浮游藻类细胞显微明场图像与荧光同步测量图像配准方法研究
下载: 618次
Detection of phytoplankton diversity is an important part of a water quality bioassessment. The traditional manual microscopic detection of algae species requires professional operation and is time-consuming and laborious; therefore, these challenges can be overcome by the development of a method for automatic identification of phytoplankton cell images. Similar to manual identification, deep learning and other automatic identification technologies identify phytoplankton cells based on the morphological characteristics of bright field cell images; however, the practical applications of such technologies encounter complications, such as the difficulty of accurate segmentation of phytoplankton cells and the limitation in high recognition accuracy of algae species only with a small range of groups. Previous studies have shown that the accuracy of algal cell segmentation and recognition can be effectively improved by fusing the bright field and fluorescence images of phytoplankton cells. However, the fusion of bright field and fluorescence images synchronously measured from phytoplankton cells requires significantly high accuracy and shockproof capability of the mechanical structure of the acquisition system. Under a high-power microscope, even a small error in the mechanical structure or a small vibration of the camera can lead to a dislocation between the bright field and fluorescence images, thus causing difficulties in the fusion of the images. Therefore, the registration of bright field and fluorescence images of algae is of great significance for the automatic identification of phytoplankton.
The displacement between bright field and fluorescence images can be represented by a rigid transformation model, which consists of three parameters: the translation in the x direction, translation in the y direction, and rotation angle. Normalized mutual information is used to calculate the similarity between the bright field and fluorescence images. The goal of image registration is to find a set of rigid transformation parameters that maximize the normalized mutual information of the two images. Owing to the significant difference between the bright field and fluorescence images of phytoplankton cells, the similarity is difficult to be characterized by directly calculating the normalized mutual information. In this study, the normalized mutual information of the S channel binary image of the bright field HSV color space and the binary image of the fluorescence gray level was assumed as the similarity between the bright field and fluorescence images. The S component of the bright field HSV image and the fluorescence gray image were decomposed using a two-dimensional discrete wavelet transform, and the low-frequency components were binarized, to accelerate the registration. First, the particle swarm algorithm was used to register the low-frequency components of the five-level wavelet decomposition. Subsequently, the translation and rotation angle of the preliminary registration were assumed as the initial values, and the low-frequency components of the wavelet three-level decomposition are further registered using Powell’s algorithm.
Scenedesmus sp., Selenastrum capricornutum, and Nostoc sp. are used as experimental objects. The similarity and registration methods are compared and analyzed. As shown in Figs. 3 and 4, the normalized mutual information of the bright field S channel and the fluorescence grayscale image has an obvious peak value after binarization, which is extremely conducive to parameter optimization in the registration process. As shown in Fig. 5, after the bright field and fluorescence images are decomposed by the wavelet, the noise in the high-frequency component is concentrated, and the low-frequency component has a higher normalized mutual information. Therefore, the normalized mutual information of binary images of low-frequency components after wavelet decomposition is chosen as the similarity measurement index for bright field and fluorescence images. Table 1 presents an experimental comparison between the proposed method and other registration methods. Compared with particle swarm optimization algorithm, the proposed method reduces the mismatch rate by 0, 8.1, and 0.7 percentage points, shortens the running time by 128.26 s, 448.95 s and 237.20 s, and improves the normalized mutual information by 0.065, 0.083, and 0.106; Powell’s method depends on the initial value; therefore, it is easy to fall into the local maximum in the process of optimization, which leads to a high mismatch rate; compared with GA, the mismatch rates of proposed method are reduced by 6.1, 26.2, and 10.2 percentage points, the running time is shortened by 23.78 s, 60.95 s and 33.74 s, and the normalized mutual information after registration is improved by 0.149, 0.170, and 0.180. The experimental results demonstrate that the proposed method has obvious advantages in terms of registration accuracy and running time compared with other registration methods.
