在线推断校准的小样本目标检测
1 引言
目标检测是计算机视觉领域的基本问题之一,其任务是找出图像中所有感兴趣的目标,确定它们的类别和位置。深度检测模型的成功很大程度上需要大量的数据进行训练,在训练样本较少的条件下,模型容易过拟合,检测效果不佳。收集数据并对数据集进行标注,会耗费大量的人力、物力以及时间。在实际应用中,有相当一部分数据难以获取,严重限制了在一些特殊任务的可扩展性。小样本目标检测技术致力于在少量学习样本条件下,根据仅有的特征信息进行分类和回归,完成目标检测任务,具有学习成本低、学习速度快、可扩展性强等优点。
基于深度学习的目标检测器分为两阶段检测器和单阶段检测器[1]。不同于单阶段检测器直接对目标进行检测,两阶段检测器,R-CNN[2]、SPP-Net[3]、Fast R-CNN[4]、Faster R-CNN[5]有一个单独的模块用于生成区域候选框,在第一阶段找到一定数量的候选目标,并在第二阶段对预测目标进行定位及分类。单阶段检测器,YOLO[6]、SSD[7]、YOLOv2[8]、RetinaNet[9]、YOLOv3[10]、YOLOv4[11]通过密采样直接对语义目标进行分类和定位,它们使用预定义的不同比例和长宽比的先验框来定位目标。相比于单阶段检测器直接完成分类任务和回归任务,两阶段检测器在执行推断任务时由于能对初步预测目标进行精确修正,因此具有更高的检测精度[12]。
尽管以上的检测器性能优异,但难以直接应用于小样本任务。YOLOMAML[13]采用了MAML[14]的方法训练YOLO检测器,但其本质上是用小样本数据直接训练网络。实验结果中出现大量目标错检、目标漏检以及回归框定位不准确的问题。该文章认为直接用小样本数据训练网络效果并不理想,需要寻找新的方法来解决以上问题。Meta R-CNN[15]为验证元知识在小样本检测任务中的有效性,直接用小样本数据训练Faster R-CNN,并对Meta R-CNN和Faster R-CNN性能进行对比。结果显示,Faster R-CNN检测器在1 shot上的检测精度仅为2.7,而Meta R-CNN在1 shot上的精度为19.9。因此,普通的检测器无法胜任小样本检测任务,我们需要引入适用于小样本任务的检测方法。
小样本学习(Few-shot learning),能够有效解决在现实中缺乏大量训练数据以及遇到从未见过的新类别的问题[16-17]。根据在学习时采用的方法不同,小样本学习的方法可分为:数据增强、基于迁移学习的方法、度量学习以及基于元学习的方法。
数据增强可分为对训练数据容量进行扩充和对提取到的特征进行增强。通过对图片进行翻转、缩放、裁剪、亮度变换处理以及通过生成对抗网络来合成相似图像的样本[18-20],以此扩充训练数据容量往往能够对网络性能进行一定程度上的提升。AFHN[21]利用生成对抗网络实现数据集的扩充并引入了两种新的正则化子,分类正则化子和反折叠正则化子,分别提高合成特征的可分辨性和多样性。SARN[22]提出了一个用于小样本学习的自注意关系网络,通过注意力模块对学习到的特征进行增强,再利用关系模块进行比较,提高模型在小样本任务中的分类性能。AAM[23]提出了一种注意力自适应模块,用于调整类别表征和查询样本的特征向量,使其与对应类别的类别表征之间的距离更近。CADA-VAE[24]提出一种基于变分自动编码器的广义零样本学习算法,通过结合图像特征信息和描述特征信息来构建包含重要的多模型信息的潜在特征,并借此实现对未见过的样本的分类。
基于迁移学习的方法[25-27],将经过丰富标注数据进行训练的骨干特征提取网络迁移到小样本任务框架中。LSTD[26]引入全新的小样本检测正则化迁移学习框架,将迁移知识和背景抑制作为正则项,使模型充分利用源域和目标域中的知识,能够缓解小样本带来的迁移问题。
