面向黏膜组织诊断的亚扩散域漫反射与荧光联合光谱测量系统
下载: 542次
Early-stage detection and treatment of mucosal tissue lesions are effective means for curbing mucosal cancer. Histopathological examination is the gold standard for the clinical examination of mucosal tissue lesions. However, this method is invasive and easily affected by doctors' experience and is unsuitable for large-scale and rapid screening of precancerous lesions. Spectral technologies can detect the changes in optical properties caused by the morphological changes of mucosal tissue cells in a real-time, in vivo, and noninvasive way, and can objectively analyze them using reasonable algorithms, which provides low-cost and large-scale screening of mucosal tissue lesions. In this study, we develop a mucosal lesion diagnosis system using joint sub-diffusive domain diffuse reflectance and fluorescence spectroscopy to improve sensitivity and specificity. The system can efficiently collect diffuse reflectance and autofluorescence spectra of mucosal tissues at varying depths. Furthermore, it extracts the characteristics of spectral data to realize the rapid screening of mucosal lesions and the real-time diagnosis of malignant tumor grade.
In this study, the mucosal lesion diagnostic system is composed of a light source module, detection module, main control module, man-machine interface module, and an optical fiber probe to measure the joint spectrum signal and real-time analysis and display tissue lesion information. First, we evaluate the stability of the light source module of the system using phantom experiments. Next, the in vitro experiments are conducted to verify the ability of the system to distinguish different tissues, including chicken and pork tissues. In the spectral pretreatment, the spectral data are preprocessed by smoothing and normalization to eliminate the system and environmental noise. Furthermore, the first derivative, principal component analysis, kurtosis, skewness, mean, and variance are used as input information for subsequent tissue classification to extract spectral features and realize the feature extraction. Next, we employ the support vector machine to distinguish the spectral characteristics of different tissues due to its advantages in dealing with small sample pattern recognition. To further verify the effectiveness and recognition ability of the proposed system, we conduct in vivo experiments on the human oral mucosa. The diffuse reflectance and autofluorescence spectra of the lower lip and tongue mucosal tissues are collected for tissue spectrum comparison.
The results of the phantom experiments show that the proportion of the intensity standard deviation of the two LEDs in the overall intensity is below 1%, implying that the system has excellent measurement stability (Fig. 7). The in vitro tissue experiments show that the system has excellent classification ability (the classification accuracy of different tissues is above 98%; the classification accuracy of different parts in a specific tissue is above 74%) (Tables 1 and 2). The in vivo experiments preliminarily verify the application potential of the system in mucosal tissue diagnosis and classification (Fig. 10).
In this study, we develop a mucosal lesion diagnosis system using joint sub-diffusive domain diffuse reflectance and fluorescence spectroscopy, which is noninvasive, operates in real-time, and is cost effective. First, the phantom with stable diffuse reflectance verifies that the system meets the required steady-state measurement. Next, the classification results of different tissues by the diffuse reflectance and autofluorescence spectra verify that our proposed system can realize the classification of different in vitro tissues. Finally, the in vivo experiments on human oral mucosa preliminarily verify that the system has the ability of in vivo detection. In the future, the detection of diseased mucosal tissue will be performed to further explore the clinical potential of the developed system. These findings support the utility of the system. In conclusion, the system provides strong systematic support and is an important method of reference for low-cost, large-scale screening of precancerous lesions of mucosal tissue.
