拉曼分布式光纤温度传感:技术发展与应用综述
下载: 1669次特邀综述
Temperature measurement is a common requirement in the daily life of human beings and production activities. Abnormal and abrupt temperature variations in such diverse important fields as civil engineering, industrial machinery, aerospace, and infrastructure can cause significant economic losses and are even life-threatening. High-accuracy and real-time measurements of temperature distributions are thus demanding, and different technologies have been developed accordingly. Specifically, optical fiber-based temperature sensors have been demonstrated as unparalleled modalities in harsh-environment applications, which is due to their distinct advantages over conventional electrical devices, such as small size, light weight, immunity to electromagnetic interference and chemical corrosion, and importantly, the capability for spatially distributed sensing.
As one of the most important optical fiber temperature sensing technologies, Raman distributed optical fiber temperature sensing (RDTS) systems have outstanding advantages of electromagnetic compatibility, long sensing distance, and wide temperature range. Thus, they are widely adopted in the safety monitoring of infrastructure structures, the oil and gas industry, fire detection, and many other fields. The first RDTS system can be dated back to 1985, when a sensing distance of 1 km is realized with a spatial resolution of 3 m based on the first generation of opto-electronic devices. Recent years have witnessed tremendous growth and advancement in light sources, detectors, sensing elements (i.e., optical fibers), and signal demodulation techniques, which leads to new generations of RDTS systems with significantly enhanced performance measures. It is of significance to summarize and discuss the existing research progress and future development trends for further development in RDTS systems.
We review the advanced RDTS technology based on the performance improvement methods mainly in three aspects of system optimization, temperature demodulation, and data processing. Firstly, the system optimization is focused on the system structures and components. The structure optimization is mainly conducted as the double-ended or loop structure, which involves the adoption of optical switches or an additional mirror at the optical fiber end to reduce the uncertainty of measured temperature in the long-term utilization. The components optimization mainly involves the optimization of the light source, detector, and sensing fiber. In the light source, related research concentrates on the wavelength and pulse width selection, application of pulse coding technology, and employment of new-type light sources. In terms of detectors, with the rapid development of single-photon detectors, their applications in ROTDR systems are bound to bring greater performance improvement. In terms of sensing fiber, the adoption of dispersion shift fiber (DSF), dispersion compensation fiber (DCF), and low water peak fiber provide a new idea for long-distance sensing. Few-mode fibers (FMFs) combine the advantages of single-mode and multi-mode fibers and yield better performance in RDTS systems. Secondly, in temperature demodulation, a variety of calibration and compensation methods are summarized in the problems of optical power fluctuation, Rayleigh scattered light residue, and differential temperature sensitivity for Stokes and anti-Stokes. Thirdly, the data processing mainly involves the applications of various denoising algorithms to improve the spatial resolution and signal-to-noise ratio (SNR) of the systems.
Subsequently, a global market survey of RDTS systems is summarized. The main research and development institutions and manufacturers at home and abroad are listed by combining the performance comparison of their typical products. The typical applications in various engineering scenarios are presented.
The current research achievements of RDTS systems can be comprehensively summarized from three perspectives including system optimization, temperature demodulation, and data processing. In terms of system optimization, pulse coding technology can enhance the SNR by over 10 dB without compromising the system's spatial resolution. The application of genetic optimization algorithms to find the optimal pulse coding sequence maximizes the advantages of pulse coding, leading to more than seven-fold improvement in temperature resolution. By introducing chaotic laser sources into the RDTS system and combining relevant demodulation algorithms, the spatial resolution can be increased from 50 m to 0.3 m. The utilization of FMFs provides a viable solution to overcome both the low SRS threshold of single-mode fibers (SMFs) and the large modal dispersion of multi-mode fibers (MMFs). Compared to SMF (MMF), this approach significantly enhances system temperature (spatial) resolution with minimal influence on spatial (temperature) resolution. In the context of temperature demodulation, various calculation methods of temperature calibration are introduced to mitigate the effect of system power fluctuations, signal crosstalk, and losses on measurement results. Appropriate calibration methods can improve temperature accuracy by over 14 ℃. Regarding data processing, in recent years, algorithms based on image processing and artificial intelligence have been successively applied to RDTS systems, both improving SNR and contributing to spatial resolution enhancement. Under the applications of these algorithms, spatial resolution can increase by five times, and temperature resolution reaches the order of 0.01 ℃.
Furthermore, we summarize the domestic and international market trends of the RDTS systems and major vendors and compile information on typical products offered by these vendors. However, compared to foreign products, there is room for further improvement in the technical indicators of domestic products.