In this study, the bright field image is converted into a binary image by processing the S channel of the HSV color space; the fluorescence gray image is binarized; normalized mutual information is used as the similarity criterion to characterize the similarity of the bright field and fluorescence image. Using Scenedesmus sp., Selenastrum capricornutum, and Nostoc sp. as experimental objects, the application of wavelet decomposition in the registration of microscopic bright field and fluorescence images of phytoplankton cells was studied. The global search advantage of the particle swarm optimization algorithm is used for preliminary registration in the high-level decomposition component, and the local search ability of Powell algorithm is used for fine-tuning the registration accuracy in the low-level decomposition component. A comparative analysis is performed with other commonly used registration methods, and the experimental results verify the feasibility of the registration method.
1 引言
浮游藻类多样性检测是水质生物评价的重要组成部分。传统的藻类群落结构的显微镜检法需要专业人员进行操作,且耗时费力。因此,发展浮游藻类细胞图像的自动鉴定方法十分有必要[1-3]。与人工识别类似,深度学习[4-6]等自动识别技术根据明场细胞图像的形态特征参数对浮游植物藻类细胞进行鉴定。然而,实际应用中常常存在精确分割浮游藻类细胞困难[7]、高识别精度仅限于类群范围较小的藻种等问题[4]。研究人员[8-9]通过对显微镜的同一个视场分别采集浮游藻类细胞的明场图像和自发荧光图像,然后利用阈值法等图像处理技术对荧光图像进行分割,并将分割结果映射到明场图像中,实现了对浮游藻类细胞图像的分割。Dunker等[10]的研究结果表明,通过融合浮游藻类细胞的明场图像和荧光图像,可以有效提高浮游藻类细胞的识别准确率。