1 引言
黏膜组织病变的早期诊断和及时干预对组织癌变的预防和治疗具有重要意义。组织病理学检测是临床上黏膜组织病变检查的“金标准”,但该方法具有创伤性,且容易受到医生水平的影响,不适用于早期癌前病变的大规模快速筛查。
近年来,光谱检测技术凭借无创、高灵敏性、实时便捷等优点在黏膜组织病变的快速筛查中展现出良好的应用前景。常用的光谱检测技术包括拉曼光谱、漫反射光谱和自体荧光光谱,其在肺癌[1]、宫颈癌[2]、口腔癌[3]等疾病诊断方面都取得了一定的进展。拉曼光谱[4]根据组织病变等产生的不同波长光谱信息,分析特定化学物质的含量,进而实现组织病变程度的判断,诊断精度较高,但其系统复杂,成本较高,需要在暗室的环境下采集光谱,不适用于黏膜组织病变的大规模快速筛查。漫反射光谱技术(DRS)[5]和自体荧光光谱技术(AFS) [6-7]利用宽谱漫反射信息或固有荧光色团激发产生的荧光来分析特定化学物质的含量变化,系统实现简单,但由于单一技术采集的信息有限,其检测结果的特异性和灵敏度存在一定局限。
综上,为了减小经验误差,同时兼顾成本和信息丰富度,实现无创、高效的黏膜组织病变的快速筛查,本文设计了一种基于漫反射和自体荧光光谱的黏膜组织癌前病变筛查系统,利用宽谱白光和紫外光探测黏膜组织在亚扩散域的漫反射光谱和自体荧光光谱,通过提取组织的联合光谱特征,对病变程度进行自动化判定,实现低成本黏膜组织癌前病变的快速筛查。
2 系统原理与方法
2.1 筛查原理
漫反射光谱是生物组织自身固有吸收和散射之间相互作用的结果,主要取决于组织的光学性质。与正常组织相比,组织病变会导致细胞形态、细胞核大小、核浆比发生变化,这些变化会引起光学参数的明显变化,从而反映在漫反射光谱中。自体荧光光谱通过检测组织自发荧光物质受特定入射光的激发而释放的荧光来反映黏膜组织病变程度。在不同的生理和病理条件下,黏膜组织中的荧光物质还原型烟酰胺腺嘌呤二核苷酸(NADH)和黄素腺嘌呤二核苷酸(FAD)的浓度会不同。
由于黏膜组织病变起源于上皮组织,其平均厚度为200~350 μm,因此将探测光纤放置到光源附近的亚扩散域进行测量,其源探距离(SDS)小于几个输运平均自由程,光子输运经历较少的散射,携带更多与被测组织微观形态特征相关的信息[8-9]。光在亚扩散域的传输示意图如
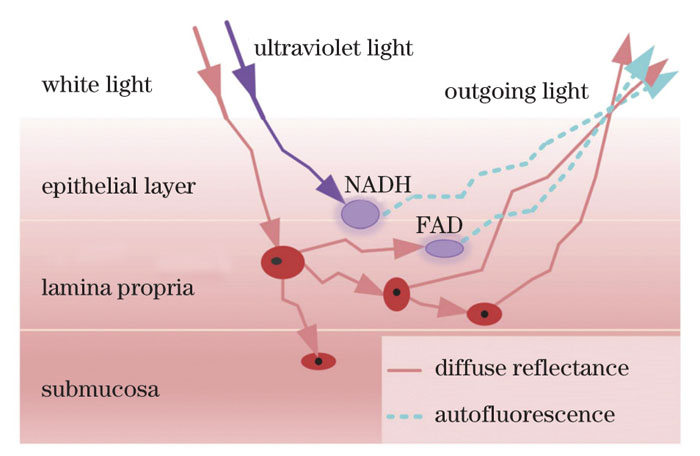
图 1. 光在黏膜组织亚扩散域的传输示意图
Fig. 1. Diagram of light transmission in sub-diffusive domain of mucosal tissue
2.2 系统设计
本文设计了一套面向黏膜组织诊断的亚扩散域漫反射与荧光联合光谱测量系统,如
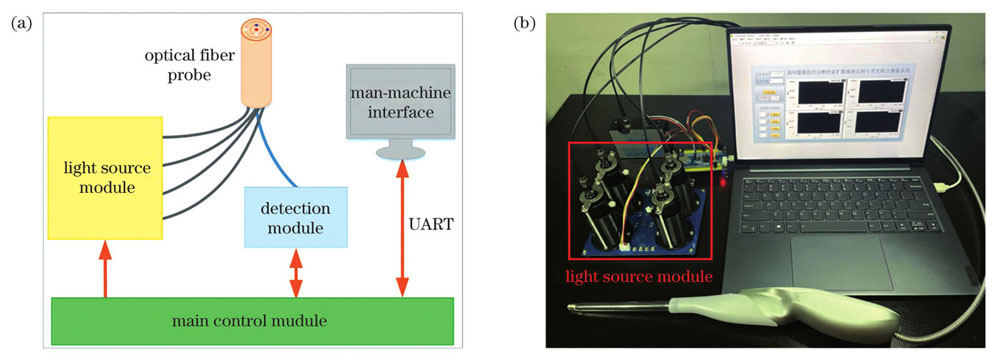
图 2. 系统原理和实物图。(a)原理图;(b)实物图
Fig. 2. Schematic and physical picture of system. (a) Schematic of system; (b) physical picture
2.2.1 光源模块