With an increasing number of proposed ideas, there is considerable potential for enhancing the performance of RDTS systems. Achieving miniaturization by device integration and enhancing portability is a long-term theme for the development. The application and optimization of various artificial intelligence algorithms will also equip the systems with faster processing speeds and superior performance. Additionally, rational design optimization of sensing optical fibers and other system-related aspects could further expand the systems' applications in extreme environments such as ultra-high and low temperatures, and nuclear radiation. Many potential applications of this deserve further exploration.
1 引言
温度传感器在人类日常生活及生产活动中随处可见,随着其应用范围的日益扩大,实现温度传感的方法也在不断改进。传统的电式温度传感器通常基于热电偶或热电阻实现温度传感。而绝大部分电式温度传感器都是点式传感器,无法实现复用或分布式测量。值得一提的是,近年来,基于射频同轴电缆的传感技术得到了一定的发展。通过在一根同轴电缆中引入两个反射点,形成了一个微波频率下的法布里-珀罗(FP)干涉仪,甚至通过多个微波FP腔级联实现准分布式温度探测[1-3]。然而,同轴电缆体积较大,传输损耗高,抗电磁干扰能力劣于光纤传感器,不适用于长距离传感应用。近年来,基于光纤的传感技术得益于体积小、质量轻、本征抗电磁干扰等特性得到了迅速发展。其中,光纤温度传感器有望取代机电式温度传感器,成为一些复杂应用环境中最有潜力的温度传感解决方案[4]。
基于光纤布拉格光栅(FBG)的传感技术[5]、光纤干涉型传感技术[6-7](马赫-曾德尔干涉仪以及FP干涉仪等)以及光纤分布式温度传感(DTS)技术[8]是光纤温度传感中的几种重要技术。基于FBG的超长阵列可以实现准分布式温度测量[9-10],虽数量上可以达到上万个传感单元,但解调难度增大且易受应变串扰影响。干涉型传感技术通常只能实现单点式监测[11]。相比上述两者,分布式光纤温度传感(DFTS)系统能够提供连续的时空温度分布信息,具有测量距离长、监测范围广的突出优势。
DFTS系统的探测原理主要基于光纤中后向散射光的探测,包括瑞利散射、布里渊散射和拉曼散射三种,温度的变化导致散射光特性的改变,由此得到测量环境的温度分布[12-14]。其中,瑞利散射光随温度的变化较小,且瑞利和布里渊散射过程同时受到应变和温度的影响,因此在工业应用中并非测量温度的最佳手段。而拉曼散射过程只对温度变化敏感,目前基于拉曼散射的RDTS技术是DTS中最知名和成熟的技术。其实现方式基于光时域反射(OTDR)或光频域反射(OFDR)技术,其中OTDR技术应用更为广泛。最早的RDTS系统可以追溯至1985年,当时的系统实现了1 km传感距离内空间分辨率大约3 m的指标[15]。近几十年间,随着相关技术的不断探索优化,目前RDTS系统的最长传感距离已经达到80 km以上[16],在百米级传感距离下空间分辨率可以提升至厘米级[17-22]。
本文从系统性能优化和应用两方面综述了拉曼分布式温度传感系统的最新研究进展。第二节介绍了拉曼分布式温度传感的基本原理。第三节从系统结构及部件、温度校准以及信号处理等方面综述了目前针对RDTS系统性能优化的研究进展。第四节报道了RDTS系统的市场调研结果。第五节内容为RDTS系统在各领域的应用。第六节是对本文内容的总结。
2 拉曼分布式温度传感原理
2.1 拉曼散射及光时域反射基本理论
光在非均匀介质中传播时与物质相互作用,从而使得一部分光散射开来的现象称为光散射。在经典电磁理论中,介质分子振动导致极化率的改变,在频率为ω0的光场作用下形成感生电偶极子。电偶极子振荡辐射出频率为ω0-ωm和ω0+ωm的拉曼散射光,分别称为Stokes光和Anti-Stokes光。在量子理论中,拉曼散射光的强度与分子的能级分布有关,服从玻色-爱因斯坦统计分布[23],如
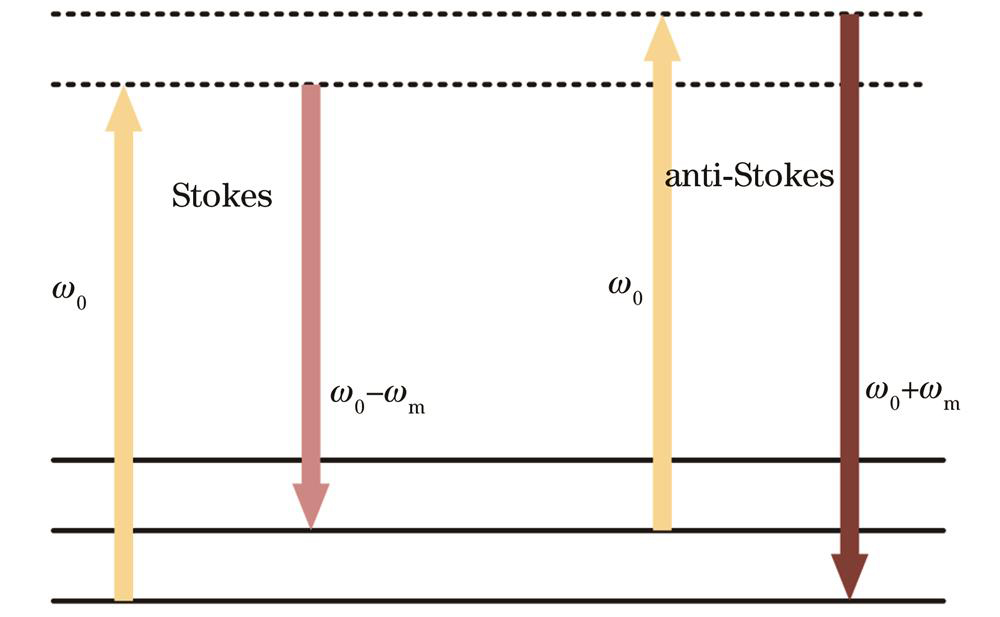
图 1. 拉曼散射中分子能级跃迁示意图
Fig. 1. Schematic diagram of the molecular energy level transition in Raman scattering
1976年,首次提出OTDR分布式传感技术,用于检测光纤的损耗等特性[24]。OTDR技术的原理如
式中:n为光纤的折射率。因此可将采集到信号的时间定位到光纤的各处测量位置。理想情况下,OTDR技术传感距离的上限Lmax取决于脉冲光源的重复频率fp,而空间分辨率δL可由光脉冲的脉宽τ获得,
在RDTS系统中,当光纤某位置处温度变化时,拉曼信号对应位置处的强度就会发生相应的波动。由此得到光纤各测量位置处的温度。测量温度T可由Anti-Stokes光和Stokes光的信号强度比R计算得到
式中:
式中:Ns为数字平均带来的信噪比提升的倍数。
2.2 光频域反射技术
一般而言,OFDR技术分为相干(COFDR)和非相干(ICOFDR)两种。在RDTS系统中主要使用的是ICOFDR技术。ICOFDR技术所需基本装置与OTDR类似,不同之处在于其光源是波长连续(CW)光在正弦射频信号调制下获得的,其技术原理如
在ICOFDR技术中,系统可测量的最长传感距离
ICOFDR技术相比于OTDR技术在提高系统信噪比(SNR)上有明显优势。一方面,ICOFDR技术的光源为正弦调制的CW光,相比于脉冲光,耦合入光纤的光能量更大,能获得更强的拉曼散射信号,因此也降低了对探测器放大能力的要求。另一方面,因为ICOFDR技术中频域信号是通过光源频率的步进逐点测得的,每次测量所需的探测器带宽相对较小,因此噪声也得以降低,可以获得较高的SNR。除此之外,ICOFDR技术可以使用激光二极管代替昂贵的脉冲光源,具有更高的硬件成本优势。但ICOFDR技术需要同时记录信号的强度和相位,对信号探测提出了更多的要求,此外,相比于OTDR技术,ICOFDR的信号处理系统更加复杂。目前,市场上的拉曼OFDR产品主要来自LIOS(现已被LUNA收购),其可实现的空间分辨率高于25 cm[26]。
在OTDR和OFDR技术中,前者仍是目前RDTS系统中最成熟和应用最广泛的技术。本文的重点集中在基于OTDR技术的拉曼分布式温度传感系统上。
3 RDTS系统的优化
RDTS系统主要的性能参数包括传感距离、空间分辨率、温度分辨率、温度精度和测量时间等方面。
传感距离上限由脉冲的重复频率决定。在实际应用中,考虑到后续的信号探测和处理过程,传感距离还与光在光纤中的衰减以及系统噪声等情况有关[27]。系统空间分辨率的提升可以从较窄的脉冲宽度、较大的探测器带宽,以及减小传感光纤的色散等方面入手[28]。温度分辨率与系统的信噪比和数据采集过程的分辨率有关,主要涉及系统噪声的处理[29]。温度精度除与上述两者有关外,也会受到环境波动以及损耗的影响[30]。系统的测量时间与需要达到的其他指标相对应,增大数据平均次数可以提高系统信噪比,但测量时间也会随之增加[31-32]。
RDTS系统的优化涉及到多种学科的交叉,系统性能的优化可从光、电、算等多个方面进行,旨在提升上述性能参数。拉曼散射光本身强度微弱,且由于受激拉曼散射阈值的限制难以进行光学预放大,因此一般需要综合多种手段达到最佳效果。
近些年来,RDTS系统性能优化的研究主要从三方面入手:系统结构及部件优化,温度解调优化以及信号处理优化。本章将上述三方面内容分为三小节阐述,在每一节中将相关的进展按其提升的目标参数进行分类。
3.1 系统结构及部件优化
RDTS的系统部件主要有光源、滤波器、传感光纤、光电探测器和数据采集模块等。通过系统结构和部件的优化,可以从硬件方面改善系统配置,提升系统性能。
3.1.1 温度精度与分辨率提升
1)系统结构
系统结构的优化目的主要在于温度精度的提升。
传感光纤与系统的连接方式除了传统的单端检测的形式外,还有利用光开关实现从传感光纤两端的信号检测的双端检测(环路检测)结构,其结构示意图如

图 5. RDTS系统双端检测结构示意图
Fig. 5. Schematic diagram of the double-ended detection structure of RDTS system
双端检测结构的原理如下,传感光纤从中间位置弯曲,两端均与系统相连,每一个测温点处对应两个光纤位置点。若传感光纤总长l,那么距离光纤一端z位置处应与l-z处对应同一个测温点,因此温度一致,此处用于温度解调的拉曼散射光强度比可由光沿前向传输时z处和后向传输时l-z处的散射光强度比的几何平均值
式中:
2011年,意大利Scuola Superiore Sant’Anna的Soto等[34]基于双端检测结构和单路解调方案,使用1550 nm波段、脉宽10 ns、输出平均功率1.5 mW的脉冲光源在40 s测量时间内,在9 km测量距离处实现了1.1 ℃的温度分辨率。2013年,山东大学的Wang等[33]报道了使用2.5 km长的多模光纤(MMF)双端检测RDTS系统。其使用最大峰值功率30 W的脉冲光源,经过10 s累加平均时间,在120 m测量距离处将温度精度由单端检测的1.87 ℃提升至1.06 ℃。2018年,有研究基于双端检测的原理,通过两个通道实现双端检测。RDTS系统在1.8 km传感距离处将温度测量误差从1 ℃减小至0.5 ℃以下[35]。2019年,太原理工大学的Li等[36]使用13 km长的MMF传感光纤和最高峰值功率30 W的光源,基于双端检测结构和差分色散补偿方法,在11.83 km位置处经过2.5 s平均时间测量得到的温度精度为1.2 ℃。
2010年,韩国Gwangju Institute of Science and Technology的Hwang等[37]报道了通过在传感光纤端面引入反射镜,仅利用Anti-Stokes及其反射光实现温度分布测量的研究成果。由于无须测量Stokes光分量,因此该方法本质上不存在差分衰减,且由于探测通道的减少,具有较高的成本效益,结构也更简单。其原理如
式中:P0为入射光功率;α0和αAS分别为泵浦光和Anti-Stokes光的衰减系数;r0和rAS分别为泵浦光和Anti-Stokes光波长处反射镜的反射率。由
式中:指数衰减项为沿整个传感光纤的积分,是常数,因此消除了与位置相关的传输损耗的影响。系统使用波长1550 nm、脉宽50 ns、重复频率5 kHz的脉冲光源,单模光纤(SMF)作为传感光纤,在47 s的测量时间内实现了2.95 ℃的温度分辨率,作为对比,单端检测和双端检测的分辨率分别为2.37 ℃和1.45 ℃。这种差距推测是由反射镜引起的信号减弱和额外的噪声引起的[37],有望通过反射镜的优化进一步提高性能。
2)光源
在系统光源方面,SensorTran公司提出了使用两个不同波长的光源代替双端检测结构以消除因两拉曼散射光分量的差分衰减导致的系统测量精度下降[38]。Suh等[38-39]基于这种方法,使用940 nm和975 nm以及980 nm和1064 nm两种组合方式的双激光源搭建了RDTS系统,提高了系统的温度测量精度。该方法中,一个光源的波长与另一光源的拉曼散射光的波长重合,使用光开关实现两光源的交替测量,抵消了两个波长的差分衰减因子,相比双端检测法理论上能获得更高的测量精度且无需额外的测量通道。其缺点在于实际应用中激光波长及其强度的稳定性会影响系统测量结果,额外的光源也意味着更高的成本[40]。
除调整波长外,研究者们还提出了脉冲编码技术,在不产生非线性效应和不影响空间分辨率的前提下增加入射光能量,获得更强的散射光信号,提高系统信噪比[41]。传统的脉冲编码方式主要分为Golay编码[42-43]、Simplex编码[44-47]和Cyclic编码[48-50]等。Golay编码的原理基于互补序列对的相关运算。在编码过程中将互补序列对整合为一组双极性码序列,并使用四组单极性序列替代这组双极性码序列分别对光脉冲进行编码。探测得到四组编码脉冲光入射到传感光纤中获得的拉曼散射光,将探测信号与脉冲编码序列进行相关求和运算,即可得到系统的单脉冲响应信号。Golay编码技术中,N位脉冲编码理论上可将系统SNR提升
表 1. 不同类型脉冲编码技术特性对比[54]
Table 1. Comparison of characteristics of different pulse coding types[54]
|
3.1.2 空间分辨率提升
1)光源
