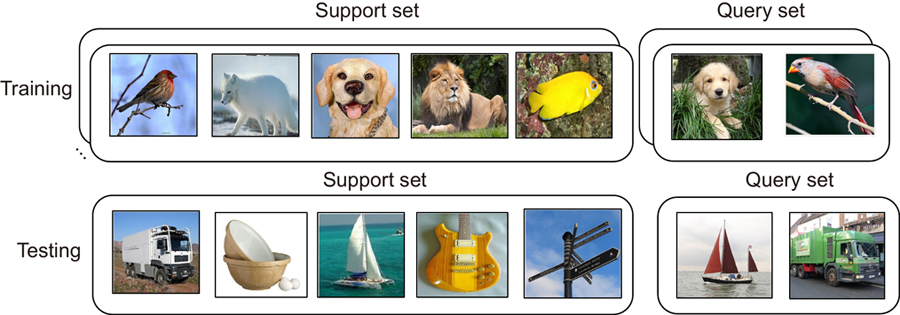
1 中国科学院光电技术研究所,四川 成都 610209
2 中国科学院大学电子电气与通信工程学院,北京 100049
To improve the performance of few-shot classification, we present a general and flexible method named Multi-Scale Attention and Domain Adaptation Network (MADA). Firstly, to tackle the problem of limited samples, a masked autoencoder is used to image augmentation. Moreover, it can be inserted as a plug-and-play module into a few-shot classification. Secondly, the multi-scale attention module can adapt feature vectors extracted by embedding function to the current classification task. Multi-scale attention machine strengthens the discriminative image region by focusing on relating samples in both base class and novel class, which makes prototypes more accurate. In addition, the embedding function pays attention to the task-specific feature. Thirdly, the domain adaptation module is used to address the domain shift caused by the difference in data distributions of the two domains. The domain adaptation module consists of the metric module and the margin loss function. The margin loss pushes different prototypes away from each other in the feature space. Sufficient margin space in feature space improves the generalization performance of the method. The experimental results show the classification accuracy of the proposed method is 67.45% for 5-way 1-shot and 82.77% for 5-way 5-shot on the miniImageNet dataset. The classification accuracy is 70.57% for 5-way 1-shot and 85.10% for 5-way 5-shot on the tieredImageNet dataset. The classification accuracy of our method is better than most previous methods. After dimension reduction and visualization of features by using t-SNE, it can be concluded that domain drift is alleviated, and prototypes are more accurate. The multi-scale attention module enhanced feature representations are more discriminative for the target classification task. In addition, the domain adaptation module improves the generalization ability of the model.
小样本图像识别 注意力机制 领域自适应 相似性度量 few-shot image classification attention mechanism domain adaptation similarity metric
北京大学 薄膜晶体管与先进显示重点实验室, 广东 深圳 518055
采用氢(H)扩散掺杂源漏的方法对自对准顶栅a-IGZO TFT的制备工艺进行了研究。氢的扩散掺杂通过PECVD生长SiNx钝化层而实现。实验结果显示, 在栅电极图形化后, 是否继续进行栅介质刻蚀对器件性能有较大影响。对刻蚀了SiO2栅介质层的器件, 发现其泄漏电流较大, 这可能是由于有源层侧壁的刻蚀残留物导致的; 短沟道器件阈值电压偏负且在经过退火后迁移率减小, 则是由于严重的H横向扩散导致的。对未刻蚀SiO2栅介质层的器件, 发现其阈值电压相对偏正, 应该是因为SiO2栅介质对H的掺杂有一定的阻挡作用, 导致H的横向扩散得到了抑制; 器件在经过退火后迁移率上升, 开态电流增大, 应该是因为未刻蚀栅介质中的H热扩散到下方的源漏区域, 降低了源漏电阻。
非晶铟镓锌氧薄膜晶体管 自对准顶栅 栅介质刻蚀 氢掺杂 a-IGZO TFTs self-aligned top gate gate dielectric etching H-doped