光源模块由两个中心波长为380 nm的紫外光和两个白光二极管(LED)及其驱动单元和滤波准直单元组成,原理如
2.2.2 光纤联合探头
黏膜组织早期病变发生时,黏膜上皮底部的细胞开始异常,并跟随异型增生细胞逐渐侵入上皮表层。如前所述,为了确保所设计系统的两个源探通道可以有效反映黏膜病变过程中组织参数深度的变化信息,本文根据黏膜组织的生理特性,构建了一个基于表层厚度变化的双层组织模型,并利用蒙特卡洛(MC)方法探究不同SDS测量通道的有效深度,其中有效深度定义为入射光子能量衰减50%时的深度信息[10]。如
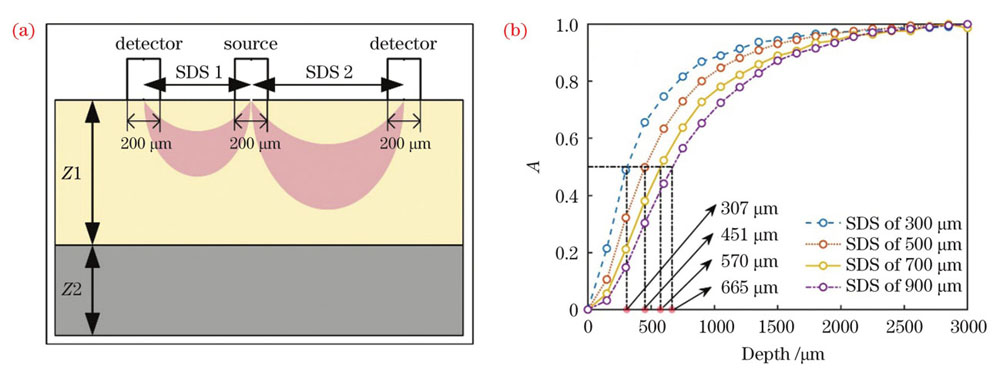
图 4. MC模拟模型及结果。(a)两层结构的MC模型;(b)未到达Z1与到达Z1深度的光子数量比曲线
Fig. 4. MC simulation model and results. (a) MC model with two-layer structure ; (b) ratio of number of photons not reaching Z1 depth to that reaching Z1 depth
设计的光纤联合探头如
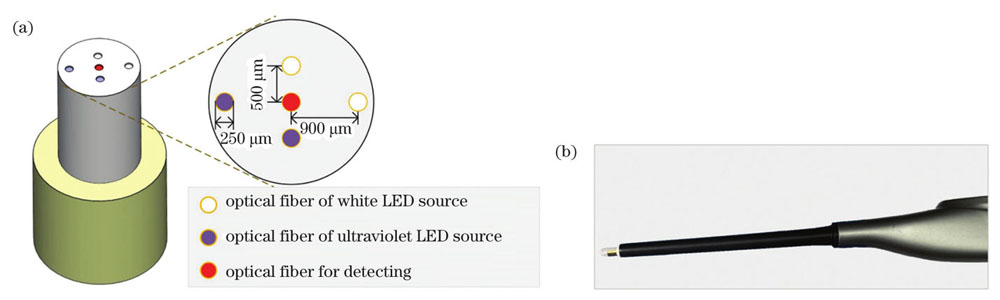
图 5. 光纤联合探头。(a)探头尖端示意图;(b)实物图
Fig. 5. Optical fiber combined probe. (a) Schematic of probe tip; (b) physical picture
2.2.3 探测模块
探测模块采用海洋光学光纤光谱仪将组织出射的光信号转换为光谱电信号,其探测波长范围为178.0~882.1 nm,光谱分辨率约为0.2 nm。为了减少数据冗余,只选取波长范围为400~700 nm的光谱数据。为了便于数据信息的获取和分析算法的应用,本文采用光谱仪内置的标准串口实现测量数据与人机交互模块的通信,可根据测量需要对系统光源激励顺序、光谱仪积分时间、光谱采集命令、数据校验、转换存储等功能进行设计。
2.2.4 主控模块
主控模块系统开机后先完成初始化,检测到人机交互模块发出的开始测量信号后进入光谱测量主循环:对4个光源通道依次进行光源激励,设置光源光谱积分时间,发送光谱采集信号,进入光谱测量模式,将采集到的光谱数据传输至主控模块,判断数据标志有效后进行光谱数据转换存储。主循环一次后判断测量是否结束,若未结束则控制光源模块另一光源通道进行光谱测量,直至系统完成4条联合光谱测量。系统运行流程如
2.3 系统稳定性评估
如前所述,光源模块持续稳定的工作能力是系统性能的重要保证,因此本文首先对系统的光源模块进行稳定性评估。实验中分别选取一个白光和一个紫外LED进行稳定性测量,采用漫反射率稳定的聚甲醛平板作为测试对象。每次只打开一个LED光源,垂直照射聚甲醛平板的探测光源是SDS为500 μm的出射光,积分时间设置为1 s,每隔2 min测量一次,连续测量10次。计算10条光谱的平均光谱强度,然后计算每个波长下的强度标准偏差(SD,S):
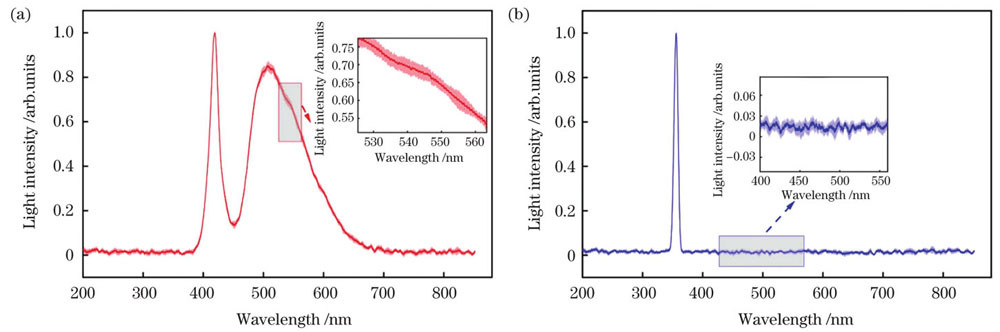