在光源脉宽的调整上,2019年,俄罗斯Bauman Moscow State Technical University的Ososkov等[18]首次将锁模飞秒脉冲激光器应用于RDTS系统中,目的在于通过减小光源脉宽提高系统空间分辨率。光源发射的光脉冲脉宽为180 fs,但由于探测器的带宽限制,系统最终实现的空间分辨率为10 cm。另一方面,由于系统噪声的限制,系统的有效传感距离为3 m。
除了脉冲光源外,太原理工大学的科研工作者通过理论分析和数值仿真探索了放大的自发辐射(ASE)光源[55]和混沌激光源[56]在RDTS系统中的应用。基于混沌激光器的RDTS系统的原理图如
2)探测器
目前,研究学者在探索将单光子光电探测器应用于RDTS系统中以实现系统性能优化的可能性。相比模拟光电探测器,单光子探测器具有更小的等效噪声功率NEP,同时极大程度上消除了传统模拟光电探测器带宽的限制,在实现系统高空间分辨率方面取得了重要进展。2011年,英国Heriot-Watt University的Tanner等[58-59]将超导纳米线单光子探测器(SNSPD)应用于RDTS系统中,配合fs级的脉冲光源实现了1 cm量级的空间分辨率。但由于光源的重复频率较大,系统的传感距离被限制在约2.8 m。2014年,澳大利亚Department of Defence的Vo等[19]基于超导纳米线单光子探测器和2.7 m长的As2S3传感光纤搭建了RDTS系统,使用1550 nm波段、脉宽10 ps的激光源实现了亚cm级的系统空间分辨率。2022年,University of Applied Sciences and Arts Western Switzerland的Gasser等[20]报道了使用超导纳米线单光子探测器的RDTS系统在500 m传感距离下实现10 cm空间分辨率的研究成果。系统所用光源脉宽为50~250 ps。在5 m的短距离传感下,系统的空间分辨率达到3 cm。随着单光子探测器的快速发展,单光子探测技术在RDTS系统中的应用研究势必带来更大的性能提升。
3.1.3 传感距离提升
传感距离的限制主要来源于受激拉曼阈值(SRS)限制了能够耦合进光纤的最大光强。根据受激拉曼的特性可以从光源、光纤等方面提升传感距离。
1)光源
2017年,Russian Academy of Sciences的Kuznetsov等[16]使用1.63 μm波长的种子光源,通过拉曼放大器放大,输出峰值功率为370 mW的光脉冲,脉冲重复频率为100 Hz,脉宽为8 μs。使用带宽10 MHz的光电探测器,实现了基于单模光纤的85 km超长传感距离的成果。1.63 μm的泵浦光源相比1550 nm波段,产生的Anti-Stokes光损耗更小,且由于Stokes光衰减系数增大SRS阈值也相应提高,因此有效延长了系统传感距离。系统测量的温度精度为8 ℃,使用的光源脉宽为8 μs,对应的空间分辨率为800 m。此外使用非偏振光源和频谱宽度较宽的激光有利于提高受激拉曼阈值。
2)传感光纤
在传感光纤方面,除了常见的标准SMF和MMF,科研工作者们也探索了其他多种光纤在RDTS系统中的应用。
韩国Seoul National University的Park等[45]提出使用色散补偿光纤(DCF)和色散位移光纤(DSF)等拉曼系数不同的光纤级联以延长系统传感距离的方法,为不同传感光纤的应用提供了新思路。由于DSF光纤的衰减系数与单模相似,但增益系数较大,因此DSF中的Anti-Stokes信号的强度总是大于SMF。DCF的增益和损耗均大于SMF,在一定传输距离内的AS信号强度优于SMF。为了延长传感距离,可以在SMF后面连接增益系数较大的DSF,而后将增益系数更大的DCF连接至传感光纤尾端使传感距离进一步延长。
![基于光纤级联的RDTS系统装置示意图[45]](/richHtml/gxxb/2024/44/1/0106011/img_09.jpg)
图 9. 基于光纤级联的RDTS系统装置示意图[45]
Fig. 9. Schematic diagram of RDTS system device based on fiber optic cascade[45]
2022年,华中科技大学的Wu等[60]自制了在1360~1460 nm波段内传输损耗较小的低水峰光纤(LWPF),并将其用作DTS系统的传感光纤,以减小1450 nm处Anti-Stokes信号光的传输损耗,延长传感距离。结合神经网络降噪算法提高温度测量精度,DTS系统的传感距离最终达到24 km。
SMF在RDTS系统中,由于模场直径的限制,SRS阈值较小,限制了传感距离的提升。而多模光纤较大的模间色散又会导致长距离传感下系统空间分辨率的降低。因此,2017年,华中科技大学的Wang等[61]提出使用少模光纤(FMF)作为传感光纤,与单模光纤相比减小了光传输过程中的SRS等非线性效应,与多模光纤相比减小了模式色散,在长距离传感下获得了较好的性能。通过比较四模、二模和单模光纤的表现,得到使用二模光纤在20 km传感距离下,实现6 ℃温度分辨率、3 m空间分辨率的最佳结果。温度分辨率相比于单模光纤提升约4 ℃。2018年,上海交通大学的Liu等[62]使用大有效模场和低模间色散的渐变折射率少模光纤在25 km传感距离,于少模模式下实现了1 ℃的温度分辨率和1.13 m的空间分辨率,相比MMF温度分辨率仅降低0.3 ℃,而空间分辨率提升了一倍多。同时于准单模模式下实现了4.7 ℃的温度分辨率,与SMF相比,提高了2.2 ℃,而空间分辨率没有下降,证明了少模光纤相比SMF和MMF的优势。
为了更直观地阐述近年来基于系统结构及部件优化的研究进展,
表 2. 基于系统结构及部件优化的RDTS系统性能比较
Table 2. Performance comparison of RDTS system based on structure and component optimization
|
3.2 温度解调优化
温度解调优化旨在提高RDTS系统的温度精度。
温度解调是由系统检测的信号强度信息获得沿传感光纤的温度分布信息的过程。实际应用中需要通过温度校准明确信号强度与温度的对应关系。然而,由于光源功率或探测器响应的波动、滤波器的隔离度不够导致的信号串扰、传感光纤的局部损耗以及两种拉曼散射光分量由于波长不同导致的传输损耗差等,都会影响解调结果的准确性和分辨率[63]。因此研究学者们针对这些问题提出了一系列方案以实现温度解调精度的优化。
早在2007年,印度Raja Ramanna Centre for Advanced Technology的Chakraborty等[64]针对双路解调结构中作为参考光的Stokes光也会受到温度的影响从而导致测量误差的问题,提出使用室温下Anti-Stokes与Stokes光的光强比,而非Stokes光的强度作为参考,用于计算温度分布。使得系统在不易获得室温下信号强度数据的情况下仍能工作,提高了系统的适用性。2015年,吉林大学的Yu等[65]提出一种动态采样校准方案,通过添加一个辅助温度传感模块监测参考光纤的温度,结合消除与测量温度无关的信号直流分量的温度校准计算方法,削弱了环境波动对系统性能的影响。由于直流信号的干扰,系统测量的电信号强度可以表示为
式中:
若系统参考温度为
因此,校准后的传感光纤拉曼散射信号光子数比值为
式中:
使用上述方法,系统在17.34 km传感距离下,3 s系统响应时间内,将温度测量误差降至1 ℃以内,空间和温度分辨率分别为1 m和0.1 ℃[65]。
近些年,太原理工大学在RDTS系统的温度解调方面做出了一系列创新工作[66-68]。2019年,Yan等[68]提出光学动态差分补偿方法,仅使用Anti-Stokes光进行温度解调,同时引入一段置于已知温度的恒温槽中的差分补偿光纤。由于光功率的波动,在温度校准阶段和测量阶段光功率是不同的,用
式中:
将一段
光功率的波动可由(22)和(23)式的比值得到:
将(24)式代入(21)中可得:
其中,
以差分补偿光纤在校准和测量过程中得到的信号强度为参考,消除了由于光功率波动导致的校准与测量过程中光功率不同对测量结果产生的影响,此研究将系统在18 km传感距离下测量的温度精度由7 ℃提高至1.9 ℃[68]。
同年,太原理工大学的Li等[66]发现拉曼后向散射光的温度敏感性是随传感距离变化的,进而提出在拉曼散射光强度表达式的指数温度相关项中加入距离相关的M因子,以提高温度测量精度的方法。优化后的拉曼散射光强度比的表达式为
结合参考光纤的拉曼散射光强度比
式中:M因子可由实验中测得的大数据点拟合得到。此方法在单端检测结构的单路解调,双路解调以及双端检测结构中的应用均取得了明显效果,如
![RDTS系统三种不同测量方式下M因子校准前后测温精度对比[66]。(a)单端检测结构双路解调结果;(b)单端检测结构单路解调结果;(c)双端检测结果](/richHtml/gxxb/2024/44/1/0106011/img_10.jpg)
图 10. RDTS系统三种不同测量方式下M因子校准前后测温精度对比[66]。(a)单端检测结构双路解调结果;(b)单端检测结构单路解调结果;(c)双端检测结果
Fig. 10. Comparison of temperature measurement accuracy before and after M factor calibration under three different measurement methods[66]. (a) Result of dual-demodulation based on single-ended detection structure; (b) result of self-demodulation based on single-ended detection structure; (c) result of double-ended detection
2020年,Yan等[67]针对系统中WDM隔离度不够而导致Anti-Stokes光中残留部分瑞利散射光的问题,提出了相应的校准和测量相结合的方法以消除瑞利散射光的影响。其中校准过程分为两步,第一步将
式中:
而后进行温度测量过程,将参考光纤温度设置为
分别求解式(
其中,
从(35)式可看到,此方法不仅消除了拉曼后向散射光中瑞利噪声的影响,而且消除了光源功率和探测器响应波动的影响。RDTS系统使用MMF作为参考光纤,光源波长和峰值功率分别为1550 nm和30 W,信号经过15000次累加平均。温度校准后,相比传统解调方法温度分辨率提高了1.5 ℃,在9.1 km传感距离下将系统的温度测量精度从6.2 ℃提升至1.7 ℃。
3.3 信号处理优化
系统采集的原始电信号数据中包含信号产生和探测等各个阶段的噪声,需要经过去噪处理提高SNR以获取有用信息,提高系统测量分辨率。最基本的去噪处理算法通过提高平均时间降低随机噪声的影响,随着RDTS系统的发展,各种去噪算法被相继提出。在信号处理优化方面,现有的研究主要致力于系统空间分辨率、温度精度与分辨率的提升。
3.3.1 温度精度与分辨率提升
2014年,印度Raja Ramanna Centre for Advanced Technolog的Saxena等[69]报道了使用短时傅里叶变换(STFT)技术减小温度测量误差的研究。系统使用1064 nm、脉宽为10 ns、脉冲能量10 μJ的光源,在230 m测量距离处的测量的温度误差小于5 ℃。此外,还有一些研究报道了小波变换(WD)在RDTS系统中信号去噪方面的应用。华北电力大学的Hou等[70]将累加平均算法与小波变换相结合,证明了其相比于传统的累加平均算法具有更好的去噪效果,在提升系统的信噪比的同时将测量时间从33 s缩短至6 s。2015年,Saxena等[71]又报道了使用小波变换法在205 m测量距离处,25~295 ℃温度测量范围内实现小于3.5 ℃温度测量误差的研究成果。系统中使用的光源为1064 nm,脉冲能量为100 μJ。山东大学的Wang等[72]在RDTS系统中使用小波变换模极大值法(WTMM)去噪,将系统在120 m测量距离处的温度测量误差减小了1 ℃。太原理工大学的Li等[73]使用WTMM算法改进RDTS系统,其光源波长1550 nm,传感光纤为MMF。最终使系统10.4 km测量距离处的温度精度提高至1.58 ℃,并且传感距离从3 km延长至11.5 km,增大了3.8倍。2016年,印度的Saxena等[74]使用经验模态分解法(EMD),通过解析本征模态函数的面积参数将RDTS系统中65 m传感距离处由弯曲损耗引起的测量误差由24 ℃减小至3.5 ℃。2019年,成都理工大学的Wang等[75]将二分奇异值分解法(D-SVD)用于RDTS系统去噪,将585 m传感距离处,10k次累加平均处理下系统的测量误差减小约2.5 ℃。2022年,河海大学的Xu等[76]将经验模态分解、模糊熵与小波阈值去噪算法结合起来用于拉曼信号降噪。首先将输入信号通过经验模态分解解构得到本征模态函数。而后计算每个本征模态函数的模糊熵,并由此设定阈值区分有效信号模态函数与带噪模态函数。其中带噪模态函数通过小波阈值去噪处理后与有效信号模态函数结合起来重构拉曼信号。此方法在保留信号变化特征的同时将系统的SNR提升了4.83 dB。
除了上述方法外,近些年来,一系列新型算法如机器学习和图像处理算法等也被用于RDTS系统的性能提升。2016年,EPFL Swiss Federal Institute of Technology 的Soto等[77]将图像和视频处理中使用的非局部平均算法(NLM)引入到RDTS系统的信号处理中。将系统测量数据以测量距离和时间为二维(2D)数据“图像”的横纵坐标轴,在此基础上划分相似性窗口,通过窗口在二维数据平面上的滑动计算加权均值,利用系统测量信号的高冗余度去噪,提高SNR。实验结果表明,NLM算法使RDTS系统在9 km传感距离处的SNR获得了13.6 dB的大幅提升,如
