基于卡尔曼预测的光频域反射分布式光纤传感性能提升方法
下载: 523次亮点文章特邀研究论文
Distributed fiber optic sensing technology based on optical frequency domain reflectance (OFDR) has found extensive application in areas such as monitoring the health of structures and measuring temperature/strain in harsh environments. It has proven advantageous due to its ability to provide high spatial resolution, compact design, lightweight nature, and excellent immunity to electromagnetic interference. However, since the backward Rayleigh scattered light used for localization in OFDR is usually weak, the reduction in similarity (SD) between the reference spectrum and the measurement spectrum due to noise can significantly impact the robustness and accuracy of the system's measurements, especially in situations involving long distances, high temperatures, or a significant number of range strains. To address this problem, in this paper, we develop a tuning nonlinearity compensation model for tunable laser sources, finding that the residual tuning nonlinearity may lead to a random position deviation (PoD) for each sensing gauge. Based on the PoD statistical analysis, we build a system for evaluating the SD between the reference and measurement spectra. Combining with Kalman prediction and local search, the proposed method can match the reference and measurement spectra efficiently and accurately, resulting in compensation for the random PoD introduced in the sensing gauge of interest. We hope to extend the sensing range while realizing increased spatial resolution, robustness, and speed.
The research on tuning nonlinearity starts from the schematic diagram of a polarization diversity OFDR system. By examining the origins of its residual tuning nonlinearities, we employ statistical techniques to explore how they impact the PoD in each sensing gauge. The analyses illustrate that the innate noise from the tunable laser, similar to the outer strain or temperature variations, could contribute to the PoD. In particular, because of the statistical portrayal of the residual tuning nonlinearities, the additionally generated PoDs exhibit an approximately standard distribution. Based on this finding, we further design a process based on Kalman filtering (KF) and local search to compensate for the random PoDs from tuning nonlinearities, wherein two judgment conditions (JC1 and JC2) determine whether to enter/break the local search loop. Compared with other post-filtering methods, this method updates the measurement information by satisfying JC1 < TJC1 or minimizing JC2. This procedure is closer to real sensing scenarios and therefore improves the SD. Besides, we start the local search loop from the center (j = ±1) with higher probabilities to the distal (j = ±M) and break the loop once JC1 < TJC1. Thus, the presented strategy could accelerate the search process.
We compare the distributed sensing results recovered by the proposed method with the existing methods (Fig. 5). It is evident that the currently available approaches have limitations in terms of measurement length and strain/temperature measurement range due to the residual tuning nonlinearities. In contrast, the presented method can recover the strain/temperature distributed along the fiber axis without observing outliers, suggesting it can sufficiently compensate for the innate SD degradation due to the residual tuning nonlinearities. In particular, the robustness of the proposed method has a significant advantage when the measured strain or temperature is beyond 5000 με or 300 ℃, respectively. Additional examinations of the PoD random variations caused by the tuning nonlinearities and external stress indicate that the amplitude and range of the former are weaker (Fig. 7), implying that it is typically confined and temporary. The requirement to implement the adaptive judgment conditions JC1 and JC2 is verified in parallel. The distributed fiber optic strain/temperature sensing equipment and its software can achieve a sensing distance of greater than 150 m and a spatial resolution of 5 mm (Fig. 9), and the completion time of a single measurement under the full sensing range and the highest spatial resolution is less than 6 s. The system could measure strains varying from 2000 to 10000 με at about 140 m. A lateral comparison of each curve reveals that the shape of the data sets is similar, and the height of the "platform" is directly proportional to the applied strain. It is evident that the system effectively measures the magnitude and location of the sensing event; a horizontal comparison of the data sets demonstrates that the shape of the data sets is comparable, and the height of the "high platform" is linearly correlated to the applied strain.
In conclusion, the random PoD due to the residual tuning nonlinearities is theoretically verified to decrease the SD between the reference and measurement spectra in OFDR systems. A novel local search and dynamic prediction method based on KF is then proposed. This method can effectively compensate for the random PoD by local search and accelerate the search process by the KF prediction. Experiments show that the proposed method can significantly improve the robustness of the sensing system under the limited range (temperature of 450 ℃ and strain of 10000 με) sensing application. Moreover, it can compress the computation to 5.8%-28.6% of that without dynamic prediction operations.
