考虑时间权重的可调谐滤波器温漂补偿方法
Fiber Fabry-Perot tunable filters (FFP-TF) controlled by piezoelectric ceramics are prone to temperature drift in fiber Bragg grating (FBG) sensing systems. During the long-term measurement process, FFP-TF will cause continuous drift of the output wavelength, which will adversely damage the FBG sensing system's measurement accuracy. At the moment, FFP-TF temperature drift compensation primarily entails adding hardware calibration modules to the FBG sensing system, such as the reference grating method, F-P etalon method, gas absorption method, and composite wavelength reference method. Although these technologies can efficiently adjust for temperature drift, they greatly increase the system's cost and complexity. As a result, utilizing software approaches to compensate for temperature drift in FFP-TF is a practical and low-cost method. However, most contemporary temperature drift compensation approaches based on artificial intelligence technologies neglect temperature drift data's temporal features. In fact, the fresh sample has a higher impact on the prediction outcomes of the following data than the old sample. As a result, this work extensively addresses the impact of temporal features on temperature drift compensation when processing temperature drift and other highly time-dependent data. A tunable filter temperature drift compensation approach with time weight is suggested based on the AdaBoost-SVM algorithm and time weight.
We use FBG0 as the reference grating and the other three FBGs as sensing gratings, and each sensing grating is modeled individually. The temperature-related values of the experimental environment are chosen as the model's input features in this investigation. Furthermore, because the wavelength drift errors of each FBG in the FFP-TF output spectrum have a high correlation, we use the drift of the reference grating as an input feature of the dynamic compensation model to compensate for the lack of accurate temperature information in the F-P cavity. The significant link between the temperature drift sequence data before and after is taken into account in full by this investigation. The idea of time weight is introduced in the process of modeling the temperature drift of FFP-TF to assign various temporal attributes to each sample. After that, temperature drift samples are modeled using support vector machines (SVM) as weak learners, and several SVM learning models are integrated using the AdaBoost framework. In the integrated prediction process, the time attribute of samples has an impact on the update of sample weights in addition to the prediction performance of each model. Multiple temperature change modes have been used to validate the aforementioned procedure.
First, the temperature drift compensation results of the proposed algorithm are compared with the conventional AdaBoost-SVM algorithm for three transmission gratings in the 2 ℃ narrow changing temperature environment experiment of cooling and heating (Table 3). Secondly, in the 15 ℃ cooling amplitude experiment, the temperature drift compensation results of the proposed algorithm are compared with the traditional AdaBoost-SVM algorithm for three transmission gratings. The experimental results show that the maximum temperature drift compensation error of the traditional AdaBoost-SVM algorithm is 10.83 pm, while the maximum temperature drift compensation error of the AdaBoost-SVM based on time weight is reduced to 7.04 pm. The results show that the classic AdaBoost-SVM algorithm's maximum error is approximately 11.57 pm, whereas the maximum error of the AdaBoost-SVM based on time weight is only approximately 4.05 pm. The strategy suggested in this research, however, outperforms unoptimized machine learning methods in terms of superior stability, stronger reliability, and higher prediction accuracy (Table 4). The aforementioned findings show that the method suggested in this article may successfully determine samples' temporal properties, allowing for more reasonable sample weight allocation, a decrease in model performance fluctuations, and an increase in model accuracy.
First, the high link between the temperature drift sequence data before and after is thoroughly taken into account in this article. The ratio of new and old samples is altered by applying various new weights at various time points, which makes the distribution of sample weights more logical and enhances the model's performance. The experiment next establishes a nonlinear model between the filter surface temperature and output drift error using the spectral locations of three reference gratings as input features. Experiments are carried out on two datasets with different temperature change patterns, and the results reveal that the first dataset does not fully comply with the more important rule of closer samples in general time series proposed in this article due to the short-term fluctuation of temperature changes, so the performance improvement of the model is not significant; the temperature change in the second dataset demonstrates a monotonic cooling trend with apparent gradients, which is more consistent with the more important principles of closer samples, and the performance gain is more significant. Unlike typical hardware techniques, the method suggested in this paper does not require any additional hardware, resulting in a novel approach to temperature drift compensation of tunable filters.
