Imaging through dynamic scattering media with stitched speckle patterns
Download: 810次
Seeing through scattering media is highly desired in many occasions, such as remote sensing through clouds, fog, and haze; underwater detection; anti-terrorism surveillance; biological tissue imaging; and clinical diagnosis. However, multiple scattering experienced by photons when propagating in scattering media breaks the point-to-point correspondence required by traditional imaging methods[1,2]. In order to solve the problem, people developed many techniques including time gating[35" target="_self" style="display: inline;">–
In speckle autocorrelation imaging, the autocorrelation of a speckle pattern consists of an autocorrelation of the object with a magnification factor determined by the experimental setup and a random noise background, which leads to a disturbed frequency spectrum of the object and sequentially a degraded reconstruction quality. This issue can be tackled by capturing a speckle pattern with a large sampling ratio
In experiment, for a small
In this Letter, by utilizing the equivalence between the spatial and temporal ensemble average of speckles[2], we propose a simple and fast method for imaging through dynamic scattering media with a stitched speckle pattern. In particular, we acquire many speckle patterns with different point spread functions in sequence with a small sensor, stitch them together to get a big speckle pattern, and then reconstruct the image from the stitched pattern. In this way we can reconstruct the image well even when the sensor area is insufficient for a large image distance without any need for hardware upgrade. Compared to purely averaging (or summing) the autocorrelation, the stitching method can suppress the background noise and simultaneously increase the resolution of the power spectrum for more details, which is crucial in applications like remote sensing.
In the following, we first show the equivalence between the stitched speckle pattern and the one captured with a large camera sensor. Then we experimentally demonstrate the improvement of image quality with increased stitching number compared to pure averaging. Last, we perform simulations to study the trend of image quality with stitching number and the theoretical lower threshold of
The ME is the prerequisite of speckle autocorrelation imaging, which essentially demonstrates that speckle patterns generated by different points within the ME range are shifted but highly correlated[31,32]. When an object hidden behind the scattering medium (within the ME range) is illuminated by a spatially incoherent light source, the corresponding speckle pattern on the camera can be seen as a superposition of speckle patterns generated by all points of the object[33]. In other words, the speckle pattern is a convolution of the object intensity and the point spread function (PSF), i.e.,
According to the Wiener–Khinchin theorem, the power spectrum of an object is equal to the Fourier transform of its autocorrelation,
Then we can reconstruct the image with a phase retrieval algorithm[27,34,35].
Considering an imaging system with a detector of a large enough area, the PSF can be divided into
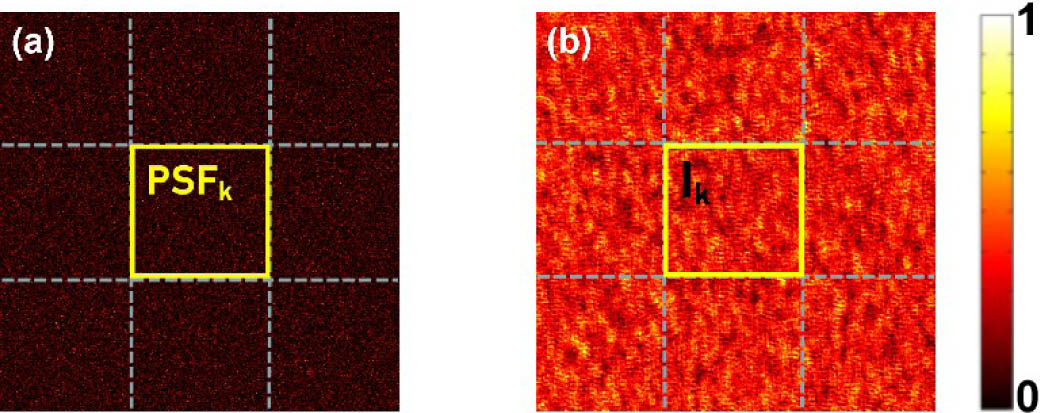
Fig. 1. Schematic illustration of stitching. (a) A big speckle pattern is split into many subblocks, and each subblock has its own independent . In other words, the big speckle pattern is obtained by stitching all independent subblocks together. (b) The segmentation of a big speckle pattern .
For a dynamic scattering medium, here, only Brownian motion is considered, the PSF varies with time and the intensity fluctuation is ergodic in both spatial and temporal domains[2], and the decorrelation time
![Diagram of piecing together a series of speckle patterns {Itk}k=1n×n [shown as subfigure (a)] into (b) a big speckle pattern; the yellow square frame denotes a single speckle pattern Itk.](/richHtml/col/2020/18/4/042604/img_002.jpg)
Fig. 2. Diagram of piecing together a series of speckle patterns [shown as subfigure (a)] into (b) a big speckle pattern; the yellow square frame denotes a single speckle pattern .
