基于注意力机制的自适应光学开环斜率预测【增强内容出版】
Adaptive optics (AO) are commonly utilized for real-time wavefront detection and aberration correction. Nevertheless, a typical AO system often experiences a time delay equivalent to at least 2?3 sampling periods due to wavefront sensor readout data delay and control calculation delay. Hence, existing AO systems do not offer truly real-time corrections. When the frequency of turbulence alterations surpasses the closed-loop bandwidth of the AO system, this delay leads to the compensation wavefront on the deformable mirror (DM) trailing the changes in the distortion wavefront. This lag substantially hampers the correction efficacy of the AO technology. Consequently, predicting future atmospheric turbulence information in real-time bears significant research importance and practical value in AO technology.
In this paper, a spatiotemporal prediction network was proposed for AO wavefronts based on the attention mechanism. This network simultaneously considered the temporal and spatial characteristics of atmospheric turbulence and selected the slope data, which was easier to acquire in the AO system and had a lower dimension, as both the input and output of the network. With only six frames of previous wavefront slope information, the wavefront slope of the second frame at a subsequent moment was determined. The network initially employed the spatial attention mechanism to capture similar target features in each frame of the distorted wavefront. It then utilized the residual learning strategy to remove redundant information between the input wavefronts of two consecutive frames, producing refined target features. Additionally, recognizing that the degree of target feature information in each frame of the distorted wavefront varied, the channel attention mechanism was further employed. This mechanism emphasized distorted wavefronts containing a richer set of target feature values rather than evenly weighting the wavefront from each frame. Following these steps, the final prediction of the wavefront slope was realized.
The generalizability of the distorted wavefront dataset is tested under different atmospheric turbulence intensities. As the turbulence intensity increases, the structural similarity (SSIM) between the predicted and true wavefronts stabilizes above 0.9300, and the ratio of the root-mean-square wavefront error (RMSe) between the predicted and true wavefronts to the true RMS is stable at approximately 5.00% (Table 2). In the two-frame delay system, when compared to the non-prediction method, the performance improvement of the proposed prediction method is stable at approximately 40% (Fig. 4). Extended tests are performed on the three-frame-delay AO system under the assumption that the RMSe increases when compared with the two-frame delay prediction; however, the performance of the proposed prediction method increases by more than 43% when compared with that of the non-predicted method (Fig. 5). Additionally, a set of ablation comparison experiments are conducted. Under the assumption of a two-frame delay system, compared to the non-prediction method, the RMSe based on the attention mechanism and residual learning prediction methods reduce by approximately 29% and 16%, respectively, while that based on the proposed method reduces by approximately 40% (Fig. 7). To further verify the performance of the proposed prediction network, we test the one-time open-loop data collected by an actual 1 km laser atmospheric transmission system (Table 3); the average ground truth RMS of the experimental data is 0.9488λ, the average prediction RMS is approximately 0.9557λ, the average RMSe is approximately 0.0675λ, and the RMSe is approximately 7.1% of the real true RMS (Fig. 9). Furthermore, to demonstrate the prediction capability of the network for the experimental data, we extend the simulation of the three-frame delay open-loop correction process again. Compared to the non-predicted method, in two-frame delay and three-frame delay systems, the average performance improvements of the proposed prediction method are 41.2% and 42.9%, respectively (Fig. 10). This reiterates the effectiveness of the proposed open-loop slope prediction network and the feasibility of applying this network model to open-loop correction systems.
In this study, an AO open-loop slope-prediction-network-based attention mechanism is proposed to achieve high-precision wavefront slope prediction. The effectiveness of the network is experimentally proven, which can effectively overcome the inherent delay problem of real AO systems. Additionally, the network uses only six consecutive frames of wavefront slope prior information to achieve a high-precision actual wavefront for correction. This input and output configuration reduces the hardware system's load capacity and enhances the network model's running speed, offering crucial guidance and application value for the subsequent deployment of an actual AO delay system.