1 引言
光纤布拉格光栅(FBG)具有体积小、质量轻、成本低、性能优异与光学系统兼容性好等优点,已经在光纤通信和光纤传感领域得到了越来越广泛的应用[1-2]。光纤法布里-珀罗可调滤波器(FFP-TF)是一种高灵敏度的波长解调器件,常被用于FBG传感系统的信号解调[3-4],其基本原理是根据压电陶瓷(PZT)在电场的激励下产生逆压电效应来调整FFP-TF的腔长,使得特定波长的光以最大透射率通过FFP-TF[5-6]。FFP-TF在温变环境中输出波长会产生漂移,这是因为温度变化影响PZT材料的弹性模量和压电系数,进而导致FFP-TF输出波长随着环境温度变化而发生持续漂移[7]。
当前,为了修正FFP-TF的漂移误差,研究人员提出了多种方法对FFP-TF的真实中心波长进行标定,主要有FBG参考光栅法[8]、F-P标准具法[9-10]、气体吸收法[11]和复合波长参考法[12-13]。但利用额外的硬件模块来对波长进行标定会大幅提高系统的经济成本,也使得解调系统变得更加复杂。近年来,随着人工智能技术的飞速发展,利用软件模拟方法对FFP-TF进行温漂补偿成为一种可行且成本低的方法。2014年,Cheng等[14]提出一种基于粒子群优化支持向量机(SVM)的多温度变量建模方法,其采用温度作为模型的输入特征。2016年,Shen等[15]提出一种基于遗传算法和埃尔曼神经网络的多输入模型,该模型采用温度和温度变化率等温度相关参数作为输入特征。近年来,本课题组先后提出了基于集成窗口的最小二乘支持向量机(LSSVM)[16]、具有不对称噪声区间的自适应权重LSSVM[17]、基于误差率差值更新弱学习器权重的AdaBoost算法[18]和多参考光栅作为模型特征的方法[19],以准确补偿FFP-TF的温漂。经典AdaBoost预测算法在确定当前弱学习器权重系数时仅仅依据误差率,忽略了弱学习器之间的相互关系,在弱学习器权重分配上存在不足。因此,文献[18]通过对比当前弱学习器与上次迭代生成弱学习器的误差率,计算权重更新系数,然后根据该系数更新当前弱学习器的权重,降低迭代的随机性,提高弱学习器的集成效率。但是,文献[18]仅对AdaBoost算法的结构进行改进,并未考虑样本本身的时间特性。当前大部分基于人工智能技术的温漂补偿方法都忽略了温漂数据的时间特性。实际上,相比于旧样本,新样本对后续数据的预测结果影响较大,换言之,在处理温漂这类与时间高度相关的数据时,应该在实验研究中充分考虑时间特性对温漂补偿的影响。因此,本文提出一种考虑时间权重的AdaBoost-SVM算法,按照样本的时间顺序,将不同的权重赋予温漂数据中的各个样本。所提算法中,样本的时间顺序和弱学习器的预测准确度共同决定样本权重的更新,因此所提算法对FFP-TF的温漂建模具有更好的适应性。
2 基于时间权重的AdaBoost-SVM算法
AdaBoost是一种集成学习算法,它能根据弱学习器的性能赋予权重,并通过加权组合弱学习器来提升整体性能。在使用AdaBoost算法对FFP-TF进行温漂补偿时,由于支持向量机相比于传统的机器学习方法具有更好的泛化能力,并且在引入核函数后能较好地应对温漂补偿的非线性问题,因此本研究采用SVM作为弱学习器。在建立模型的过程中,先通过比较弱学习器预测误差与阈值来确定预测结果,再根据预测结果来更新样本权重与弱学习器权重,并对弱学习器进行加权集成,最终形成一个具有更高精度的强学习器。