According to Eqs. (
Then the object can be reconstructed by an iterative phase-retrieval algorithm[27]. This method increases the detection area digitally by stitching small speckle patterns together into a large one, and thus is especially suitable for the conditions with a small
A schematic experimental setup is shown in Fig.
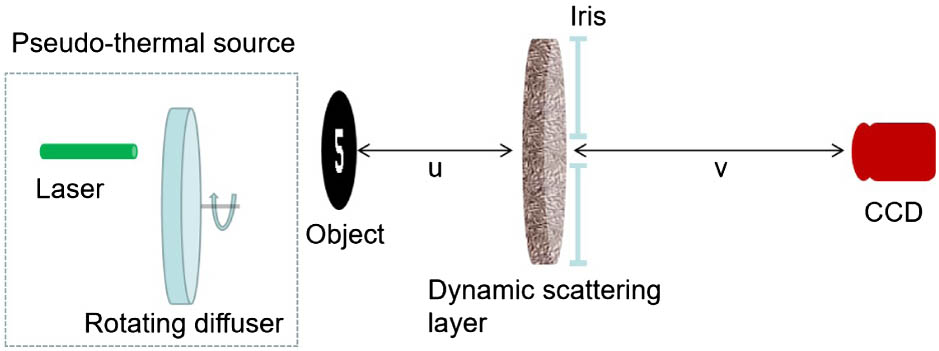
Fig. 3. Experimental setup. and are distances from the object and the camera to the scattering layer, respectively.

Fig. 4. Experimental results. Rows I, II, and III correspond to , 5.4, and 3.6, respectively. (a) Examples of captured speckle patterns, and (b) the corresponding autocorrelations of (a). (c) The power spectra of (a), (d) the averaged power spectra, and (e) the images reconstructed from (d). (f) The stitched speckle patterns at different sampling ratios and numbers of frames: , frames (row I); , frames (row II); and , frames (row III), respectively. (g) The corresponding power spectra of (f), and (h) the images reconstructed from (g). (II,i) The autocorrelation and (III,i) the frequency spectrum of (I,i), the object. The inserts in rows II and III, and columns (c), (d), and (h) show zoom-ins of the center dash squares.
Figure
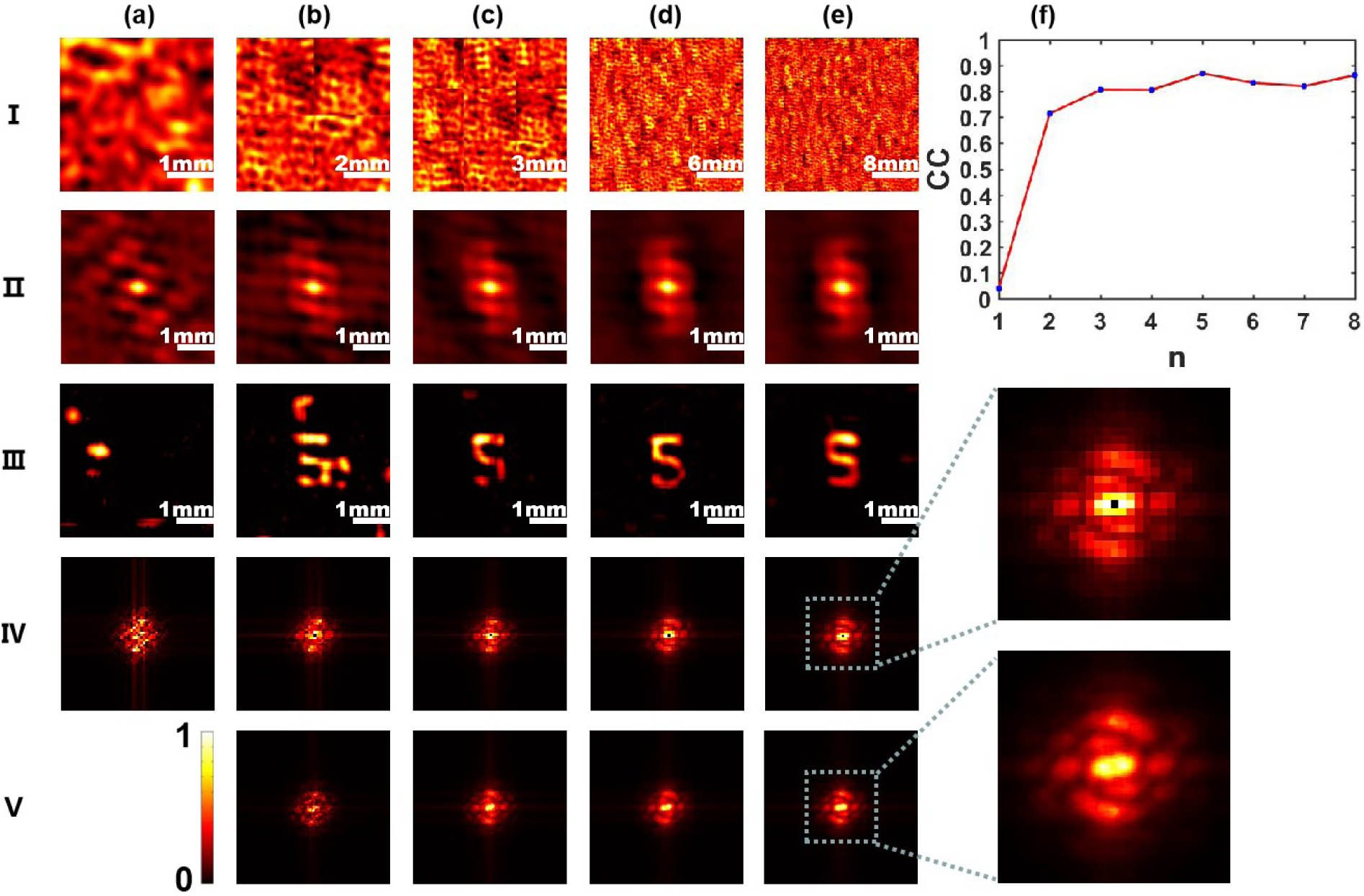
Fig. 5. Simulation results of different stitching numbers at . Row I. (a) A single original speckle pattern; and (b) 4, (c) 9, (d) 36, and (e) 64 original patterns stitched together equally in two directions. Row II. The autocorrelations corresponding to I. Row III. The respective images reconstructed. Row IV. The averaged power spectra of 1, 4, 9, 36, and 64 frames of the original