利用高斯函数拟合自体荧光光谱诊断乳腺癌的研究
下载: 835次
Breast cancer, the most common malignant disease with high mortality and morbidity, is the leading cause of death in women worldwide, especially in developing countries. The current methods for breast cancer diagnosis are time-consuming, expensive, and have low sensitivity, and these should be urgently addressed. Spectroscopy appears to offer a new method for clinical detection of normal and cancerous tissues. Fluorescence spectroscopy is a tool for the noninvasive acquisition of biochemical information and extracellular matrix, and its use is rapidly expanding owing to its safety and efficiency.
Breast tissue autofluorescence spectroscopy mostly uses a light source in the 420-512 nm band, and the tryptophan and tyrosine residues in the tissue are more sensitive to a laser with a wavelength of 405 nm. Therefore, this study used a 405 nm laser source to obtain autofluorescence spectra of breast tissue sections to avoid the interference of fat and blood on the spectrum during in vivo detection and to improve the spectrum intensity. Various biological macromolecules or molecular groups in human tissues emit fluorescence, and the fluorescence spectrum is a superimposed spectrum of various luminescent substances. Because of the short distance between the fluorescence wavelengths emitted by some fluorescent substances, a peak overlapping phenomenon occurs, and the spectral characteristic peaks are not easy to distinguish. On directly using the fluorescence spectral peak area for quantitative analysis, the quantification is neither objective nor accurate. Therefore, this study proposes a Gaussian function to fit the fluorescence spectrum of breast tissue slices. Because the Gaussian function describes the normal distribution, it effectively separates the overlapping peaks, simplifies the spectrum, and extracts information using the characteristic parameter peaks of the fitted spectrum. The area ratio method was used to analyze the differences in the spectra of the normal and cancerous breast tissues. Finally, after studying several samples, this paper proposes the use of an SVM algorithm to classify the fluorescence spectrum and analyzes the feasibility of this algorithm for the classification of the breast tissue fluorescence spectrum.
After the breast tissue becomes cancerous, the content of some fluorescent substances also changes. The normalized mean fluorescence spectra of normal and cancerous breast tissues show obvious red shifts in the fluorescence peaks of cancerous tissues relative to normal tissues (Fig. 4). The difference in fluorescent substances in normal and cancerous breast tissues was analyzed using area ratio method. The peak area ratios (A517/A492,A635/A492) of cancerous tissues are 2.4-8.5 times that of normal tissues, which can be considered as criteria for the diagnosis of breast cancer (Table 3). The discrimination results of the SVM algorithm are as follows: accuracy rate of 87.50%, recall rate of 93.94%, precision of 88.57%, and F1-score of 91.18%. This proves that the method has high sensitivity and recognition ability for the fluorescence spectral information of breast cancer tissue, and can map normal and cancerous tissue fluorescence spectral signatures in different states for classification.
In this study, the fluorescence spectrum of breast tissues under a 405 nm laser was collected. After fitting the fluorescence spectrum with a Gaussian function, the fluorescence spectrum peak area ratios for normal and cancerous tissues were calculated, and the effect of the SVM algorithm on the fluorescence spectrum was analyzed. The autofluorescence spectrum of cancerous breast tissues has a red-shift phenomenon compared with that of normal tissues; after separating the overlapping peaks by Gaussian function fitting fluorescence spectrum, the peak areas at 517 nm, 635 nm, and 492 nm of cancerous tissues have a significant ability to distinguish between normal and cancerous tissues. The SVM algorithm is feasible for the classification of breast tissue fluorescence spectrum, with an accuracy of 87.50%. This proves that the method can recall and pinpoint cancerous breast tissues, and has a strong balance between recall rate and precision, thus, providing a rapid diagnosis of breast cancer. In summary, the Gaussian function fitting the fluorescence spectrum can obtain the spectral characteristic information of the fluorophore in breast cancer and the peak area ratio can be used as the standard for diagnosing breast cancer. Moreover, the combination of the fluorescence spectrum and the SVM classification algorithm model can be used for multi-sample breast cancer, providing a feasible method for rapid diagnosis.
