基于偏振和明场多模态显微成像技术的乳腺癌智能诊断研究
下载: 795次
近年来,乳腺癌已成为威胁女性健康的头号恶性肿瘤,对其实现快速精准的筛查和诊断变得尤为重要。针对现有临床病理学检查需要染色突显细胞形态并依赖医生主观经验判断的局限性,立足于明场显微成像和偏振显微成像构建多模态显微成像技术,获取正常与癌变乳腺组织未染色冰冻切片的形态结构及其异质性信息并对之进行分析和诊断研究。首先对正常与癌变组织切片进行多角度正交偏振成像、明场显微成像并分析图像差异性;然后实施像素级图像融合;接着利用卷积神经网络模型,对多模态融合图像进行深度学习的特征提取与分类,有效提升准确率(0.8727)和受试者特征曲线下面积(AUC,0.9400)等参数,进而实现精准的乳腺癌智能诊断。该技术有助于医生进行快速准确的临床诊断,为实施乳腺癌术中快检以辅助精准手术治疗提供有效的技术手段,具有突出的临床潜力和应用前景。
Breast cancer has been the most common life-threatening cancer of women in recent years. Both diagnostic imaging and pathology are routinely employed to diagnose breast cancer, while the latter is considered the "gold standard" for cancer diagnosis. In clinical practice, however, the routine pathological diagnosis is strongly hindered by the complicated and time-consuming process of staining the biopsy sections with hematoxylin and eosin (H&E) for highlighting the fine structures of cells and tissues. Moreover, the diagnosis is manually performed by pathologists, therefore the diagnostic speed and accuracy are highly dependent on their knowledge and experience. In order to better serve the treatment of breast cancer, the pathological diagnosis is greatly expected to be accelerated and automated, especially providing the efficient intraoperative assessment for precision surgical therapy. In this study, a rapid, accurate and automatic diagnostic technique of breast cancer is proposed based on the polarization and bright-field multimodal microscopic imaging. To accelerate the pathological diagnosis, the breast biopsy sections are directly investigated without the H&E staining, while the cell structures are no longer distinct under the bright-field microscopic imaging. In this case, the polarization microscopic imaging is introduced to further extract the morphological difference between the normal and cancerous breast tissues. Collagen fiber is an important part of the extracellular matrix (ECM), and the organization of collagen fibers has been found to be closely related with the cancer progression. Due to the inherent optical anisotropy, collagen fibers can be examined by cross-polarization imaging. To perform the automatic diagnosis, deep learning is employed to distinguish the normal and cancerous breast tissues, where the convolutional neural network (CNN) classification model is established to extract features from the multimodal microscopic images and make the accurate and reliable judgements.
In this study, 23 breast biopsies from 16 patients were first rapidly frozen in liquid nitrogen and cut into sections with the thickness of 15 μm using a cryotome. Without the H&E staining, the bright-field microscopic imaging and polarization microscopic imaging were sequentially performed through switching the light source, polarizer and analyzer of the custom-made transmission polarization microscope. The polarization microscopic imaging was operated in the cross-polarization mode, where the polarizer was orthogonal to the analyzer. Since the period of cross-polarization imaging is 90°, the polarizer-analyzer pair was rotated by 0°, 30° and 60° to characterize the biopsy sections. Following the microscopic imaging, the pixel-level image fusion was conducted to transform the four multimodal images into a single fused image for breast cancer diagnosis. In order to perform the deep learning-assisted automatic diagnosis, the classical CNN ResNet34 was utilized to develop a classification model, whose input is the pixel-level fused image. In addition to the pixel-level fusion, the decision-level fusion was also examined for comparison, where two classification models were created based on the bright-field and polarization microscopic imaging, respectively. In this way, the final classification result was generated according to the weight coefficients determined using the logistic regression algorithm. To evaluate the performance of CNN classification model, four parameters including accuracy, sensitivity, specificity and AUC [area under receiver operating characteristic (ROC) curve] were calculated. Due to the rather small amount of breast biopsies, the leave-one-out cross validation was performed in order to avoid overfitting.