传统AdaBoost-SVM算法能够根据预测结果的好坏更新样本权重,并且对多次预测结果进行集成。但是,对于FFP-TF的温度漂移数据,不能仅根据预测结果来确定样本权重。在时间序列数据的建模过程中,新样本距离测试数据近,参考价值大,在建模过程中应该获得较大的权重。相反地,旧样本距离测试数据远,参考价值小,在建模过程中应该获得较小的权重。因此,本文在AdaBoost算法中引入Klinkenberg[20]提出的时间加权方法来解决此类问题,对新旧样本进行区分,样本权重由时间权重和弱学习器预测精度共同决定,这不仅使新样本在训练时具有更大的权重,同时也在迭代过程中增加低预测精度新样本的权重,降低高预测精度新样本的权重。温漂数据样本的时间权重赋予方式为
式中:
所提算法的流程如下:
1)初始化输入带有权重的训练数据集
2)对每一轮迭代,
3)使用当前带有权重
式中:
4)根据错误率,按照
5)对样本赋予时间权重
6)按
式中:
7)最终输出强学习器
3 实验结果与分析
3.1 数据获取
本实验是在基于FFP-TF的FBG解调系统上进行的,该系统主要由光源、耦合器、FBG、FFP-TF、光电探测器、数据采集卡和计算机组成,其工作原理如
用放大自发辐射(ASE)光源输出宽带光,该输出光通过3 dB耦合器耦合进入4个FBG。光电探测器用于FBG反射光谱测量,并将光强信号转换成电压幅值,以计算其特征波长。数据采集卡接收电压信号,并输出1 Hz的锯齿波电压(2~4.5 V)来驱动FFP-TF,在每个扫描周期测量出FBG的反射波峰,在调谐期间的不同时刻可以检测到每个FBG的反射峰。将所有FBG浸入提供稳定环境(18 ℃)的恒温水箱中,使FBG处于相同的环境。将FFP-TF放置在温箱中,在其表面贴有校准热敏电阻,用于读取温度数值。在本实验中采用ESPEC公司的GSH-24V温箱,热敏电阻温度传感器选择测温准确度为±0.001 ℃的Fluke5641。利用安捷伦公司的高分辨率光波分析仪(HP8164B)来确定4个FBG的初始中心波长,其值如
表 1. FBG的特征波长
Table 1. Characteristic wavelengths of FBGs
|
3.2 实验与结果分析
为了验证所提算法的有效性,运用所提算法对FBG温漂数据进行建模并补偿。采用参考光栅法,选取FBG0作为参考光栅,其余3个FBG作为传感光栅,并对3个传感光栅分别进行建模。由于FFP-TF的温漂结果并不仅仅由温度决定,也受到驱动电压的影响,3个传感光栅在光谱中的位置不同,对应在锯齿波驱动电压中,扫描到该光栅的驱动电压也会不同,因此3个传感光栅的温漂数据是不相同的。温箱的温度在开始时从27.6 ℃降低到25.6 ℃,然后增加到27.1 ℃。数据集共有1260个波长漂移样本点,如
式中:
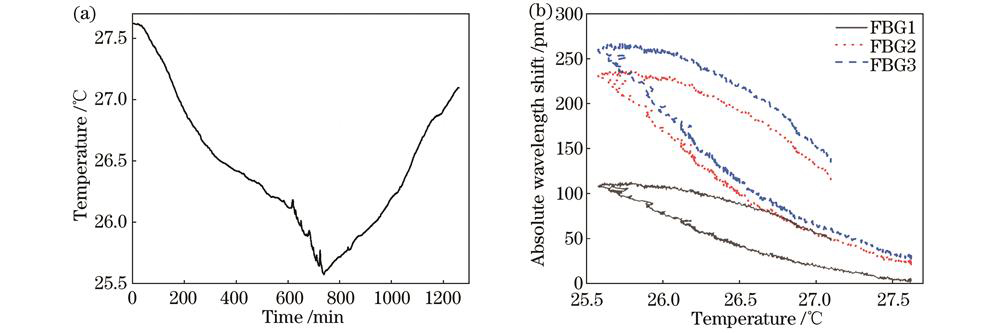
图 2. FBG的温度测量与温度漂移。(a)滤波器表面温度;(b)3个传感FBG的绝对波长漂移