1 引言
乳腺癌是最常见的恶性疾病,具有较高的死亡率和发病率,是全球特别是发展中国家女性死亡的主要原因之一[1-3]。乳腺癌筛查方法有X光摄影、钼靶检查、乳腺超声和乳腺增强磁共振等,但这些方法作为临床辅助手段,无法作出最终诊断,须以临床组织学结论为诊断金标准。由于组织学诊断易受医师经验等外界因素的影响,临床上迫切需要客观、快速、高准确率的诊断方法。
光谱学为临床检测正常和癌变组织提供了一种新方法[4]。作为获取与生物化学信息相关的代谢性质以及组织中细胞外基质结构成分的工具,荧光光谱法具有无损、安全、高效的优点[5-7]。丁建华等[8]使用488 nm激光源获取离体乳腺正常组织、良性瘤组织和癌组织的自体荧光光谱,分析后发现三种组织的荧光光谱具有明显差异。荧光光谱是多个显著荧光团的叠加光谱,直接分辨谱峰具有较高难度。已有研究表明,可以使用高斯函数拟合方法、Gram-Schmidt方法以及光谱反褶积等方法得到荧光光谱的基谱,实现对疾病的诊断。秦艳利等[9]使用高斯曲线拟合方法分别对健康牙齿以及患有牙结石牙齿的荧光光谱进行拟合,结果发现,676 nm和486 nm处的荧光峰强度比可以作为诊断牙结石的标准。Pu等[10]使用Gram-Schmidt方法得到了乳腺组织中关键荧光团的光谱,有效检测了乳腺组织中荧光团的组成随癌症发展的变化。Chithra等[11]使用光谱反褶积方法计算不同内源荧光团的荧光强度、峰值波长和全波半峰全宽最大值,得到了乳腺癌患者血浆中的多种荧光基团,并发现荧光基团信息可以将治疗和未治疗患者区分开来。
乳腺组织自体荧光光谱法多采用420~512 nm波段的光源,而本团队采用波长为405 nm的激光源采集乳腺组织切片的自体荧光光谱,避免了活体检测时脂肪和血液对光谱的干扰,提高了光谱强度。由于部分荧光物质所发射的荧光波长距离较近,会出现重叠现象,直接使用荧光光谱峰面积进行量化分析,会导致量化不客观、不准确等问题。为了解决这一问题,本文使用高斯函数拟合乳腺组织切片的自体荧光光谱,然后利用拟合后的峰面积比分析正常组织和癌变组织的荧光光谱信息(峰面积、中心波长、半峰全宽)。研究表明,使用高斯函数拟合荧光光谱可以简化提取的光谱信息,并且可以准确、客观地分析正常组织和癌变组织的荧光光谱,最终诊断是否为乳腺癌。对较大数量样本研究后,本文决定采用支持向量机(SVM)算法对荧光光谱进行分类,从而实现对乳腺癌的快速诊断。
2 实验系统和方法
实验装置如
表 1. 实验仪器信息表
Table 1. Experimental instrument information table
|
用于实验的乳腺组织切片样本共80片,包括28片SP染色正常组织切片,10片苏木精-伊红(HE)染色癌变组织切片,10片未染色癌变组织切片,32片SP染色癌变组织切片。SP染色法中使用的染色剂由蒸馏水、DAB A、DAB B、DAB C按体积比为5∶1∶1∶1混合配制而成,HE染色法中的染色剂由苏木精与伊红按体积比为1∶1混合配制而成。染色剂配比时效为0.5 h,需在该时间内完成光谱采集。
