基于线阵扫描成像的太赫兹图像超分辨率重建【增强内容出版】
Terahertz waves are widely used in security checks and nondestructive testing. However, because of limitations in imaging devices and algorithms, terahertz images face challenges, such as limited spatial resolution and blurry features. Currently, two main approaches are used to enhance the resolution of terahertz images. The first involves improving the imaging system, whereas the second uses super-resolution reconstruction algorithms to improve image quality. In recent years, research teams have applied convolutional super-resolution networks to terahertz image reconstruction. However, these methods construct network models by increasing the number of convolutional layers, leading to an exponential expansion of the parameters and a lack of specific structures to enhance the reconstruction of image details. Although deeper networks can extract more information for image reconstruction, overfitting complicates training. In this study, a super-resolution reconstruction algorithm is developed based on residual-attention generative adversarial networks to further improve the quality of reconstructed images.
The proposed super-resolution reconstruction algorithm is based on a residual attention generative adversarial network. First, a contrast-limited adaptive histogram equalization method is introduced for terahertz images, effectively addressing the low-contrast issue. Second, building upon the generative adversarial network, a residual generative adversarial network incorporating an improved attention mechanism achieves super-resolution reconstruction of terahertz scanning images. This algorithm features a multibranch residual block convolutional structure and extracts and fuses the feature information from each layer during the feature extraction process. In addition, an enhanced attention mechanism combining spatial and channel attention is added to the residual block. Beyond channel information extraction, it includes orientation awareness and position-sensitive information, compelling the network to focus more on texture and image details while maintaining image shape integrity and reducing the network parameters. Finally, a spectrum-normalized U-net network is employed to discriminate the reconstructed images generated by the generator, thereby enhancing training stability.
Test images from the terahertz transmission scanning dataset are selected [Fig.5(a)] to validate the effectiveness of the proposed algorithm in image super-resolution reconstruction. The results of the two images indicate that the proposed algorithm clarifies edge information [Fig.5(f)]. In contrast, other algorithms such as bicubic interpolation, SRResNet, SRGAN, and ESRGAN exhibit various issues [Figs.5(b)?(e)]. The traditional bicubic interpolation algorithm is overall unsatisfactory and appears blurry. Images reconstructed by the SRResNet and SRGAN algorithms show problems, such as increased noise and suboptimal visual coherence. Although the ESRGAN algorithm improves the clarity compared to the original image, pseudoartifacts in the edges lead to image blurriness. The objective evaluation metrics for the images processed using the algorithm in this study show a significant improvement over those of the original images, and the enhancement is higher compared with those of bicubic interpolation, SRResNet, SRGAN, and ESRGAN (Table 1). The experimental results demonstrate that compared to other image super-resolution algorithms, the proposed algorithm uses low-resolution image information more comprehensively, improving information utilization and exhibiting superior reconstruction performance.
A terahertz image super-resolution reconstruction algorithm based on generative adversarial networks is established to address issues, such as low clarity and blurred edges in images captured by terahertz line-scan cameras. Building on the SRGAN, a residual dense network incorporating an enhanced attention mechanism is introduced, and a spectrum-normalized U-net is used as the discriminative network. The critical parameters in this network can be adaptively updated using the attention model by incorporating pixel coordinates into the attention mechanism. A terahertz image training dataset is established to apply this network to terahertz image super-resolution reconstruction. The experimental results indicate that the proposed super-resolution reconstruction algorithm, which is based on generative adversarial networks and attention mechanisms, qualitatively aligns better with human observation habits, highlighting more nuanced edge information in the reconstructed images. Quantitatively, various metrics for the reconstructed high-resolution images show improvements, with a 7% increase in edge intensity, 12% increase in average gradient, 13% increase in peak signal-to-noise ratio, and 14% increase in structural similarity. These results validate the superiority and effectiveness of the proposed algorithm in enhancing the clarity of terahertz images.