度量学习[28-30]通过度量空间计算不同种类之间的距离,使得相同类别的图片之间的相似度大,不同类别的图片的相似度小。TOPIC[31]提出一种用于解决小样本类别增量学习的算法,利用神经气体网络通过增加AL损失和MML损失项解决了FSCIL中的灾难性遗忘和新样本过拟合的问题。L-GNN[32]提出一种三元损失函数,用来引导网络拉近类内距离,增加类间距离。FSCE[25]提出对比建议编码来学习对比感知的目标建议编码,减小类内距离,增大类间距,加强类别的区分能力。RepMet[28]提出一种基于表征的度量学习方法,在单个端到端的训练过程中同时学习骨干网络参数、嵌入空间和该空间中每个训练类别的多模态分布,解决小样本分类和目标检测问题。AGCM[33]提出一种促进类特征集群之间的正交性的度量学习策略,减少对象类的内类方差和类间偏差,克服小样本目标检测中的类不平衡问题。
元学习致力于找到神经网络中对每个任务较为敏感的全局最优参数,通过微调这些参数,让模型的损失函数快速收敛。MAML[14],Reptile[34],Meta-SGD[35],Meta-LSTM[36]通过学习多组不同的小样本任务,获得一组全局最优初始化参数。Meta R-CNN[15]通过对图像标记部分加上掩码,引入类注意力向量,对提取的特征进行特征融合,并重组预测头,完成小样本目标检测任务。DCNet[27]在基于元学习的框架上提出了具有上下文感知的密集关系蒸馏方法,通过利用支持集的特征来捕获查询图片的细粒度特征获得更全面的特征表示来解决小样本目标检测问题。Meta-yolo[37]使用元特征学习器和一个调整权重模块来解决小样本目标检测问题。Meta-DETR[38]提出类间相关的元学习策略,将查询特征与多个支持类同时聚合,捕获类间相关性,强化模型的泛化能力。
目前主流的方法主要从提升特征提取能力方面对模型进行优化,并没能够充分利用好样本信息。样本不仅可用于学习,也可在进行推断任务时,用于对检测框进行校准。针对上述问题,本文提出了一种在线推断校准的小样本目标检测框架(few-shot object detection via online inferential calibration, FSOIC)。我们在骨干网络上引入了全新的Attention-FPN网络和多组ROI模块,能够在不影响基类信息的条件下学习新类知识,从而引导RPN提取更多高质量的新类前景目标,解决目标漏检问题。同时,本文设计的在线校准模块通过类模板特征对候选目标评分进行校准,促使预测头选择更精确的预测目标,改善目标错检问题。本文在VOC数据集的三个新类子集上进行了大量实验,与14种主流算法进行对比,定量和定性实验结果说明了算法能够有效提升网络检测性能。
2 相关工作
2.1 Faster R-CNN
Faster R-CNN[5]是目标检测中经典的双阶段网络,由骨干网络、RPN模块、ROI模块以及预测头组成,如
Faster R-CNN的骨干网络不唯一,VGG、Resnet都可作为其特征提取网络[39]。第一阶段,将整张图片输入骨干网络进行特征提取后,RPN会对目标生成大量的区域建议框,并进行二分类,判断生成框属于前景还是背景。第二阶段,其内部的ROI模块会对每一个区域候选框进行尺寸固定,筛选感兴趣的区域,最后由分类预测头和回归预测头进行分类和目标定位。由于其出色的检测性能,以及强大的区分前景和背景的能力,能够有效解决单阶段检测器在训练样本稀少时无法定位目标的问题,更适合小样本目标检测任务。
LSTD[26]在小样本任务中用SSD[7]设计边界盒回归,用Faster-RCNN设计目标分类,根据RPN提取的候选前景目标分数选择提案目标。MetaDet[40]引入了一个权重预测元模型,以Faster-R-CNN为框架,对参数化权值预测的元模型进行端到端的训练。该算法将RPN视为与类别无关的组件,利用基类中的元知识促进新类的生成,完成小样本目标检测任务。目前主流的小样本检测器,如FSCE[25]、TIP[41],FSDetView[42]均采用Faster-RCNN为检测器。但以上检测器并未能充分利用样本信息,本文对Faster R-CNN做进一步优化,设计在线校准模块对样本信息进行充分利用,提高网络检测精度。
