激光选区熔化成形316L不锈钢下倾斜面粗糙度的预测与模型建立【增强内容出版】
Selective laser melting (SLM) is a metal-additive 3D printing technology based on layer-by-layer printing. It is one of the most promising industrial additive manufacturing technologies for manufacturing metal components. SLM has the advantages of a high degree of freedom and high material utilization; however, the available surface roughness limits the industrial implementation of this technology. Surface roughness refers to the unevenness of the machined part surface with small spacing, small peaks, and valleys: the distance between two peaks or valleys is very small and belongs to the microgeometric shape error. The Ra value of the parts prepared via SLM is usually between 10 and 30 μm; surface roughness is an important indicator of surface quality, and good surface roughness is widely needed to avoid premature failure caused by surface-induced cracks. Poor surface quality not only reduces the strength, wear resistance, and corrosion resistance of the parts, but also affects the accuracy of corresponding processed parts. Excessive surface roughness can directly lead to an inability to meet assembly conditions, usually requiring surface post-treatment, which takes a significant amount of time and operational cost accumulation, reducing the flexibility advantages of SLM. Therefore, optimization of the manufacturing processes and improving the surface quality of metal parts prepared via SLM are crucial.
The main factors that affect surface roughness were identified, their influencing mechanisms were understood, the roughness influencing factors that needed to be modeled were determined, and the step effect, powder adhesion, and warping deformation were comprehensively considered to establish a prediction model. Based on the processing principle of the SLM technology, a mathematical model was established for the step effect of the downward-inclined surface, determining the angle range without support, and improving the traditional roughness prediction model. To understand the influence of powder adhesion and warping deformation on the surface roughness, a schematic of powder adhesion and warping deformation was drawn, and the influence functions of powder adhesion and warping deformation on the surface roughness were calculated. A mathematical expression was further integrated to establish a downward-inclined surface roughness prediction model. The predicted values were compared with measured data, the accuracy of the model was verified, and the errors were analyzed.
The step effect model adopts a method combined with the morphology of the melt channel, replacing the step effect function with the physical quantity of "maximum height difference between peaks and valleys" (Fig. 4). The powder adhesion model adopts an equivalent method to reduce the three-dimensional surface to a two-dimensional surface, and obtains a mathematical model of powder adhesion using the one-dimensional calculation formula for Ra (Fig. 5). The warping deformation is physically represented by the "correction angle" (Fig. 6), which is combined with the step effect model and the powder adhesion model to obtain a mathematical model for predicting the roughness of the entire downward-inclined surface. Comparing the measured data with the predicted data, in the first group, c=11.2 μm was calculated, while the second group of measured data verified the accuracy of the prediction model.
The step effect model optimizes the right-angle edge into a curved edge, calculates the "maximum height difference between the peaks and valleys," and obtains the step effect function. Powder adhesion, as an essential modeling factor, is calculated using the "equivalent transformation" method, which converts 3D to 2D. The metal powder particles that adhered to the surface were equivalent to a rectangular body next to the edge of the step, with a square cross-section of side length c. The uncertainty of side length c was considered to represent different degrees of powder adhesion. The first set of measurement data was used to fit and compare c, and the minimum average error was used as the standard to determine the value of c. The warping deformation was considered as a change in the inclination angle and the overhanging part as a cantilever beam with a constantly changing cross-section. Using the classical Euler-Bernoulli beam theory, the axial and bending deformations of the highest point in the overhanging area were calculated, its shape deviation was predicted, and expressed in the form of a "corrected angle." The comparison analysis between the predicted roughness values of the downward-inclined surface and the roughness measurement data shows that the prediction model can accurately predict the roughness of the downward-inclined surface, and the significant errors generated are due to two reasons: the maximum height difference between peaks and valleys and powder adhesion.
