光学学报, 2020, 40 (23): 2312003, 网络出版: 2020-11-23
基于机器学习对火焰温度场和CO2浓度场的同步重建
下载: 1305次
Machine-Learning-Based Reconstruction of Flame Temperature and CO2 Concentration Fields
图 & 表
图 1. 轴对称火焰温度场和CO2浓度场的测量原理
Fig. 1. Schematic of temperature and CO2 concentration fields measurements for axisymmetric flames
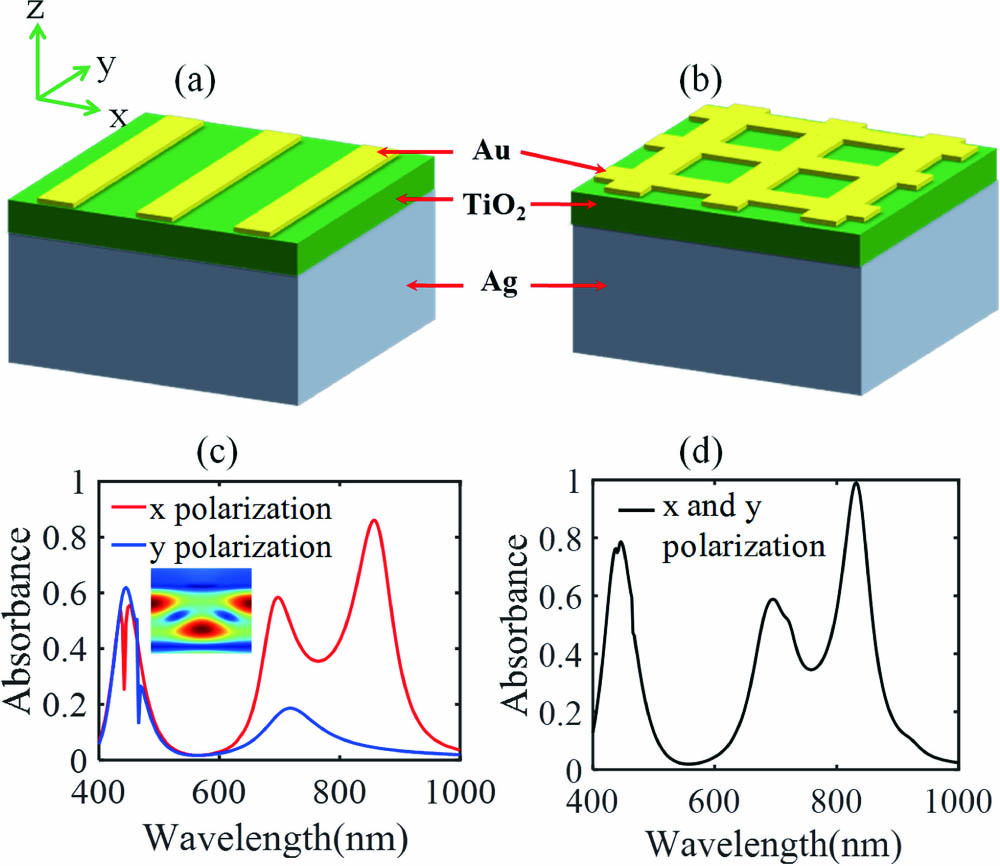
图 2. 传统测量方法示意图。(a)基于轴向和径向空间扫描式激光吸收光谱测量反演重建火焰温度场和CO2浓度场;(b)基于轴向扫描式激光吸收光谱测量反演重建火焰温度场和CO2浓度场
Fig. 2. Schematics of traditional measurement method. (a) Reconstruction of