本次实验中对每个组织样本分别检测10个不同的位置点,每一个位置点均采集三次光谱,共获取4类样本的2400组数据。采集光谱积分时间为112 ms,波长采集范围为450~750 nm。为避免日光等照明光源对实验结果造成干扰,以上实验操作均在暗室条件下完成。
数据处理采用Origin软件,先对4类乳腺组织样本的荧光光谱进行平均处理,然后使用Savitzky-Golay函数进行平滑处理,接着使用最值法将光谱峰值归一化到(0,1)范围内,最后使用高斯函数对归一化后的荧光光谱进行拟合。
3 分析与讨论
3.1 背景荧光
实验中使用的载玻片和染色剂产生的背景荧光会影响组织样品的荧光光谱,因此本次实验分别采集了载玻片和染色剂的荧光光谱,如
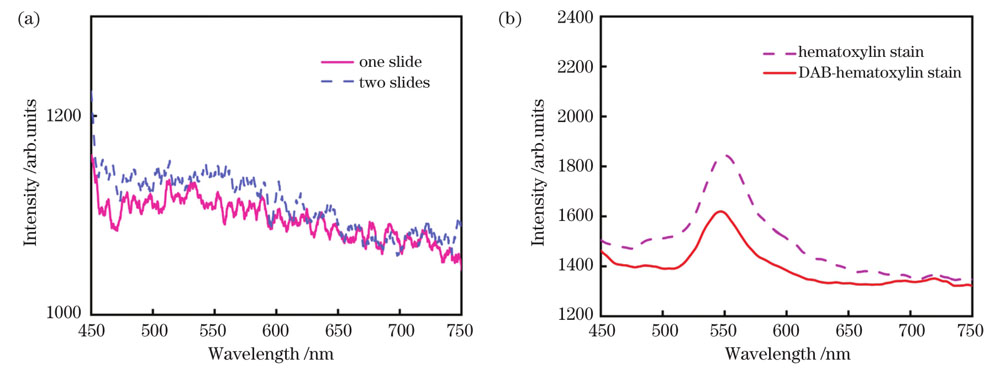
图 2. 载玻片和染色剂的荧光光谱。(a)载玻片的荧光光谱;(b)染色剂的荧光光谱
Fig. 2. Fluorescence spectra of slide and stain. (a) Fluorescence spectra of slide; (b) fluorescence spectra of stain
3.2 乳腺组织的荧光光谱分析
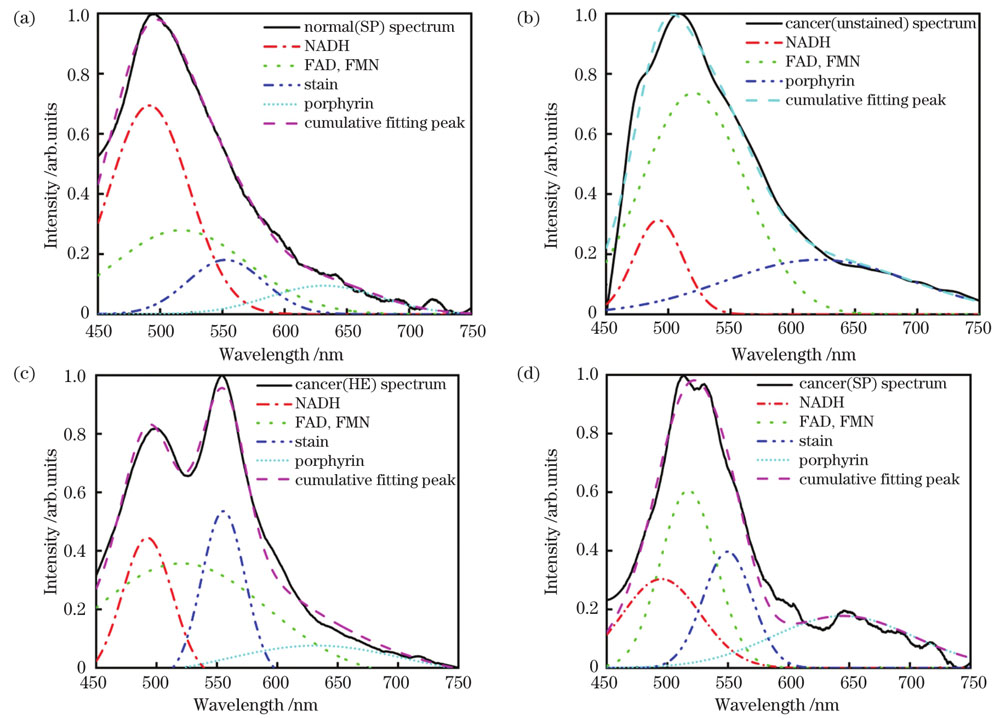
图 4. 高斯函数拟合的归一化平均荧光光谱图。(a)SP染色正常乳腺组织;(b)未染色癌变乳腺组织;(c)HE染色癌变乳腺组织;(d)SP染色癌变乳腺组织
Fig. 4. Normalized mean fluorescence spectrograms fitted by Gaussian function. (a) Normal breast tissue by SP staining; (b) unstained cancerous breast tissue; (c) cancerous breast tissue by HE staining; (d) cancerous breast tissue by SP staining
表 2. 高斯拟合峰的特征参量信息
Table 2. Characteristic parameter information of Gaussian fitting peak
|
由
表 3. 正常乳腺和癌变乳腺组织的峰面积比值A517/A492和A635/A492
Table 3. Peak area ratios of A517/A492 and A635/A492 of normal and cancerous breast tissues
|
由
综上可知:HE染色中使用的苏木精对伊红染液具有荧光淬灭效应,对组织中内源性荧光物质的影响较小,而SP染色过程中使用的封闭液对组织中内源性荧光物质的影响较大,在采集乳腺组织的自体荧光光谱时,应尽量选用石蜡包埋或HE染色切片。
3.3 荧光光谱分类算法实验