1 引言
近年来,基于光频域反射(OFDR)原理的分布式光纤传感技术已经广泛应用于结构健康监测、恶劣环境下的温度和应变测量等场景,并展示出了空间分辨率高、结构紧凑、轻量化和抗电磁干扰性良好等优点[1-2]。与弱反射光纤光栅阵列的逐点访问相比,单模光纤中的后向瑞利散射为光纤自有的特性,不依赖于特定位置的折射率调制,这使得基于后向瑞利散射的OFDR系统能够实现无盲区的分布式传感[3-5]。但是,由于后向瑞利散射光的强度较光纤光栅反射更加微弱,噪声作用下参考光谱和测量光谱间的相似度(SD)的退化将显著影响系统测量的鲁棒性和精度,特别是在长距离、高温或大量程应变的测试场景中,这一问题将变得更加严峻[6-9]。
目前,国内外针对参考光谱和测量光谱间的SD退化问题已经开展了一系列研究。例如,Feng等[7]发现,大应变下,参考光谱和测量光谱之间交叠比例的减少将显著影响互相关结果的信噪比(SNR)及系统的测量范围,而如果从参考光谱和测量光谱中提取更相似的局部指纹特征,再进行互相关处理,则能够使SD提高4倍,进而实现超过3000 µε的应变测量范围及3 mm的空间分辨率。之后,Luo等[8]分析了光热、光弹效应造成的光学路径位置偏移(PoD)以及由此导致的SD退化。通过迭代更新的方式,他们实现了对PoD累积效应的补偿和瑞利光谱互相关SD的提高,其装置在25 m的待测光纤上实现了0.5 mm的空间分辨率和高达2500 µε、500 ℃的测量范围。在此基础上,Li等[9]通过结合PoD补偿与图像小波去噪的方式进一步寻找了互相关SD最高的位置,其系统在~20 m位置处实现了200 µε到2000 µε范围内的应变测量,且精度从±80 µε提高到±20 µε。总体上,这些研究重点关注的是外部应变、温度变化引起的SD退化,而对于OFDR系统的内源性因素,如可调谐激光源(TLS)输出特性非理想所导致的SD退化,则没有足够重视[10-13]。