![NLM算法去噪后(红色)与原始数据(蓝色)的温度测量结果对比[77]。(a)SNR;(b)温度分辨率](/richHtml/gxxb/2024/44/1/0106011/img_11.jpg)
图 11. NLM算法去噪后(红色)与原始数据(蓝色)的温度测量结果对比[77]。(a)SNR;(b)温度分辨率
Fig. 11. Temperature measurement results compared to the original data (blue) after denoising by the NLM algorithm (red) [77]. (a) SNR; (b) temperature resolution
2021年,华中科技大学的Zhang等[79]使用一维(1D)去噪卷积神经网络(1DDCNN)的深度学习算法,通过大量随机的强度波动模拟RDTS系统的信号特征,每个强度代表一个温度值。将这些随机信号作为训练数据输入到如
3.3.2 空间分辨率提升
传统印象中,RDTS的空间分辨率受制于激光器脉冲宽度和系统带宽。然而近年来通过结合智能算法,逐渐突破了硬件系统上的极限限制,将RDTS的研究推向人工智能时代,形成了新的研究热潮。
2005年,上海理工大学的Liu等[82]提出可将拉曼分布式传感系统近似为线性系统,系统探测的拉曼散射光强度可由输入系统的拉曼散射光信号与系统的脉冲响应函数的卷积得到。此后,基于此原理,使用反卷积(deconvolution)算法获得温度分布信息的方法逐渐在RDTS系统中得到了应用。2009年,清华大学的Zhang等[83]将此卷积概念应用于RDTS系统中,将Anti-Stokes拉曼散射光的强度表述为入射泵浦光脉冲与光纤温度分布函数的卷积,并用频域反卷积法求得光纤的温度分布信息。作者在仿真中使用60 ns的脉冲激光源,获得了1.5 m的空间分辨率,表明频域反卷积法可将系统的空间分辨率提升4倍。2011年,伊朗Department of Physics of Sharif University of Technology的Bahrampour等[84]将基于傅里叶小波正则变换的反卷积法(ForWaRD)应用于拉曼OTDR系统中,在仿真计算中于5 km传感距离处有效复原了两个间隔距离为7 m的温区的温度分布曲线,在保证空间分辨率的同时实现了信号去噪的效果,将系统信噪比提高了24 dB。相关研究表明,ForWaRD算法相比于傅里叶反卷积法在提升系统信噪比方面更加有效[85]。2016年,巴西Federal University of Technology-Paraná的Bazzo等[21]使用全变分反卷积(TVD)算法在实验中准确重构了RDTS系统中13 m传感距离下长度为15 cm的阶梯形加热区域(
![使用全变分反卷积法重构的15 cm长加热区域[21]](/richHtml/gxxb/2024/44/1/0106011/img_13.jpg)
图 13. 使用全变分反卷积法重构的15 cm长加热区域[21]
Fig. 13. 15 cm long heated area reconstructed using total variational deconvolution[21]
2018年,巴西Federal University of Espı´rito Santo的Silva等[22]使用带有外部输入神经网络的非线性自回归算法(NARX)处理RDTS系统的响应信号,完成信号重构,最终完全校正了传感器的测量温度,并将系统的空间分辨率提升至5 cm。2021年,Silva等[87]基于图像处理算法,将DTS系统测量的1D数据根据测量时间重构为2D数据。基于热点区域与背景像素强度不同的特点,以图像强度的突变点为边界划分热区,同时结合测量温度误差与热源大小的关系分析修正误差,用阈值分割的方法将实际热区区分出来。基于这种阈值分割的图像处理算法,系统改善了小于空间分辨率的热源的温度探测精度恶化的问题,最终实现了3 cm大小的热源探测,精度大于98%。虽然此算法对更复杂的温度分布数据的探测性能仍待研究,但该结果为RDTS系统用于比空间分辨率更小的区域的温度探测提供了一个新思路。2021年,太原理工大学的Li等[88]提出RDTS系统采集的温区处的拉曼信号为该处脉冲宽度对应的空间段内拉曼信号强度的叠加,在脉冲进入和出温区过程中的信号强度为部分非温区强度与部分温度强度的叠加。因此由温区对应的信号区域边沿的斜率可解调得到精确的待测温度。并基于此理论建立了相应的数学模型,通过温区边沿斜率解调实现了厘米级空间范围内温度的精确测量。2022年,华中科技大学的Wu等[89]将反卷积算法与卷积神经网络结合,通过实验数据获得反卷积算法中的卷积核,并基于获得的卷积核通过仿真产生训练数据,将其用于后续改进的卷积神经网络的训练。整体模型被命名为超空间分辨率深度卷积神经网络(SSRNet),最终将DTS系统的空间分辨率提升了5倍。
近年来基于信号处理优化研究的RDTS系统的性能参数汇总于
表 3. 基于信号处理优化的RDTS系统性能比较
Table 3. Performance comparison of RDTS system based on signal processing optimization
|
4 市场调研
RDTS技术起源于20世纪80年代,随着其发展而被广泛应用于消防、电力、城市轨道以及石油化工等行业,如今在各类光纤传感器中,RDTS是应用成熟度最高也是市场份额最高的。根据北京凌云光子技术集团提供的《光纤传感行业应用技术发展及解决方案》,2006年至2019年光纤传感市场中RDTS的市场份额自2011年起位居首位,为30%~40%,其次为FBG,2015年以来为25%~30%[90]。经过三十多年的市场化发展,RDTS的行业格局已相对成熟稳定,并呈现出自己的特色。RDTS的市场也已初具规模,且未来的上升空间较大。
4.1 市场行情
关于分布式温度传感器的全球市场,不同的调研机构给出的数据并不完全一致。根据爱尔兰权威调研机构Research and Markets发布的数据,如
![DTS的全球市场与中国市场体量现状与预测[91]](/richHtml/gxxb/2024/44/1/0106011/img_14.jpg)
图 14. DTS的全球市场与中国市场体量现状与预测[91]
Fig. 14. Current situation and forecast of global and Chinese DTS market scale[91]
4.2 供应链及销售模式
RDTS的供应链大致可以细分为元器件供应商、模块供应商、主机供应商以及弱电集成商。主机供应商通过采购元器件以及模块进行主机制造,其中的模块通常是将激光器与部分信号采集、处理功能的器件进行了整合封装,简化了后续搭建主机的复杂性。为了利润的最大化,目前模块供应商与主机供应商逐步进行激光器模块封装等高价、关键的元器件的开发,呈现出供应链垂直整合的趋势。
RDTS的销售模式通常是产品同服务捆绑的形式,定制化程度较高。由于RDTS乃至所有光纤传感器都需要集成在各种不同的应用场景中使用,这决定了RDTS行业需要与特定应用行业进行密切对接并对产品做深度优化。终端用户往往以项目招标的形式发布需求,RDTS的供应商在投标书中将产品打包为成套解决方案的形式进行报价,报价中除包含实体产品本身外,还包括传感光缆敷设施工的费用、弱电集成的成本以及后期运维服务的人力成本等,打包后的报价往往达到单机产品价格的三倍甚至更多,从这个统计口径得到的RDTS的国内市场可以达到人民币数十亿元。
国内外主流厂家的典型产品信息汇总于
表 4. 国内外部分主流厂家的典型产品信息
Table 4. Typical product information of some mainstream manufacturers at home and abroad
|
4.3 标准化情况
RDTS的标准化工作在国内外还处于相对起步阶段。目前与之相关性较强的国际标准为国际电工委员会制定的《IEC 61757—2018 Fibre Optic Sensors - Generic Specification》,与其对应的国家标准为《GB/T 18901.1—2019光纤传感器 第1部分:总规范》,二者均属于通用规范,即从最宏观的层面定性规定了点式光纤传感器与分布式光纤传感器仪器需测试的指标名称及测试方法,但未定量给出指标需要达到的数值范围。
国内的行业标准针对电力行业中分布式温度传感器发布了《DL/T1573—2016电力电缆分布式光纤测温系统技术规范》及《DL/T1894—2018电力光纤传感器通用规范》等标准,定量给出了DTS仪器应满足的基本要求。
针对分布式光纤传感器所用的光纤与光缆,国内外尚无传感光纤标准或者传感光缆标准,目前执行的标准为常规光纤与光缆的标准。
因此对于RDTS而言,在消防、城市轨道以及石化等领域处于行业标准或团体标准空白的状态。
4.4 行业发展趋势
RDTS的发展一方面是替代传统温度传感器,另一方面是主动适应新兴行业的需求。对于前者,RDTS在现有的消防、电力、轨交以及石化等领域会进一步提升应用量,虽然将同时面对光纤光栅以及布里渊光时域反射技术(BOTDR)和布里渊光时域分析技术(BOTDA)的市场竞争,市场需求量仍呈稳步上升的趋势;对于后者,新能源等新兴产业的蓬勃发展也成为RDTS可观的潜在增长点,例如新能源电池的生产、贮存及运输等环节均涉及到大量的温度监测刚需,RDTS作为无源检测的手段在安全性方面具备先天优势。从地域分布来看,RDTS主要伴随着大型基础设施的新建以及电力、能源等产业的规模扩大,这使得我国中西部地区以及沿海发达城市等地区对于RDTS的需求量明显高于其他地区,预计未来相当一段时间这一趋势将会得到延续。随着RDTS的行业规模日益扩大以及市场的进一步细分,相关行业标准必定会陆续制定,RDTS的产品质量将更加可控可比,国内外市场将更加规范有序。
5 RDTS系统的应用
5.1 建筑结构安全监测
建筑结构与人类活动息息相关,其损坏会直接造成人员伤亡或巨大的经济损失。因此建筑结构的安全监测对于提高其管理的成本效益及人类生产活动的进行都具有重要意义。下面重点介绍RDTS系统在堤坝安全监测、管道泄漏两方面的应用。
堤坝损坏的诱因主要为水位的突然变化,这会导致堤坝基体内部渗流状态的变化,形成侵蚀结构。使用RDTS实现安全监测的原理在于:当堤坝上有水位变化导致的过滤流剧烈变化时,堤坝内部温度不再只是平流和热传导共同作用的结果,而是会产生显著波动。因此温度测量是识别堤坝上异常水流的有用工具[108]。由于堤坝的几何尺寸较大,因此具有长测量范围的DTS系统是理想测量工具,其中主要使用的是RDTS系统[63,109-122],其在堤坝安全监测应用中的结构示意图如
![RDTS系统在堤坝安全和管道泄漏监测中的应用。(a)堤坝安全监测应用结构示意图[108];(b)渗漏测试现场[114];(c)管道泄漏监测中的应用示意图[125];(d)管道泄漏监测现场[124]](/richHtml/gxxb/2024/44/1/0106011/img_15.jpg)
图 15. RDTS系统在堤坝安全和管道泄漏监测中的应用。(a)堤坝安全监测应用结构示意图[108];(b)渗漏测试现场[114];(c)管道泄漏监测中的应用示意图[125];(d)管道泄漏监测现场[124]
Fig. 15. Application of RDTS system in embankment dams safety and pipeline leakage monitoring. (a) Schematic diagram of the dam safety monitoring application structure[108]; (b) leakage test site[114]; (c) schematic diagram of the pipeline leakage monitoring[125]; (d) pipeline leakage monitoring site[124]
DTS系统还可以用于监测输送气体或液体的管道泄漏问题,输送高压气体的泄漏导致的压力降低会造成局部温度下降,而管道输送的液体温度一般与管道外环境温度不同,因此通过监测温度的变化可以检测到管道泄漏。2017年,新加坡Nanyang Technological University的Wang等[124]报道了RDTS系统在监测天然气管道中水泄漏问题的应用研究,其实验测试装置见
除上述几种应用之外,RDTS系统在其他建筑结构[127]、土木及水文系统的探测中也有重要应用[128-133]。2023年,天津大学的Liu等[134]在基于RDTS的建筑火灾探测场景中,使用非线性拟合和机器学习算法获得系统的温度测量误差模型。基于该模型实现了系统测量温度的动态校准,在600 ℃以下测量误差最小达3.5%,600 ℃以上测量误差最小为1.5%。
5.2 电力系统安全监测
电力系统中温度监测的对象主要为电线、电缆和供电设备。电力系统中电线和电缆分布范围广,供电设备与其他电气设备相互连接,使用过程中电阻增大、短路、过负荷、绝缘损坏等情况引起的局部温度过高可能引起大范围的重大火灾事故。通过温度监测保证电力系统安全运行的原理在于:温度的大幅度变化可能引起电路损坏,除此之外,电网故障前期一般伴随着温度的剧烈变化,因此温度的变化能反映电力系统的状态。RDTS系统用于电缆安全的监测通常需要将传感光纤集成在电缆中,如