然而,融合明场图像和荧光图像需要建立在明场图像与荧光图像已经配准的基础之上。在采用显微镜对浮游植物进行显微图像采集过程中,采集平台的微小抖动等不可控因素往往会导致高倍显微镜下明场图像与荧光图像的错位,这种情况在野外场景下尤为严重,给明场图像与荧光图像的融合带来了一定困难。实现藻类细胞明场图像与荧光图像的配准是实现浮游植物自动鉴定等的基础。目前,有关如何实现浮游植物藻类细胞明场图像与荧光图像配准的文献较少。Wang等[11]通过图像配准方法将藻的明场图像与叶绿素的荧光图像进行配准,然后利用明场图像获取藻的形态特征,同时利用叶绿素荧光图像获取藻的活性特征,实现了船舶压载水中藻活性和藻浓度的同时检测;但配准结果如何,他们没有进一步讨论。经典的图像配准方法有遗传算法(GA)、鲍威尔算法(Powell)、粒子群优化算法(PSO)等[12-13],其中:鲍威尔算法具有较好的局部搜索能力,但该算法依赖于初始值,易陷入初始值附近的局部极小值;粒子群优化算法具有较好的全局搜索能力,可以使配准参数逐渐收敛至全局最优解附近[14]。浮游藻类细胞显微图像的数据量较大,导致计算归一化互信息比较耗时。於时才等[15]的研究表明,对图像进行小波分解可以实现图像的快速配准。为了实现浮游藻类细胞显微明场图像与荧光图像的有效快速配准,本团队以归一化互信息为相似度度量方法,将多分辨率图像配准技术应用到浮游藻类细胞显微明场图像与荧光图像的配准中,并通过与其他配准方法进行对比验证了所提方法的有效性。
2 实验与方法
2.1 浮游藻类细胞显微明场图像和显微荧光图像的采集
采用中国科学院安徽光学精密机械研究所研制的双光路成像系统采集实验室培养的栅藻(Scenedesmus sp.)、羊角月牙藻(Selenastrum capricornutum)、念珠藻(Nostoc sp.)三种浮游藻类细胞的显微明场图像和显微荧光图像。藻种均采购于中国科学院水生生物研究所淡水藻种库。
双光路成像系统的结构如
2.2 藻的显微荧光图像与显微明场图像的配准方法
图像的配准模型可以用刚体变换模型表示。假设荧光图像中的点(x,y)旋转θ角度并在x方向平移tx、在y方向平移ty后到达明场图像上的点(x′,y′),则可以通过式(1)表示变换模型。
归一化互信息(NMI)被用于计算明场图像与荧光图像的相似度,计算公式为
由于浮游植物藻类细胞显微明场图像和荧光图像的差异比较大,直接计算两幅图像的归一化互信息难以表征其相似度。在藻类的显微明场图像中,藻的颜色特征十分明显,如绿藻呈现绿色而蓝藻呈现蓝色,因而将采集的RGB(Red Green Blue)格式转换为HSV(Hue Saturation Value)颜色空间,利用饱和度S通道较好地定位藻所在区域[18]。如
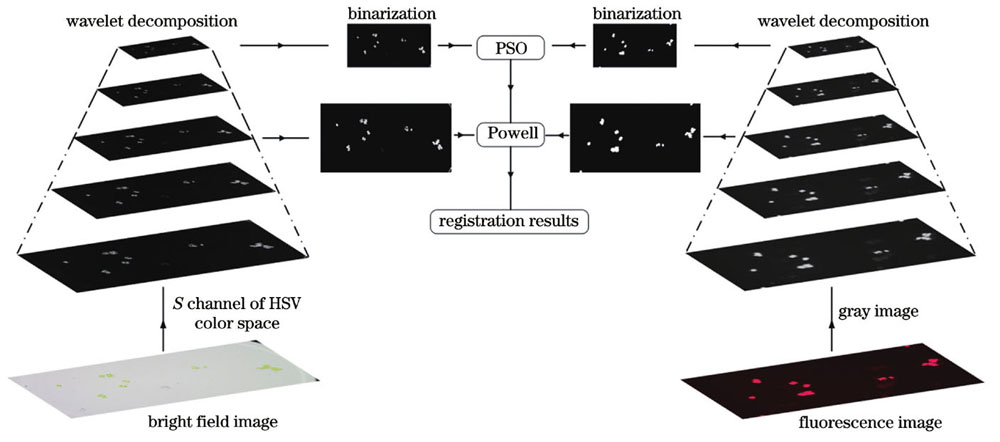
图 2. 明场图像与荧光图像配准方法的流程图
Fig. 2. Flow chart of bright field and fluorescence images registration method