Fig. 2. Temperature measurement and temperature drift of FBG. (a) Filter surface temperature; (b) absolute wavelength drift of three sensing FBGs
因为FFP-TF的应用都处于长期变温环境中,所以其样本特征总是随时间而变化,即温漂数据中存在概念漂移现象。采用滑动窗口并使用SVM模型来检验时间序列中的概念漂移现象。首先,设定最大预测步数
表 2. FBG时间序列概念漂移的检验结果
Table 2. Test results of concept drift of FBGs time series
|
在验证完温漂数据后,采用引入时间权重的AdaBoost-SVM算法(ADASVM-TW)对训练数据进行建模训练,并使用测试数据对所建立的模型进行测试评估。同时将该算法与传统AdaBoost-SVM算法进行对比,两种算法的补偿结果如

图 3. AdaBoost-SVM与ADASVM-TW算法的波动补偿结果。(a)FBG1;(b)FBG2;(c)FBG3
Fig. 3. Fluctuation compensation results of AdaBoost-SVM and ADASVM-TW algorithms. (a) FBG1; (b) FBG2; (c) FBG3
表 3. ADASVM-TW与AdaBoost-SVM算法的评价指标对比
Table 3. Comparison of evaluation indicators between ADASVM-TW and AdaBoost-SVM algorithms
|
由
一些常见的CART回归树、SVM模型、随机森林(RF)模型也可以用于FFP-TF的温度漂移建模,将这些算法与所提出的ADASVM-TW算法进行比较,以传感光栅FBG1为实验组,其补偿结果如
表 4. 不同算法结果统计
Table 4. Statistics results of different algorithms
|
由
为了验证所提算法的有效性,在更宽的温度范围内进行温漂补偿实验。将FFT-TF的工作环境温度升到38 ℃,随后自然冷却至23 ℃,温度变化曲线如
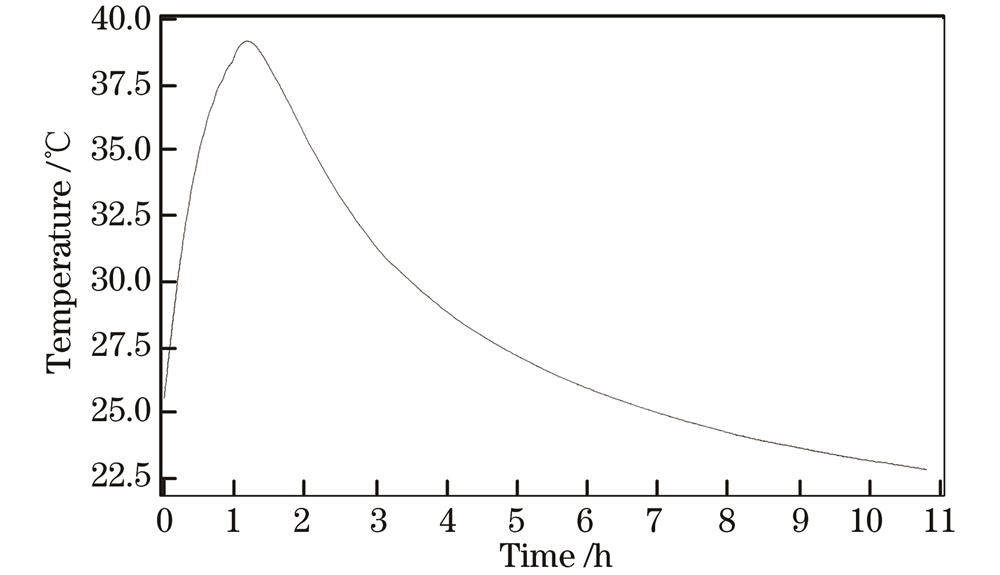
图 5. FFP滤波器的表面温度在自然降温过程中随时间的变化曲线
Fig. 5. Surface temperature variation curve of FFP filter with time during natural cooling
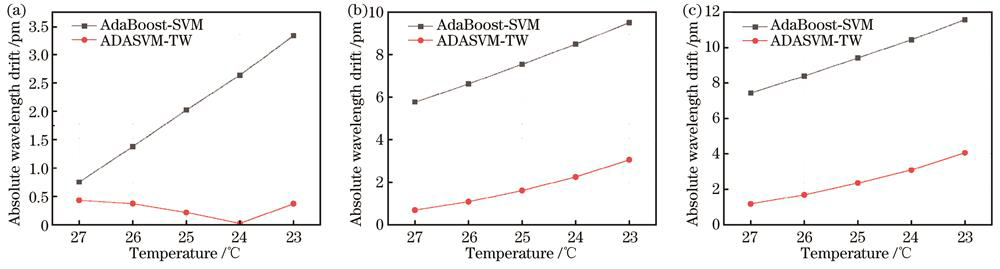
图 6. AdaBoost-SVM与ADASVM-TW算法的补偿结果比较。(a)FBG1;(b)FBG2;(c)FBG3
Fig. 6. Compensation results of AdaBoost-SVM and ADASVM-TW in free cooling process. (a) FBG1; (b) FBG2; (c) FBG3
表 5. ADASVM-TW与AdaBoost-SVM算法的评价指标对比
Table 5. Comparison of evaluation indicators between ADASVM-TW and AdaBoost-SVM algorithms