patterns. Row V. The corresponding power spectra of row I. (f) The evolution of the correlation coefficient with stitching number . In rows IV and V, all have the same spectrum range because of the same pixel pitch. However, the power spectra of the stitched patterns have a higher resolution determined by the array size, which can be seen clearly from the amplified dash squares.
Next, we investigated the relationship between the image quality and
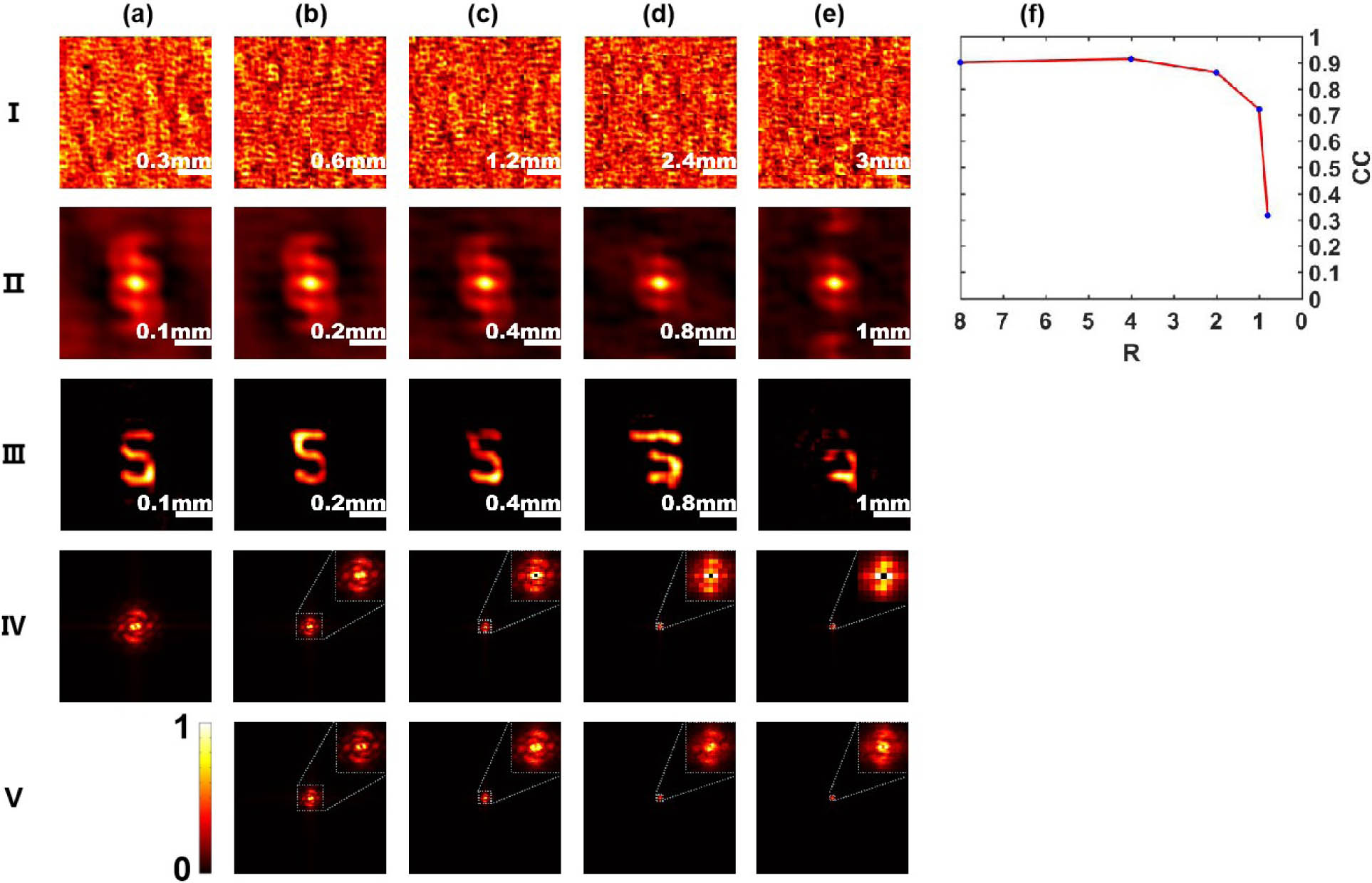
Fig. 6. Simulation results of different sampling ratios . Row I. (a) A speckle pattern at , and stitched speckle patterns of different sampling ratios and numbers of frames: (b) , frames; (c) , frames; (d) , frames; and (e) , frames, respectively. Row II. Autocorrelations corresponding to I. Row III. Reconstructed images, respectively. Row IV. Averaged power spectra. Row V. Corresponding power spectra of I (b)–(e). (f) The evolution of the correlation coefficient versus sampling ratio . Again, all power spectra in rows IV and V have the same spectral range but different resolutions.
In Fig.
In this Letter, we should keep in mind that in the experiment the exposure time of the CCD camera should be less than the decorrelation time, and the speckle recording must comply with the Nyquist sampling law. In the above experiments and simulations, each reconstruction is carried out under the same number of iterations of the phase retrieval algorithm. Due to the randomness caused by phase retrieval algorithm, the image quality could fluctuate slightly. Because of the background noise, the actual stitching number required in the experiment is