The cross-polarization microscopic imaging of normal breast tissues exhibits the distinct periodic change in the brightness with the rotation of the polarizer-analyzer pair from 0° to 90°, while that of cancerous breast tissues is almost unchanged (Fig. 3). This polarization-sensitive brightness change results from the anisotropic organization of collagen fibers in the normal breast tissues, which alters during the cancer progression. Further, the classification result of the multi-polarization imaging is better than that of the single-polarization imaging (Table 1). Following the pixel-level fusion of multimodal images, the CNN classification model based on ResNet34 was established. As a result, the accuracy of 0.8727 and AUC of 0.9400 were realized, better than bright-field (0.8540, 0.9013) and polarization (0.8575, 0.9307) microscopic imaging, respectively (Table 2). In addition, the decision-level fusion was also evaluated, achieving the accuracy of 0.8710 and AUC of 0.9367. The weight coefficients of bright-field and polarization imaging were calculated to be 0.3971 and 0.6029, respectively.
A deep learning-assisted multimodal microscopic imaging technique is proposed for the rapid, accurate and automatic diagnosis of breast cancer, combining bright-field and cross-polarization microscopic imaging. In this scheme, the time-consuming process of H&E staining can be removed to accelerate the breast cancer diagnosis, and the automatic diagnosis can be performed with the CNN classification model. Moreover, the accurate diagnosis can be realized, since the cross-polarization microscopic imaging can further extract the polarization-sensitive morphological change closely related with the cancer