在上述研究背景下,本文研究了可调谐激光光源调谐非线性补偿模型,发现补偿的残余误差会引起传感单元的随机位置偏差,并采用统计方法研究了其分布特征。为减小残余调谐非线性造成的不利影响,提出了基于卡尔曼滤波的光谱互相关SD局部寻优策略,以实现测量光谱和参考光谱的最相似位置匹配;在此基础上,根据卡尔曼滤波的动态预测和误差估计对SD局部寻优过程进行了加速。实验结果表明,相较于Luo等的算法未能考虑残余调谐非线性,本文提出的方法能够有效补偿调谐非线性残余误差引起的PoD波动,进而提高参考光谱和测量光谱之间的SD;同时,利用动态预测策略可使SD局部寻优的运算量降低到原来的5.8%~28.6%。在50 m长度的被测光纤(FUT)上,该方法实现了测量范围(最高温度~450 ℃,最大应变~10000 με)、鲁棒性和解调效率的提升。
2 基本原理(实验仿真)
2.1 调谐非线性补偿残余误差及其统计特征分析
式中:
式中,非负整数
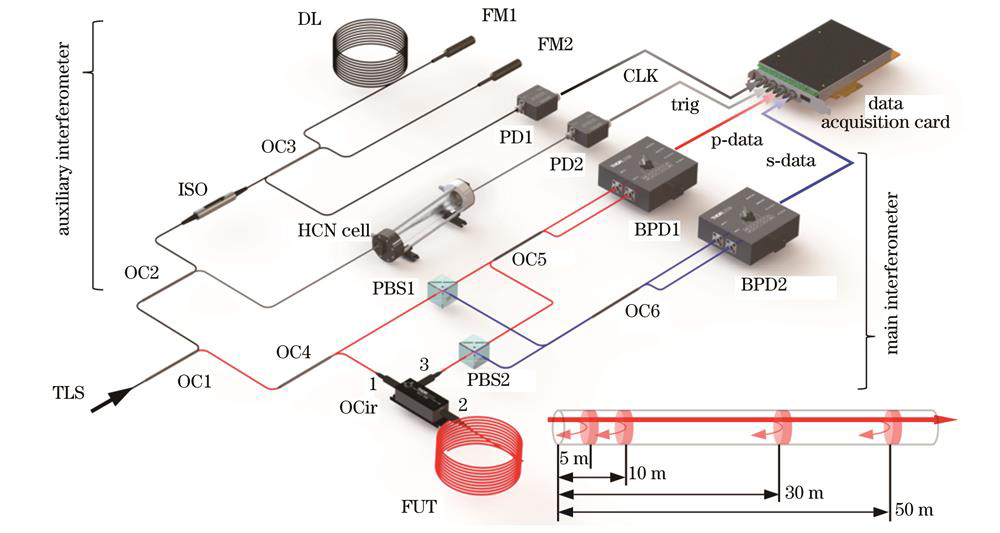
图 1. 典型偏振分集OFDR系统的原理示意图
Fig. 1. Schematic diagram of typical polarization diversity OFDR system
由
扫频过程中TLS输出光束的相位
根据
图
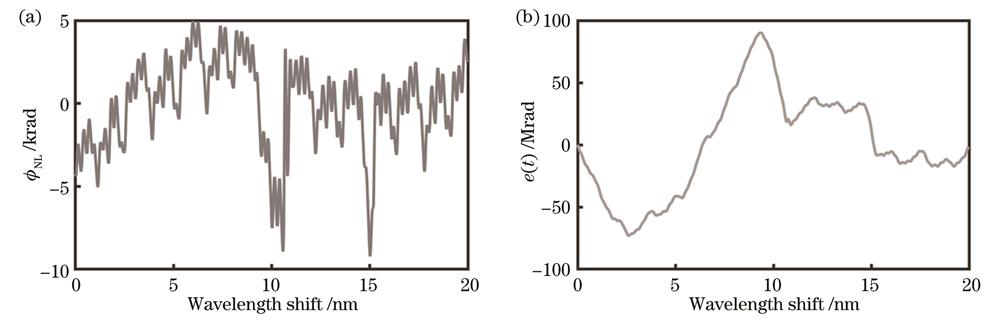
图 2. TLS调谐非线性的分析。(a)相干探测信号的非线性相位;(b)TLS输出光束的非线性相位
Fig. 2. Analysis of TLS tuning nonlinearity. (a) Nonlinear phase of coherent detection signal; (b) nonlinear phase of TLS output beam
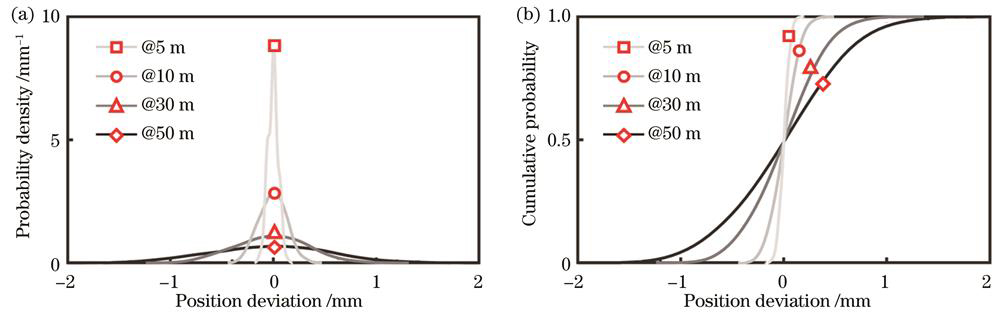
图 3. 频率采样误差作用下,不同位置传感单元的PoD统计结果。(a)概率密度函数;(b)累积分布函数
Fig. 3. PoD fluctuations of sensing gauges at different positions induced by frequency sampling error. (a) Probability density function; (b) cumulative distribution function