1 引言
激光选区熔化(SLM)技术是一种基于“离散-堆积”思想的金属增材3D打印技术[1-4]。该技术以激光作为热源,按照三维切片软件中规划好的扫描路径在金属粉末床上进行逐层扫描,扫描路径上的金属粉末在激光能量的输入下快速熔化并凝固,实现所设计的金属零件的成形[5-7]。相比于其他制造方法,SLM技术具有设计自由度极高、生产周期短、材料利用率相对较高等优点,适用于航空航天复杂构件的高精制造[8-11]。
SLM技术在航空航天领域主要用于中小型复杂结构件的制造,在一体化复杂内流道结构、小型燃气涡轮发动机和轻量化结构等方面的应用较多[12-13]。澳大利亚莫纳什大学[14]采用SLM技术制造了世界上第一台燃气轮机发动机,并为其他公司打印了涡轮发动机零件。GE公司[15]采用SLM技术打印LEAP发动机燃油喷嘴(由20个组件整合成一个整体结构),实现了发动机燃油喷嘴的一体化设计与制造。西门子[16]公司采用SLM技术制备了多晶镍基高温合金涡轮叶片,其复杂的内部结构使得热传递和冲击冷却效果达到最好。NASA的研究人员采用SLM技术打印了首个全尺寸铜合金火箭发动机,制造速率相比传统工艺提高了10倍而成本却降低50%以上。Shi等[17]研究了重载航空航天支架,在考虑机械力和温度负荷的条件下通过拓扑优化使SLM增材制造零件的质量减轻了18%以上。Tomlin等通过拓扑优化使Ti6Al4V A320机舱铰链支架减重64%(质量仅为326 g)[18]。但是,SLM成形件表面过于粗糙,不能满足装配要求。针对这一问题,国内外研究人员对SLM零件表面粗糙度的预测进行了探讨。Hertlein等[19]采用一种连续域的贝叶斯网络模型来预测零件的质量特征(密度、硬度、表面粗糙度、表层表面粗糙度),该模型以激光功率、扫描速度、扫描间距、切片厚度作为输入,网络以概率分布的形式对不同倾斜角度表面的粗糙度进行预测。Ahn等[20]认为可以通过预先预测表面粗糙度来实现合理的工艺规划,他们研究了表面粗糙度分布的理论和实际特征,以反映预测的实际粗糙度分布,并利用实测的粗糙度数据和插值方法,得到了可以计算所有表面角度下表面粗糙度值的粗糙度分布表达式。Feng等[21]研究了悬垂区域表面粗糙度演变的机制,研究结果表明:悬垂面的表面粗糙度受边缘实际轮廓轨迹、粉末粘附、翘曲变形和浮渣的综合影响。基于此,他们建立了与倾斜角有关的表面粗糙度理论模型。Strano等[22]研究了SLM制造的316L不锈钢零件的表面粗糙度和形貌,基于表面轮廓仪的测量结果和扫描电镜(SEM)的显微分析,建立了一个新的预测粗糙度的数学模型,该模型考虑了粘附在表面的粉末颗粒。结果表明,该模型的准确性较高,能够较为准确地预测SLM零件下表面的粗糙度。
综上所述,目前对SLM试样表面形貌及粗糙度预测模型建立方面的研究仍比较单一,缺乏对影响因素的系统把控。鉴于此,笔者利用SLM制备不同倾斜角度的316L不锈钢样件,测量其表面粗糙度,建立了表面粗糙度的数学模型(该模型考虑了台阶效应、粉末粘附、翘曲以及熔道形貌),并对模型预测值与实验测量值进行了对比分析。
2 实验材料和方法