progression, such as the organization of the optically anisotropic collagen fibers. In this sense, the multimodal microscopic imaging diagnosis has great potential to better serve the surgical treatment of breast cancer with the efficient intraoperative assessment.
1 引言
乳腺癌是女性最常见的恶性肿瘤。根据世界卫生组织(WHO)的统计,2020年乳腺癌已经取代肺癌,成为全球女性第一大癌[1]。因此,对其实施高效的筛查和诊断变得越来越重要。癌症常见的诊断方式主要包括影像学检查和病理学检查。影像学检查主要包括计算机断层扫描(CT)、磁共振成像(MRI)、正电子发射断层扫描(PET)等手段。CT存在辐射危险且有对高密度乳腺组织不敏感等问题,不适合常规筛查[2],MRI无放射性损害,但检测费用更高且更耗时[3],并且这两种影像学诊断方式对乳腺癌诊断的精确度都有待提高。PET-CT混合技术是可视化肿瘤扩散或检测肿瘤对治疗反应的最准确方法,但是对于乳腺癌中微小病灶的检出率较低[2,4-5]。病理学检查被认为是癌症诊断“金标准”,通常采用苏木精-伊红染色法(H&E染色)突显组织内细胞形态,再由病理医生在显微镜下观察组织结构和细胞病变特征并做出疾病诊断,但无论是术中的冰冻切片检查还是术后的石蜡切片检查都不可避免存在病理切片染色制作流程复杂、耗时较长和诊断精确度受限于病理医生经验等问题。因此,需要发展一种在术中快速、准确、客观地诊断乳腺癌的自动诊断技术[6-7]。
近年来对癌症的深入研究发现,癌症的发展与细胞外基质(ECM)和肿瘤微环境有着密切的联系[8-10]。在癌症侵袭期间,肿瘤基质结构伴随着ECM通过胶原蛋白降解、再沉积和交联进行重塑[11],其中纤维状胶原蛋白构成各向异性的组织形态,具有强烈的双折射效应,是重要的癌症诊断信息[12-15]。偏振显微成像技术通过正交偏振检测,无需染色即可对组织切片中具有双折射效应的组织形态实施针对性显微成像,可表征癌症发展过程中胶原纤维的大体变化信息[16]。同时,常规的明场显微成像技术基于不同组织成分对光的吸收特性差异形成视野中的明暗差别,在组织切片未染色处理的情况下,仍可反映癌症组织与正常组织的形态结构以及边缘细节差异,为癌症诊断提供异质性信息[13]。这两种显微成像技术的物理机制不同,可相互融合与补充,挖掘癌变过程中更多的生理信息,从而有望实现更加准确可靠的癌症诊断。为了充分利用多模态显微图像所表征的正常和癌变组织的光学差异性信息实施智能癌症诊断,需要发展智能算法挖掘特征信息并构建判别模型。
深度学习算法已被验证可以实现癌症等疾病的信息提取、自动分析和智能诊断[17-19]。随着多模态显微图像数量的快速增长,自动分析代替人工成为必然的发展趋势,并且深度学习智能诊断还可以提高诊断精确度、缩短诊断时间、解决依赖病理医生主观判断等问题[20-21]。基于深度学习的多模态图像融合技术可以实现多模态图像数据融合,达成多种异质性信息的互补,为判别模型分类决策提供更多的信息,从而提高癌症诊断的精确度[22]。之前的一些研究中,多模态图像融合技术已经开始运用到乳腺癌[22]、脑肿瘤[23-24]等癌症诊断中,并取得了一定成果,表明基于多模态成像的诊断体系具有巨大的研究潜力。然而,之前的多模态成像癌症诊断研究仍然是基于H&E染色组织切片,不利于术中快速癌症检测的实现。
本文基于未染色乳腺组织冰冻切片,创新性地提出了一种基于偏振和明场多模态显微成像技术的乳腺癌智能诊断方法。结合偏振显微成像和明场显微成像构建多模态显微成像技术并采集显微图像,运用深度学习算法实施图像信息融合并构建判别模型,实现乳腺癌的快速精确智能诊断。测试结果表明,这是一套高效精准的多模态成像及智能诊断体系。
2 实验方法
2.1 组织切片制作