1 引言
自适应光学(AO)技术已被广泛应用于波前的实时探测与像差校正[1-4],然而,实际的AO系统由于波前传感器(SHWS)读出数据延迟和控制计算延迟等,通常存在至少2~3个采样周期的时延[5-7]。因此,目前的AO系统本质上并不是一个真正的实时校正系统。当湍流变化的时间频率大于AO系统的闭环带宽时,系统的固有时延将严重影响其校正性能[8-9]。虽然闭环带宽可以通过增加探测器的采样频率得以提升,但这同时带来了弱光条件下难以适应以及系统硬件成本增大的问题。如果能够根据过去和当前的湍流分布来预测未来的湍流分布,并将其转化为变形镜(DM)的校正量,就可以有效抵消AO系统的时延误差,在相同的采样频率下提升系统的校正性能。
目前,AO系统的预测校正技术已有大量的公开报道,其中最为经典的迭代预测方法,如递归最小二乘(RLS)控制算法[10-13],对系统误差进行最小化处理,具有计算过程简单、不容易陷入局部最小点的优点,但当像差的模式阶数较高时无法在短时间内收敛。线性预测方法,如基于卡尔曼滤波的线性二次高斯(LQG)控制算法[14-19],通过大气湍流模型的先验知识来预测波前像差的变化,具有较好的预测效果,但其依赖于湍流的线性空间状态和DM的动力学分布,这需要非常精确的校准模型参数。
神经网络类方法因具有较强的非线性拟合能力、抗干扰鲁棒性和泛化能力,在AO预测校正中展现出了独特优势[20-22]。基于神经网络的预测方法最早由Jorgenson于1992年引入AO系统[20],结果显示,引入预测可将波前校正误差降为原来的1/7左右。但是包括文献[23-26]在内的这些基于多个全连接层构建的预测模型与线性预测模型类似,只关注了波前的空间特征,同样难以适应不断变化的大气湍流。2020年,Liu等[27]着重考虑了大气湍流在时间域上的非平稳特性,利用两个基于长短期记忆(LSTM)单元的预测模型对不同风速和风向下的开环波前进行了性能测试。但是大气湍流的时空特征是物理耦合的,在预测过程中不能分离,由某个独立特征建立的网络结构会导致最终预测结果不准确且不稳定[28]。为此,笔者提出了一种基于注意力机制的AO开环斜率预测网络。该网络利用空间注意力机制关注每一帧畸变波前中的相似目标特征,采用残差学习模块消除相邻两帧输入波前之间的冗余信息,以此来获得时间域上更加精细的相似结构特征。在具有两帧延迟的AO系统仿真中,本文所提预测方法使波前校正残差均方根(RMSe)下降了约40%,并且在不同的大气湍流强度下均表现出稳定的预测精度,预测RMSe仅为真实畸变波前均方根(RMS)的5.00%。最后,使用1 km激光大气传输系统采集的实验数据进行了测试,验证了开环斜率预测网络的有效性。
2 基本原理
在传统的无预测AO系统中,DM通过加载历史波前信息来补偿当前的大气湍流。为进一步提高AO系统的实时校正能力,笔者提出了一种神经网络类方法,用其进行未来波前斜率的预测。其原理如
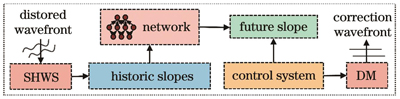
图 1. 基于深度神经网络的AO预测与校正模型原理图
Fig. 1. Schematic diagram of forward prediction and correction model of AO system based on deep neural network
首先,AO系统利用SHWS探测大气湍流的波前像差;然后,深度神经网络通过将历史波前斜率数据作为输入来预测未来时刻的波前像差,波前控制器将预测后的波前斜率转换为DM控制电压,DM对时延后的畸变波前斜率进行实时校正。具体来说,为了适应绝大多数的两帧延迟系统,根据开环条件下SHWS探测的连续6帧历史波前斜率序列预测未来时刻第2帧(即第8帧)的未校正波前斜率。
2.1 大气湍流模拟
大气冻结湍流假设是一种被广泛采用的针对大气湍流的建模方法[29-32]。在仿真中,笔者模拟了一个基于Kolmogorov湍流统计理论[33]的1 km近地面激光水平大气传输观测系统,并根据HV-57折射率结构常数模型模拟了5组不同湍流强度的大气湍流训练数据集,以提高网络的泛化能力。实验测量结果显示实验系统所在地区全年在同一高度下的折射率结构常数(
表 1. 大气湍流模拟参数设置
Table 1. Simulation parameter setting of atmospheric turbulent
|
不同的
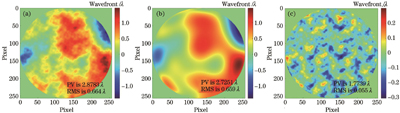
图 2. 大气模拟畸变波前、重构波前以及它们的残余波前。(a)模拟波前;(b)重构波前;(c)残余波前
Fig. 2. Atomspheric simulation distorted wavefront, reconstructed wavefront and their residual wavefront. (a) Simulated wavefront; (b) reconstructed wavefront; (c) residual wavefront
2.2 网络模型设置