![RDTS系统在电力系统安全监测中的应用。(a)光纤在电缆中的集成[135];(b)架空电缆热导模型示意图[138];(c)在光伏板温度监测中的应用[139];(d)在电机温度监测中的应用[140]](/richHtml/gxxb/2024/44/1/0106011/img_16.jpg)
图 16. RDTS系统在电力系统安全监测中的应用。(a)光纤在电缆中的集成[135];(b)架空电缆热导模型示意图[138];(c)在光伏板温度监测中的应用[139];(d)在电机温度监测中的应用[140]
Fig. 16. Application of RDTS system in power system safety monitoring. (a) Integration of optical fiber in the cable[135]; (b) thermal conductivity model of the overhead cable [138]; (c) application in photovoltaic panel temperature monitoring[139]; (d) application in motor temperature monitoring[140]
2006年,土耳其Uludag University 的Yilmaz等[135]将RDTS系统应用于154 kV的交联聚乙烯绝缘电缆温度监测中,在412 m电缆温度监测实验中实现了1 ℃的温度分辨率和1.22 m的空间分辨率,此外,针对10 km长电缆的仿真结果表明,系统测得的温度与模型温度吻合良好。之后,2009年,郑晓亮等[136]将以瑞利散射光为参考光的RDTS系统应用于井下的电缆温度监测,系统使用包裹有机外护套的单模光纤光缆,将其固定在电缆外表面。系统传感距离可达10 km,空间和温度分辨率分别为1 m和0.1 ℃,具有数据采集显示、超温报警、数据查询等功能。西安工业大学的周芸等[137]使用最佳空间和温度分辨率分别为0.1 m和0.01 ℃的RDTS系统在线监测高压电力电缆的温度分布,将该技术向该领域的应用更进一步。他们自主设计数据采集和存储电路,结合信号平均处理,实现了预设温度的有效检测。2020年,印度National Aerospace Laboratories 的Datta等[138]报道了RDTS系统在由24股光纤组成的300 m长架空电缆中的演示应用,将传感光纤嵌入架空电缆里。系统采用双端检测结构、单路解调方案以及全变分去噪方法,在待测电缆两端分别连接2 km的光纤置于系统内部作为参考光纤,实现了0.33 ℃的温度分辨率和8 m空间分辨率。电缆的热传导模型结构如
2021年,太原理工大学的Yu等[139]使用RDTS系统,结合模糊温差阈值法预测温度变化,用于光伏板的温度监测以保证其高效运行。使用WTMM法去噪,对光伏板连续6 h的温度监测结果表明温度测量误差为1.08 ℃,误差波动范围为3.7 ℃,可以有效预测光伏板的温度变化[
5.3 火灾探测
在火灾探测应用中,对RDTS系统最重要的要求为其还原空间温度分布情况的能力[17]。在实际应用时,传感光纤的外部布线需要适用于其应用场景,在一些恶劣环境中,传感光纤的排布需坚固可靠,耐潮湿、腐蚀和抗啮齿动物啃咬等。此外,火灾发生的判断需要根据沿传感光纤的多区域、多阈值参数综合判定以避免错误警报[142]。
在室内火灾探测方面,2016年,中国科学院的Sun等[143]通过两段平行排布的MMF传感光纤,测量密闭房间中空气流的温度实现火灾定位,通过燃烧实验证明了系统的可靠性。在交通运输系统的火灾探测方面,早在2002年,法国Aerospace R&D Department 的Odic等[144]就已将RDTS系统应用到飞机货舱的火灾探测中。2013年,中国计量大学的桑雷等[145]报道了在地铁的区间隧道中布局复合架空地线光纤实现火灾探测的应用,光纤置于隧道屏蔽网的侧壁,RDTS系统的试运行结果监测到了50 ℃的异常温度热点,证明了系统可靠性。2019年,太原理工大学的Yan等[68]将RDTS系统应用于隧道火灾探测中(
5.4 油气开采
石油和天然气不仅是重要的能源资源,也是许多工业产品,如塑料、化学制品、洗涤剂等的生产基础。其开采对国家经济发展及人民生活至关重要。DTS系统在油气开采行业中已经得到了大量的应用[146-153],仅Schlumberger一家公司就已经利用DTS系统在全球范围内完成了数千口井的钻井作业。RDTS系统在油气开采中的应用主要包含井下液流监测、注水监测以及蒸汽辅助重力泄油等[154]。井下液流监测的原理是液体的流入会带来与井筒的热交换,使井筒的温度发生变化,由此定位液流注入点。注水监测过程的目的是判断用于提高油藏压力的注入水的位置移动,原理同样基于注入水引起的温度变化。蒸汽辅助重力泄油通过蒸汽降低稠油浓度,增加流动性,便于开采,该过程可以通过温度变化监测确定完成情况[155]。
除此之外,2014年,哈尔滨工业大学的Peng等[146]报道了RDTS系统在油井液位探测中的应用,系统传感光纤中含有辅助加热电缆,通过液体和气体的不同热传导参数导致的不同温度分布检测出液面所处位置,
![RDTS系统在石油井中的应用示意图[146]](/richHtml/gxxb/2024/44/1/0106011/img_18.jpg)
图 18. RDTS系统在石油井中的应用示意图[146]
Fig. 18. Application schematic diagram of of RDTS system in oil well [146]
5.5 高低温传感
在工业生产及科研等领域有众多的高低温传感应用场景,如工业品炼制、煤气炉、航空发动机等的高温检测以及用于空间探索研究的散热器,超导应用等的低温检测。近几年来,RDTS系统应用于高低温传感的研究逐渐增多。
RDTS系统实现高温传感的重点为耐高温光纤的应用。1998年,希腊National Technical University 的Zouboulis等[158]指出由于黑体辐射在短波长域的快速衰减,使用短波长光源可以削弱高温检测下黑体辐射的影响,文中在266 nm光源激发下成功探测到了1420 ℃高温下蓝宝石的拉曼光谱。此后,美国Virginia Tech的Liu等[159]考虑了高温传感下黑体辐射和荧光的影响,使用基于532 nm光源的脉冲激光器,并以1 m长的单晶光纤作为传感元件搭建了首个适用于超高温监测的RDTS系统,于2016年实现了1200 ℃的高温检测,温度分辨率和空间分辨率分别达到3.7 ℃和14 cm。2018年,使用2 m长的单晶光纤实现了1400 ℃的高温检测,空间分辨率为12.4 cm[160]。而后,他们又将新型的图像处理算法用于高温分布式传感系统中,结合数据平均,实现了从室温到1400 ℃范围内1 s响应时间下0.62 ℃的温度分辨率或1 ms平均时间下8 ℃的温度分辨率[161]。此后该小组又将全分布式高温传感的温度上限提高至1600 ℃,在1 s的响应时间下实现了1.08 ℃的温度分辨率,空间分辨率达到6 cm。
除单晶光纤外,镀金光纤也是高温应用场景下常见的传感光纤。镀金光纤的长期使用温度一般在700 ℃以下。2015年,有工作报道了使用15 m长的镀金光纤实现600 ℃高温检测的研究成果,测量误差小于1.5 ℃[162]。此后,2017年,西班牙Photonics Engineering Group of Universidad de Cantabria 的Laarossi等[163]使用79 m的镀金光纤以及1064 nm的光源,对比了标准MMF与镀金光纤在高温传感下的表现,镀金光纤在不同温度下的测量结果如
![RDTS系统在高低温传感中的应用。(a)使用镀金光纤在不同温度下的测量结果[163];(b)测量的低温下散射器的温度分布[164]](/richHtml/gxxb/2024/44/1/0106011/img_19.jpg)
图 19. RDTS系统在高低温传感中的应用。(a)使用镀金光纤在不同温度下的测量结果[163];(b)测量的低温下散射器的温度分布[164]
Fig. 19. Application of RDTS system in high and low temperature sensing. (a) Measured temperature distribution using gold-coated fibers at different temperatures[163]; (b) measured temperature distribution of the scatterer at low temperature[164]
低温检测方面,中国科学院的Xin等[42]于2018年将带软聚合物涂层的680 m光纤应用于RDTS系统中,结合Golay编码法,成功实现了变化的磁场下低至77 K的温度检测,测量温度波动小于1.5 ℃。一年后,北京航天器环境工程研究所的Zhang等[164]通过热电偶辅助的温度测量求解校准参数,用于RDTS系统的温度校准。将光纤排布于近真空且温度低至-173 ℃的散热器内进行温度测量实验,获得的结果如
5.6 核工业安全监测
对生产核能的实验反应堆以及反应结束后高放射性废物存放的安全监测是核工业生产活动的基础保障。核反应堆中的高辐射环境导致的光纤降解,传输损耗增大引起的信噪比降低以及差分损耗增大等是实现温度监测的难点。针对上述问题,比利时Université libre de Bruxelles的Fernandez等[167]使用双端检测的RDTS系统结构,结合商用聚酰亚胺和丙烯酸酯涂层光纤实现了高耐辐射的RDTS系统,在总辐射剂量超过300 kGy环境中的检测结果显示辐射前后温度差值小于0.5 ℃。2015年,瑞士European Organization for Nuclear Research的Toccafondo等[168]将RDTS系统应用于欧洲核子研究组织的高能混合加速器设施中进行测温实验,实验现场布局示意图如
![RDTS系统在核工业安全监测中的应用。(a)核辐射场中应用示意图[168];(b)用于放射性废弃物安全检测的结构示意图[170]](/richHtml/gxxb/2024/44/1/0106011/img_20.jpg)
图 20. RDTS系统在核工业安全监测中的应用。(a)核辐射场中应用示意图[168];(b)用于放射性废弃物安全检测的结构示意图[170]
Fig. 20. Application of RDTS system in nuclear industry safety monitoring. (a) Schematic diagram of application in nuclear radiation field[168]; (b) schematic diagram of the structure used for the safety monitoring of radioactive waste [170]
6 结束语
本文概述了RDTS系统的基本原理,重点介绍了RDTS系统的优化、市场调研和应用情况。其中从系统结构及部件优化、温度解调优化、信号处理优化三方面综述了目前RDTS系统的研究成果。在系统结构及部件优化方面,脉冲编码技术可以在不影响系统空间分辨率的前提下将信噪比提高10 dB以上,通过遗传优化算法寻找最佳脉冲编码序列可将脉冲编码的优势最大化,将系统温度分辨率提高7倍以上。在RDTS系统中引入混沌激光源,结合相关的解调算法可以将空间分辨率从50 m提升至0.3 m。少模光纤的使用是同时克服单模光纤的低SRS阈值和多模光纤的大模间色散的可行性方案,与SMF(MMF)相比,可在几乎不影响空间(温度)分辨率的前提下,提升系统温度(空间)分辨率。在温度解调方面,介绍了多种温度校准计算方法,用于削弱系统功率波动、信号串扰和损耗等对测量结果的影响,提高系统温度测量精度,合适的校准方法可将系统温度精度提高14 ℃以上。在信号处理方面,近些年,基于图像处理的算法、人工智能算法等被相继应用于RDTS系统,一些算法不仅提高了系统SNR而且可用于空间分辨率的提升,在这些算法的应用下,RDTS系统的空间分辨率可提升5倍,温度分辨率已达到0.01 ℃量级。除此之外,文中总结了RDTS系统的国内外市场行情及主流商家,汇总了各商家典型产品信息,与国外产品相比,国内产品的技术指标有待进一步提升。最后,详细列举了RDTS系统在建筑结构、电力系统安全监测、火灾探测、油气开采、高低温传感、核工业安全监测等各领域的应用。
随着越来越多的新观点被提出,预期RDTS系统的性能还有较大的提升空间。基于器件集成等实现系统小型化,提高便携性是RDTS系统长远发展的主题之一。各类人工智能算法的应用与优化也将赋予系统更快的处理速度与更优越的性能。除此之外,对传感光纤以及系统其他相关方面的合理设计优化可使系统在超高低温、核辐射等恶劣环境中的应用更加深入,由此带来的许多潜在应用值得进一步探索。
[1] Zhu C, Gerald R E, Chen Y Z, et al. Probing the theoretical ultimate limit of coaxial cable sensing: measuring nanometer-scale displacements[J]. IEEE Transactions on Microwave Theory and Techniques, 2020, 68(2): 816-823.