temperature and CO2 concentration fields based on axial and radial laser spectral absorption measurements; (b) reconstruction of temperature and CO2 concentration fields based on axial laser spectral absorption measurement
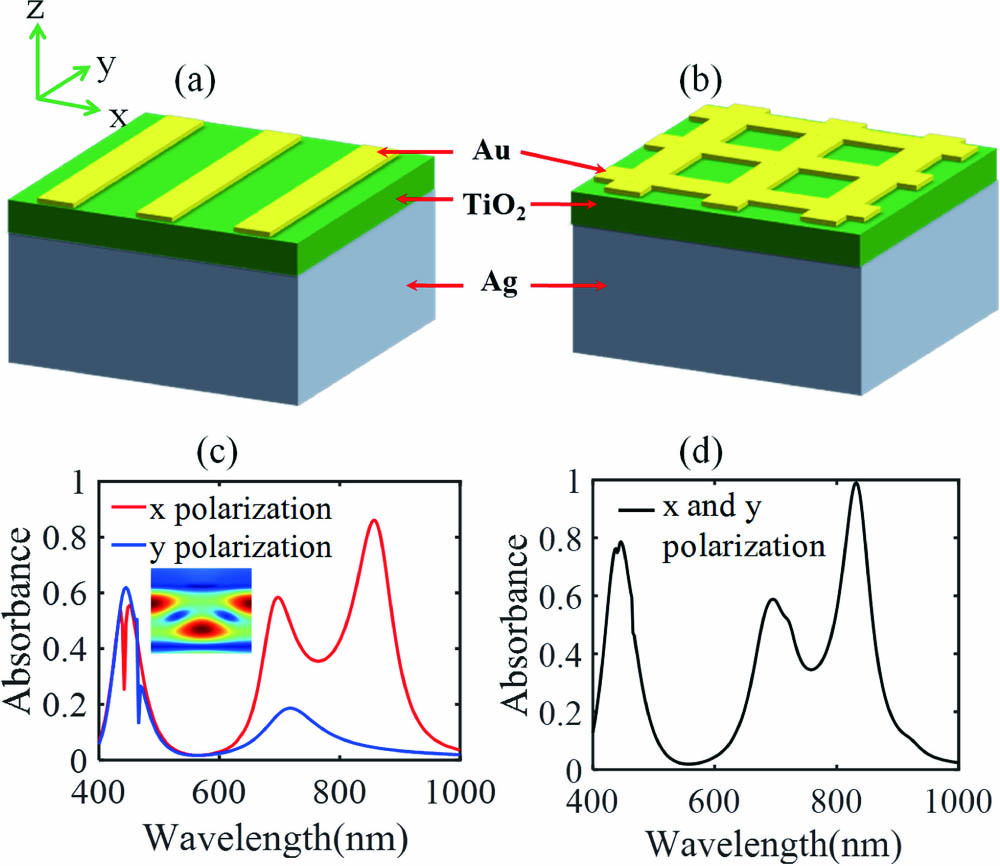
图 3. 基于机器学习的温度场和浓度场的同步反演重建模型
Fig. 3. Machine-learning-based reconstruction model for temperature and concentration fields retrieval
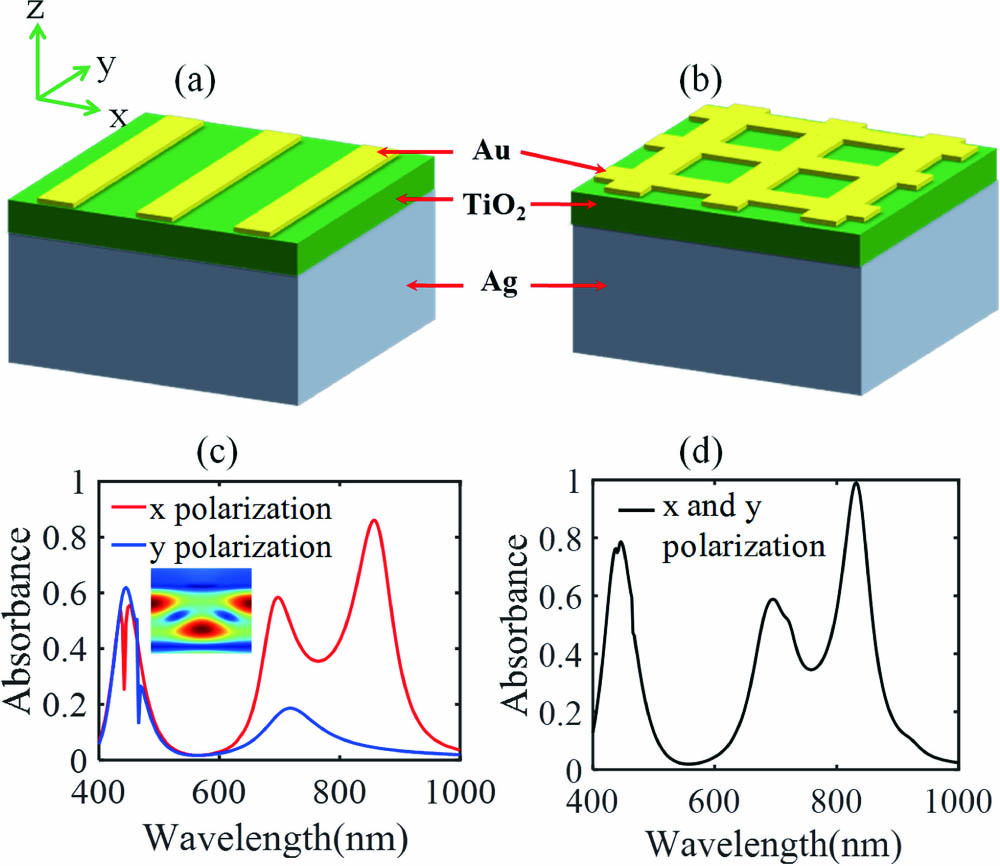
图 4. 甲烷和空气混合气体层流同轴扩散火焰的模拟。(a)火焰模拟的计算网格和边界条件;(b)温度分布;(c) CO2浓度分布