笔者提出了一种基于注意力机制和残差学习的预测网络。该网络首先采用空间注意力机制获得每一帧畸变波前中相似的目标特征,随后利用残差学习策略消除相邻两帧输入波前之间的冗余信息,得到精化后的目标特征。考虑到每帧畸变波前中包含目标特征信息的程度不同,继续使用通道注意力机制重点关注那些包含有更多目标特征价值的畸变波前,而不是同等地对待来自每一帧波前的特征。经过上述处理后,输出波前斜率预测结果。所提预测网络的结构示意图如

图 3. 结合注意力机制和残差学习的时空预测网络。(a)预测网络的整体构架;(b)预测网络的详细结构
Fig. 3. Spatial-temporal prediction network combining attention mechanism and residual learning. (a) Overall structure of the forecast network; (b) detailed structure of the forecast network
网络模型的具体预测步骤如下:
1)特征提取。首先通过多个卷积层进行下采样,提取连续输入波前斜率特征,如
2)获得空间注意力机制权重特征波前。分别通过两个1×1和3×3卷积层提取连续畸变波前斜率中的相似目标特征,赋予每帧波前中每个元素不同的权重,从而产生一个权重特征波前,然后通过单层1×1卷积和归一化指数函数(Softmax 函数)得到空间注意力图As。As分别与6帧连续的畸变波前斜率
3)残差学习。
4)获得通道注意力机制权重特征波前。通过通道注意力机制关注具有更多目标特征的连续波前,赋予每个通道不同的权重,将精化后的特征波前
5)预测结果。将Ac分别与
本文网络模型采用L1损失函数来最小化真实值与预测值之间的所有绝对值误差之和,即
式中:
3 仿真结果分析
通过验证集选取最优预测模型之后,采用两组测试来验证所提网络的预测能力。在以下所有测试中,为了对预测模型进行充分验证,选取了1000组数据进行测试,并取其平均值作为仿真结果进行展示。
在第一组测试中,利用从未被网络训练过的不同
3.1 泛化能力分析
在第一组测试中,测试集的大气湍流畸变波前数据虽然不是训练数据集的一部分,从未被网络识别过,而且与训练数据分布相隔2000帧,但每组测试集的风矢量等参数都与同一大气湍流强度下的训练数据保持一致。因此,在这种情况下,预测器有望达到其最佳性能。如
表 2. 不同 下平均真值RMS、平均预测RMS、平均RMSe及SSIM
Table 2. Average truth RMS, average prediction RMS, average RMSe and SSIM under different values
|
为了更直观地证明所提出网络的预测能力,进行了开环校正过程模拟。其中两帧和三帧延迟系统中的非预测方法是指直接利用历史波前相位补偿当前波前相位。例如,在两帧延迟系统中,用t-2时刻的波前补偿t时刻的畸变波前,这也是目前实际AO系统中最常用的补偿方式。本文所提预测方法是指用预测出的波前直接补偿t时刻的畸变波前。
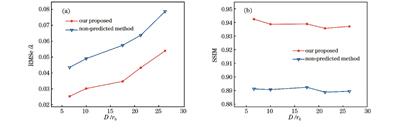
图 4. 本文所提预测方法与非预测方法在两帧延迟系统中不同 下的性能对比。(a)RMSe对比;(b)SSIM对比
Fig. 4. Comparison of the performance of our proposed prediction method and non-predicted method in two-frame delay system under different values. (a) RMS wavefront error (RMSe) comparison; (b) SSIM comparison
除此之外,为了证明本文所提预测方法的有效性,在三帧延迟AO系统假设下进行了测试。

图 5. 本文所提预测方法在两帧和三帧延迟系统下的预测性能
Fig. 5. Prediction performance of our proposed prediction method under two-frame and three-frame delay systems
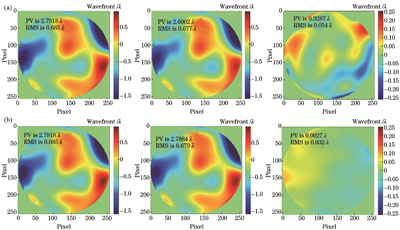
图 6. 两帧延迟系统中不同方法对随机一帧真实畸变波前的开环校正结果对比。(a)真实波前、非预测波前以及它们的残差波前;(b)真实波前、预测波前以及它们的残差波前
Fig. 6. Comparison of open-loop correction results of different methods for real distorted wavefront of a random frame in two-frame delay system. (a) True wavefront, non-predicted wavefront and their residual wavefront; (b) true wavefront, predicted wavefront and their residual wavefront