实验材料为316L不锈钢粉末,其粒径分布为正态分布,平均粒径为35.12 μm,球形度为0.94。316L不锈钢粉末的化学成分和粒径正态分布图分别如
表 1. 316L不锈钢粉末的化学成分
Table 1. Chemical composition of 316L stainless steel powder
|
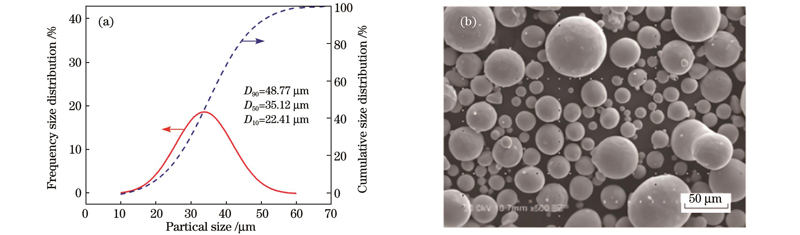
图 1. 316L不锈钢粉末。(a)粒径分布图;(b)SEM微观图像
Fig. 1. 316L stainless steel powder. (a) Particle size distribution; (b) SEM microscopic image
在粗糙度预测模型中,倾斜角度和切片层厚是影响台阶效应和悬垂部分面积的主要因素,因此本次SLM实验选定切片层厚为0.02 mm,将倾斜角度作为变量,其他参数作为固定值(激光功率为220 W,激光扫描速度为960 mm/s,扫描间距为0.08 mm)。利用Materialise Magics 21.0三维建模软件进行建模,利用BLT-S210 3D打印机打印不同倾斜角度(从5°开始,每隔5°递增,一直到90°)的试样,试样尺寸为10 mm×10 mm×2 mm。打印的试样如
3 粗糙度预测模型的建立
3.1 粗糙度影响因素分析
由于切片层厚和倾斜角度的存在,SLM试样会产生具有不同表面形貌的倾斜面,而且上倾斜表面和下倾斜表面也会呈现不同的表面形貌,如
悬垂部分的下方由金属粉末作为支撑,热量不易散失,会产生温度梯度,进而产生热应力,因此每一层的悬垂部分都会发生翘曲变形现象。同时,部分粉末颗粒粘附在台阶边缘,在激光熔化过程中,边缘处的热量不足以完全熔化颗粒,因此粉末颗粒不会与层完全融合,倾向于在台阶边缘处粘附到表面。因此,影响下倾斜表面粗糙度的主要因素有台阶效应、粉末粘附和翘曲变形。
3.2 熔道宽度的确定
无论是上倾斜面还是下倾斜面,在建立熔道搭接模型过程中,都必须进行熔道宽度的计算。对于水平表面来说,粗糙度主要由熔道形貌和熔道搭接情况决定,其中的熔道形貌主要决定于熔道宽度和扫描间距。熔道宽度与激光光斑直径无太大关系,熔道宽度a可由
式中:ξ为粉床对激光的吸收率,ξ=0.35;P为激光功率,P=220 W;k为材料的热导率,k=35.2;Ts为316L不锈钢粉末的熔点,Ts=1670 K;T0为打印前的初始温度,T0=373.15 K;e为自然常数;ρ为材料的密度,ρ=72.835 g/cm³;Cp为材料的比热容,Cp=683.1 J/(kg/K);v为激光扫描速度,v=960 mm/s。从
3.3 台阶效应建模
在SLM激光束照射区域,能量呈高斯分布。金属粉末受到激光作用后熔化并快速凝固。对于下倾斜面来说,台阶效应示意图中的第一层上弧受熔道宽度和切片层厚的限制,可以看作是整个圆弧的一部分;而第一层下弧的圆心到最低点的距离(a/2)代表熔池深度,其值理论上比模型中的深度大,为了便于计算,将其看作是以熔道宽度为直径的圆的面积的一半。多道次激光熔池之间的搭接以及层与层之间的搭接形成了理论截面,如
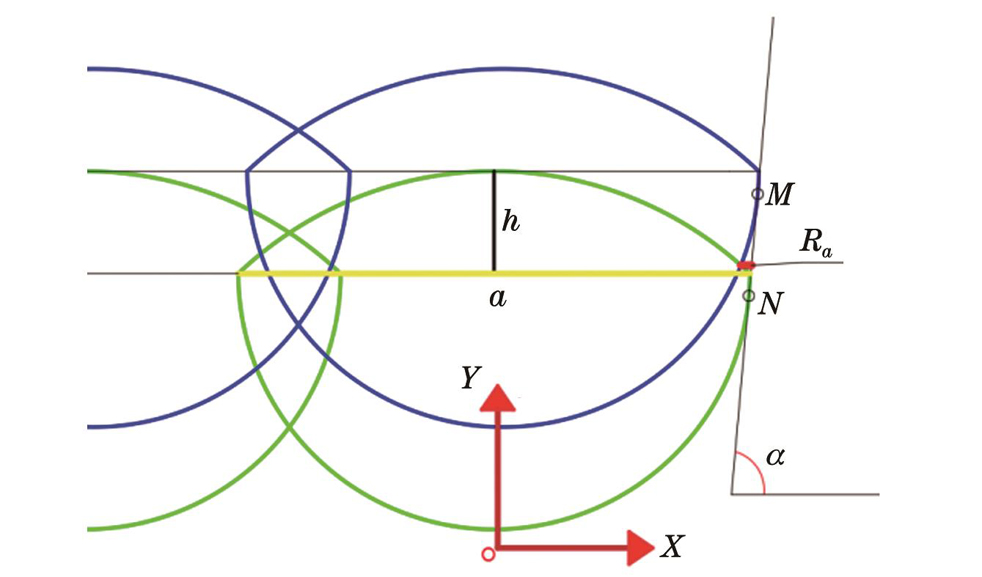
