倒转的概念,提出了一种高效率产生多光束照明信号的新方法.这种技术可望应用于研制阵列处理器或光计算机所需的阵列信号光源.本文给出了产生阵列信号光源的实验结果,并进行了讨论.
光计算 阵列照明器 反差倒转
将二进制数的先行进位全加器的逻辑操作引入到光计算中,提出了用光学并行处理方法实现先行进位全加器逻辑操作的建议。信号以空间阵列方式编码,利用空间逻辑阵列,实现并行逻辑操作。研究了PD-LED的光电混合逻辑操作,利用PD—LED逻辑器件,从实验上验证了先行进位光学并行多位全加器,获得了满意的结果。
光计算 全加器 PD-LED逻辑