3.2 消融对比实验
在第二组测试中,为了证明所提预测网络模型中两个模块存在的有效性和必要性,进行了一组消融对比实验。其中消融实验分别使用的是只基于注意力机制模块的网络模型以及只基于残差学习模块的网络模型,可以理解为这两个网络模型分别是只重点关注空间特征和只关注时间特征的神经网络模型。为了保证测试结果的准确性,利用与之前训练本文所提预测网络模型完全一致的数据集对这两个网络模型进行同等训练。
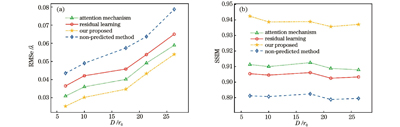
图 7. 所提预测方法与非预测方法及消融实验方法在两帧延迟系统中的性能对比。(a)RMSe的对比;(b)SSIM的对比
Fig. 7. Performance comparison of our proposed prediction method, non-predicted method and ablation experimental methods in two-frame delay systems. (a) RMSe comparison; (b) SSIM comparison
4 实验验证
为了进一步验证所提预测网络的性能,对实际的1 km激光大气传输系统所采集的一次性开环数据进行了测试,如
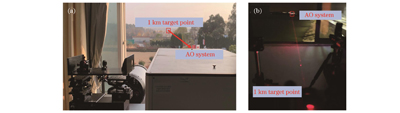
图 8. 1 km激光大气传输系统。(a)接收端(AO系统)视角;(b)发射端(靶点纯合作目标光源)视角
Fig. 8. A 1 km laser atmospheric transmission system. (a) Receiving end (AO system) view; (b) transmitting end (target pure cooperative target light source) view
SHWS实验数据是在D/r0=18.8、采样频率为500 Hz、采集时间为10 s的条件下采集的一组开环斜率数据。
表 3. 1 km激光大气传输系统相关参数
Table 3. Parameters related to 1 km laser atmospheric transmission system
|
为了使预测网络更好地适应实验数据与仿真数据的区别(风速、风向等),一般通过实验数据再训练的方式对网络参数进行微调。在微调过程中,将采集到的哈特曼波前斜率数据直接作为训练集数据,同时预留了1000帧数据作为测试集,不参与训练过程,所以测试集数据没有被提前训练过。
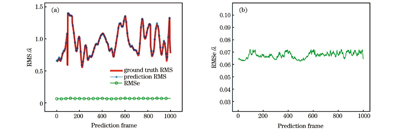
图 9. 1000帧实验数据的测试结果。(a)真实值RMS、预测值RMS和RMSe对比;(b)RMSe的详细分布
Fig. 9. Test results of 1000 frames of experimental data. (a) Comparison of ground truth RMS, prediction RMS and RMSe; (b) detailed RMSe distribution
为了证明网络对实验数据的预测能力,再次模拟了开环校正过程。在两帧延迟和三帧延迟的AO系统假设下进行了性能测试,
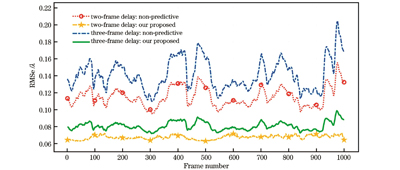
图 10. 所提预测网络在两帧延迟和三帧延迟系统下对实验数据的预测性能
Fig. 10. Prediction performance of our proposed prediction network for experimental data under two-frame and three-frame delay systems
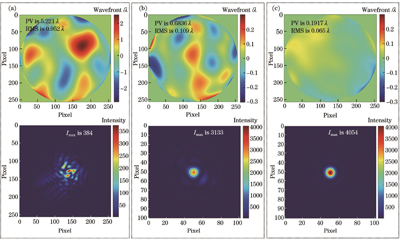
图 11. 实验数据集中随机一帧畸变波前及其开环校正结果。(a)真实的畸变波前及其远场;(b)非预测方法的开环校正残差波前及其远场;(c)所提预测网络的开环校正残差波前及其远场
Fig. 11. A random frame distorted wavefront and its open-loop correction results in the experimental data set. (a) True distorted wavefront and its far-field; (b) open-loop correction of residual wavefront and its far-field under the non-predicted method; (c) open-loop correction of residual wavefront and its far-field under our predicted network
5 结论