综上所述,浮游植物藻类细胞显微明场图像与荧光图像配准方法的具体执行步骤如下:
1) 将明场RGB格式图像转换为HSV格式图像,得到明场S分量图像;
2) 对荧光图像进行灰度化处理,得到荧光灰度图像;
3) 采用小波变换分别对明场S分量和荧光灰度图像进行五级分解,得到分解后各自的低频分量,并对低频分量进行二值化处理;
4) 利用粒子群优化算法对五级分解的低频分量进行粗配准,得到初步配准的平移量tx、ty和旋转角度θ;
5) 将平移量 4tx、4ty和旋转角度θ[19]设置为初始位置,利用鲍威尔算法对三级分解的低频分量进行细配准,从而对平移量和旋转角度进行微调。
3 实验结果与分析
3.1 藻样显微明场图像与荧光图像的相似度分析
实现图像配准需要找到一种可有效计算显微明场图像与荧光图像相似度的方法。本节以栅藻为例,分析2.2节中的图像处理对显微明场图像与荧光图像相似度的影响。
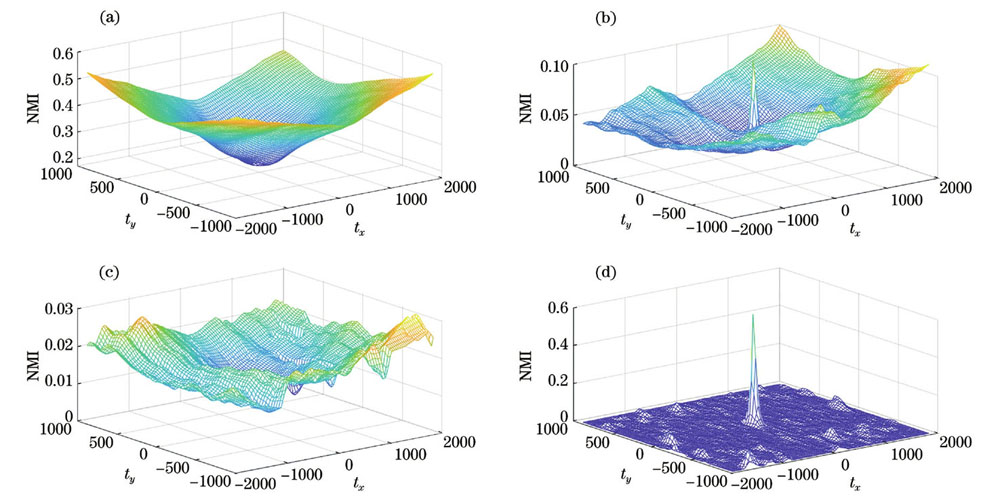
图 3. 平移量与归一化互信息。(a) 明场灰度图像与荧光灰度图像;(b)明场S通道与荧光灰度图像;(c)明场灰度二值化图像与荧光灰度二值化图像;(d)明场S通道二值化图像与荧光灰度二值化图像
Fig. 3. Translation and normalized mutual information. (a) Gray image of bright field and fluorescence; (b) bright field S channel and fluorescence gray image; (c) binarized gray image of bright field and fluorescence; (d) binarization of bright field S channel and fluorescence gray image
因此,藻细胞的显微明场S通道二值化图像与荧光灰度二值化图像可以更好地表征藻细胞明场图像与荧光图像的相似度,可将其作为实现藻种明场图像与荧光图像配准的相似度评价指标。
然而,显微藻细胞图像数据量大,直接对其进行配准比较耗时。为加快显微藻细胞图像配准的速度,采用二维离散小波变换对显微藻细胞图像进行处理。
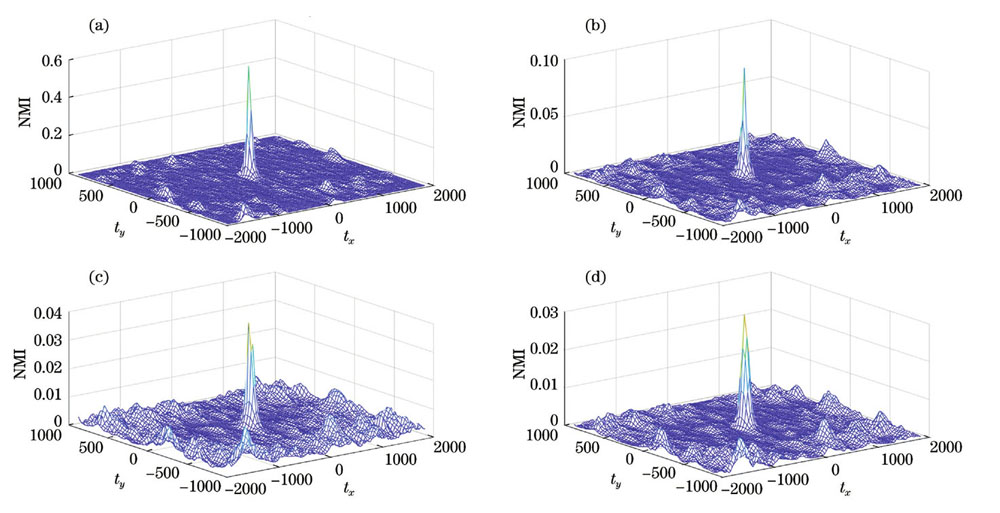
图 5. 小波分解后各分量的归一化互信息。(a)低频分量;(b)水平高频分量;(c)垂直高频分量;(d)对角高频分量
Fig. 5. Normalized mutual information after wavelet decomposition. (a) Low frequency component; (b) horizontal high frequency component; (c) vertical high frequency component; (d) diagonal high frequency component