Fig. 4. Numerical simulation of CH4-air laminar coaxial diffusion flame. (a) Computation grid and boundary conditions for simulating flame; (b) temperature field; (c) CO2 concentration field
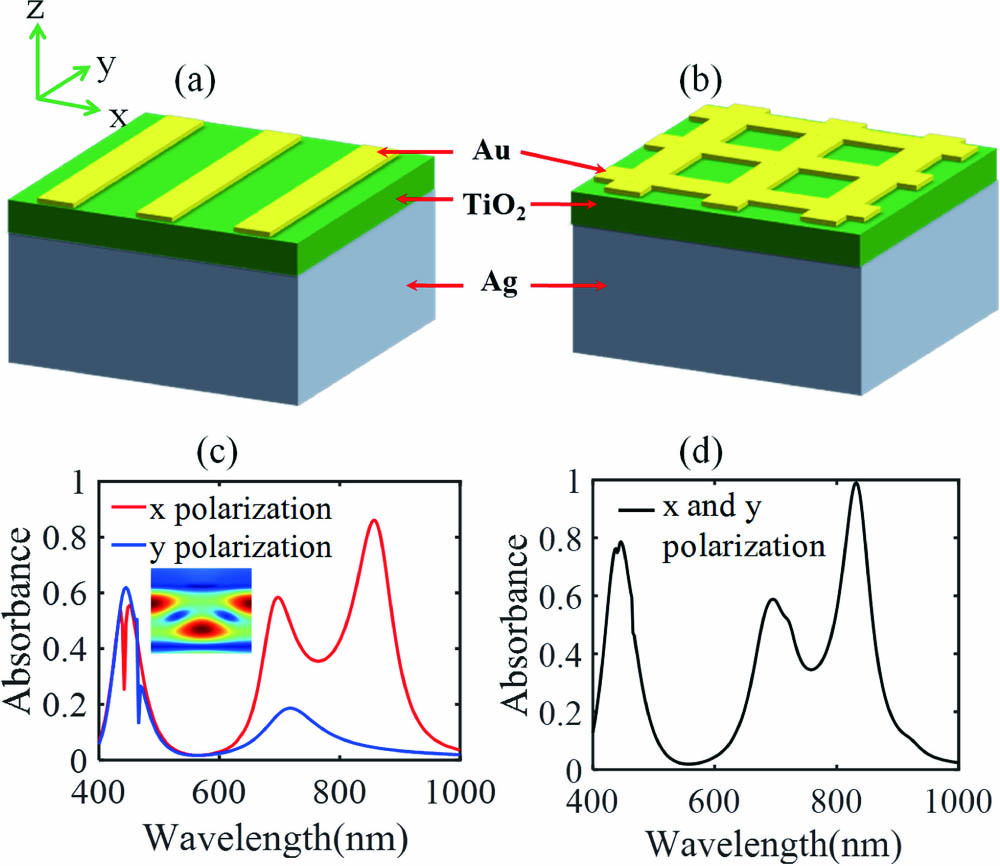
图 5. 火焰温度场和CO2浓度场的真实分布以及加入2%、5%和10%高斯随机噪声后机器学习模型的预测结果。(a)温度场; (b) CO2浓度场
Fig. 5. True flame temperature and CO2 concentration fields, as well as machine-learning-based model predicted fields with 2%, 5% and 10% Gaussian random noises. (a) Temperature fields; (b) CO2 concentration fields