2.2 FPN
FPN[43]即特征图金字塔网络主要用于解决物体检测中的多尺度问题。通过简单的网络连接,对不同尺度的特征图进行融合,能在几乎不增加网络推理计算量的情况下提升网络检测性能。
FPN并不是一种特定的网络结构,而是一种可自行设计特征融合方式的网络。这也使得FPN网络变得灵活多样,可以根据任务的不同,设计网络结构。在神经网络前向传播的过程中,随着网络层数的增加,特征图会逐渐缩小,每个特征点相对于原始图片的感受野增大,语义信息变得更加丰富。然而,目标位置信息则变得越来越粗略。在小样本目标检测任务中,可学习的样本知识有限,导致底层特征并不能很好地学习到丰富的语义信息。为增强底层特征的语义信息,本文设计了一个由上至下的多尺度特征融合网络,将具有丰富语义信息的高层特征与底层特征进行融合。
2.3 TFA
两阶段微调的方法(TFA)[44]是一种模型学习策略,将具有丰富标签的数据类别定义为基类(base class),仅有的少量的标签数据类别定义为新类(novel class)。在第一阶段,使用基类目标对网络进行预训练,使得网络的骨干网络具有良好的特征提取能力。第二阶段将少量基类和新类目标送入模型中,对骨干网络的参数进行冻结,防止特征提取网络由于数据稀少而发生过拟合,仅微调最后一层分类器,使分类器具备区分不同类别的能力。
TFA w/cos[44],以Faster-RCNN为检测器,采用TFA的学习策略,仅微调检测器的最后一层并固定模型的其余参数。结果显示,TFA的方法可以显著提高检测精度,在性能上优于基于元学习的方法。在TFA w/cos[44]提出后,后续的主流小样本检测算法TFA w/cos+Halluc[18],Retentive R-CNN[45]均采用了TFA的学习策略。经过实验分析,本文认为TFA的学习策略过于统一,难以引导检测器在不同shot任务中拟合参数。本文对TFA w/cos[44]方法进行优化,提出分级冻结的学习机制,使检测模型在训练阶段能够更好地拟合网络参数。
3 方法
在实际推断阶段,有相当一部分候选框回归精确但因为评分较低而被淘汰。为充分利用样本信息,我们设计了一个在线推断校准模块,作用于模型预测头。该模块根据标签文件提取到类实例,通过类模板生成模块,将提取到的特征图压缩成一维类向量,并在进行推断任务时,对众多候选预测框进行匹配校准,获得更多高质量的候选框。同时,本文保留了更多的区域候选框,促使更多的候选框可以跟模板进行匹配校准,提升识别的准确率。
3.1 FSOIC整体框架
改进后的Faster R-CNN检测框架由Attention-FPN骨干网络、RPN模块、多组ROI模块、在线校准模块以及预测头组成,如
大量实验证明,在小样本条件下检测精度过低并非回归精度不足,而是检测器出现大量错分类和漏检问题。直接在少量样本条件下训练网络会导致模型过拟合。因此,基于TFA的方法冻结了整个模型,仅更新模型最后一层的分类回归器参数,导致RPN无法获取足够的新类信息。由于缺乏新类信息,RPN难以正确识别新类前景目标,导致检测器出现错检与漏检现象。如
为防止骨干网络过度拟合新类特征,本文在冻结骨干网络的基础上设计了Attention-FPN网络,学习新类知识。同时,根据训练样本数量选择是否更新RPN与多组ROI参数,并调整RPN保留的建议框数量。为使RPN更好地学习新类特征,并让ROI充分提取感兴趣的特征,我们引入分级冻结学习机制。相比TFA仅对最后一层的预测头参数进行更新,本文并未对全部的模块进行冻结,而是有针对性地冻结部分网络,如
表 1. 分级冻结机制
Table 1. Hierarchical freezing mechanism
|
分类预测头和回归预测头分别输出预测框目标的种类和位置坐标。训练时,模型的损失函数由RPN模块的候选目标框损失
用Smooth L1 Loss函数(用Ls表示)将标签数据的位置信息定义为
二分类交叉熵函数用于判别两个概率分布之间的距离,将预测目标定义为