采用高斯函数对乳腺组织切片的自体荧光光谱进行拟合,可以提取光谱中的关键荧光基谱,然后通过峰面积比就可以判断组织是否癌变。为客观、准确、高效地筛查乳腺癌变组织,本次实验将采集的乳腺组织切片自体荧光光谱作为数据集,分析了SVM算法对乳腺组织荧光光谱进行分类、判别的可行性。SVM算法是一种有监督的分类算法,利用最优超平面对预测样本点的类别进行判断[22-24],具有适用于多样本数据集的优势。
正常和癌变乳腺组织的荧光光谱在490~540 nm波段内有较大重叠,不利于判断乳腺组织是否癌变。SVM算法使用核函数将正常和癌变乳腺组织的荧光光谱数据映射为可分数据,可以快速区分癌变组织。采用SVM算法对乳腺组织的荧光光谱进行判别,判别结果的准确率是87.50%,说明SVM算法对乳腺组织荧光光谱分类具有潜在的可行性。后续研究可以从电压、细胞 pH 值等影响组织样品荧光光谱的外界环境因素出发,通过抑制噪声干扰来提高信噪比,进而提高准确率。判别结果的召回率和精度分别为93.94%、88.57%,说明SVM算法可以将癌变乳腺组织荧光光谱的特征信息查全、查准,对乳腺癌变组织的荧光光谱信息具有较高的敏感度和识别能力。判别结果的F1分数为91.18%,说明SVM算法在检测乳腺组织荧光光谱中平衡召回率和精度的能力较强。为了与SVM算法的判别结果进行对比,后续工作拟考虑应用K最近邻(KNN)、卷积神经网络(CNN)等算法分析乳腺组织的荧光光谱,进而进行分类判别。
4 结论
本团队搭建了405 nm激光荧光光谱采集系统,并采用该系统采集了乳腺组织切片的自体荧光光谱;之后提出了使用高斯函数拟合自体荧光光谱的方法,计算了正常和癌变组织在517 nm和635 nm处的荧光光谱峰面积分别与492 nm处荧光光谱峰面积的比值;最后分析了SVM算法对荧光光谱的分类能力。研究结果表明:癌变后,细胞中的荧光基团受代谢影响,导致癌变乳腺组织较正常组织的自体荧光光谱出现了红移现象;使用高斯函数拟合荧光光谱可将重叠峰分离,得到癌变组织在517 nm和635 nm处的峰面积与492 nm处峰面积之比分别为正常组织的2.4~5.5倍和5.4~8.5倍,说明峰面积比对正常与癌变组织具有较强的区分能力。SVM算法的判别结果(准确率为87.50%,召回率为93.94%,精度为88.57%,F1分数为91.18%)证明该方法对癌变乳腺组织具有查全、查准能力,对召回率和精度的平衡能力较强,为乳腺癌的快速诊断提供了研究方向。
综上所述,采用高斯函数拟合荧光光谱可以得到乳腺癌组织中荧光基团的光谱特征信息,峰面积比可以作为诊断乳腺癌的标准,荧光光谱与SVM分类算法模型的结合可以为多样本乳腺癌的快速诊断提供可行途径。
[1] Akram M, Iqbal M, Daniyal M, et al. Awareness and current knowledge of breast cancer[J]. Biological Research, 2017, 50(1): 33.