[2] Zhu C, Zhuang Y Y, Chen Y Z, et al. Contactless liquid interface measurement based on a hollow coaxial cable resonator[J]. Sensors and Actuators A: Physical, 2019, 285: 623-627.
[4] Roman M, Balogun D, Zhuang Y Y, et al. A spatially distributed fiber-optic temperature sensor for applications in the steel industry[J]. Sensors, 2020, 20(14): 3900.
[5] Li T L, Tan Y G, Han X E, et al. Diaphragm based fiber Bragg grating acceleration sensor with temperature compensation[J]. Sensors, 2017, 17(12): 218.
[6] Vargas-Rodriguez E, Guzman-Chavez A D, Baeza-Serrato R, et al. Optical fiber FP sensor for simultaneous measurement of refractive index and temperature based on the empirical mode decomposition algorithm[J]. Sensors, 2020, 20(3): 664.
[7] Geng Y F, Li X J, Tan X L, et al. Compact and ultrasensitive temperature sensor with a fully liquid-filled photonic crystal fiber Mach-Zehnder interferometer[J]. IEEE Sensors Journal, 2014, 14(1): 167-170.
[8] Li J, Yan B Q, Zhang M J, et al. Long-range Raman distributed fiber temperature sensor with early warning model for fire detection and prevention[J]. IEEE Sensors Journal, 2019, 19(10): 3711-3717.
[9] Ma L M, Ma C, Wang Y M, et al. High-speed distributed sensing based on ultra weak FBGs and chromatic dispersion[J]. IEEE Photonics Technology Letters, 2016, 28(12): 1344-1347.
[10] 李政颖, 孙文丰, 王洪海. 基于光频域反射技术的超弱反射光纤光栅传感技术研究[J]. 光学学报, 2015, 35(8): 0806003.
[11] StanimirovićI, StanimirovićZ. Introductory chapter: temperature sensing - the book[M]. Rijeka: IntechOpen, 2018.
[12] Sang A K, Froggatt M E, Gifford D K, et al. One centimeter spatial resolution temperature measurements in a nuclear reactor using Rayleigh scatter in optical fiber[J]. IEEE Sensors Journal, 2008, 8(7): 1375-1380.
[13] Bao X, Webb D J, Jackson D A. Combined distributed temperature and strain sensor based on Brillouin loss in an optical fiber[J]. Optics Letters, 1994, 19(2): 141-143.
[14] Ko P K Y, Demokan S, Tam H. Distributed temperature sensing with erbium-doped fiber amplifiers[J]. Journal of Lightwave Technology, 1996, 14(10): 2236-2245.
[15] Dakin J P, Pratt D J, Bibby G W, et al. Temperature distribution measurement using Raman ratio thermometry[J]. Proceedings of SPIE, 1986, 0566: 249-256.
[16] Kuznetsov A G, Kharenko D S, Babin S A, et al. Ultralong fibre-optic distributed Raman temperature sensor[J]. Quantum Electronics, 2017, 47(10): 967-970.
[17] Failleau G, Beaumont O, Razouk R, et al. A metrological comparison of Raman-distributed temperature sensors[J]. Measurement, 2018, 116: 18-24.
[18] Ososkov Y Z, Chernutsky A O, Dvoretskiy D A, et al. Fiber optic Raman distributed temperature sensor based on an ultrashort pulse mode-locked fiber laser[J]. Optics and Spectroscopy, 2019, 127(4): 664-668.
[19] Vo T D, He J K, Magi E, et al. Chalcogenide fiber-based distributed temperature sensor with sub-centimeter spatial resolution and enhanced accuracy[J]. Optics Express, 2014, 22(2): 1560-1568.
[20] Gasser J, Warpelin D, Bussières F, et al. Distributed temperature sensor combining centimeter resolution with hundreds of meters sensing range[J]. Optics Express, 2022, 30(5): 6768-6777.
[21] Bazzo J P, Pipa D R, Martelli C, et al. Improving spatial resolution of Raman DTS using total variation deconvolution[J]. IEEE Sensors Journal, 2016, 16(11): 4425-4430.
[22] Silva L C B D, Samatelo J L A, Segatto M E V, et al. NARX neural network model for strong resolution improvement in a distributed temperature sensor[J]. Applied Optics, 2018, 57(20): 5859-5864.
[23] Höbel M, Ricka J, Wüthrich M, et al. High-resolution distributed temperature sensing with the multiphoton-timing technique[J]. Applied Optics, 1995, 34(16): 2955-2967.
[24] Barnoski M K, Jensen S M. Fiber waveguides: a novel technique for investigating attenuation characteristics[J]. Applied Optics, 1976, 15(9): 2112-2115.
[25] KaramehmedovicE. Incoherent optical frequency domain reflectometry for distributed thermal sensing[D]. Kongens Lyngby: Technical University of Denmark, 2007.
[26] 张旭苹. 全分布式光纤传感技术[M]. 北京: 科学出版社, 2013.
ZhangX P. Fully distributed optical fiber sensing technology[M]. Beijing: Science Press, 2013.
[27] 方星. 分布式光纤拉曼温度传感系统关键技术的研究[D]. 成都: 电子科技大学, 2020.
FangX. Research on key technologies of distributed optical fiber Raman temperature sensing system[D]. Chengdu: University of Electronic Science and Technology of China, 2020.
[28] 周正仙. 分布式光纤温度传感器的设计和优化[D]. 上海: 上海交通大学, 2009.
ZhouZ X. Design and optimization of distributed optical fiber temperature sensor[D]. Shanghai: Shanghai Jiao Tong University, 2009.
[29] 方曼. 分布式拉曼光纤温度传感器系统及温度分辨率提高的研究[D]. 成都: 电子科技大学, 2004.
FangM. Research on distributed Raman fiber optic temperature sensor system and improving temperature resolution[D]. Chengdu: University of Electronic Science and Technology of China, 2004.
[30] 王伟杰. 基于拉曼散射的分布式光纤测温系统设计及优化[D]. 济南: 山东大学, 2013.
WangW J. Design and optimization of distributed optical fiber temperature measurement system based on Raman scattering[D]. Jinan: Shandong University, 2013.
[31] 刘恒, 喻俊松, 万生鹏, 等. 基于FPGA的分布式拉曼光纤传感系统[J]. 激光与光电子学进展, 2021, 58(5): 0506006.
[32] 刘德明, 孙琪真. 分布式光纤传感技术及其应用[J]. 激光与光电子学进展, 2009, 46(11): 29-33.
[33] Wang Z L, Zhang S S, Chang J, et al. Attenuation auto-correction method in Raman distributed temperature measurement system[J]. Optical and Quantum Electronics, 2013, 45(10): 1087-1094.
[34] Soto M A, Signorini A, Nannipieri T, et al. High-performance Raman-based distributed fiber-optic sensing under a loop scheme using anti-Stokes light only[J]. IEEE Photonics Technology Letters, 2011, 23(9): 534-536.
[35] Liu X J, Lu H, Yuan B X, et al. A double-ended Raman temperature measurement method for hazardous chemicals warehouse[J]. Optik, 2018, 169: 62-68.
[37] Hwang D, Yoon D J, Kwon I B, et al. Novel auto-correction method in a fiber-optic distributed-temperature sensor using reflected anti-Stokes Raman scattering[J]. Optics Express, 2010, 18(10): 9747-9754.
[38] Suh K, Lee C, Sanders M, et al. Active plug and play distributed Raman temperature sensing[J]. Proceedings of SPIE, 2008, 7004: 700435.
[39] Suh K, Lee C. Auto-correction method for differential attenuation in a fiber-optic distributed-temperature sensor[J]. Optics Letters, 2008, 33(16): 1845-1847.
[40] Bao X Y, Chen L. Recent progress in distributed fiber optic sensors[J]. Sensors, 2012, 12(7): 8601-8639.
[41] Everard J K A. Novel signal processing techniques for enhanced OTDR sensors[J]. Proceedings of SPIE, 1987, 0798: 42-47.
[42] Xin C J, Guan M Z. The sensitivity of distributed temperature sensor system based on Raman scattering under cooling down, loading and magnetic field[J]. Cryogenics, 2019, 100: 36-40.
[43] Soto M A, Sahu P K, Faralli S, et al. Distributed temperature sensor system based on Raman scattering using correlation-codes[J]. Electronics Letters, 2007, 43(16): 862-864.
[44] Lee D, Yoon H, Kim P, et al. Optimization of SNR improvement in the noncoherent OTDR based on simplex codes[J]. Journal of Lightwave Technology, 2006, 24(1): 322-328.
[45] Park J, Bolognini G, Lee D, et al. Raman-based distributed temperature sensor with simplex coding and link optimization[J]. IEEE Photonics Technology Letters, 2006, 18(17): 1879-1881.
[46] Wang Y P, Sun X H, Xue Q, et al. The study of the Raman-based optical fiber-folded distributed temperature sensing system with simplex code[J]. Optics Communications, 2018, 420: 200-204.
[47] DaiG Y, FanX Y, HeZ Y. A long-range fiber-optic Raman distributed temperature sensor based on dual-source scheme and RZ simplex coding[C]//2018 Asia Communications and Photonics Conference (ACP), October 26-29, 2018, Hangzhou, China. New York: IEEE Press, 2018.
[48] SotoM A, NannipieriT, SignoriniA, et al. Advanced cyclic coding technique for long-range Raman DTS systems with meter-scale spatial resolution over standard SMF[C]//SENSORS, 2011 IEEE, October 28-31, 2011, Limerick, Ireland. New York: IEEE Press, 2012: 878-881.
[49] Taki M, Signorini A, Oton C J, et al. Hybrid Raman/Brillouin-optical-time-domain-analysis-distributed optical fiber sensors based on cyclic pulse coding[J]. Optics Letters, 2013, 38(20): 4162-4165.
[50] Soto M A, Nannipieri T, Signorini A, et al. Raman-based distributed temperature sensor with 1 m spatial resolution over 26 km SMF using low-repetition-rate cyclic pulse coding[J]. Optics Letters, 2011, 36(13): 2557-2559.
[51] 孙乔. 基于时域脉冲编码的长距离高空间分辨率光纤布里渊分布式传感技术研究[D]. 长沙: 国防科学技术大学, 2016.
SunQ. Research on long-distance and high spatial resolution fiber Brillouin distributed sensing technology based on time domain pulse coding[D]. Changsha: National University of Defense Technology, 2016.
[52] Datta A, Srimal V, Srinivasan B. Performance enhancement of Raman optical time domain reflectometer using Golay codes[J]. Proceedings of SPIE, 2011, 8173: 81731R.
[53] LeeD, YoonH, KimN Y, et al. Analysis and experimental demonstration of simplex coding technique for SNR enhancement of OTDR[C]//Proceedings of the Lightwave Technologies in Instrumentation and Measurement Conference, October 20-20, 2004, Palisades, NY, USA. New York: IEEE Press, 2004: 118-122.
[54] Sun X Z, Yang Z S, Hong X B, et al. Genetic-optimised aperiodic code for distributed optical fibre sensors[J]. Nature Communications, 2020, 11: 5774.
[55] Li J, Zhou X X, Yin Z T, et al. Reconstruction compression correlation demodulation for Raman optical time domain reflection[J]. Advanced Photonics Research, 2021, 2(10): 2100047.
[56] Zhou X X, Li J, Xu Y, et al. Chaos Raman optical time-domain reflectometry for millimeter-level spatial resolution temperature sensing[J]. Journal of Lightwave Technology, 2021, 39(23): 7529-7538.