3.2 Attention-FPN骨干网络
Tsung-Yi Lin在FPN[43]一文指出,高分辨率特征图对物体的识别表征能力较弱。底层特征虽然具有更强的位置信息,但缺少全局视野导致语义信息薄弱。高层特征虽然分辨率低下,却具有丰富的语义信息。将高层特征与底层特征进行融合,可以有效增强底层特征的语义信息。为解决错分类与漏检问题,我们需要引导RPN提取更多的新类目标。因此,本文设计了一个自上而下的注意力多尺度特征融合网络Attention-FPN。通过增强底层特征的语义信息,RPN能够获取更多丰富的新类知识,提取更精准的新类前景目标。如
本文分别对Resnet-101网络不同尺度的特征图由上至下进行特征融合,输出4个不同尺度的特征图。在特征融合网络的前三个输入端,引入了通道注意力机制。对特征图进行压缩,经过两层全连接层,将展成一维向量进行sigmoid,为每一个特征通道生成权重,建立各个通道之间的相关性,根据类别为输入特征进行权重分配,建模各个通道间的依赖关系,如
骨干网络输出的特征图,将作为RPN模块的输入用于生成区域建议框。同时,本文引入4组ROI Align池化层,用于对不同尺度候选区域的特征图进行区域选取和尺寸固定,并将提取到的候选特征图作用于预测头进行分类和回归预测。
3.3 候选框校准
由于样本特征不均衡性,导致新类候选目标评分过低,在进行预测框筛选时被过滤,造成检测器错检与漏检。
由于神经网络的封闭性,单纯地进行特征融合具有不确定性,特征图很难按照让我们满意的方向进行融合。直接将样本模板特征与预测目标特征进行融合,效果并不理想。
模板更新[46-50]的方法常用于目标跟踪任务中,将前一帧图像中目标与当前帧的目标进行匹配,跟踪当前帧图像中的目标,取得了巨大的成功。本文认为这类方法同样适用于小样本目标检测任务,并设计了一个作用于推断任务的候选框在线评分校准模块,包括一个类模板生成模块、模板匹配模块以及评分校准模块。
类模板生成模块由Faster R-CNN的骨干特征提取网络、四个ROI模块以及两个全连接层组成,构成一个分类器。具体的网络结构如
首先,骨干网络对样本图片进行信息编码,生成多个不同尺度的特征图。其次,ROI模块根据样本自带的标签位置信息裁剪特征图,过滤图片中的背景信息,并将多尺度的特征图转化为固定大小的特征图。最后,通过两层全连接层将特征图尺寸转换为大小为
在特征度量空间中,不同类别的特征向量方向差异明显,而相同类别的特征向量具有相近的方向。为合成类模板,模板生成模块将各个类别生成的特征向量融合成一个向量,作为类模板,并作用于特征相似度量空间,如
模板生成模块将生成的第j个类向量定义为
模板匹配模块将i类样本模板定义为
候选框评分校准模块将原始候选目标评分定义为
候选框校准模块对每个新类候选预测框的评分进行加权更新,分类预测头将根据候选框的置信度进行筛选得出最佳的预测框。
如
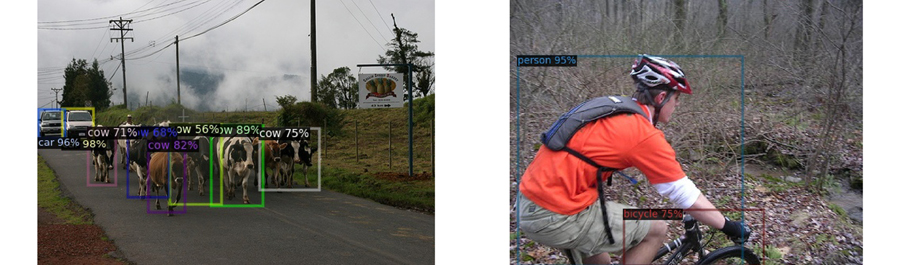
图 9. 10 shot任务中遮挡条件下的检测结果
Fig. 9. Detection results under the occlusion conditions in the 10 shot task
4 实验
4.1 实验设置
本文在配有8张NVIDIA GeForce RTX 3090显卡的服务器上进行实验。基于Pascal VOC数据集,我们将小样本目标检测任务按照训练的样本数量划分为1、2、3、5、10 shot共5个任务。基于COCO数据集,我们将检测任务分为10 shot和30 shot,实验参数如
表 2. 数据集实验设置
Table 2. Experimental settings of the dataset
|
4.2 实验结果比较
为了进一步验证改进后的算法性能,本文将FSOIC算法与目前最先进的小样本目标检测算法在通用数据集PASCAL VOC的三个新类子集上进行性能比较,测试集共4952张图片,包括14976个目标实例,实验结果如