从江苏省肿瘤医院采集了16位患者的乳腺组织,经机构审查委员会批准使用。患者被标记为S1~S16,其中,S1~S7含有癌症样本和正常样本,S8~S14只含癌症样本,S15~S16只含正常样本。新鲜组织样本由手术切取后,立即水埋并通过液氮快速冷冻,以保持样本的新鲜性和完整形态;然后样本被固定在低温切片机(Leica CM1950,德国)样品架上被切割成厚度为15 μm的组织切片。从16位患者总共得到14个癌症切片和9个正常切片,切片制作整体流程中没有使用固定剂,并且多模态显微成像技术无需对组织切片进行H&E染色,因此可避免化学药剂对组织切片中生物成分及结构造成影响[22]。
2.2 多模态图像采集、融合及分类
图像采集设备基于透/反射偏振显微镜(BM2100POL,永新光学)进行定制,通过切换偏振片、调整光源实现明场和偏振两种成像模式。采用20倍物镜进行显微成像,使用图像采集软件(ScopeImage 9.0)设置不同成像模式参数,图像采集视场大小为 1 mm×1 mm。
在明场显微成像中,照明光源在载物台下面提供明场照明,透射后通过物镜会聚成像,由探测器捕获,以获取组织的形态结构信息。正交偏振显微成像中,光源发出的自然光通过起偏器变成线偏振光照射在组织切片上,其透射光通过物镜与检偏器(与起偏器正交)后由探测器捕获。保持起偏器与检偏器正交设置,改变正交偏振片组合与组织切片的相对角度,考虑到偏振检测周期为90°,采集一组由3张角度分别为0°、30°和60°的正交偏振图像构成偏振图集,以表征组织中胶原纤维的分布和结构信息。
从23块组织切片中共取得2302组多模态显微图像,同组图像取自同一切片的同一部位,并且采集区域尽量靠近切片的中心位置,避免了切片边缘可能存在的癌旁组织。每组图像包括3张不同角度的正交偏振图像和1张明场显微图像。流程如

图 1. 多模态显微图像像素级融合流程
Fig. 1. Flow chart of pixel-level fusion of multimodal microscopic images
多模态图像采集、融合及分类模型如
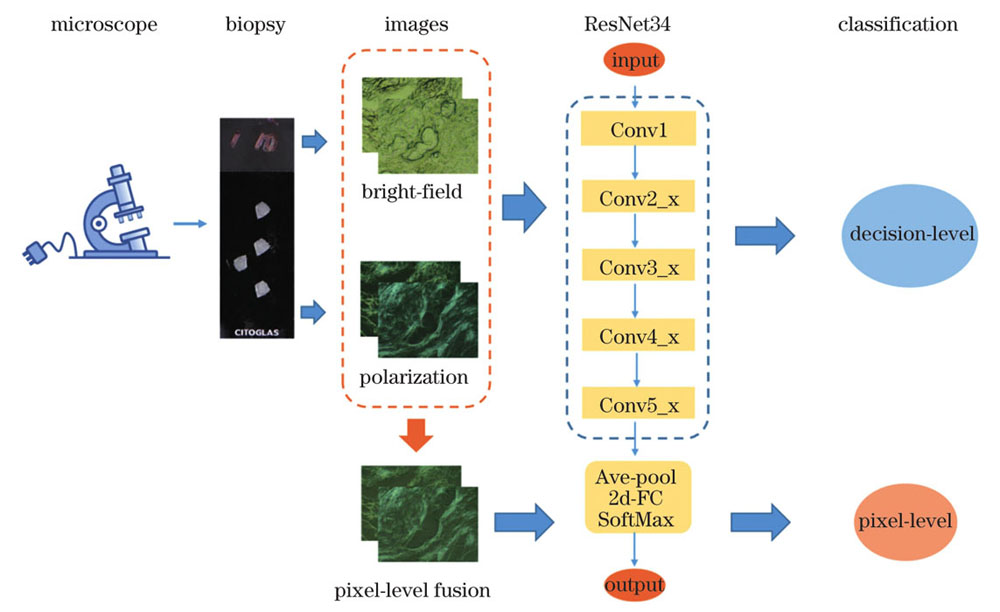
图 2. 多模态显微成像癌症诊断方法示意图
Fig. 2. Diagram of cancer diagnosis based on multimodal microscopic imaging
利用准确度、敏感度、特异度、受试者特征曲线下面积(AUC)等4个参数评估模型性能[27]。为了避免小样本过拟合的影响,用于训练分类模型的患者样本与用于测试分类模型的患者样本完全不同,训练模型时将单独一个患者的数据作为测试集,其余图像数据作为训练集。同时为减少模型性能可能受所选测试集患者的数据分布的影响,以更客观准确地评估性能,采用留一交叉验证法对模型进行进一步评估,即在模型训练过程中,根据测试集所选患者的不同将数据集划分16次,分别输入到网络中进行训练与验证。鉴于每个患者采集到的图像数量有所差异,且有些患者缺乏癌症样本或者正常样本,最终模型的性能参数将根据测试图片数量对16次模型测试结果加权平均得到。
3 结果与分析
3.1 多模态显微图像差异分析
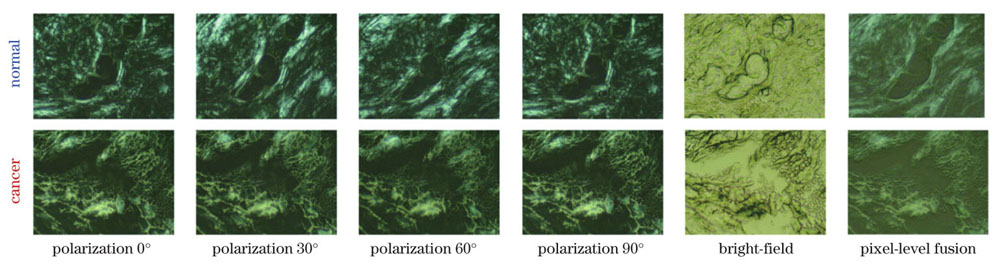
图 3. 正常和癌变乳腺组织的多角度偏振显微图像、明场显微图像及像素级融合图像
Fig. 3. Multi-angle polarization microscopic images, bright-field microscopic images and pixel-level fused images of normal and cancerous breast tissues, respectively
3.2 单角度和多角度偏振成像诊断性能分析
表 1. 单角度偏振成像和多角度偏振成像分类模型性能
Table 1. Classification performances of single-angle and multi-angle polarization microscopic imaging