图 4. 下倾斜面台阶效应示意图
Fig. 4. Schematic diagram of the step effect of the downward-inclined surface
对于下倾斜面,台阶效应依然是影响其表面粗糙度的主要因素,不可忽略。在实验过程中,打印倾斜角度为40°的无支撑试样时,由于翘曲变形严重出现了卡刀现象。倾斜角度为45°的试样可以顺利打印,因此,以45°作为有无支撑结构的临界角。本模型只预测了45°到90°的下倾斜面。在
计算相邻两层下弧交点时,为了计算方便,将坐标轴向上平移a/2,求出交点坐标后只要进行坐标变换即可。相邻熔道下弧的曲线方程为
将
将
将
随着倾斜角度增大,交点坐标发生改变,位于第一层上弧线上。同理,可求得第一层上弧与第二层下弧的交点坐标为
式中:h为切片层厚;R1为熔道上弧所在圆的直径。
通过计算可得不同倾斜角度下的切线方程和交点的表达式,如
表 2. 不同倾斜角下切线方程和交点的表达式
Table 2. Tangent equation and intersection expressions under different tilt angles
|
进而可以得到下倾斜面台阶效应影响函数为
3.4 粉末粘附建模
收集的粗糙度实验数据与仅考虑台阶效应的下倾斜面粗糙度预测模型相比,不足以完整地预测不同倾斜角度表面粗糙度的变化规律,还需要考虑粉末粘附对表面的影响。
由于粉末粘附的不规则性,将其直接转化为对粗糙度的影响函数较为困难。本文采用理论等效的思想,将每一层的粘粉情况等效为紧贴台阶的长方体,该长方体贯穿整个台阶。粘粉等效后的主视图为正方形,如
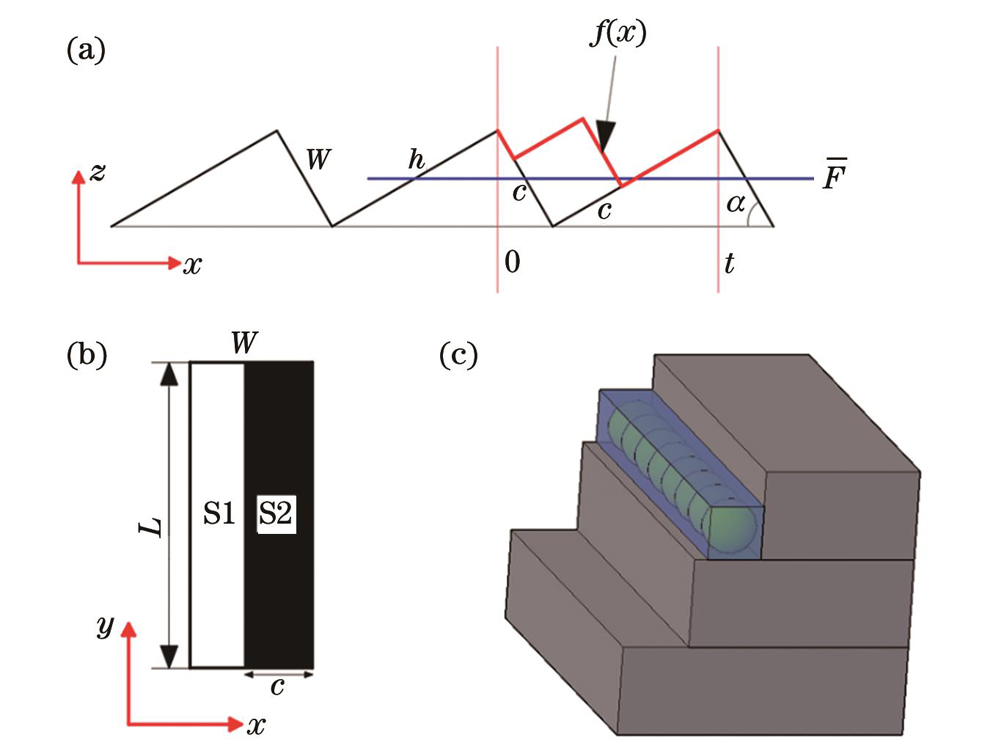
图 5. 等效粘粉示意图。(a)截面图;(b)俯视图;(c)三维图
Fig. 5. Schematic diagrams of equivalent sticky powder. (a) Cross-sectional drawing; (b) top view; (c) three-dimensional drawing
式中:f(x)为一个周期内的轮廓曲线;t为一个周期的长度;
由表面粗糙度的一维定义式可得
计算不同范围内
综上所述,若零件的粉末粘附情况严重,截面正方形的边长c就会很大,根据表面粗糙度的一维定义式求出的数值也会随之变大;反之,若粘粉情况不严重,c的值会较小。可以根据表面粗糙度测量数据来确定c的值,粘粉影响函数值的大小在一定范围内与c成正比例关系。
3.5 翘曲建模
悬垂结构的存在会使最终零件的形状和尺寸精度达不到要求,甚至整个加工过程都不顺利,这是因为发生了翘曲现象。从宏观来看,翘曲现象会使零件下倾斜面的角度发生变化,实际轮廓发生偏移,粘粉位置发生改变。这里,笔者将这种变化归结于角度的改变,并提出了一个新概念——修正角度,该修正角度是翘曲后的角度与设计角度之间的差值。翘曲会导致实际倾斜角度(相对于水平方向)变大,表面更倾向于向垂直方向倾斜。因此,需要求出修正角度的函数表达式。
Kamat等[23]基于Euler-Bernoulli梁弯曲理论提出了一种新的分析模型,用于估计水平打印的内部通道的残余应力引起的变形,如