图 7. 平均光谱强度和光谱强度标准偏差。(a)白光LED;(b)紫外光LED
Fig. 7. Mean spectral intensity and standard deviation of spectral intensity . (a) White LED; (b) ultraviolet LED
2.4 组织分类算法
在系统测量中,系统采集宽谱白光测量下SDS为500 μm和900 μm的2条漫反射光谱和紫外光测量下SDS为500 μm和900 μm的2条自体荧光光谱,得到4条含有生物组织特征的光谱信息,分别命名为DRS-500、DRS-900、AFS-500和AFS-900。
首先对光谱数据进行移动窗口平均平滑(smoothing)和归一化(normalization)预处理,以消除仪器和环境噪声的影响。接着,为了提取光谱信息中的特征量,采用一阶导数处理、主成分分析(PCA)、峰度(kurtosis)、偏度(skewness)、均值(mean)和方差(variance)实现特征抽取,并将结果作为输入信息进行后续组织分类研究。支持向量机在小样本模式识别过程中存在一定优势,本文选用基于线性内积核函数的支持向量机来区分不同组织的光谱特征,并按3∶1的数量比例随机划分训练集和测试集。由于训练和测试的数据量较少,为了更好评估分类性能,采用了五折交叉验证法对特征集进行训练。
3 离体与在体实验
3.1 离体实验验证
为了验证系统区分不同组织的能力,首先开展了离体组织的分类实验。如

图 8. 四种动物的离体组织。(a)猪肌肉;(b)鸡胸肉;(c)猪五花肉;(d)猪前腿肉
Fig. 8. In vitro tissues of four animals. (a) Porcine muscle; (b) chicken breast; (c) pork belly; (d) pork shoulder
分析离体组织光谱AFS-500和AFS-900发现:两条光谱的自体荧光现象不明显,可能与离体组织中含有的内源性荧光团活性丧失有关。此处只讨论漫反射光谱DRS-500和DRS-900。如
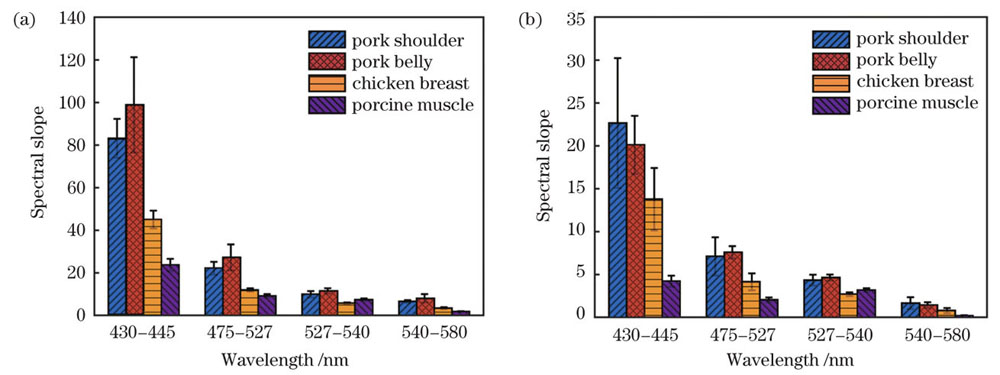
图 9. 不同组织在不同波段的斜率。(a)SDS为500 μm;(b)SDS为900 μm
Fig. 9. Spectral slopes of different tissues at different wavelengths. (a) SDS of 500 μm; (b) SDS of 900 μm
首先,将预处理后的全波段光谱数据进行分类,三组离体组织的分类结果如
表 1. 三组离体组织的全波段光谱分类结果
Table 1. Classification results of three groups of in vitro tissues in full-band spectra
|
表 2. 三组离体组织的特征波段光谱分类结果
Table 2. Classification results of three groups of in vitro tissues in characteristic band spectra