由于高频分量容易受到噪声、纹理细节等的影响,藻样明场S通道与荧光灰度图像进行小波分解后,各自低频分量二值化图像的归一化互信息可以达到0.5,而其他归一化互信息均小于0.1。因此,选择小波分解后的低频分量二值化图像的归一化互信息作为藻样明场图像与荧光图像相似度的度量指标。接下来将在此基础上对配准方法进行详细的对比分析。
3.2 藻样显微明场图像与荧光图像配准方法的比较分析
由3.1节所述藻样显微明场图像和荧光图像的相似度分析可知,可以将显微明场S通道图像与荧光灰度图像的小波分解低频二值化图像的归一化互信息作为图像的相似度指标。
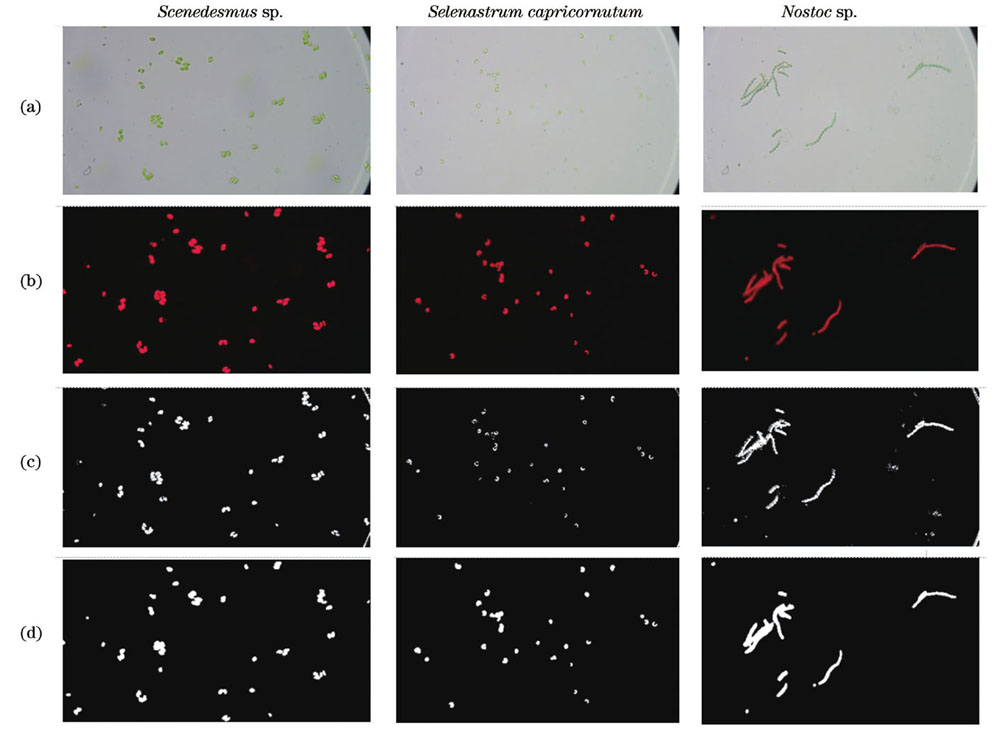
图 6. 栅藻、羊角月牙藻和念珠藻细胞的显微明场图像和荧光图像。(a)明场图像;(b)荧光图像;(c)明场S通道三级小波分解的低频分量的二值化图像;(d)荧光灰度图像三级小波分解的低频分量的二值化图像
Fig. 6. Bright field and fluorescence images of cells of Scenedesmus sp., Selenastrum capricornutum and Nostoc sp. (a) Bright images; (b) fluorescence images; (c) binarization images of low frequency component of three-level wavelet decomposition in bright field S channel; (d) binarization images of low frequency component of three-level wavelet decomposition in fluorescence gray image
通过明场图像与荧光图像进行像素级加法融合可以直观地观察出是否配准成功。
实验使用2.1节所示双光路成像系统分别采集栅藻、羊角月牙藻、念珠藻各160张显微藻细胞图像。从主观评价和客观评价两个角度对配准方法进行分析,通过人眼观察的形式统计误配率进行主观评价,采用配准后的相似度NMI和运行时间进行客观评价。由3.1节分析可知,当明场图像与荧光图像配准时,两张图像的相似度NMI应达到最大值。
表 1. 明场图像与荧光图像配准方法的对比
Table 1. Comparison of bright field and fluorescence images registration methods
|
对于栅藻、羊角月牙藻、念珠藻图像,本文方法与粒子群优化算法相比误配率分别降低了0、8.1、0.7个百分点,运行时间分别缩短了128.26、448.95、237.20 s,配准后的归一化互信息分别提高了0.065、0.083、0.106。鲍威尔算法的配准效果依赖于初始值,在寻优过程中易陷入局部最小值,导致其具有较高的误配率。与遗传算法相比,本文方法的误配率分别降低了6.1、26.2、10.2个百分点,运行时间分别缩短了23.78、60.95、33.74 s,配准后的归一化互信息分别提高了0.149、0.170、0.180。同时可以观察到,藻的大小会对配准结果产生一定影响。如:羊角月牙藻体积较小,导致其配准时间长于栅藻和念珠藻,配准精度略低于栅藻和念珠藻。实验结果表明,与其他配准方法相比,所提方法在配准精度、运行时间等方面具有明显优势。