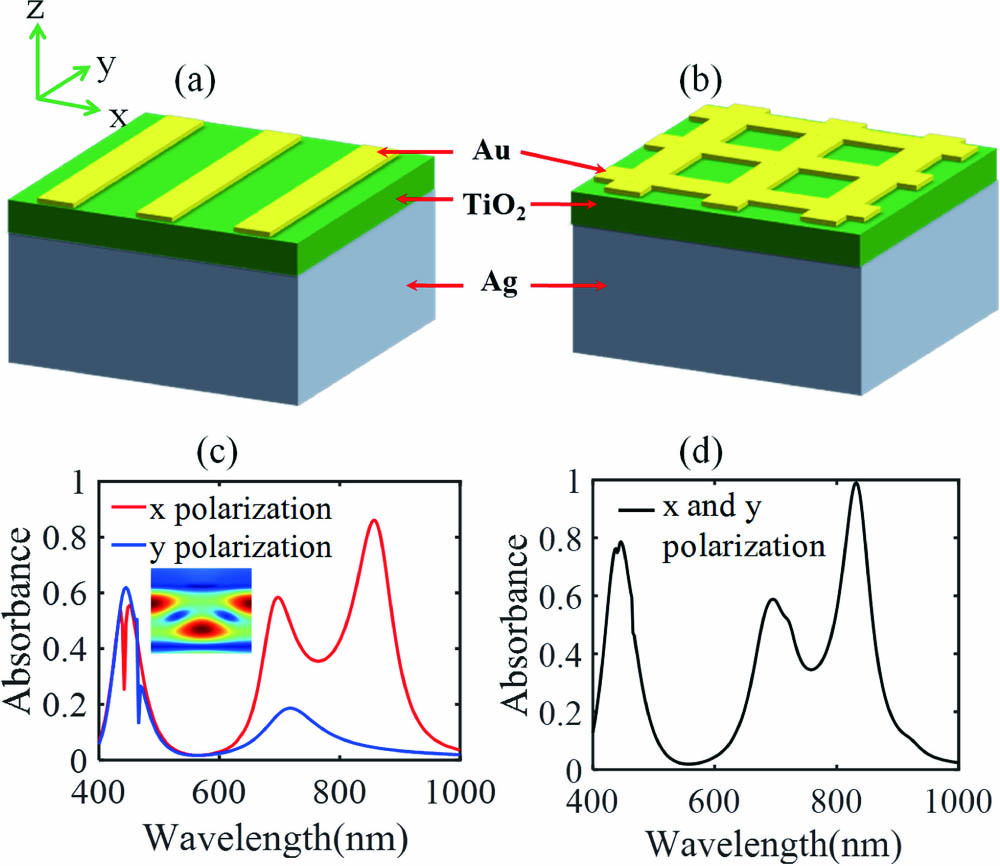
图 6. 真实的火焰温度场和CO2浓度场以及不同随机噪声下机器学习模型得到的预测结果的对比。(a) 2% 随机噪声;(b) 5% 随机噪声;(c) 10% 随机噪声
Fig. 6. Comparisons of true temperature and CO2 concentration fields (ideal) with machine-learning-based model predicted ones (MLP) with different random noises. (a) 2% random noise; (b) 5% random noise; (c) 10% random noise
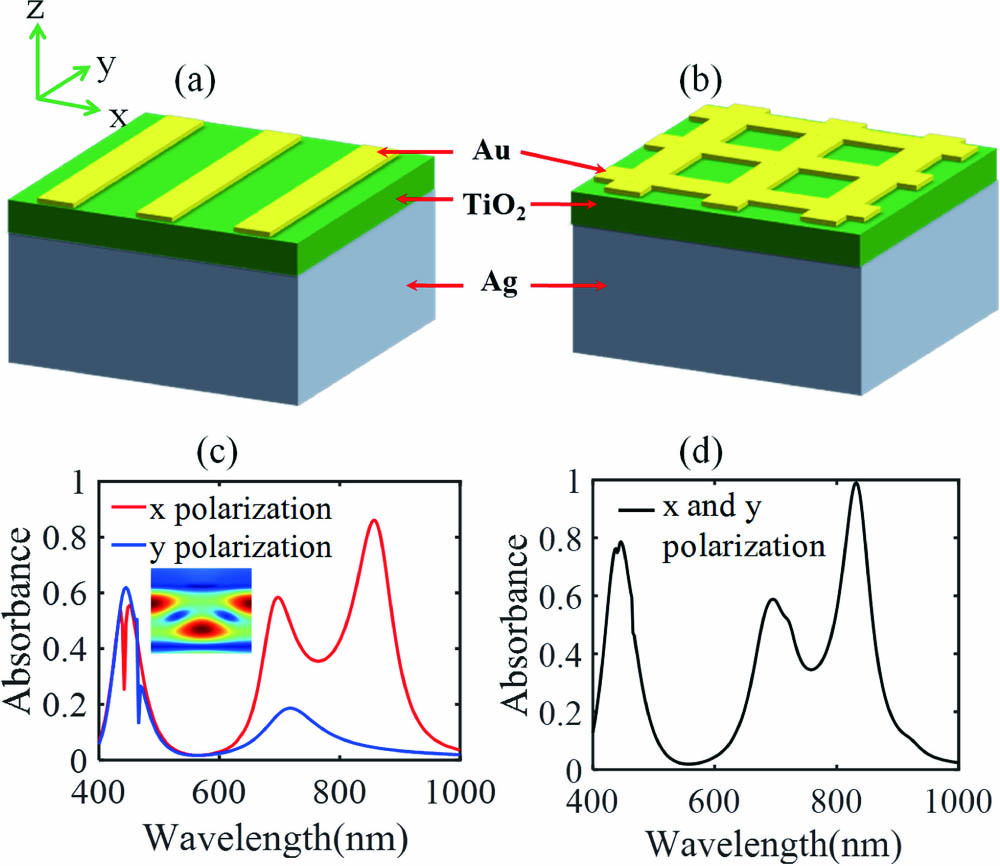
图 7. 火焰高度为40 mm处真实温度和CO2浓度的径向分布与机器学习模型得到的预测值之间的对比。(a) 2% 随机噪声;(b) 5% 随机噪声;(c) 10% 随机噪声