|
4 结论
为了对FFP-TF进行温漂补偿,提出一种考虑时间权重的可调谐滤波器温漂补偿方法,通过对不同时间点的样本赋予新的权重,从而改变新旧样本的权重,使样本权重分配更合理。首先,在降温-升温的2 ℃窄变温环境进行温漂补偿实验,结果表明,所提算法在温漂补偿精度上均优于传统的AdaBoost-SVM、SVM、CART和RF算法。然后,在15 ℃降温幅度下进行实验,所提算法的实验结果远优于传统的AdaBoost-SVM算法。对比两次实验结果,在第一个数据集中,所提算法相比传统的AdaBoost-SVM算法在MAXE上的提升范围为27.95%~34.97%,而在第二个数据集中所提算法相比传统算法的MAXE提升范围达到64.93%~87.09%。这是因为缓慢降温过程的后半段存在温度的短期上下反复,这并不完全符合所提的在一般时间序列中更近的样本更重要的规律,因此所提模型的性能提升并不大;在第二个数据集中,温度降低呈现单调递减,梯度较大,也更加符合更近的样本更重要的规律。因此,所提算法引入时间权重进行建模,性能提升更加明显。此外,相比于传统的硬件补偿方法,所提出的基于机器学习的补偿方法不需要额外的硬件设备,更利于移植。
[1] 张劲松, 陈根祥, 黄力群, 等. 光纤光栅在光通信中的应用[J]. 光通信研究, 1998(3): 50-53.
Zhang J S, Chen G X, Huang L Q, et al. The applications of fiber gratings in optical communication[J]. Study on Communications, 1998(3): 50-53.
[2] 叶振兴, 苏洋, 朱勇, 等. 基于光纤光栅斯托克斯参量的压力传感测量研究[J]. 中国激光, 2012, 39(6): 0605003.
[3] Li Z Y, Xu Z Q, Tang Z H, et al. Research of high-speed FBG demodulation system for distributed dynamic monitoring of mechanical equipment[J]. Advances in Mechanical Engineering, 2013, 5: 107073.
[4] Zhao X L, Zhang Y X, Zhang W G, et al. Ultra-high sensitivity and temperature-compensated Fabry-Perot strain sensor based on tapered FBG[J]. Optics & Laser Technology, 2020, 124: 105997.
[5] Sheng W J, Peng G D, Liu Y, et al. An optimized strain demodulation method for PZT driven fiber Fabry-Perot tunable filter[J]. Optics Communications, 2015, 349: 31-35.