4 结论
利用浮游藻类细胞颜色特征明显的特点,本团队通过对明场图像HSV颜色空间的S通道进行处理,将明场图像转化为二值化图像,并对荧光灰度图像进行二值化处理,采用归一化互信息作为相似度度量指标,较好地表征了明场图像与荧光图像的相似度。以栅藻等藻种为例,研究了小波分解在浮游藻类细胞显微明场图像与荧光图像配准中的应用。利用粒子群优化算法的全局搜索优势对高层分解分量进行初步配准,使用鲍威尔算法的局部搜索能力对底层分解分量进行配准精度微调。将所提方法与其他常用配准方法(如遗传算法)进行了对比分析,实验结果验证了本文所提配准方法的可行性。
[1] Schulze K, Tillich U M, Dandekar T, et al. PlanktoVision: an automated analysis system for the identification of phytoplankton[J]. BMC Bioinformatics, 2013, 14: 115.
[2] Wei L, Su K, Zhu S Q, et al. Identification of microalgae by hyperspectral microscopic imaging system[J]. Spectroscopy Letters, 2017, 50(1): 59-63.
[3] First M R, Drake L A. Performance of the human “counting machine”:evaluation of manual microscopy for enumerating plankton[J]. Journal of Plankton Research, 2012, 34(12): 1028-1041.
[4] Yadav D P, Jalal A S, Garlapati D, et al. Deep learning-based ResNeXt model in phycological studies for future[J]. Algal Research, 2020, 50: 102018.
[5] 柯宝生, 李颖, 任振波, 等. 基于深度学习的活体细胞有丝分裂检测方法[J]. 光学学报, 2021, 41(15): 1511001.
[6] 梁霄, 李家炜, 赵小龙, 等. 基于深度学习的红外目标成像液位检测方法[J]. 光学学报, 2021, 41(21): 2110001.
[7] Borges V R P, Oliveira M, Silva T G, et al. Region growing for segmenting green microalgae images[J]. IEEE/ACM Transactions on Computational Biology and Bioinformatics, 2018, 15(1): 257-270.