表 3. 小样本目标检测算法在VOC新类划分集的性能分析比较表
Table 3. Performance analysis and comparison of the few shot object detection algorithm in VOC new class partition sets
|
小样本检测在COCO数据集上的结果如
表 4. 小样本目标检测算法在COCO数据集的性能分析比较
Table 4. Performance analysis and comparison of few shot object detection algorithms in the COCO datasets
|
4.3 消融实验
为探究Attention-FPN网络、模板匹配模块以及调整RPN模块的候选框数量对小样本目标检测性能的影响,本文以nAP50为评价标准,对算法优化的方法进行了消融实验,如
表 5. 消融实验性能比较
Table 5. Comparison of the ablation experimental performance
|
为验证各个模块的实际检测性能,本文在
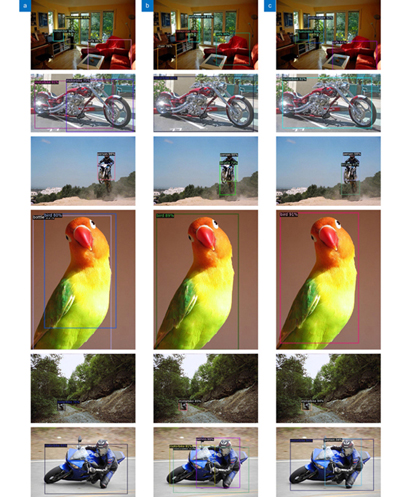
图 10. 10 shot 任务下的检测结果。(a) 基于TFA的Faster R-CNN网络检测结果;(b) 使用在线推断校准模块的FasterR-CNN网络检测结果;(c) 使用在线推断校准模块并添加Attention-FPN网络的Faster R-CNN网络检测结果
Fig. 10. 10 shot task detection results. (a) Detection results of the Faster R-CNN network based on TFA; (b) Detection results of the Faster R-CNN net work using the online inference calibration module; (c) Detection results of the Faster R-CNN network using the online inference calibration module and adding the Attention-FPN network
5 总结
为解决小样本检测的错检与漏检问题,本文对TFA w/cos[44]算法进行优化。本文在训练阶段,引入Attention-FPN和多组ROI模块,并使用分级冻结的学习策略引导RPN学习新类知识,提升网络对新类特征提取能力;在目标预测阶段,引入评分校准模块对候选目标预测评分进行修正并过滤评分较低的候选框,纠正错检目标;通过调整RPN模块来增大候选目标框的数量,对更多的候选目标框进行校准,避免模型漏检。实验结果表明,本文提出的FSOIC算法有效提升了检测器在小样本目标检测任务中的性能。本文的下一步工作考虑对RPN模块进行优化,采用双RPN结构,分别对基类目标和新类目标进行特征提取,根据预测的种类筛选不同的特征,提高对目标的识别和回归精度。
[1] 陈旭, 彭冬亮, 谷雨基于改进YOLOv5s的无人机图像实时目标检测光电工程202249321037210.12086/oee.2022.210372
[2] Girshick R, Donahue J, Darrell T, et al. Rich feature hierarchies for accurate object detection and semantic segmentation[C]//Proceedings of the 2014 IEEE Conference on Computer Vision and Pattern Recognition, 2014: 580−587. https://doi.org/10.1109/CVPR.2014.81.