[2] Siegel R L, Miller K D, Jemal A. Cancer statistics, 2021[J]. CA: A Cancer Journal for Clinicians, 2021, 71(1): 7-30.
[3] Sung H, Ferlay J, Siegel R L, et al. Global cancer statistics 2020: GLOBOCAN estimates of incidence and mortality worldwide for 36 cancers in 185 countries[J]. CA: A Cancer Journal for Clinicians, 2021, 71(3): 209-249.
[5] Andreeva V, Aksamentova E, Muhachev A, et al. Preoperative AI-driven fluorescence diagnosis of non-melanoma skin cancer[J]. Diagnostics, 2021, 12(1): 72.
[6] Litvinova K S, Rafailov I E, Dunaev A V, et al. Non-invasive biomedical research and diagnostics enabled by innovative compact lasers[J]. Progress in Quantum Electronics, 2017, 56: 1-14.
[7] 陈毛宁, 吴玲玲. 荧光分析法在早期龋齿检测中的应用研究[J]. 激光与光电子学进展, 2022, 59(13): 1330001.
[8] 丁建华, 余虹, 张志麟, 等. 离体乳腺正常组织、良性瘤及癌的自体荧光光谱[J]. 应用激光, 2000, 20(1): 37-39.
Ding J H, Yu H, Zhang Z L, et al. Laser induced fluorescence spectra of normal, benign and malignant tissues of breast in vitro[J]. Applied Laser, 2000, 20(1): 37-39.
[9] 秦艳利, 刘奕, 徐送宁, 等. 利用高斯函数拟合荧光光谱诊断龈下结石的研究[J]. 光电子·激光, 2009, 20(7): 988-990.
Qin Y L, Liu Y, Xu S N, et al. Detection of subgingival calculus by Gaussian curve fitting of laser-induced fluorescence emission[J]. Journal of Optoelectronics·Laser, 2009, 20(7): 988-990.
[10] Pu Y, Sordillo L A, Yang Y L, et al. Key native fluorophores analysis of human breast cancer tissues using Gram-Schmidt subspace method[J]. Optics Letters, 2014, 39(24): 6787-6790.
[11] Chithra K, Aruna P, Einstein G, et al. Monitoring breast cancer response to treatment using Stokes shift spectroscopy of blood plasma[J]. Journal of Fluorescence, 2019, 29(3): 803-812.
[12] Xue J P, Pu Y, Smith J, et al. Machine learning based analysis of human prostate cancer cell lines at different metastatic ability using native fluorescence spectroscopy with selective excitation wavelength[J]. Proceedings of SPIE, 2018, 10504: 105040L.
[13] 宋晓康, 赵强, 张元志, 等. 利用三维荧光光谱与平行因子分析法测定细胞培养基中多类代谢成分的含量[J]. 中国激光, 2022, 49(9): 0911001.
[14] 王可人, 叶玉琴, 朱珠, 等. 荧光光谱学在乳腺疾病诊治中的研究进展[J]. 中国实验诊断学, 2013, 17(2): 386-388.
Wang K R, Ye Y Q, Zhu Z, et al. Research progress of fluorescence spectroscopy in diagnosis and treatment of breast diseases[J]. Chinese Journal of Laboratory Diagnosis, 2013, 17(2): 386-388.