横向对比PoD分布的概率密度函数和累积分布函数,可发现各区间PoD的波动展示出一定的随机性,且离散的程度随时延的增加而增加(当感兴趣的传感单元从5 m位置处变化到50 m位置处时,位置测量的95%置信区间从~0.2 mm变化到~2.2 mm)。这表明频率采样的残余误差产生了一个内源性的PoD变化,将会对需要远距离、高空间分辨率的分布式传感应用产生显著影响。
2.2 基于卡尔曼滤波的局部寻优方法
为补偿频率采样误差造成的PoD波动,对
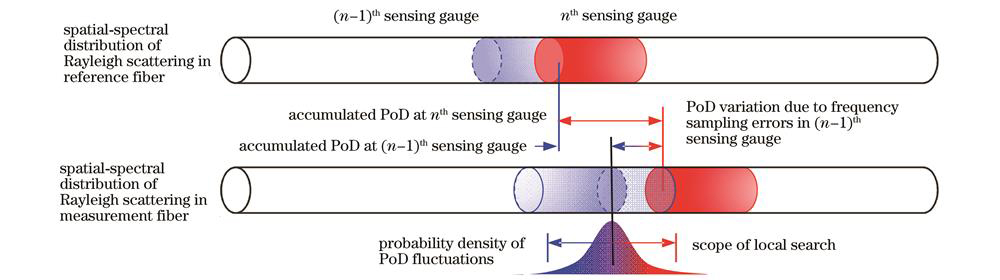
图 4. 通过局部寻优补偿PoD累积的原理示意图
Fig. 4. Schematic diagram of compensation of accumulated PoD through local search
通常,SD局部寻优算法涉及大量的傅里叶变换、傅里叶逆变换和互相关运算,将产生沉重的运算负担并显著影响系统的时效性。针对这一问题,进一步根据PoD波动的特点设计SD局部寻优的动态预测策略。考虑从第(n-1)级传感单元到第n级传感单元的PoD累积量更新,可以构建卡尔曼滤波器的预测方程[17]:
以及更新方程
式中:
预测结果
表 1. 卡尔曼动态预测局部寻优位置的伪代码
Table 1. Pseudo-code for Kalman dynamic prediction of local search location
|
3 实验结果与讨论
根据
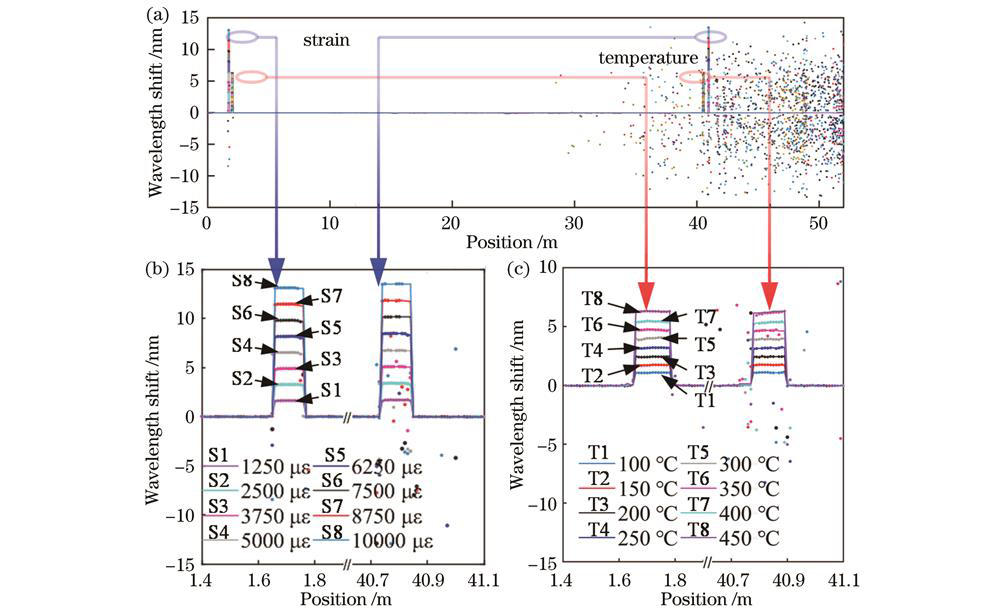
图 5. 应变和温度的分布式传感结果。(a)应变和温度的全局分布;(b)应变传感单元;(c)温度传感单元
Fig. 5. Distributed sensing results of strain and temperature. (a) Global distributions of strain and temperature; (b) sensing gauge of strain; (c) sensing gauge of temperature
在此基础上,

图 7. 应变为5000 με时FUT轴向PoD的估计