[4] Girshick R. Fast R-CNN[C]//Proceedings of the 2015 IEEE International Conference on Computer Vision, 2015: 1440–1448. https://doi.org/10.1109/ICCV.2015.169.
[5] Ren S Q, He K M, Girshick R, et al. Faster R-CNN: towards real-time object detection with region proposal networks[C]//Proceedings of the 28th International Conference on Neural Information Processing Systems, 2015, 91–99.
[6] Redmon J, Divvala S, Girshick R, et al. You only look once: unified, real-time object detection[C]//Proceedings of the 2016 IEEE Conference on Computer Vision and Pattern Recognition, 2016: 779–788. https://doi.org/10.1109/CVPR.2016.91.
[7] Liu W, Anguelov D, Erhan D, et al. SSD: single shot MultiBox detector[C]//14th European Conference on Computer Vision, 2016: 21–37. https://doi.org/10.1007/978-3-319-46448-0_2.
[8] Redmon J, Farhadi A. YOLO9000: better, faster, stronger[C]//2017 IEEE Conference on Computer Vision and Pattern Recognition, 2017: 6517–6525. https://doi.org/10.1109/CVPR.2017.690.
[9] Lin T Y, Goyal P, Girshick R, et al. Focal loss for dense object detection[C]//Proceedings of the 2017 IEEE International Conference on Computer Vision, 2017: 2999−3007. https://doi.org/10.1109/ICCV.2017.324.
[10] Redmon J, Farhadi A. YOLOv3: an incremental improvement[Z]. arXiv: 1804.02767, 2018. https://arxiv.org/abs/1804.02767.
[11] Bochkovskiy A, Wang C Y, Liao H Y M. YOLOv4: optimal speed and accuracy of object detection[Z]. arXiv: 2004.10934, 2020. https://arxiv.org/abs/2004.10934.
马梁, 苟于涛, 雷涛, 等基于多尺度特征融合的遥感图像小目标检测光电工程202249421036310.12086/oee.2022.210363
[13] Bennequin E. Meta-learning algorithms for few-shot computer vision[Z]. arXiv: 1909.13579, 2019. https://arxiv.org/abs/1909.13579.
[14] Behl H S, Baydin A G, Torr P H S. Alpha MAML: adaptive model-agnostic meta-learning[Z]. arXiv: 1905.07435, 2019. https://arxiv.org/abs/1905.07435.
[15] Yan X P, Chen Z L, Xu A N, et al. Meta R-CNN: towards general solver for instance-level low-shot learning[C]//2019 IEEE/CVF International Conference on Computer Vision (ICCV), 2019: 9576–9585. https://doi.org/10.1109/ICCV.2019.00967.
[16] Wang Y Q, Yao Q M. Few-shot learning: a survey[Z]. arXiv: 1904.05046v1, 2019. https://arxiv.org/abs/1904.05046v1.
[17] Duan Y, Andrychowicz M, Stadie B, et al. One-shot imitation learning[C]//Proceedings of the 31st International Conference on Neural Information Processing Systems, 2017: 1087–1098.
[18] Zhang W L, Wang Y X. Hallucination improves few-shot object detection[C]//Proceedings of the 2021 IEEE/CVF Conference on Computer Vision and Pattern Recognition, 2021: 13003–13012. https://doi.org/10.1109/CVPR46437.2021.01281.
[19] Zhu J Y, Park T, Isola P, et al. Unpaired image-to-image translation using cycle-consistent adversarial networks[C]//2017 IEEE International Conference on Computer Vision, 2017: 2242–2251. https://doi.org/10.1109/ICCV.2017.244.
[20] Goodfellow I J, Pouget-Abadie J, Mirza M, et al. Generative adversarial nets[C]//Proceedings of the 27th International Conference on Neural Information Processing Systems, 2014: 3672–2680.