1 引言
太赫兹波位于微波和红外之间的频段,其频率范围为0.1~10.0 THz。太赫兹波具有一些独特的特性,如瞬态、低能量和穿透性,这些特点使其在医学成像[1]、安全检测[2]、爆炸物检测[3-4]、通信以及生物研究[5]等领域中展现出巨大的应用潜力。然而,受限于太赫兹波的长波长和软硬件的限制,传统太赫兹成像系统所成的太赫兹图像存在对比度低、分辨率差、边缘信息丢失严重等问题。
目前主要有两种方法可以提高太赫兹图像的分辨率:第一种是对成像系统进行改进。利用近场探测倏逝波可突破衍射极限,基于该思路设计相关光学器件,通过采集近场倏逝波,可实现超分辨率成像。虽然这种方法可以从源头上提升成像的分辨率,但是在实际应用中,从硬件层面对系统进行改动将面临高成本的问题。第二种方法是利用图像处理算法来提高太赫兹图像的质量[6]。利用传统数学模型或基于深度学习,恢复或合成低分辨率图中的信息,可生成高分辨率图像[7]。Dong等[8]利用卷积神经网络(CNN)实现图像的超分辨率,提出了一种基于CNN的超分辨率重建算法。同时,也有研究团队将卷积超分辨率网络应用于太赫兹图像重建[9-11]。然而,这些方法通过增加卷积层的数量来构建网络模型,参数呈指数级增加,并且没有引入特定的结构来增强图像细节的重建。Ledig等[12]利用生成对抗网络(GAN)实现图像的超分辨率,提出了一种基于生成对抗网络的超分辨率重建算法(SRGAN),利用感知损失驱动重建出逼真的高分辨率图像。Su等[13]提出了一种新的子空间和注意力引导恢复网络,该网络通过注意力引导来融合幅度和相位的空间频谱特征。沈松[14]通过引入稠密连接残差模块代替SRGAN的残差模块,并删除了批量正则化层,以挖掘更深层的特征并改善网络性能。
针对太赫兹扫描成像设备存在图像清晰度差、边缘模糊等问题,本文提出了一种基于残差注意力生成对抗性网络的超分辨率重建算法。该算法具有多分支残差块卷积结构,在特征提取过程中从每一层获得特征信息并进行特征融合。此外,将结合了空间注意力和通道注意力的增强注意力机制添加到残差块。除了从通道中提取信息外,获得的特征信息还包含方向感知和位置敏感信息,使得网络更多地关注纹理和图片细节,同时保持图像形状的完整性并减少网络参数。此外,本文还采取可实现频谱归一化(SN)[15]的U-net判别器来提高判别器的能力,同时稳定训练过程,实现了局部细节增强和伪影抑制之间的良好平衡。
2 基本原理
2.1 太赫兹图像获取装置与图像预处理
实验使用的太赫兹线阵快速相机成像系统包括太赫兹雪崩发射源、反射镜、线阵太赫兹探测器、传送带、一维扫描电机等,该系统光路图如
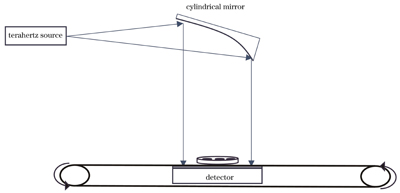
图 1. 太赫兹线阵扫描成像系统光路图
Fig. 1. Optical path diagram of terahertz linear array scanning imaging system
将装在箱子里的剪刀和牙刷放置在传送带平台上(
扫描得到的太赫兹图像对比度低,边缘模糊。为了解决这一问题,基于自适应直方图均衡化技术[16],提出了一种处理图像时引入限制对比度的自适应直方图均衡化方法,提高了对比度。该方法增强了不同区域的对比度,抑制了不必要的信息,突出了样本与背景的对比度。
2.2 太赫兹图像超分辨率算法的构建
利用基于注意力机制和残差密集模块的生成对抗性网络,本文提出了一种太赫兹图像超分辨率算法,该网络由生成网络和判别网络组成。判别网络用于检测生成的超分辨率图像与原始图像之间的差异,并在生成器网络的训练中加入判别器损失,使网络能够更好地恢复真实的图像特征。生成网络用于将低分辨率图像映射到超分辨率图像中。
如
第二部分为浅层特征抽取网络,提取浅层特征。低分辨率图像将被输入到一个3×3的卷积层中,并在卷积操作后应用LReLU激活函数。这个卷积层的输出通道数被设置为64,以便通过卷积操作对输入图像进行特征提取和维度调整。第三部分为深度特征映射提取模块,该模块由增强注意力残差密集块(EARDB)组成,EARDB的架构如
除了改进生成器的结构外,采用频谱归一化的U-Net作为判别网络,其结构图如
为了防止在训练过程中出现过度拟合和梯度消失问题,本文采用频谱归一化来提高U-net的泛化性能、训练稳定性和鲁棒性。此外,我们观察到SN也有利于减少GAN训练引入的过于尖锐和令人讨厌的伪影。通过这些调整,我们能够轻松地训练太赫兹图像样本,增加局部细节并去除伪影。
2.3 建立太赫兹图像损失函数模型
对于建立的太赫兹图像损失函数模型,其生成模块的损失函数可以分为三个部分:
式中:
式中:
式中:
式中:
2.4 太赫兹重建图像的质量评价指标
本文利用主观和客观双重评价方法对太赫兹重建图像的质量进行比较。主观评价使用自然图像质量评估[17](NIQE),表达式为