式中:σx为材料的屈服应力,σx=170 MPa;m为熔体深度与层厚之比,在本模型中,m=1+2h/a,h为铺粉层厚;α为倾斜角;Hj为整个台阶效应的高度;E为材料的弹性模量。
翘曲变形后点p的坐标变为
这样可以得出不同倾斜角度下的翘曲影响函数为
综上所述,下倾斜面的粗糙度预测模型为
式中:
4 模型验证与误差分析
对SLM制备的316L不锈钢下倾斜面粗糙度进行量化建模,得到了表面粗糙度的预测模型。为了验证预测模型的准确性,将实验测量数据与模型预测值进行了比较,如
表 3. 下倾斜面粗糙度的测量数据
Table 3. Measured roughness of downward-inclined surface
|
由
5 结论
对SLM技术制备的316L不锈钢样件的下倾斜面的粗糙度进行了研究,本研究的主要工作如下:
1)确定了下倾斜面粗糙度的影响因素。确定以台阶效应、熔道形貌、粉末粘附和翘曲变形作为建模的影响因素,并将其综合考虑。
2)建立了粗糙度预测模型。对直角边进行优化,将其转化为曲边,计算“峰谷之间最大高度差”,得到了台阶效应函数。粉末粘附是必不可少的建模因素,采用“等效变换”法将三维计算转化为二维计算,将粘附在表面的金属粉末颗粒等效成紧靠台阶边缘的长方体,其截面是边长为c的正方形,以边长c作为不确定量来表示不同程度的粘粉情况。以第一组测量数据对c进行拟合,以最小平均误差作为标准,确定c=11.2 μm。将翘曲变形视为倾斜角度的变化,以新提出的“修正角度”作为翘曲变形的数学模型,将修正后的角度代入所求的台阶效应模型和粉末粘附模型中,完善预测模型。
3)验证了预测模型的准确性。对下倾斜面粗糙度预测值与测量数据进行对比分析,结果表明,该预测模型能够较为准确地预测下倾斜面的粗糙度,产生的较大误差是由“峰谷之间最大高度差”和粉末粘附两方面因素造成的。
[1] Wang D, Liu Y, Yang Y Q, et al. Theoretical and experimental study on surface roughness of 316L stainless steel metal parts obtained through selective laser melting[J]. Rapid Prototyping Journal, 2016, 22(4): 706-716.
[2] 秦艳利, 孙博慧, 张昊, 等. 选区激光熔化铝合金及其复合材料在航空航天领域的研究进展[J]. 中国激光, 2021, 48(14): 1402002.
[3] 宋剑锋, 宋有年, 王文武, 等. 金属粉末选区激光熔化成形表面粗糙度预测及控制方法研究[J]. 中国激光, 2022, 49(2): 0202008.
[4] 蒋浩, 潘涛, 吴文征. 选区激光熔化技术特性及其研究现状[J]. 科学技术创新, 2021(9): 9-12.
Jiang H, Pan T, Wu W Z. Characteristics and research status of selective laser melting technology[J]. Scientific and Technological Innovation, 2021(9): 9-12.