本文提出了一种考虑了大气时空耦合特性的开环波前斜率预测网络。该网络结合了注意力机制模块与残差学习模块的优势,可以通过仅6帧先验连续畸变波前斜率数据预测输出未来时刻第2帧(即第8帧)的高精度待校正大气湍流畸变波前。本文所提预测网络在不同大气湍流强度下的预测RMSe与真实畸变波前RMS的比值均稳定在5.00%左右,表现出了较高的预测精度和泛化能力。在两帧延迟系统中,与非预测方法相比,所提预测网络的RMSe值减小了40%左右。最后,针对1 km激光大气传输系统所采集的开环实验数据进行测试,进一步验证了所提预测网络的有效性。
[1] Gavel D T. Adaptive optics control strategies for extremely large telescopes[J]. Proceedings of SPIE, 2002, 4494: 215-220.
[2] 姜文汉. 自适应光学发展综述[J]. 光电工程, 2018, 45(3): 170489.
[4] 孔令曦, 程涛, 苏春轩, 等. 基于滤波型线性自抗扰预估的倾斜镜控制方法[J]. 中国激光, 2023, 50(13): 1305001.
[5] Fried D L. Time-delay-induced mean-square error in adaptive optics[J]. Journal of the Optical Society of America A, 1990, 7(7): 1224-1225.
[6] Sivo G, Kulcsár C, Conan J M, et al. First on-sky SCAO validation of full LQG control with vibration mitigation on the CANARY pathfinder[J]. Optics Express, 2014, 22(19): 23565-23591.
[7] Kulcsár C, Raynaud H F, Petit C, et al. Minimum variance prediction and control for adaptive optics[J]. Automatica, 2012, 48(9): 1939-1954.
[8] 李新阳, 姜文汉. 自适应光学控制系统的有效带宽分析[J]. 光学学报, 1997, 17(12): 1697-1702.
[9] 李新阳, 姜文汉. 自适应光学系统的控制残余方差分析[J]. 光学学报, 2000, 20(10): 1328-1334.
[10] PageK, SchöckM. Analysis of atmospheric turbulence with applications to linear predictions[C]∥2002 European Southern Observatory Conference and Workshop Proceedings. Garchingi, Germany: European Southern Observatory, 2002, 58: 367-372.
[11] 颜召军, 李新阳, 饶长辉. 一种自适应光学闭环系统预测控制算法的仿真研究[J]. 光学学报, 2011, 31(1): 0101003.
[12] BeghiA, CenedeseA, MasieroA. Atmospheric turbulence prediction: a PCA approach[C]∥2007 46th IEEE Conference on Decision and Control, December 12-14, 2007, New Orleans, LA, USA. New York: IEEE Press, 2008: 566-571.
[13] Fraanje R, Doelman N. Modeling and prediction of turbulence-induced wavefront distortions[J]. Proceedings of SPIE, 2010, 7736: 773617.
[14] Correia C, Raynaud H F, Kulcsár C, et al. On the optimal reconstruction and control of adaptive optical systems with mirror dynamics[J]. Journal of the Optical Society of America A, 2010, 27(2): 333-349.
[15] WibergD M, MaxC E, GavelD T. A spatial non-dynamic LQG controller: part I, application to adaptive optics[C]∥2004 43rd IEEE Conference on Decision and Control (CDC) (IEEE Cat. No.04CH37601), December 14-17, 2004, Nassau, Bahamas. New York: IEEE Press, 2008: 3326-3332.
