激光与光电子学进展, 2019, 56 (15): 153002, 网络出版: 2019-08-05
基于分数阶微分和连续投影算法-反向传播神经网络的小麦叶片含水量高光谱估算
下载: 976次
Hyperspectral Estimation of Wheat Leaf Water Content Using Fractional Differentials and Successive Projection Algorithm-Back Propagation Neural Network
图 & 表
图 1. 春小麦冠层光谱曲线。(a)不同含水量春小麦冠层光谱曲线;(b) 0~2阶微分春小麦冠层光谱曲线
Fig. 1. Canopy spectral curves of spring wheat. (a) Canopy spectral curves of spring wheat with different water contents; (b) canopy spectral curves of spring wheat with 0-order to 2-order differentials
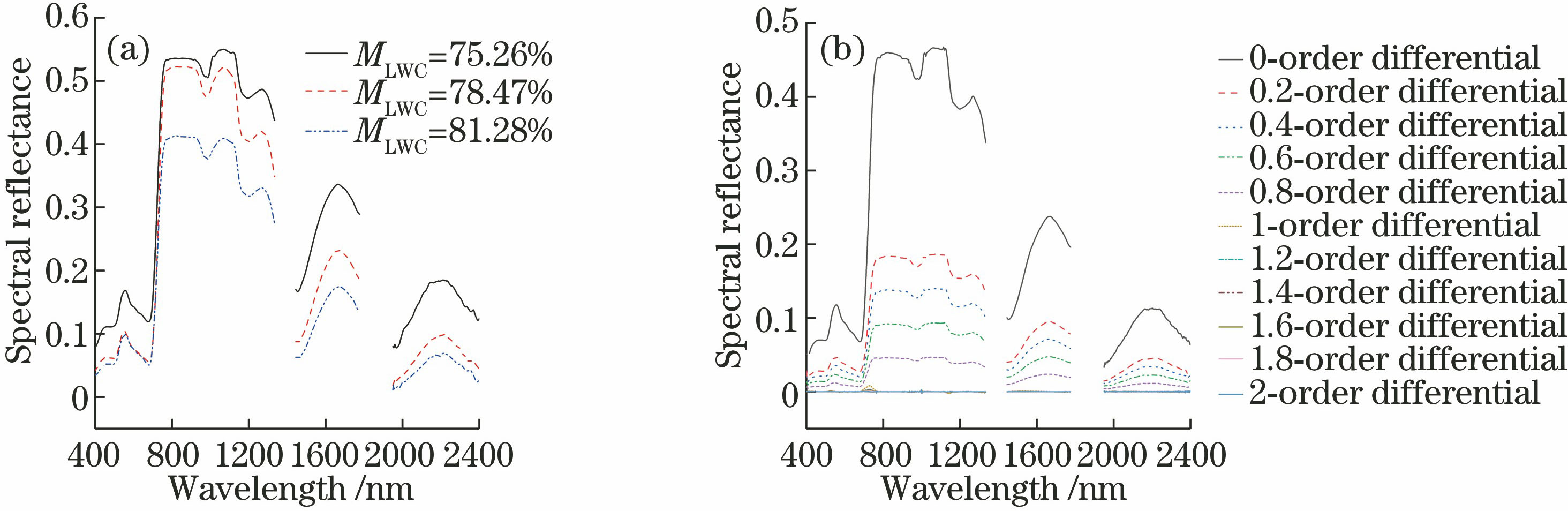
图 2. MLWC和光谱反射率的相关系数。(a) MLWC和0阶光谱的相关系数;(b) MLWC和0.2、0.4、0.6、0.8阶光谱的相关系数;(c) MLWC和1阶光谱的相关系数;(d) MLWC和1.2阶光谱的相关系数;(e) MLWC和1.4阶光谱的相关系数;(f) MLWC和1.6阶光谱的相关系数;(g) MLWC和1.8阶光谱的相关系数;(h) MLWC和2阶光谱的相关系数
Fig. 2. Correlation coef?cients between MLWC and spectral re?ectance. (a) Correlation coefficients between MLWC and 0-order spectrum; (b) correlation coefficients between MLWC and 0.2, 0.4, 0.6, 0.8-order spectra; (c) correlation coefficients between MLWC and 1-order spectrum; (d) correlation coefficients between MLWC and 1.2-order spectrum; (e) correlation coefficients between MLWC and 1.4-order spectrum; (f) correlation coefficients between MLWC and 1.6-order spectrum; (g) correlation coefficients bet

图 3. BP神经网络模型实测值与预测值的拟合分析结果。(a) 1阶微分;(b) 1.2阶微分;(c) 1.4阶微分;(d) 1.6阶微分;(e) 1.8阶微分;(f) 2阶微分
Fig. 3. Fitting analysis results between measured values and predicted values by BP neural network model. (a) 1-order differential; (b) 1.2-order differential; (c) 1.4-order differential; (d) 1.6-order differential; (e) 1.8-order differential; (f) 2-order differentials

表 1SPA筛选出的波段个数及组合
Table1. Numbers and combinations of bands selected by SPA
|
表 2建模结果比较
Table2. Comparison of modeling results
|
吾木提·艾山江, 买买提·沙吾提, 马春玥. 基于分数阶微分和连续投影算法-反向传播神经网络的小麦叶片含水量高光谱估算[J]. 激光与光电子学进展, 2019, 56(15): 153002. Hasan Umut, Sawut Mamat, Chunyue Ma. Hyperspectral Estimation of Wheat Leaf Water Content Using Fractional Differentials and Successive Projection Algorithm-Back Propagation Neural Network[J]. Laser & Optoelectronics Progress, 2019, 56(15): 153002.