式中:
太赫兹重建图像的客观评价指标分为三项。第一项为结构相似度[18](SSIM),是从亮度、对比度和结构三个方面来衡量参考图像与失真图像之间的结构相似性。SSIM表达式为
式中:
第二项为峰值信噪比(PSNR)。PSNR通过比较两个图像之间的相似度,评判重建太赫兹图像的质量。但这两项指标需要比较原始高分辨率图像和重建高分辨率图像,而太赫兹成像无高分辨率图,所以只能通过双三次插值算法重建太赫兹图像以对比其他算法的结果。PSNR值由均方误差(MMSE)决定,其表达式为
式中:
PSNR表达式为
式中:
第三项是以图像边缘信息为评判指标的边缘强度(ES)和平均梯度(AG)[19]。边缘强度反映了图像中边缘点的梯度幅值。平均梯度用来反映图像的清晰度,定义为图像边缘的灰度变化快慢,其数值越大视觉效果越好。
3 模型训练与结果分析
3.1 太赫兹重建图像超分辨率模型的训练
太赫兹重建图像的超分辨率模型使用PyTorch框架和NVIDIA RTX 4090 GPU进行训练。本实验采用扫描的太赫兹图像(共2000幅)构建数据集,同时再添加100幅图像作为测试集。在构建数据集的过程中,需要对太赫兹图像进行适当的样本扩充,因此针对太赫兹图像采用旋转和尺度缩放的方式进行样本扩充。同时对太赫兹图像采用自适应直方图进行均衡化处理,增加了太赫兹图像的对比度,有利于超分辨率重建。
训练生成对抗网络时,batch size设置为16,优化器设置为Adam优化器,初始学习率设置为2×10-4。优化器参数
3.2 图像超分辨率重构结果的分析
为了验证本文改进算法对图像的超分辨率重构效果,选取测试集中的太赫兹透射扫描图片。对图片进行4倍放大的超分辨率重建,并将重构结果与双三次插值(bicubic interpolation)、SRResnet、SRGAN、ESRGAN算法进行比较。对不同算法的重建结果进行对比,通过主观和客观评价指标量化进行分析。
各个算法重建图像的对比图如

图 5. 牙刷太赫兹图像的实验结果。(a)低分辨率图像;(b)双三次插值;(c)SRResnet;(d)SRGAN;(e)ESRGAN;(f)本文算法
Fig. 5. Experimental results of toothbrush THZ image. (a) Low resolution image; (b) bicubic interpolation; (c) SRResnet; (d) SRGAN;(e) ESRGAN; (f) our method

图 6. 剪刀太赫兹图像的实验结果。(a)低分辨率图像;(b)双三次插值;(c)SRResnet;(d)SRGAN;(e)ESRGAN;(f)本文算法
Fig. 6. Experimental results of scissors THZ image. (a) Low resolution image; (b) bicubic interpolation; (c) SRResnet; (d) SRGAN; (e) ESRGAN; (f) our method
从
表 1. 不同算法重建图像的客观评价结果
Table 1. Objective evaluation results of reconstructed images by different algorithms
|
4 结论
针对太赫兹线阵扫描相机所获得的图像存在的清晰度差、边缘模糊等问题,提出了基于生成对抗网络的太赫兹图像超分辨率重建算法。在SRGAN的基础上引入了增强注意力机制的残差密集网络,并采用频谱归一化的U-net作为判别网络。该网络中的关键参数可以通过注意力模型自适应更新,且像素坐标信息被纳入注意力机制。为了将该网络应用于太赫兹图像超分辨率重建,建立了太赫兹图像训练数据集。实验结果表明:所提出的基于生成对抗网络和注意力机制的超分辨率重建算法,其重建结果从主观上更符合人眼的观察习惯,边缘信息更加细腻突出;从客观评价标准来看,重建的高分辨率图像的各指标均有所提升,边缘强度提高了7%,平均梯度提高了12%,峰值信噪比提高了13%,结构相似性提高了14%。研究结果验证了该算法的优越性和有效性,提高了太赫兹图像的清晰度。
[1] Chen C, Wu F L, Zhou H M, et al. Research progress in the application of terahertz spectroscopy and imaging technology in stomatology[J]. Journal of Sichuan University. Medical Science Edition, 2023, 54(1): 203-207.