[21] Li K, Zhang Y L, Li K P, et al. Adversarial feature hallucination networks for few-shot learning[C]//Proceedings of the 2020 IEEE/CVF Conference on Computer Vision and Pattern Recognition, 2020: 13467–13476. https://doi.org/10.1109/CVPR42600.2020.01348.
[22] Hui B Y, Zhu P F, Hu Q H, et al. Self-attention relation network for few-shot learning[C]//2019 IEEE International Conference on Multimedia & Expo Workshops (ICMEW), 2019: 198–203. https://doi.org/10.1109/ICMEW.2019.00041.
[24] Schönfeld E, Ebrahimi S, Sinha S, et al. Generalized zero-and few-shot learning via aligned variational autoencoders[C]//2019 IEEE/CVF Conference on Computer Vision and Pattern Recognition (CVPR), 2019: 8239–8247. https://doi.org/10.1109/CVPR.2019.00844.
[25] Sun B, Li B H, Cai S C, et al. FSCE: few-shot object detection via contrastive proposal encoding[C]//Proceedings of the 2021 IEEE/CVF Conference on Computer Vision and Pattern Recognition, 2021: 7348–7358. https://doi.org/10.1109/CVPR46437.2021.00727.
[26] Chen H, Wang Y L, Wang G Y, et al. LSTD: a low-shot transfer detector for object detection[C]//Proceedings of the Thirty-Second AAAI Conference on Artificial Intelligence and Thirtieth Innovative Applications of Artificial Intelligence Conference and Eighth AAAI Symposium on Educational Advances in Artificial Intelligence, 2018: 346.
[27] Hu H Z, Bai S, Li A X, et al. Dense relation distillation with context-aware aggregation for few-shot object detection[C]//Proceedings of the 2021 IEEE/CVF Conference on Computer Vision and Pattern Recognition, 2021: 10180–10189. https://doi.org/10.1109/CVPR46437.2021.01005.
[28] Karlinsky L, Shtok J, Harary S, et al. RepMet: representative-based metric learning for classification and few-shot object detection[C]//Proceedings of the 2019 IEEE/CVF Conference on Computer Vision and Pattern Recognition, 2019: 5197–5206. https://doi.org/10.1109/CVPR.2019.00534.
[30] Sung F, Yang Y X, Zhang L, et al. Learning to compare: relation network for few-shot learning[C]//Proceedings of the 2018 IEEE/CVF Conference on Computer Vision and Pattern Recognition, 2018: 1199–1208. https://doi.org/10.1109/CVPR.2018.00131.
[31] Tao X Y, Hong X P, Chang X Y, et al. Few-shot class-incremental learning[C]//Proceedings of the 2020 IEEE/CVF Conference on Computer Vision and Pattern Recognition, 2020: 12180–12189. .
[32] Wang Y, Wu X M, Li Q M, et al. Large margin few-shot learning[Z]. arXiv: 1807.02872, 2018. https://doi.org/10.48550/arXiv.1807.02872.
[33] Agarwal A, Majee A, Subramanian A, et al. Attention guided cosine margin to overcome class-imbalance in few-shot road object detection[C]//2022 IEEE/CVF Winter Conference on Applications of Computer Vision Workshops (WACVW), 2022: 221–230. https://doi.org/10.1109/WACVW54805.2022.00028.
[34] Nichol A, Achiam J, Schulman J. On first-order meta-learning algorithms[Z]. arXiv: 1803.02999, 2018. https://arxiv.org/abs/1803.02999.
[35] Li Z G, Zhou F W, Chen F, et al. Meta-SGD: learning to learn quickly for few-shot learning[Z]. arXiv: 1707.09835, 2017. https://arxiv.org/abs/1707.09835.
[36] Ravi S, Larochelle H. Optimization as a model for few-shot learning[C]//5th International Conference on Learning Representations, 2016.