|
3.2 人体口腔黏膜组织在体实验
为进一步验证系统对不同类型组织的识别能力,本文征集三名被试者开展了在体口腔黏膜实验,分别采集被试者的下唇(lower lip)和舌部(tongue)两处黏膜组织的漫反射光谱和自体荧光光谱,积分时间分别设置为2 s和10 s,其光谱结果如
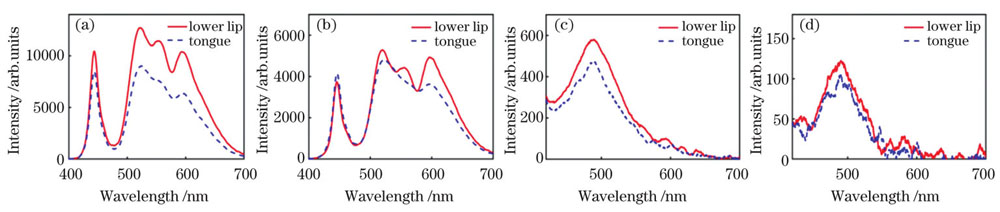
图 10. 两种黏膜组织的光谱。(a)DRS-500;(b)DRS-900;(c)AFS-500;(d)AFS-900
Fig. 10. Spectra of two mucosal tissues. (a) DRS-500; (b) DRS-900; (c) AFS-500; (d) AFS-900
4 结论
设计了一套面向黏膜组织诊断的亚扩散域漫反射与荧光联合光谱测量系统,以无创、实时、高性价比的方式实现了黏膜组织病变的快速筛查和恶性肿瘤等级的实时诊断。首先,进行了漫反射率稳定的聚甲醛仿体实验,验证了系统具有良好的测量稳定性。接着,通过系统采集离体猪肉和鸡肉组织的漫反射和自体荧光光谱,并利用光谱特征信息开展组织分类研究,结果表明,系统可以实现不同离体组织的准确分类。最后,开展了面向人体口腔黏膜组织的在体实验,采集了下唇和舌部黏膜组织的光谱信息,初步验证了系统具备在体探测的能力。未来将通过病变黏膜组织检测,进一步探究所设计系统的临床潜力。所设计的面向黏膜组织诊断的亚扩散域漫反射与荧光联合光谱测量系统为低成本大规模黏膜组织癌前病变的筛查提供了有力的支持。
[1] Spliethoff J W, Evers D J, Klomp H M, et al. Improved identification of peripheral lung tumors by using diffuse reflectance and fluorescence spectroscopy[J]. Lung Cancer, 2013, 80(2): 165-171.
[2] Liu W W, Jin X F, Li J J, et al. Study of cervical precancerous lesions detection by spectroscopy and support vector machine[J]. Minimally Invasive Therapy & Allied Technologies, 2021, 30(4): 208-214.
[3] de Veld MSc D C G, Marina S, Witjes M J H, et al. Autofluorescence and diffuse reflectance spectroscopy for oral oncology[J]. Lasers in Surgery and Medicine, 2005, 36(5): 356-364.
[4] 刘风翔, 张礼豪, 黄霞. 拉曼光谱技术在肿瘤诊断中的应用[J]. 激光与光电子学进展, 2022, 59(6): 0617016.