[6] Park H J, Song M. Linear FBG temperature sensor interrogation with Fabry-Perot ITU multi-wavelength reference[J]. Sensors, 2008, 8(10): 6769-6776.
[7] 路元刚, 王缘, 彭楗钦, 等. 迟滞和蠕变补偿的F-P滤波器波长解调方法研究[J]. 数据采集与处理, 2018, 33(1): 12-21.
Lu Y G, Wang Y, Peng J Q, et al. Study on F-P filter-based wavelength demodulation method with hysteresis and creep compensation[J]. Journal of Data Acquisition and Processing, 2018, 33(1): 12-21.
[8] Liu K, Jing W C, Peng G D, et al. Investigation of PZT driven tunable optical filter nonlinearity using FBG optical fiber sensing system[J]. Optics Communications, 2008, 281(12): 3286-3290.
[10] 郭海若, 刘琨, 江俊峰, 等. 基于可调谐激光器的光纤高低温力热复合多参量传感系统[J]. 中国激光, 2021, 48(19): 1906003.
[11] Fan X J, Jiang J F, Zhang X Z, et al. Self-marked HCN gas based FBG demodulation in thermal cycling process for aerospace environment[J]. Optics Express, 2018, 26(18): 22944-22953.
[12] Rivera E, Thomson D J. Accurate strain measurements with fiber Bragg sensors and wavelength references[J]. Smart Materials and Structures, 2006, 15(2): 325-330.
[13] 江俊峰, 臧传军, 王双, 等. 变温环境FBG解调仪复合多波长参考稳定方法研究[J]. 光电子·激光, 2018, 29(6): 575-581.
Jiang J F, Zang C J, Wang S, et al. Investigation of composite multi-wavelength reference stablization method for FBG demodulator in unsteady temperature environment[J]. Journal of Optoelectronics·Laser, 2018, 29(6): 575-581.
[14] Cheng J C, Fang J C, Wu W R, et al. Temperature drift modeling and compensation of RLG based on PSO tuning SVM[J]. Measurement, 2014, 55: 246-254.
[15] Shen C, Song R, Li J, et al. Temperature drift modeling of MEMS gyroscope based on genetic-Elman neural network[J]. Mechanical Systems and Signal Processing, 2016, 72/73: 897-905.
[16] 盛文娟, 董壮志, 杨宁, 等. 基于集成移动窗口的可调谐滤波器温度补偿研究[J]. 光学学报, 2021, 41(23): 2306005.
[17] Sheng W J, Dang H Q, Peng G D. Hysteresis and temperature drift compensation for FBG demodulation by utilizing adaptive weight least square support vector regression[J]. Optics Express, 2021, 29(24): 40547-40558.
[18] 盛文娟, 娄海涛, 彭刚定. 基于最小二乘支持向量机和多参考光栅的可调谐滤波器解调误差动态补偿[J]. 光学学报, 2023, 43(7): 0706003.
[19] 盛文娟, 赖振谱, 杨宁, 等. 基于改进AdaBoost算法的可调谐F-P滤波器温漂补偿方法[J]. 光学学报, 2023, 43(3): 0306004.
[20] Klinkenberg R. Learning drifting concepts: example selection vs. example weighting[J]. Intelligent Data Analysis, 2004, 8(3): 281-300.
[21] 李南. 概念漂移数据流分类算法及应用[D]. 福州: 福建师范大学, 2013: 37-41.
LiN. Classification algorithm of concept drift data stream and its application[D]. Fuzhou: Fujian Normal University, 2013: 37-41.
Article Outline
盛文娟, 钟处宁, 彭刚定. 考虑时间权重的可调谐滤波器温漂补偿方法[J]. 光学学报, 2023, 43(21): 2105001. Wenjuan Sheng, Chuning Zhong, Gangding Peng. Temperature Shift Compensation of Fiber Fabry-Perot Tunable Filter Based on Time Weight[J]. Acta Optica Sinica, 2023, 43(21): 2105001.