always larger than that in the simulation to achieve a comparable reconstruction quality under the same sampling ratio. Compared to simple averaging, stitching can suppress the background noise, and simultaneously enhance the resolution in the frequency domain to see more details, which is critical to reconstruct the image, especially for a small sampling ratio
Different from solid bodies like rotating ground glass disks, white paint, tissue mimicking phantoms, and biological tissues, the decorrelation time of fog and polluted water is orders-of-magnitude smaller, with a characteristic time of microseconds[36]. By utilizing the shorter decorrelation time, an automatic stitching speckle pattern can be realized by scanning over an array of cheap industrial CCD cameras, which is competitive in cost, dynamic range, and extensibility compared to a billion-pixel camera. It provides an alternative real-time imaging solution. In addition, the stitching method can also apply to a moving object, as long as the object is approximately stationary within each exposure time and the object itself is within the memory effect range.
In conclusion, we reveal a relationship between detector and object sizes for the minimum requirement to ensure image reconstruction by defining a sampling ratio
[1]
[3]
[4]
[5]
[6]
[7]
[8]
[9]
[10]
[11]
[12]
[13]
[14]
[15]
[16]
[17]
[18]
[19]
[20]
[21]
[22]
[23]
[24]
[25]
[26]
[27]
[28]
[29]
[30]
[31]
[32]
[33]
[34]
[35]
[36]
Xin Wang, Honglin Liu, Meijun Chen, Zhentao Liu, Shensheng Han. Imaging through dynamic scattering media with stitched speckle patterns[J]. Chinese Optics Letters, 2020, 18(4): 042604.