[8] Hense B A, Gais P, Jütting U, et al. Use of fluorescence information for automated phytoplankton investigation by image analysis[J]. Journal of Plankton Research, 2008, 30(5): 587-606.
[9] Verikas A, Gelzinis A, Bacauskiene M, et al. An integrated approach to analysis of phytoplankton images[J]. IEEE Journal of Oceanic Engineering, 2015, 40(2): 315-326.
[10] Dunker S, Boho D, Wäldchen J, et al. Combining high-throughput imaging flow cytometry and deep learning for efficient species and life-cycle stage identification of phytoplankton[J]. BMC Ecology, 2018, 18(1): 51.
[11] Wang Y J, Wang J S, Wang T Q, et al. Simultaneous detection of viability and concentration of microalgae cells based on chlorophyll fluorescence and bright field dual imaging[J]. Micromachines, 2021, 12(8): 896.
[12] 陈显毅. 图像配准技术及其MATLAB编程实现[M]. 北京:电子工业出版社,2009: 93-134.
ChenX Y. Image registration technology and its MATLAB programming implementation[M]. Beijing: Publishing House of Electronics Industry, 2009: 93-134.
[13] 柏连发, 韩静, 张毅, 等. 采用改进梯度互信息和粒子群优化算法的红外与可见光图像配准算法[J]. 红外与激光工程, 2012, 41(1): 248-254.
Bai L F, Han J, Zhang Y, et al. Registration algorithm of infrared and visible images based on improved gradient normalized mutual information and particle swarm optimization[J]. Infrared and Laser Engineering, 2012, 41(1): 248-254.
[14] 吴建辉, 章兢, 陈红安. 融合Powell搜索法的粒子群优化算法[J]. 控制与决策, 2012, 27(3): 343-348, 354.
Wu J H, Zhang J, Chen H A. Particle swarm optimization algorithm combination with Powell search method[J]. Control and Decision, 2012, 27(3): 343-348, 354.
[15] 於时才, 吕艳琼. 一种图像快速配准算法的研究[J]. 激光与红外, 2009, 39(4): 447-449.
Yu S C, Lü Y Q. Study of image registration fast algorithm[J]. Laser & Infrared, 2009, 39(4): 447-449.
[16] 殷高方, 赵南京, 胡丽, 等. 基于色素特征荧光光谱的浮游植物分类测量方法[J]. 光学学报, 2014, 34(9): 0930005.
[17] 程钊, 赵南京, 殷高方, 等. 基于SWTATLD算法的藻类群落离散三维荧光光谱识别方法[J]. 光学学报, 2021, 41(14): 1430001.
[18] Zheng H Y, Wang N, Yu Z B, et al. Robust and automatic cell detection and segmentation from microscopic images of non-setae phytoplankton species[J]. IET Image Processing, 2017, 11(11): 1077-1085.
[19] 刘斌, 彭嘉雄. 图像配准的小波分解方法[J]. 计算机辅助设计与图形学学报, 2003, 15(9): 1070-1073.
Liu B, Peng J X. Wavelet decomposition based image registration[J]. Journal of Computer Aided Design & Computer Graphics, 2003, 15(9): 1070-1073.
Article Outline
贾仁庆, 殷高方, 赵南京, 徐敏, 胡翔, 黄朋, 梁天泓, 何前锋, 陈晓伟, 甘婷婷, 张小玲, 马明俊. 浮游藻类细胞显微明场图像与荧光同步测量图像配准方法研究[J]. 中国激光, 2022, 49(24): 2407202. Renqing Jia, Gaofang Yin, Nanjing Zhao, Min Xu, Xiang Hu, Peng Huang, Tianhong Liang, Qianfeng He, Xiaowei Chen, Tingting Gan, Xiaoling Zhang, Mingjun Ma. Registration Method of Microscopic Bright Field and Fluorescence Synchronous Measurement Images of Phytoplankton Cells[J]. Chinese Journal of Lasers, 2022, 49(24): 2407202.