[15] 罗贤懋, 林培中, 刘雨菁, 等. 核黄素预防恶性肿瘤的研究进展[J]. 癌症进展, 2020, 18(4): 325-330.
Luo X M, Lin P Z, Liu Y J, et al. Research progress in prevention of riboflavin malignant tumor[J]. Oncology Progress, 2020, 18(4): 325-330.
[16] 王豪杰. 基于辅酶FAD设计的新型5-氰基-6-苯基嘧啶类LSD1抑制剂及其生物活性研究[D]. 郑州:郑州大学,2020.
WangH J. Design and activity evaluation of novel 5-cyano-6-phenyl pyrimidine LSD1 inhibitors based on coenzyme FAD[D]. Zhengzhou: Zhengzhou University, 2020.
[17] Balsukuri N, Das S, Gupta I. Carbazoleâ-corrole and carbazoleâ-prophyrin dyads: synthesis, fluorescence and electrochemical studies[J]. New Journal of Chemistry, 2015, 39(1): 482-491.
[18] 陈家璧,彭润玲. 激光原理及应用[M]. 北京:电子工业出版社,2019.
ChenJ B, PengR L. Principle and application of laser[M]. Beijing: Publishing House of Electronics Industry, 2019.
[19] 翁丹枫, 唐坚清, 黎三艳. 苏木精与伊红染液在病理小标本制片中的应用比较[J]. 临床与实验病理学杂志, 2019, 35(5): 610-611, 613.
Weng D F, Tang J Q, Li S Y. Comparison of hematoxylin and eosin staining in the preparation of small pathological specimens[J]. Chinese Journal of Clinical and Experimental Pathology, 2019, 35(5): 610-611, 613.
[20] 赵箭光, 吴小玲, 杨佩青, 等. 肝癌与正常肝组织激光荧光光谱的比较研究[J]. 中国医学物理学杂志, 1996, 13(4): 220-222.
Zhao J G, Wu X L, Yang P Q, et al. Comparative research on laser fluorescence spectrum of liver cancer and normal human liver tissues[J]. Chinese Journal of Medical Physics, 1996, 13(4): 220-222.
[21] 林娟, 晋龙, 吴晶晶, 等. 不同脱黑色素方法对HE和免疫组化染色结果的影响[J]. 临床与实验病理学杂志, 2021, 37(6): 751-753.
Lin J, Jin L, Wu J J, et al. Effects of different melanin removal methods on HE and immunohistochemical staining results[J]. Chinese Journal of Clinical and Experimental Pathology, 2021, 37(6): 751-753.
[22] Yang F C, Chen W, Wei H F, et al. Machine learning for histologic subtype classification of non-small cell lung cancer: a retrospective multicenter radiomics study[J]. Frontiers in Oncology, 2021, 10: 608598.
[23] 储宝, 黄尧, 倪敬书, 等. 基于GS-SVM的脑组织差分路径因子定量方法研究[J]. 中国激光, 2022, 49(5): 0507008.
Chu B, Huang Y, Ni J S, et al. Quantitative methods of brain tissue differential pathlength factor based on GS-SVM[J]. Chinese Journal of Lasers, 2022, 49(5): 0507008.
[24] 陈鹏, 齐超, 刘人玮, 等. 基于支持向量机回归的LIBS飞灰含碳量定量分析[J]. 光学学报, 2022, 42(9): 0930003.
陈文静, 教召航, 齐东丽, 沈龙海, 许诺, 谢東非, 李宜霏, 尤佳华, 李奇, 冯瑜. 利用高斯函数拟合自体荧光光谱诊断乳腺癌的研究[J]. 中国激光, 2022, 49(20): 2007106. Wenjing Chen, Zhaohang Jiao, Dongli Qi, Longhai Shen, Nuo Xu, Dongfei Xie, Yifei Li, Jiahua You, Qi Li, Yu Feng. Diagnosis of Breast Cancer Using Gaussian Function to Fit Autofluorescence Spectrum[J]. Chinese Journal of Lasers, 2022, 49(20): 2007106.