Fig. 7. Estimation of axial PoD along FUT when strain is 5000 με
最后,比较采用与未采用卡尔曼动态预测的局部寻优效率。采用每组20次温度和应变传感数据分别利用传统数据解调方法和卡尔曼滤波动态预测数据解调方法进行测试,并得到互相关运算的操作数量的平均值。如
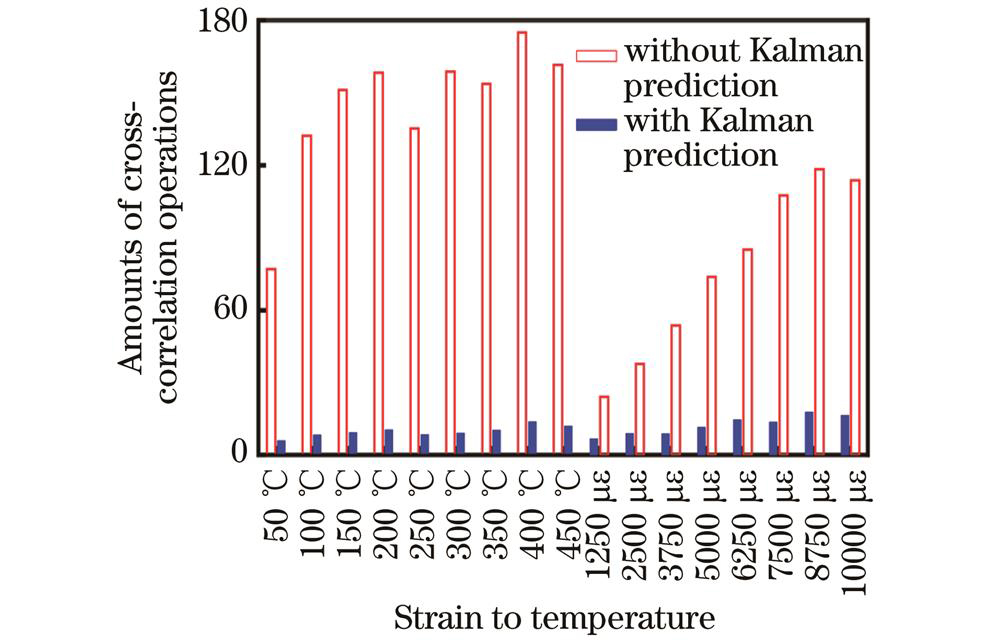
图 8. 采用/不采用卡尔曼预测时局部寻优的互相关运算量
Fig. 8. Amounts of cross-correlation operations in local search process with and without Kalman prediction
在以上理论研究的基础上,研制了如

图 9. 提出OFDR系统的集成,(a)装置实物图;(b)应变测量结果
Fig. 9. Integration of proposed OFDR system. (a) Physical diagram of configuration; (b) results of strain measurement
4 结论
本文通过研究可调谐激光光源调谐非线性补偿模型,发现补偿残余误差会引起传感单元的随机位置偏差及降低参考光谱和测量光谱之间的SD,并采用统计方法研究了其分布特征。在此基础上,提出了一种基于卡尔曼预测和局部寻优的传感单元随机位置偏差补偿方法。一方面,通过局部寻找参考光谱和测量光谱的最相似位置以确定所分析传感单元的PoD变化;另一方面,构建了相似度评价体系和自适应判据,并利用卡尔曼预测加速最相似位置的搜索过程。实验结果表明,本文提出的方法能够在50 m长度的FUT上拓展OFDR分布式传感的测量范围(空间分辨率为5 mm,最高温度为450 ℃,最大应变为10000 με),并能够大幅度提升其鲁棒性和处理速率。
[1] Bao X Y, Chen L. Recent progress in distributed fiber optic sensors[J]. Sensors, 2012, 12(7): 8601-8639.