[2] Chen Z, Ma X Y, Zhang B, et al. A survey on terahertz communications[J]. China Communications, 2019, 16(2): 1-35.
[3] 曾子威, 金尚忠, 李宏光, 等. 高湿度环境下爆炸物太赫兹光谱的特征提取与精准识别[J]. 光学 精密工程, 2023, 31(7): 1065-1073.
[4] 解琪, 杨鸿儒, 李宏光, 等. 基于太赫兹时域光谱系统的爆炸物识别[J]. 光学 精密工程, 2016, 34(10): 2392-2399.
Xie Q, Yang H R, Li H G, et al. Explosive identification based on terahertz time-domain spectral system[J]. Optics and Precision Engineering, 2016, 34(10): 2392-2399.
[6] Li Y D, Hu W D, Zhang X, et al. Adaptive terahertz image super-resolution with adjustable convolutional neural network[J]. Optics Express, 2020, 28(15): 22200-22217.
[7] 曹丙花, 张宇盟, 范孟豹, 等. 太赫兹超分辨率成像研究进展[J]. 中国光学, 2022, 15(3): 405-417.
[8] Dong C, Loy C C, He K M, et al. Image super-resolution using deep convolutional networks[J]. IEEE Transactions on Pattern Analysis and Machine Intelligence, 2016, 38(2): 295-307.
[9] Long Z Y, Wang T Y, You C W, et al. Terahertz image super-resolution based on a deep convolutional neural network[J]. Applied Optics, 2019, 58(10): 2731-2735.
[10] Wang Y, Qi F, Wang J K. Terahertz image super-resolution based on a complex convolutional neural network[J]. Optics Letters, 2021, 46(13): 3123-3126.
[11] Yang X W, Zhang D H, Wang Z M, et al. Super-resolution reconstruction of terahertz images based on a deep-learning network with a residual channel attention mechanism[J]. Applied Optics, 2022, 61(12): 3363-3370.
[12] LedigC, TheisL, HuszárF, et al. Photo-realistic single image super-resolution using a generative adversarial network[C]∥2017 IEEE Conference on Computer Vision and Pattern Recognition (CVPR), July 21-26, 2017, Honolulu, HI, USA. New York: IEEE Press, 2017: 105-114.
[13] SuW T, HungY C, YuP J, et al. Seeing through a black box: toward high-quality terahertz imaging via subspace-and-attention guided restoration[M]∥AvidanS, BrostowG, CisséM, et al. Computer vision-ECCV 2022. Lecture notes in computer science. Cham: Springer, 2022, 13667: 453-469.
[14] 沈松. 基于深度学习的毫米波无源成像超分辨算法研究[D]. 成都: 电子科技大学, 2019: 31-34.
ShenS. Resolution algorithms based on deep learning research on millimeter wave passive imaging super[D]. Chengdu: University of Electronic Science and Technology of China, 2019: 31-34.
[16] AamirM, RehmanZ, PuY F, et al. Image enhancement in varying light conditions based on wavelet transform[C]∥2019 16th International Computer Conference on Wavelet Active Media Technology and Information Processing, December 14-15, 2019, Chengdu, China. New York: IEEE Press, 2019: 317-322.
[17] Mittal A, Soundararajan R, Bovik A C. Making a “completely blind” image quality analyzer[J]. IEEE Signal Processing Letters, 2013, 20(3): 209-212.
[18] LiQ H, FangY M, LinW S, et al. Gradient-weighted structural similarity for image quality assessments[C]∥2015 IEEE International Symposium on Circuits and Systems (ISCAS), May 24-27, 2015, Lisbon, Portugal. New York: IEEE Press, 2015: 2165-2168.
[19] 王欢, 郎利影, 庞亚军, 等. 连续波太赫兹成像系统的单幅图像超分辨重建[J]. 红外与激光工程, 2023, 52(1): 20220292.
Article Outline
郭宇烽, 金尚忠, 李宏光, 曾子威, 廖文焘. 基于线阵扫描成像的太赫兹图像超分辨率重建[J]. 中国激光, 2024, 51(8): 0814001. Yufeng Guo, Shangzhong Jin, Hongguang Li, Ziwei Zeng, LiaoWentao. Super‐Resolution Reconstruction of Terahertz Image Based on Linear Array Scanning Imaging[J]. Chinese Journal of Lasers, 2024, 51(8): 0814001.