[37] Kang B Y, Liu Z, Wang X, et al. Few-shot object detection via feature reweighting[C]//Proceedings of the 2019 IEEE/CVF International Conference on Computer Vision, 2019: 8419–8428. https://doi.org/10.1109/ICCV.2019.00851.
[38] Zhang G J, Luo Z P, Cui K W, et al. Meta-DETR: image-level few-shot detection with inter-class correlation exploitation[J]. IEEE Trans Pattern Anal Mach Intell, 2022. https://doi.org/10.1109/TPAMI.2022.3195735.
马雯, 于炯, 王潇, 等基于改进Faster R-CNN的垃圾检测与分类方法计算机工程202147829430010.19678/j.issn.1000-3428.0058258
[40] Wang Y X, Ramanan D, Hebert M. Meta-learning to detect rare objects[C]//Proceedings of the 2019 IEEE/CVF International Conference on Computer Vision, 2019: 9924–9933. https://doi.org/10.1109/ICCV.2019.01002.
[41] Li A X, Li Z G. Transformation invariant few-shot object detection[C]//Proceedings of the 2021 IEEE/CVF Conference on Computer Vision and Pattern Recognition, 2021: 3093–3101. https://doi.org/10.1109/CVPR46437.2021.00311.
[42] Xiao Y, Marlet R. Few-shot object detection and viewpoint estimation for objects in the wild[C]//16th European Conference on Computer Vision, 2020: 192−210. https://doi.org/10.1007/978-3-030-58520-4_12.
[43] Lin T Y, Dollár P, Girshick R, et al. Feature pyramid networks for object detection[C]//Proceedings of the 2017 IEEE Conference on Computer Vision and Pattern Recognition, 2017: 936−944. https://doi.org/10.1109/CVPR.2017.106.
[44] Wang X, Huang T, Gonzalez J, et al. Frustratingly simple few-shot object detection[C]//Proceedings of the 37th International Conference on Machine Learning, 2020: 9919–9928.
[45] Fan Z B, Ma Y C, Li Z M, et al. Generalized few-shot object detection without forgetting[C]//Proceedings of the 2021 IEEE/CVF Conference on Computer Vision and Pattern Recognition, 2021: 4525−4534. https://doi.org/10.1109/CVPR46437.2021.00450.
[46] Bertinetto L, Valmadre J, Henriques J F, et al. Fully-convolutional siamese networks for object tracking[C]//14th European Conference on Computer Vision, 2016: 850–865. https://doi.org/10.1007/978-3-319-48881-3_56.
[47] Li B, Yan J J, Wu W, et al. High performance visual tracking with Siamese region proposal network[C]//Proceedings of the 2018 IEEE/CVF Conference on Computer Vision and Pattern Recognition, 2018: 8971−8980. https://doi.org/10.1109/CVPR.2018.00935.
[48] Zhu Z, Wang Q, Li B, et al. Distractor-aware Siamese networks for visual object tracking[C]//Proceedings of the 15th European Conference on Computer Vision, 2018: 103–119. https://doi.org/10.1007/978-3-030-01240-3_7.
赵春梅, 陈忠碧, 张建林基于卷积网络的目标跟踪应用研究光电工程202047118066810.12086/oee.2020.180668
赵春梅, 陈忠碧, 张建林基于深度学习的飞机目标跟踪应用研究光电工程201946918026110.12086/oee.2019.180261
[51] Wu J X, Liu S T, Huang D, et al. Multi-scale positive sample refinement for few-shot object detection[C]//Proceedings of the 16th European Conference on Computer Vision, 2020: 456–472. https://doi.org/10.1007/978-3-030-58517-4_27.
Article Outline
彭昊, 王婉祺, 陈龙, 彭先蓉, 张建林, 徐智勇, 魏宇星, 李美惠. 在线推断校准的小样本目标检测[J]. 光电工程, 2023, 50(1): 220180. Hao Peng, Wanqi Wang, Long Chen, Xianrong Peng, Jianlin Zhang, Zhiyong Xu, Yuxing Wei, Meihui Li. Few-shot object detection via online inferential calibration[J]. Opto-Electronic Engineering, 2023, 50(1): 220180.