[57] Li J, Wang C Y, Cao K Y, et al. Breakthrough the physical barrier on spatial resolution in Raman distributed fiber sensing using chaotic correlation demodulation[J]. APL Photonics, 2023, 8(7): 076105.
[58] Tanner M G, Dyer S D, Baek B, et al. High-resolution single-mode fiber-optic distributed Raman sensor for absolute temperature measurement using superconducting nanowire single-photon detectors[J]. Applied Physics Letters, 2011, 99(20): 201110.
[59] Dyer S D, Tanner M G, Baek B, et al. Analysis of a distributed fiber-optic temperature sensor using single-photon detectors[J]. Optics Express, 2012, 20(4): 3456-3466.
[60] Wu H, Du H Z, Zhao C, et al. 24 km high-performance Raman distributed temperature sensing using low water peak fiber and optimized denoising neural network[J]. Sensors, 2022, 22(6): 2139.
[61] Wang M, Wu H, Tang M, et al. Few-mode fiber based Raman distributed temperature sensing[J]. Optics Express, 2017, 25(5): 4907-4916.
[62] Liu Y P, Ma L, Yang C, et al. Long-range Raman distributed temperature sensor with high spatial and temperature resolution using graded-index few-mode fiber[J]. Optics Express, 2018, 26(16): 20562-20571.
[63] Ghafoori Y, Vidmar A, Říha J, et al. A review of measurement calibration and interpretation for seepage monitoring by optical fiber distributed temperature sensors[J]. Sensors, 2020, 20(19): 5696.
[64] Chakraborty A L, Sharma R K, Saxena M K, et al. Compensation for temperature dependence of Stokes signal and dynamic self-calibration of a Raman distributed temperature sensor[J]. Optics Communications, 2007, 274(2): 396-402.
[65] Yu M, Liu Y, Cui H L, et al. Ambient condition desensitization of a fiber Raman temperature sensing system based on a dynamic sampling-correction scheme[J]. Applied Optics, 2015, 54(15): 4823-4827.
[66] Li J, Zhang Q, Xu Y, et al. High-accuracy distributed temperature measurement using difference sensitive-temperature compensation for Raman-based optical fiber sensing[J]. Optics Express, 2019, 27(25): 36183-36196.
[67] Yan B Q, Li J, Zhang M J, et al. Temperature accuracy and resolution improvement for a Raman distributed fiber-optics sensor by using the Rayleigh noise suppression method[J]. Applied Optics, 2019, 59(1): 22-27.
[68] Yan B Q, Li J, Zhang M J, et al. Raman distributed temperature sensor with optical dynamic difference compensation and visual localization technology for tunnel fire detection[J]. Sensors, 2019, 19(10): 2320.
[69] Saxena M K, Raju S D V S J, Arya R, et al. Optical fiber distributed temperature sensor using short term Fourier transform based simplified signal processing of Raman signals[J]. Measurement, 2014, 47: 345-355.
[70] HouS Z, JianY H, ChenY, et al. Signal processing of single-mode fiber sensor system based on Raman scattering[C]//2010 2nd International Conference on Advanced Computer Control, March 27-29, 2010, Shenyang. New York: IEEE Press, 2010: 176-181.
[71] Saxena M K, Raju S D V S J, Arya R, et al. Raman optical fiber distributed temperature sensor using wavelet transform based simplified signal processing of Raman backscattered signals[J]. Optics & Laser Technology, 2015, 65: 14-24.
[74] Saxena M K, Raju S D V S J, Arya R, et al. Empirical mode decomposition-based detection of bend-induced error and its correction in a Raman optical fiber distributed temperature sensor[J]. IEEE Sensors Journal, 2016, 16(5): 1243-1252.
[75] Wang H H, Wang X, Cheng Y, et al. Research on noise reduction method of RDTS using D-SVD[J]. Optical Fiber Technology, 2019, 48: 151-158.
[76] Xu L, Su H Z, Cai D S, et al. RDTS noise reduction method based on ICEEMDAN-FE-WSTD[J]. IEEE Sensors Journal, 2022, 22(18): 17854-17863.
[77] Soto M A, Ramírez J A, Thévenaz L. Intensifying the response of distributed optical fibre sensors using 2D and 3D image restoration[J]. Nature Communications, 2016, 7: 10870.
[78] Malakzadeh A, Didar M, Mansoursamaei M. SNR enhancement of a Raman distributed temperature sensor using partial window-based non local means method[J]. Optical and Quantum Electronics, 2021, 53(3): 147.
[79] Zhang Z S, Wu H, Zhao C, et al. High-performance Raman distributed temperature sensing powered by deep learning[J]. Journal of Lightwave Technology, 2021, 39(2): 654-659.
[80] Datta A, Raj V, Sankar V, et al. Measurement accuracy enhancement with multi-event detection using the deep learning approach in Raman distributed temperature sensors[J]. Optics Express, 2021, 29(17): 26745-26764.
[81] Wang H H, Wang Y H, Wang X, et al. A novel deep-learning model for RDTS signal denoising based on down-sampling and convolutional neural network[J]. Journal of Lightwave Technology, 2022, 40(12): 3647-3653.
[82] Liu H L, Zhuang S L, Zhang Z X, et al. The optimization of the spatial resolution of a 30-km distributed optical fiber temperature sensor[J]. Proceedings of SPIE, 2005, 5634: 225-231.
[84] Bahrampour A R, Moosavi A, Bahrampour M J, et al. Spatial resolution enhancement in fiber Raman distributed temperature sensor by employing ForWaRD deconvolution algorithm[J]. Optical Fiber Technology, 2011, 17(2): 128-134.
[85] Pradhan H S, Sahu P K. Characterisation of Raman distributed temperature sensor using deconvolution algorithms[J]. IET Optoelectronics, 2015, 9(2): 101-107.
[86] Zhu W H, Wu H T, Chen W X, et al. Submetric spatial resolution ROTDR temperature sensor assisted by Wiener deconvolution[J]. Sensors, 2022, 22(24): 9942.
[87] Silva L C B, Ciarelli P M, Silva J C C, et al. Detection of multiple small temperature events simultaneously on a distributed temperature map[J]. IEEE Sensors Journal, 2021, 21(4): 4582-4589.
[88] Li J, Zhou X X, Xu Y, et al. Slope-assisted Raman distributed optical fiber sensing[J]. Photonics Research, 2021, 10(1): 205-213.
[89] Wu H, Zhao C, Tang M. Super spatial resolution Raman distributed temperature sensing via deep learning[J]. IEEE Journal of Selected Topics in Quantum Electronics, 2022, 28(4): 5600108.
光纤传感器未来十年市场份额将达400亿元?[EB/OL]. (2017-09-07) [2023-08-11]. https://zhuanlan.zhihu.com/p/29143215.
[94] IFOS.com–R*Sense® DTS-01[EB/OL]. [2023-08-11]. https://www.ifos.com/products/rsense-dts-01/.
[102] 杭州山旭光电有限公司. 分布式光纤测温系统[EB/OL]. [2023-08-11]. http://hzsensys.com/showproduct/4.html.
Sensys. Distributed temperature sensor[EB/OL]. [2023-08-11]. http://hzsensys.com/showproduct/4.html.
[103] 浙江振东光电科技有限公司. ZD-2线型光纤感温火灾探测器[EB/OL]. [2023-08-11]. http://zdong.net/cpzx/cpnr?id=14.
[105] 苏州光格科技股份有限公司[EB/OL]. [2023-08-11]. http://www.agioe.com/index.php/product/show/artid/3.html.
AGIOE[EB/OL]. [2023-08-11]. http://www.agioe.com/index.php/product/show/artid/3.html.
[106] 无锡布里渊电子科技有限公司[EB/OL]. [2023-08-11]. http://www.buliyuan.com/?list_7/501.html.
Buliyuan[EB/OL]. [2023-08-11]. http://www.buliyuan.com/?list_7/501.html.
[107] 武汉理工光科股份有限公司[EB/OL]. [2023-08-11]. http://www.wutos.com/product/9.html.
WUTOS[EB/OL]. [2023-08-11]. http://www.wutos.com/product/9.html.
[108] Schenato L. A review of distributed fibre optic sensors for geo-hydrological applications[J]. Applied Sciences, 2017, 7(9): 896.
[109] Yan J F, Shi B, Zhu H H, et al. A quantitative monitoring technology for seepage in slopes using DTS[J]. Engineering Geology, 2015, 186: 100-104.
[110] Khan A A, Vrabie V, Mars J I, et al. A source separation technique for processing of thermometric data from fiber-optic DTS measurements for water leakage identification in dikes[J]. IEEE Sensors Journal, 2008, 8(7): 1118-1129.
[111] Khan A A, Vrabie V, Mars J I, et al. Automatic monitoring system for singularity detection in dikes by DTS data measurement[J]. IEEE Transactions on Instrumentation and Measurement, 2010, 59(8): 2167-2175.
[112] Khan A A, Vrabie V, D'Urso G, et al. Blind source separation techniques for percolation type leakage detection in dikes using fiber optic DTS signals[J]. Proceedings of SPIE, 2008, 7004: 70042N.
[113] Su H Z, Kang Y Y. Design of system for monitoring seepage of levee engineering based on distributed optical fiber sensing technology[J]. International Journal of Distributed Sensor Networks, 2013, 9(12): 358784.
[114] AuflegerM, GoltzM, DornstädterJ, et al. Distributed fiber optic temperature measurements in embankment dams with central core: new benchmark for seepage monitoring[M]//Dams and reservoirs under changing challenges. Boston: CRC Press, 2011: 107-114.
[115] AuflegerM, GoltzM, ConradM. Distributed fibre optic temperature measurements–a competitive alternative for temperature monitoring in large RCC dams[C]//Proceedings of the 5th International Symposium on RCC Dams. 2007: 2-4.
[116] Su H Z, Tian S G, Cui S S, et al. Distributed optical fiber-based theoretical and empirical methods monitoring hydraulic engineering subjected to seepage velocity[J]. Optical Fiber Technology, 2016, 31: 111-125.
[117] Bersan S, Koelewijn A R, Simonini P. Effectiveness of distributed temperature measurements for early detection of piping in river embankments[J]. Hydrology and Earth System Sciences, 2018, 22(2): 1491-1508.
[118] Wang C H, Chen J H, Wang J, et al. Flume testing of seepage velocity monitoring using optic fiber distributed temperature sensing for embankments[J]. Sensor Review, 2016, 36: 120-129.
[120] Khan A A, Vrabie V, Beck Y L, et al. Monitoring and early detection of internal erosion: distributed sensing and processing[J]. Structural Health Monitoring, 2014, 13(5): 562-576.
[121] RadzickiK, BonelliS. Thermal seepage monitoring in the earth dams with impulse response function analysis model[C]//8th ICOLD European Club Symposium, September 22-25, 2010, Innsbruck, Austria. [S.l.: s.n.], 2010: 624-629.
[122] CôtéA, CarrierB, LeducJ, et al. Water leakage detection using optical fiber at the peribonka dam[C]//7th FMGM 2007: Field Measurements in Geomechanics, September 24-27, 2007, Boston, Massachusetts, USA. Reston: American Society of Civil Engineers, 2007: 1-12.
[123] GoltzM. A Contribution to monitoring of embankment dams by means of distributed fibre optic measurements[M]. Innrain: Innsbruck University Press, 2012.