[5] Evers D J, Nachabé R, Klomp H M, et al. Diffuse reflectance spectroscopy: a new guidance tool for improvement of biopsy procedures in lung malignancies[J]. Clinical Lung Cancer, 2012, 13(6): 424-431.
[6] Monici M. Cell and tissue autofluorescence research and diagnostic applications[J]. Biotechnology Annual Review, 2005, 11: 227-256.
[7] Davey C J, Vasiljevski E R, O'Donohue A K, et al. Analysis of muscle tissue in vivo using fiber-optic autofluorescence and diffuse reflectance spectroscopy[J]. Journal of Biomedical Optics, 2021, 26: 125001.
[8] 张琪, 张丽敏, 安敬仪, 等. 面向黏膜组织病变识别的亚扩散域空间分辨光学参数测量方法[J]. 光学学报, 2021, 41(24): 2417001.
[9] Chang V T C, Cartwright P S, Bean S M, et al. Quantitative physiology of the precancerous cervix in vivo through optical spectroscopy[J]. Neoplasia, 2009, 11(4): 325-332.
[10] Hennessy R, Goth W, Sharma M, et al. Effect of probe geometry and optical properties on the sampling depth for diffuse reflectance spectroscopy[J]. Journal of Biomedical Optics, 2014, 19(10): 107002.
Article Outline
郑杰, 刘东远, 张琪, 张丽敏, 高峰. 面向黏膜组织诊断的亚扩散域漫反射与荧光联合光谱测量系统[J]. 中国激光, 2022, 49(24): 2407101. Jie Zheng, Dongyuan Liu, Qi Zhang, Limin Zhang, Feng Gao. Mucosal Lesion Diagnosis System Using Joint Sub-Diffusive Domain Diffuse Reflectance and Fluorescence Spectroscopy[J]. Chinese Journal of Lasers, 2022, 49(24): 2407101.