[5] Li R D, Liu J H, Shi Y S, et al. Balling behavior of stainless steel and nickel powder during selective laser melting process[J]. The International Journal of Advanced Manufacturing Technology, 2012, 59(9): 1025-1035.
[6] 穆伟豪, 陈雪辉, 张雨, 等. 316L不锈钢选区激光熔化表面的形貌分析与粗糙度预测[J]. 激光与光电子学进展, 2022, 59(7): 0714011.
[7] Greco S, Gutzeit K, Hotz H, et al. Selective laser melting (SLM) of AISI 316L-impact of laser power, layer thickness, and hatch spacing on roughness, density, and microhardness at constant input energy density[J]. The International Journal of Advanced Manufacturing Technology, 2020, 108(5): 1551-1562.
[8] WeiY P, WuD Z, TerpennyJ. A decision-level data fusion approach to surface roughness prediction[C]∥Proceedings of ASME 2019 14th International Manufacturing Science and Engineering Conference, June 10-14, 2019, Erie, Pennsylvania, USA. New York: ASME Press, 2019.
[9] 李彬, 戴善波, 顾海, 等. SLM成形角度对316L不锈钢成形精度的影响[J]. 应用激光, 2021, 41(3): 454-459.
[10] Boschetto A, Bottini L, Veniali F. Roughness modeling of AlSi10Mg parts fabricated by selective laser melting[J]. Journal of Materials Processing Technology, 2017, 241: 154-163.
[11] Lyukshin V, Shatko D, Strelnikov P. Methods and approaches to the surface roughness assessment[J]. Materials Today: Proceedings, 2021, 38: 1441-1444.