[124] Wang L B, Narasimman S C, Reddy Ravula S, et al. Water ingress detection in low-pressure gas pipelines using distributed temperature sensing system[J]. IEEE Sensors Journal, 2017, 17(10): 3165-3173.
[125] Xu Y, Li J, Zhang M J, et al. Pipeline leak detection using Raman distributed fiber sensor with dynamic threshold identification method[J]. IEEE Sensors Journal, 2020, 20(14): 7870-7877.
[126] Kim H, Lee J, Kim T, et al. Advanced thermal fluid leakage detection system with machine learning algorithm for pipe-in-pipe structure[J]. Case Studies in Thermal Engineering, 2023, 42: 102747.
[127] Liu G, Meng H R, Qu G H, et al. Real-time monitoring and prediction method of commercial building fire temperature field based on distributed optical fiber sensor temperature measurement system[J]. Journal of Building Engineering, 2023, 70: 106403.
[128] Ouyang J S, Chen X M, Huangfu Z H, et al. Application of distributed temperature sensing for cracking control of mass concrete[J]. Construction and Building Materials, 2019, 197: 778-791.
[129] Selker J S, Thévenaz L, Huwald H, et al. Distributed fiber-optic temperature sensing for hydrologic systems[J]. Water Resources Research, 2006, 42(12): W12202.
[130] Vogt T, Schneider P, Hahn-Woernle L, et al. Estimation of seepage rates in a losing stream by means of fiber-optic high-resolution vertical temperature profiling[J]. Journal of Hydrology, 2010, 380(1/2): 154-164.
[131] Steele-Dunne S C, Rutten M M, Krzeminska D M, et al. Feasibility of soil moisture estimation using passive distributed temperature sensing[J]. Water Resources Research, 2010, 46(3): W03534.
[132] Ciocca F, Lunati I, Van de Giesen N, et al. Heated optical fiber for distributed soil-moisture measurements: a lysimeter experiment[J]. Vadose Zone Journal, 2012, 11(4): 0199.
[133] SaydeC, SelkerJ, EnglishM. Measuring soil moisture in a heterogeneous field[C]//World Environmental and Water Resources Congress 2009, May 17-21, 2009, Kansas City, Missouri, USA. Reston: American Society of Civil Engineers, 2009: 1-10.
[134] Liu G, Meng H R, Qu G H, et al. Distributed optical fiber sensor temperature dynamic correction method based on building fire temperature-time curve[J]. Journal of Building Engineering, 2023, 68: 106050.
[135] Yilmaz G, Karlik S E. A distributed optical fiber sensor for temperature detection in power cables[J]. Sensors and Actuators A: Physical, 2006, 125(2): 148-155.
[136] 郑晓亮, 胡业林. 基于分布式光纤测温技术的井下电缆温度监测系统设计[J]. 煤炭工程, 2009, 41(9): 19-21.
Zheng X L, Hu Y L. The temperature monitoring system of underground power cable based on distributed optic fibre sensing technology[J]. Coal Engineering, 2009, 41(9): 19-21.
[137] 周芸, 杨奖利. 基于分布式光纤温度传感器的高压电力电缆温度在线监测系统[J]. 高压电器, 2009, 45(4): 74-76, 81.
Zhou Y, Yang J L. Online temperature monitoring system for high voltage power cable based on distributed optical fiber temperature sensors[J]. High Voltage Apparatus, 2009, 45(4): 74-76, 81.
[138] Datta A, Mamidala H, Venkitesh D, et al. Reference-free real-time power line monitoring using distributed anti-Stokes Raman thermometry for smart power grids[J]. IEEE Sensors Journal, 2020, 20(13): 7044-7052.
[139] Yu T, Ren C G, Jia Y B, et al. Photovoltaic panel temperature monitoring and prediction by Raman distributed temperature sensor with fuzzy temperature difference threshold method[J]. IEEE Sensors Journal, 2021, 21(1): 373-380.
[140] de Pelegrin J, Dreyer U J, Bazzo J P, et al. Faults diagnosis in induction motors through thermal mapping produced by the RDTS system[J]. IEEE Sensors Journal, 2021, 21(18): 20061-20068.
[141] Duan R. Real-time hotspot tracing and model analysis of a distributed optical fiber sensor integrated power transformer[J]. IEEE Access, 2022, 10: 57242-57254.
[142] Bolognini G, Hartog A. Raman-based fibre sensors: trends and applications[J]. Optical Fiber Technology, 2013, 19(6): 678-688.
[143] Sun M, Tang Y Q, Yang S, et al. Fire source localization based on distributed temperature sensing by a dual-line optical fiber system[J]. Sensors, 2016, 16(6): 829.
[144] OdicR M, JonesR I, TatamR P. Distributed temperature sensor for aeronautic applications[C]//2002 15th Optical Fiber Sensors Conference Technical Digest. OFS 2002(Cat. No.02EX533), May 10, 2002, Portland, OR, USA. New York: IEEE Press, 2002: 459-462.
[145] 桑雷, 张杰, 王信群. 分布式光纤感温火灾探测系统在地铁隧道的应用[J]. 工业安全与环保, 2013, 39(8): 52-55.
Sang L, Zhang J, Wang X Q. Application of distributed optical fiber temperature sensing fire detection system in one subway tunnel[J]. Industrial Safety and Environmental Protection, 2013, 39(8): 52-55.
[146] Peng G L, He J, Yang S P, et al. Application of the fiber-optic distributed temperature sensing for monitoring the liquid level of producing oil wells[J]. Measurement, 2014, 58: 130-137.
[147] Lee D, Park K, Lee C, et al. Distributed temperature sensing monitoring of well completion processes in a CO2 geological storage demonstration site[J]. Sensors, 2018, 18(12): 4239.
[148] Ricard L P, Pevzner R. Evaluation of sensitivity of downhole temperature estimates from distributed temperature sensing measurements[J]. Energy Procedia, 2018, 154: 106-111.
[149] ChertenkovM, DeliyaS V, SemikinD A, et al. Gas breakthrough detection and production monitoring from ICD screen completion on Lukoil’s korchagina field using permanently installed distributed temperature sensors[C]//SPE Annual Technical Conference and Exhibition, October 8-10, 2012, San Antonio, Texas, USA. Texas: OnePetro, 2012.
[150] GraysonS, GonzalezY J, EnglandK, et al. Monitoring acid stimulation treatments in naturally fractured reservoirs with slickline distributed temperature sensing[C]//SPE/ICoTA Coiled Tubing & Well Intervention Conference & Exhibition, March 24-25, 2015, The Woodlands, Texas, USA. Texas: OnePetro, 2015.
[151] Johny J, Amos S, Prabhu R. Optical fibre-based sensors for oil and gas applications[J]. Sensors, 2021, 21(18): 6047.
[152] Yamate T, Fujisawa G, Ikegami T. Optical sensors for the exploration of oil and gas[J]. Journal of Lightwave Technology, 2017, 35(16): 3538-3545.
[153] BrownG, KennedyB, MelingT. Using fibre-optic distributed temperature measurements to provide real-time reservoir surveillance data on Wytch farm field horizontal extended-reach wells[C]//SPE Annual Technical Conference and Exhibition, October 1-4, 2000, Dallas, Texas, USA. Texas: OnePetro, 2000.
[154] Ashry I, Mao Y, Wang B W, et al. A review of distributed fiber-optic sensing in the oil and gas industry[J]. Journal of Lightwave Technology, 2022, 40(5): 1407-1431.
[155] BaldwinC. Fiber optic sensors in the oil and gas industry: current and future applications[M]//Alemohammad H. Opto-mechanical fiber optic sensors. Amsterdam: Elsevier, 2018: 211-236.
[156] Sharma J, Santos O L A, Feo G, et al. Well-scale multiphase flow characterization and validation using distributed fiber-optic sensors for gas kick monitoring[J]. Optics Express, 2020, 28(26): 38773-38787.
[157] Zhu Z C, Chu C W, Bian H T, et al. An integration method using distributed optical fiber sensor and Auto-Encoder based deep learning for detecting sulfurized rust self-heating of crude oil tanks[J]. Journal of Loss Prevention in the Process Industries, 2022, 74: 104623.
[158] Zouboulis E, Renusch D, Grimsditch M. Advantages of ultraviolet Raman scattering for high temperature investigations[J]. Applied Physics Letters, 1998, 72(1): 1-3.
[159] Liu B, Yu Z H, Hill C, et al. Sapphire-fiber-based distributed high-temperature sensing system[J]. Optics Letters, 2016, 41(18): 4405-4408.
[160] Liu B, Buric M P, Chorpening B T, et al. Design and implementation of distributed ultra-high temperature sensing system with a single crystal fiber[J]. Journal of Lightwave Technology, 2018, 36(23): 5511-5520.
[161] Liu X, Jie R M, Bera S, et al. High-speed and high-resolution YAG fiber based distributed high temperature sensing system empowered by a 2D image restoration algorithm[J]. Optics Express, 2023, 31(4): 6170-6183.
[162] Mandal S, Dekate S, Lee B K, et al. Characterization and calibration of Raman based distributed temperature sensing system for 600 ℃ operation[J]. Proceedings of SPIE, 2015, 9491: 94910A.
[163] Laarossi I, Ruiz-Lombera R, Quintela M A, et al. Ultrahigh temperature Raman-based distributed optical fiber sensor with gold-coated fiber[J]. IEEE Journal of Selected Topics in Quantum Electronics, 2017, 23(2): 296-301.
[164] Zhang J C, Wei P, Liu Q B. Monitoring a heatsink temperature field using Raman-based distributed temperature sensor in a vacuum and -173 ℃ environment[J]. Sensors, 2019, 19(19): 4186.
[165] Gorshkov B G, Gorshkov G B, Zhukov K M. Distributed fibre-optic temperature sensor for cryogenic applications based on detection of boson components of Raman light scattering[J]. Quantum Electronics, 2020, 50(5): 506-509.
[166] Silva M S P E, de Barros T H C, Alves H P, et al. Evaluation of fiber optic Raman scattering distributed temperature sensor between-196 and 400 ℃[J]. IEEE Sensors Journal, 2021, 21(2): 1527-1533.
[167] Fernandez A F, Rodeghiero P, Brichard B, et al. Radiation-tolerant Raman distributed temperature monitoring system for large nuclear infrastructures[J]. IEEE Transactions on Nuclear Science, 2005, 52(6): 2689-2694.
[168] Toccafondo I, Nannipieri T, Signorini A, et al. Raman distributed temperature sensing at CERN[J]. IEEE Photonics Technology Letters, 2015, 27(20): 2182-2185.
[169] Morana A, Girard S, Marin E, et al. Performances of radiation-hardened single-ended Raman distributed temperature sensors using commercially available fibers[J]. IEEE Transactions on Nuclear Science, 2020, 67(1): 305-311.
[170] Wang H H, Wang X, Tuo X G, et al. Experimental research on a Raman-based distributed temperature sensor assisted by PCA for locating the temperature abnormal event of nuclear waste drums[J]. Applied Optics, 2020, 59(2): 579-588.
Article Outline
介瑞敏, 肖春, 刘旭, 朱琛, 饶云江, 刘波. 拉曼分布式光纤温度传感:技术发展与应用综述[J]. 光学学报, 2024, 44(1): 0106011. Ruimin Jie, Chun Xiao, Xu Liu, Chen Zhu, Yunjiang Rao, Bo Liu. Raman Distributed Optical Fiber Temperature Sensing: Review of Technology and Applications[J]. Acta Optica Sinica, 2024, 44(1): 0106011.