Fig. 7. Comparisons of true temperature and CO2 concentration distributions (ideal) at the height above burner of 40 mm with machine-learning-based model predicted ones (MLP). (a) 2% random noise; (b) 5% random noise; (c) 10% random noise
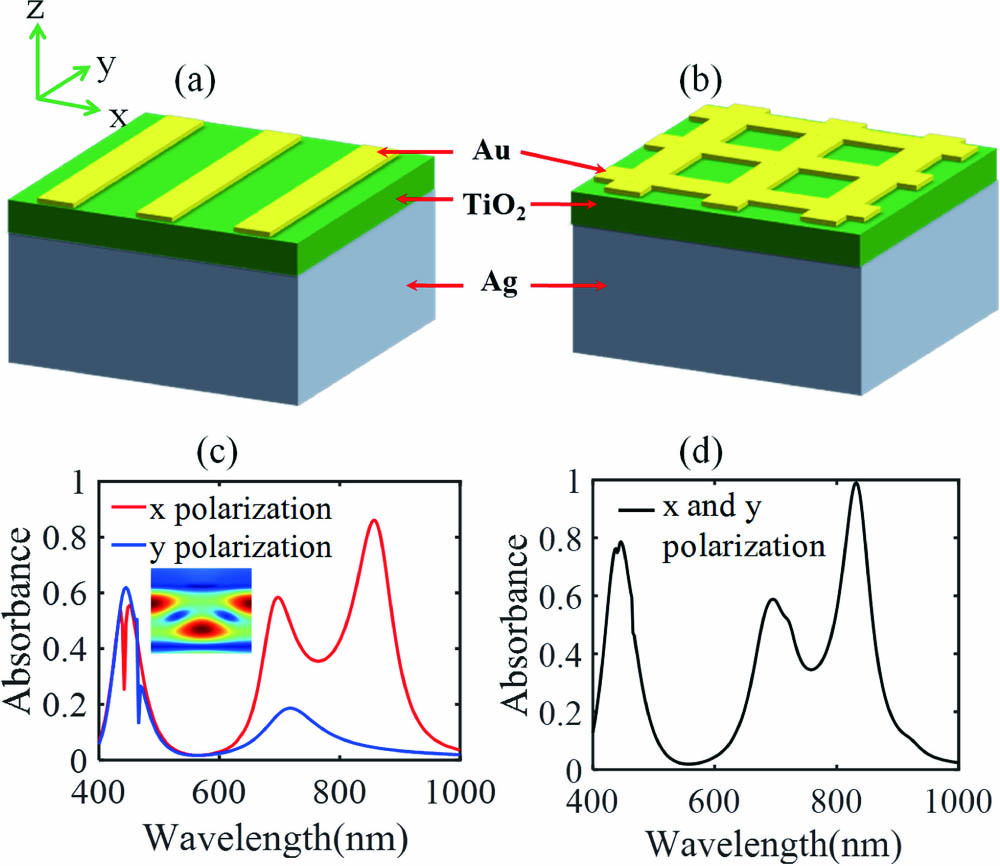
张倚成, 韩永康, 周亚, 任涛, 刘训臣. 基于机器学习对火焰温度场和CO2浓度场的同步重建[J]. 光学学报, 2020, 40(23): 2312003. Yicheng Zhang, Yongkang Han, Ya Zhou, Tao Ren, Xunchen Liu. Machine-Learning-Based Reconstruction of Flame Temperature and CO2 Concentration Fields[J]. Acta Optica Sinica, 2020, 40(23): 2312003.