[12] 樊恩想, 刘小欣, 吴欢欢. 激光选区熔化增材制造技术的发展[J]. 机械制造, 2021, 59(8): 45-49.
Fan E X, Liu X X, Wu H H. Development of laser selective melting additive manufacturing technology[J]. Machinery, 2021, 59(8): 45-49.
[13] 王茂松, 杜宇雷. 增材制造钛铝合金研究进展[J]. 航空学报, 2021, 42(7): 625263.
Wang M S, Du Y L. Research progress of additive manufacturing of TiAl alloys[J]. Acta Aeronautica et Astronautica Sinica, 2021, 42(7): 625263.
[14] 杨胶溪, 吴文亮, 王长亮, 等. 激光选区熔化技术在航空航天领域的发展现状及典型应用[J]. 航空材料学报, 2021, 41(2): 1-15.
Yang J X, Wu W L, Wang C L, et al. Development status and typical application of selective laser melting technology applications in aerospace field[J]. Journal of Aeronautical Materials, 2021, 41(2): 1-15.
[15] 杨强, 鲁中良, 黄福享, 等. 激光增材制造技术的研究现状及发展趋势[J]. 航空制造技术, 2016, 59(12): 26-31.
Yang Q, Lu Z L, Huang F X, et al. Research on status and development trend of laser additive manufacturing[J]. Aeronautical Manufacturing Technology, 2016, 59(12): 26-31.
[16] 陶云亚, 薛伟鹏, 唐洪飞, 等. 激光增材制造技术在涡轮叶片中的应用[J]. 燃气涡轮试验与研究, 2016, 29(6): 44-50, 55.
Tao Y Y, Xue W P, Tang H F, et al. Application of laser additive manufacturing technology in turbine blade and vane[J]. Gas Turbine Experiment and Research, 2016, 29(6): 44-50, 55.
[17] Shi G H, Guan C Q, Quan D L, et al. An aerospace bracket designed by thermo-elastic topology optimization and manufactured by additive manufacturing[J]. Chinese Journal of Aeronautics, 2020, 33(4): 1252-1259.
[18] 董鹏, 梁晓康, 赵衍华, 等. 激光增材制造技术在航天构件整体化轻量化制造中的应用现状与展望[J]. 航天制造技术, 2018(1): 7-11.
Dong P, Liang X K, Zhao Y H, et al. Research status of laser additive manufacturing in integrity and lightweight[J]. Aerospace Manufacturing Technology, 2018(1): 7-11.
[19] Hertlein N, Deshpande S, Venugopal V, et al. Prediction of selective laser melting part quality using hybrid Bayesian network[J]. Additive Manufacturing, 2020, 32: 101089.
[20] Ahn D, Kim H, Lee S. Surface roughness prediction using measured data and interpolation in layered manufacturing[J]. Journal of Materials Processing Technology, 2009, 209(2): 664-671.
[21] Feng S C, Kamat A M, Sabooni S, et al. Experimental and numerical investigation of the origin of surface roughness in laser powder bed fused overhang regions[J]. Virtual and Physical Prototyping, 2021, 16(sup1): S66-S84.
[22] Strano G, Hao L, Everson R M, et al. Surface roughness analysis, modelling and prediction in selective laser melting[J]. Journal of Materials Processing Technology, 2013, 213(4): 589-597.
[23] Kamat A M, Pei Y T. An analytical method to predict and compensate for residual stress-induced deformation in overhanging regions of internal channels fabricated using powder bed fusion[J]. Additive Manufacturing, 2019, 29: 100796.
Article Outline
杨光, 程凯博, 赵朔, 安达. 激光选区熔化成形316L不锈钢下倾斜面粗糙度的预测与模型建立[J]. 中国激光, 2023, 50(20): 2002301. Guang Yang, Kaibo Cheng, Shuo Zhao, Da An. Prediction and Model Establishment of Inclined Surface Roughness of Laser Selective Melting Formed 316L Stainless Steel[J]. Chinese Journal of Lasers, 2023, 50(20): 2002301.