|
3.3 单模态和多模态显微成像诊断性能分析
表 2. 单模态显微成像和多模态显微成像分类模型性能
Table 2. Classification performances of unimodal and multimodal microscopic imaging
|
根据明场和偏振融合图像分类模型的交叉验证结果,利用逻辑回归方法得出明场和偏振图像的决策级融合系数分别为0.3971和0.6029。这个结果表明偏振图像相比于明场图像具有更多特征信息,在进行多模态图像融合时,根据系数差异安排合适的决策权重可以更充分地利用各模态之间的差异,提高模型的分类精度。
如
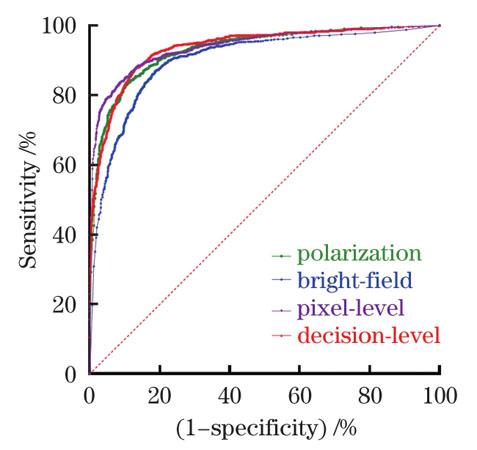
图 4. 单模态显微成像和多模态显微成像分类模型ROC图
Fig. 4. ROC diagram of classification models based on unimodal and multimodal microscopic imaging
3.4 多模态显微成像乳腺癌诊断方法分析
相比于传统的乳腺癌诊断方法,基于多模态显微成像技术的乳腺癌诊断方法利用了癌变组织和正常组织中胶原蛋白成分及其形态结构差异,通过卷积神经网络提取特征信息用于癌症诊断,使其无论在术中快检还是术后检查都具有独特优势。首先,相比于传统的术中病理诊断需要0.5~1.0 h甚至若干小时,多模态显微成像诊断从组织取样、冰冻切片到图像采集、智能预测整个诊断流程不到20 min,能够为患者的救治和医生的决策提供宝贵的时间和诊断依据。其次,多模态显微成像诊断系统的样本制作采用的是无需固定剂的水埋冰冻技术,不仅极大地简化了操作步骤、降低了操作门槛,也避免了常规染色切片制作过程中化学药剂对组织成分和结构的干扰,使诊断精度得到了进一步提升。第三,因为诊断的依据主要来自组织成分和结构的变化,而不是常规病理检测技术对细胞形态数量的检测,所以快速冰冻对细胞的影响可以忽略。最后,人工智能神经网络模型的智能判别相比于病理医生的主观判断不仅能提取更多的诊断信息,提高诊断精度,也避免了诊断结果受限于病理医生经验等问题。本研究中,无论是偏振显微图像还是明场显微图像的分析和学习分类,都是基于正常和癌变组织的明确的生理、物理、形貌等特征差异实现的,具有明确现实的生物医学、物理及光学诊断依据和基础。本文提出的多模态成像诊断系统具有简便快捷、精准有效、性价比高、操作简单、易于实施等优势,拥有巨大的潜在临床应用价值,未来有望逐步进入临床实践和应用。
4 结论
本文应用多角度正交偏振成像和明场显微成像相结合的多模态显微成像技术,并结合深度学习算法,实现了乳腺癌的快速精准智能诊断。首先采集未染色乳腺组织切片的多模态显微图像,接着分析多模态显微图像所表征信息的差异性,在此基础上,建立卷积神经网络判别模型,以多模态像素级融合图像作为输入,实施特征提取和分类,实现快速精准的正常和癌变组织判别。像素级融合判别模型的准确度为0.8727,AUC为0.9400;决策级融合判别模型的准确度为0.8710,AUC为0.9367。相较于明场显微成像、单角度或多角度融合偏振成像的判别模型性能,多模态成像在准确度、AUC等性能指标上都有了明显提高。本研究表明多模态显微成像智能诊断方法用于癌变组织诊断有其独特的优势和物理内涵,为该技术应用于术中快检和临床智能诊断奠定了研究基础。另外,该智能诊断体系此前未见报道,且性价比和临床可行性高,具有积极的临床意义和应用前景。
[1] Sung H, Ferlay J, Siegel R L, et al. Global cancer statistics 2020: GLOBOCAN estimates of incidence and mortality worldwide for 36 cancers in 185 countries[J]. CA: a Cancer Journal for Clinicians, 2021, 71(3): 209-249.