[2] Zhang P, Tang M, Gao F, et al. Simplified hollow-core fiber-based Fabry-Perot interferometer with modified vernier effect for highly sensitive high-temperature measurement[J]. IEEE Photonics Journal, 2015, 7(1): 7100210.
[3] 王紫超, 刘志昂, 杨华东. 光频域反射与超弱光纤布拉格光栅技术的结构监测性能[J]. 激光与光电子学进展, 2022, 59(21): 2106002.
[4] 尹国路, 徐州, 朱涛. 光纤形状传感器封装过程中残余应力的分布式实时监测[J]. 光学学报, 2022, 42(16): 1606002.
[5] 孙琪真, 李豪, 范存政, 等. 基于散射增强光纤的分布式声波传感研究进展[J]. 激光与光电子学进展, 2022, 59(21): 2100001.
[6] Froggatt M, Moore J. High-spatial-resolution distributed strain measurement in optical fiber with Rayleigh scatter[J]. Applied Optics, 1998, 37(10): 1735-1740.
[7] Feng K P, Cui J W, Jiang D, et al. Improvement of the strain measurable range of an OFDR based on local similar characteristics of a Rayleigh scattering spectrum[J]. Optics Letters, 2018, 43(14): 3293-3296.
[8] Luo M M, Liu J F, Tang C J, et al. 0.5 mm spatial resolution distributed fiber temperature and strain sensor with position-deviation compensation based on OFDR[J]. Optics Express, 2019, 27(24): 35823-35829.
[9] Li P F, Fu C L, Du B, et al. High-spatial-resolution strain sensor based on distance compensation and image wavelet denoising method in OFDR[J]. Journal of Lightwave Technology, 2021, 39(19): 6334-6339.
[10] Moore E D, McLeod R R. Correction of sampling errors due to laser tuning rate fluctuations in swept-wavelength interferometry[J]. Optics Express, 2008, 16(17): 13139-13149.
[11] Dang H, Tian Y Q, Liu H H, et al. Dynamic wavelength calibration based on synchrosqueezed wavelet transform[J]. Optics Express, 2022, 30(26): 46722-46733.
[12] 戴建平, 邱锦波, 刘宏睿, 等. 基于内调制DFB激光器的高空间分辨率TGD-OFDR[J]. 光学学报, 2023, 43(7): 0728001.
[13] 付彩玲, 彭振威, 李朋飞, 等. OFDR分布式光纤温度/应变/形状传感研究进展[J]. 激光与光电子学进展, 2023, 60(11): 1106007.
[14] Du Y, Liu T G, Ding Z Y, et al. Method for improving spatial resolution and amplitude by optimized deskew filter in long-range OFDR[J]. IEEE Photonics Journal, 2014, 6(5): 7902811.
[15] Fang Z, Liang C S, Xu S W, et al. Spatial resolution enhancement of OFDR sensing system using phase-domain-interpolation resampling method[J]. IEEE Sensors Journal, 2022, 22(4): 3202-3210.
[16] Dang H, Liu H H, Cheng L Q, et al. Deconvolutional suppression of resolution degradation in coherent optical spectrum analyzer[J]. Journal of Lightwave Technology, 2023, 41(13): 4430-4436.
[17] LiQ, LiR Y, JiK F, et al. Kalman filter and its application[C]//2015 8th International Conference on Intelligent Networks and Intelligent Systems (ICINIS), November 1-3, 2015, Tianjin, China. New York: IEEE Press, 2016: 74-77.
党竑, 马彬, 高超, 祖文龙, 程琳淇, 陈金娜, 刘奂奂, 冯昆鹏, 张旭苹, 沈平. 基于卡尔曼预测的光频域反射分布式光纤传感性能提升方法[J]. 光学学报, 2024, 44(1): 0106016. Hong Dang, Bin Ma, Chao Gao, Wenlong Zu, Linqi Cheng, Jinna Chen, Huanhuan Liu, Kunpeng Feng, Xuping Zhang, Ping Shen. Performance Enhancement Method of Optical Frequency Domain Reflection Distributed Fiber Sensing Based on Kalman Prediction[J]. Acta Optica Sinica, 2024, 44(1): 0106016.