[16] WibergD M, MaxC E, GavelD T. A spatial non-dynamic LQG controller: part II, theory[C]∥2004 43rd IEEE Conference on Decision and Control (CDC) (IEEE Cat. No.04CH37601), December 14-17, 2004, Nassau, Bahamas. New York: IEEE Press, 2005: 3333-3338.
[17] Looze D P. Linear-quadratic-Gaussian control for adaptive optics systems using a hybrid model[J]. Journal of the Optical Society of America A, 2008, 26(1): 1-9.
[18] Petit C, Quiros-Pacheco F, Conan J M, et al. Kalman-filter-based control for adaptive optics[J]. Proceedings of SPIE, 2004, 5490: 1414-1425.
[19] Gray M, Petit C, Rodionov S, et al. Local ensemble transform Kalman filter, a fast non-stationary control law for adaptive optics on ELTs: theoretical aspects and first simulation results[J]. Optics Express, 2014, 22(17): 20894-20913.
[20] Wong A P, Norris B R M, Tuthill P G, et al. Predictive control for adaptive optics using neural networks[J]. Journal of Astronomical Telescopes, Instruments, and Systems, 2021, 7(1): 019001.
[21] 胡逸雯, 刘鑫, 匡翠方, 等. 基于深度学习的自适应光学技术研究进展及展望[J]. 中国激光, 2023, 50(11): 1101009.
[22] 程涛, 郭思成, 王宁, 等. 基于机器学习的激光自适应光学技术研究进展[J]. 中国激光, 2023, 50(11): 1101008.
[23] Jorgenson M B, Aitken G J M. Prediction of atmospherically induced wave-front degradations[J]. Optics Letters, 1992, 17(7): 466-468.
[24] 颜召军, 李新阳. 基于神经网络的自适应光学系统变形镜控制电压预测方法[J]. 光学学报, 2010, 30(4): 911-916.
[27] Liu X W, Morris T, Saunter C, et al. Wavefront prediction using artificial neural networks for open-loop adaptive optics[J]. Monthly Notices of the Royal Astronomical Society, 2020, 496(1): 456-464.
[28] Chen J G, Shah V, Liu L L. Performance of a U-Net-based neural network for predictive adaptive optics[J]. Optics Letters, 2021, 46(10): 2513-2516.
[29] Schöck M, Spillar E J. Method for a quantitative investigation of the frozen flow hypothesis[J]. Journal of the Optical Society of America A, 2000, 17(9): 1650-1658.
[30] Poyneer L, van Dam M, Véran J P. Experimental verification of the frozen flow atmospheric turbulence assumption with use of astronomical adaptive optics telemetry[J]. Journal of the Optical Society of America A, 2009, 26(4): 833-846.
[31] Gendron E, Léna P. Single layer atmospheric turbulence demonstrated by adaptive optics observations[J]. Astrophysics and Space Science, 1996, 239(2): 221-228.
[32] Kern B, Laurence T A, Martin C, et al. Temporal coherence of individual turbulent patterns in atmospheric seeing[J]. Applied Optics, 2000, 39(27): 4879-4885.
[33] Kolmogorov A N. The local structure of turbulence in incompressible viscous fluid for very large Reynolds numbers[J]. Proceedings of the Royal Society of London Series A: Mathematical and Physical Sciences, 1991, 434(1890): 9-13.
[34] Wang N, Zhu L C, Ma S, et al. Deep learning-based prediction algorithm on atmospheric turbulence-induced wavefront for adaptive optics[J]. IEEE Photonics Journal, 2022, 14(5): 8554310.
[35] HeK M, ZhangX Y, RenS Q, et al. Delving deep into rectifiers: surpassing human-level performance on ImageNet classification[C]∥2015 IEEE International Conference on Computer Vision (ICCV), December 7-13, 2015, Santiago, Chile. New York: IEEE Press, 2016: 1026-1034.
王宁, 朱里程, 葛欣兰, 高泽宇, 王帅, 杨平. 基于注意力机制的自适应光学开环斜率预测[J]. 中国激光, 2024, 51(6): 0605001. Ning Wang, Licheng Zhu, Xinlan Ge, Zeyu Gao, Shuai Wang, Ping Yang. Open-Loop Slope Prediction in Adaptive Optics Based on Attention Mechanism[J]. Chinese Journal of Lasers, 2024, 51(6): 0605001.