[2] Rosen E L, Eubank W B, Mankoff D A. FDG PET, PET/CT, and breast cancer imaging[J]. Radiographics, 2007, 27(Suppl 1): S215-S229.
[3] Turnbull L, Brown S, Harvey I, et al. Comparative effectiveness of MRI in breast cancer (COMICE) trial: a randomised controlled trial[J]. The Lancet, 2010, 375(9714): 563-571.
[4] Qi S B, Hoppmann S, Xu Y D, et al. PET imaging of HER2-positive tumors with Cu-64-labeled affibody molecules[J]. Molecular Imaging and Biology, 2019, 21(5): 907-916.
[5] Yang S K, Cho N, Moon W K. The role of PET/CT for evaluating breast cancer[J]. Korean Journal of Radiology, 2007, 8(5): 429-437.
[6] Zhang J Y, Chen B, Zhou M, et al. Photoacoustic image classification and segmentation of breast cancer: a feasibility study[J]. IEEE Access, 2019, 7: 5457-5466.
[7] 李阳曦, 胡成全, 马龙飞, 等. 智能化精准光学诊疗技术研究进展[J]. 中国激光, 2021, 48(15): 1507002.
[8] Stylianou A, Voutouri C, Mpekris F, et al. Pancreatic cancer collagen-based optical signatures[J]. Proceedings of SPIE, 2021, 11646: 1164613.
[9] Dong Y, Qi J, He H H, et al. Quantitatively characterizing the microstructural features of breast ductal carcinoma tissues in different progression stages by Mueller matrix microscope[J]. Biomedical Optics Express, 2017, 8(8): 3643-3655.
[10] Sharma M, Shaji C, Unni S N. Optical polarization response of collagen: role in clinical cancer diagnostics: part I[J]. ISSS Journal of Micro and Smart Systems, 2022, 11(1): 3-59.
[11] Fang M, Yuan J P, Peng C W, et al. Collagen as a double-edged sword in tumor progression[J]. Tumor Biology, 2014, 35(4): 2871-2882.
[12] Martins Cavaco A C, Dâmaso S, Casimiro S, et al. Collagen biology making inroads into prognosis and treatment of cancer progression and metastasis[J]. Cancer and Metastasis Reviews, 2020, 39(3): 603-623.
[13] Ouellette J N, Drifka C R, Pointer K B, et al. Navigating the collagen jungle: the biomedical potential of fiber organization in cancer[J]. Bioengineering, 2021, 8(2): 17.
[14] Golaraei A, Kontenis L, Cisek R, et al. Changes of collagen ultrastructure in breast cancer tissue determined by second-harmonic generation double Stokes-Mueller polarimetric microscopy[J]. Biomedical Optics Express, 2016, 7(10): 4054-4068.
[15] 沈元星, 姚悦, 何宏辉, 等. 非标记、定量化穆勒矩阵偏振成像在辅助临床诊断中的应用[J]. 中国激光, 2020, 47(2): 0207001.
[16] Westreich J, Khorasani M, Jones B, et al. Novel methodology to image stromal tissue and assess its morphological features with polarized light: towards a tumour microenvironment prognostic signature[J]. Biomedical Optics Express, 2019, 10(8): 3963-3973.
[17] Kooi T, Litjens G, van Ginneken B, et al. Large scale deep learning for computer aided detection of mammographic lesions[J]. Medical Image Analysis, 2017, 35: 303-312.
[18] 高大川, 聂生东. 结合深度卷积神经网络与影像学特征的肺结节良恶性鉴别方法[J]. 光学学报, 2020, 40(24): 2410002.
[19] 杜剑, 胡炳樑, 张周锋. 基于卷积神经网络与显微高光谱的胃癌组织分类方法研究[J]. 光学学报, 2018, 38(6): 0617001.
[20] RonnebergerO, FischerP, BroxT. U-net: convolutional networks for biomedical image segmentation[M]//Navab N, Hornegger J, Wells W M, et al.Medical image computing and computer-assisted intervention-MICCAI 2015. Lecture Notes in Computer Science. Cham: Springer, 2015, 9351: 234-241.
[21] Marchetti M A, Codella N C F, Dusza S W, et al. Results of the 2016 International Skin Imaging Collaboration International Symposium on Biomedical Imaging challenge: comparison of the accuracy of computer algorithms to dermatologists for the diagnosis of melanoma from dermoscopic images[J]. Journal of the American Academy of Dermatology, 2018, 78(2): 270-277.e1.
[22] Ali N, Quansah E, Köhler K, et al. Automatic label-free detection of breast cancer using nonlinear multimodal imaging and the convolutional neural network ResNet50[J]. Translational Biophotonics, 2019, 1(1/2): 201900003.
[23] Meyer T, Guntinas-Lichius O, von Eggeling F, et al. Multimodal nonlinear microscopic investigations on head and neck squamous cell carcinoma: toward intraoperative imaging[J]. Head & Neck, 2013, 35(9): E280-E287.
[24] Meyer T, Bergner N, Krafft C, et al. Nonlinear microscopy, infrared, and Raman microspectroscopy for brain tumor analysis[J]. Journal of Biomedical Optics, 2011, 16(2): 021113.
[25] Liu G, Zhang M K, He Y, et al. BI-RADS 4 breast lesions: could multi-mode ultrasound be helpful for their diagnosis?[J]. Gland Surgery, 2019, 8(3): 258-270.
[26] Leng X L, Huang G F, Yao L H, et al. Role of multi-mode ultrasound in the diagnosis of level 4 BI-RADS breast lesions and logistic regression model[J]. International Journal of Clinical and Experimental Medicine, 2015, 8(9): 15889-15899.
[27] Lieli R P, Hsu Y C. Using the area under an estimated ROC curve to test the adequacy of binary predictors[J]. Journal of Nonparametric Statistics, 2019, 31(1): 100-130.
[28] Zaffar M, Pradhan A. Assessment of anisotropy of collagen structures through spatial frequencies of Mueller matrix images for cervical pre-cancer detection[J]. Applied Optics, 2020, 59(4): 1237-1248.
[29] Dong Y, Wan J C, Si L, et al. Deriving polarimetry feature parameters to characterize microstructural features in histological sections of breast tissues[J]. IEEE Transactions on Biomedical Engineering, 2021, 68(3): 881-892.
Article Outline
许志兵, 吴进锦, 丁路, 王子函, 周苏伟, 尚慧, 王慧捷, 尹建华. 基于偏振和明场多模态显微成像技术的乳腺癌智能诊断研究[J]. 中国激光, 2022, 49(24): 2407102. Zhibing Xu, Jinjin Wu, Lu Ding, Zihan Wang, Suwei Zhou, Hui Shang, Huijie Wang, Jianhua Yin. Intelligent Diagnosis of Breast Cancer Based on Polarization and Bright-Field Multimodal Microscopic Imaging[J]. Chinese Journal of Lasers, 2022, 49(24): 2407102.